the Creative Commons Attribution 4.0 License.
the Creative Commons Attribution 4.0 License.
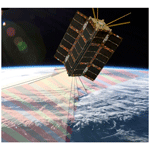
Opinion: Aerosol remote sensing over the next 20 years
Robert C. Levy
J. Vanderlei Martins
More than 2 decades ago, aerosol remote sensing underwent a revolution with the launch of the Terra and Aqua satellites. Advancement continued via additional launches carrying new passive and active sensors. Capable of retrieving parameters characterizing aerosol loading, rudimentary particle properties and in some cases aerosol layer height, the satellite view of Earth's aerosol system came into focus. The modeling communities have made similar advances. Now the efforts have continued long enough that we can see developing trends in both the remote sensing and modeling communities, allowing us to speculate about the future and how the community will approach aerosol remote sensing 20 years from now. We anticipate technology that will replace today's standard multi-wavelength radiometers with hyperspectral and/or polarimetry, all viewing at multiple angles. These will be supported by advanced active sensors with the ability to measure profiles of aerosol extinction in addition to backscatter. The result will be greater insight into aerosol particle properties. Algorithms will move from being primarily physically based to include an increasing degree of machine-learning methods, but physically based techniques will not go extinct. However, the practice of applying algorithms to a single sensor will be in decline. Retrieval algorithms will encompass multiple sensors and all available ground measurements in a unifying framework, and these inverted products will be ingested directly into assimilation systems, becoming “cyborgs”: half observations, half model. In 20 years we will see a true democratization in space with nations large and small, private organizations, and commercial entities of all sizes launching space sensors. With this increasing number of data and aerosol products available, there will be a lot of bad data. User communities will organize to set standards, and the large national space agencies will lead the effort to maintain quality by deploying and maintaining validation ground networks and focused field experiments. Through it all, interest will remain high in the global aerosol system and how that system affects climate, clouds, precipitation and dynamics, air quality, the environment and public health, transport of pathogens and fertilization of ecosystems, and how these processes are adapting to a changing climate.
- Article
(539 KB) - Full-text XML
- BibTeX
- EndNote
As NASA's Terra and Aqua satellites launched at the end of 1999 and in mid-2002, respectively, they ushered in a new era of aerosol remote sensing. These satellites carrying the MODerate resolution Imaging Spectroradiometer (MODIS) (Salomonson et al., 1989) and the Multiangle Imaging SpectroRadiometer (MISR, on Terra only) (Diner et al., 1998) offered an unprecedented view of the global aerosol system (Kaufman et al., 2002). The products derived from MODIS and MISR observations defined an aerosol climatology (Remer et al., 2008; Voss and Evan, 2020), identified regional trends (Yu et al., 2020; Zhang and Reid, 2010; Hammer et al., 2020), advanced understanding of aerosol direct radiative forcing (Christopher and Zhang, 2002; Zhang and Christopher, 2003; Yu et al., 2006; Remer and Kaufman, 2006; Bellouin et al., 2008; Li et al., 2016) and aerosol indirect radiative forcing (Peng et al., 2016), discovered associations between aerosols, clouds and precipitation that shifted our perception of the water cycle (Kaufman et al., 2005; Koren et al., 2005, 2008; Yuan et al., 2011a, b; Niu and Li, 2012), proved the importance of aerosol intercontinental transport and nutrient deposition (Y. Gao et al., 2001; Yu et al., 2013, 2019), and gave us important new tools to determine aerosol plume heights (Kahn et al., 2007, 2008; Val Martin et al., 2018) and air quality monitoring and mitigation (Engel-Cox, 2004; Van Donkelaar et al., 2006, 2016; Gupta and Christopher, 2009a, b).
During this period, aerosol remote sensing progressed as a discipline. The MODIS and MISR algorithms had to run in an operational environment, which meant they had to work for all types of aerosols and in all types of environments. The MODIS and MISR algorithms are rooted in the physics of radiative transfer and the mathematics of inversions, but they also incorporate empirical assumptions that tie results to the real world (Kaufman et al., 1997; Tanré et al., 1997; Martonchik et al., 1998, 2009; Kahn et al., 2001). Assumptions are necessary because of the fundamental lack of information content in the MODIS and MISR observations (Tanré et al., 1996; Kahn et al., 1998). MODIS provides a multispectral look at each Earth scene at a single viewing geometry. The original MODIS aerosol algorithms, Dark Target (Remer et al., 2005) and Deep Blue (Hsu et al., 2006), make use of no more than six wavelengths for their retrieval, although they use additional wavelengths for cloud masking and other peripheral needs. The MODIS Dark Target over ocean retrieval makes use of the most spectral bands, six. These six bands contain only two to three pieces of independent aerosol information (Tanré et al., 1996). Over land, fewer bands are used, and the information content available to characterize aerosol is even less (Levy et al., 2007). The MISR employs fewer wavelengths, only four from 0.486 to 0.867 µm, but adds significant additional information by viewing each scene from nine different angles. The MISR's angular information has proven to be immensely valuable in constraining aerosol type and in determining aerosol plume height (Kahn et al., 2007, 2008; Val Martin et al., 2018; Kahn and Gaitley, 2015).
However, in addition to the advances leading to the global satellite retrievals in an operational setting, these products also had to be validated and interpreted. The development of MODIS, MISR, and then the active sensors led to significant advances in ground-based remote sensing (e.g., AERONET; see Holben et al., 1998, for validation and algorithm improvements). At the same time, they also led to advances in the modeling and assimilation communities. By providing accurate global products across all types of environments, now models have (a) something to compare with and (b) something to assimilate.
MODIS and MISR created a revolution in aerosol remote sensing when they were launched in 1999, but the reasons for the revolution involved more than new satellite technology married to state-of-the-science algorithms. A combination of factors came together at just the right time. These factors include the following.
-
A policy for open data access
-
An investment in infrastructure for processing algorithms and distributing data
-
A commitment to long-term science teams for maintenance, improvement, and validation of data products including on-orbit sensor calibration
-
The vision and continuous support of the AERONET program for product validation (Holben et al., 1998)
-
The overall growth of the international aerosol community and expanded interest in the global aerosol system as part of Earth system science.
We find the first bullet point of particular interest for the celebration of the 20th anniversary of the journal Atmospheric Chemistry and Physics, which itself propelled a revolution in open access and information sharing at roughly the same time.
Thus, the lessons learned from the previous 20 years in aerosol remote sensing are that technical innovation leads to more observable information that leads to more complete aerosol characterization at higher accuracy. However, that chain of advancement will only seed new scientific endeavors if the aerosol products are accessible, validated, and accompanied by documentation that a user can actually understand and that is part of a larger data effort to characterize not only the global aerosol system, but also the Earth system as a whole. The infrastructure created for MODIS and MISR (LAADS DAAC, 2023; ASDC, 2023) continued to absorb and produce data products derived from other sensors and missions over the past 2 decades: the Aura mission, Cloud-Aerosol Lidar with Orthogonal Polarization (CALIOP) data from Cloud-Aerosol Lidar and Infrared Pathfinder Satellite Observations (CALIPSO), and the Polarization and Anisotropy of Reflectances for Atmospheric Sciences coupled with Observations from a Lidar (PARASOL) mission. Other agencies adopted NASA's open-access policies for their missions and products: the Visible Infrared Imaging Radiometer Suite (VIIRS), Himawari, Sentinels, and the Geostationary Ocean Color Instrument (GOCI). Today, a user needing local or global aerosol characterization may feel overwhelmed by the number of aerosol products available to use, both those derived directly from satellite observations and those inferred from models that use satellite information in assimilation systems (Zhang et al., 2008; Benedetti et al., 2009; Gelaro et al., 2017). The perspective from 2023 is that of an apparently data-rich era that could hardly have been imagined 23 years ago, when Terra was launched.
However, what will aerosol remote sensing and data products look like over the next 20 years?
The future of aerosol remote sensing will be propelled by advances in satellite payload technology. Instead of imaging multi-wavelength radiometers, like MODIS, we will expect the additional angular information pioneered by MISR plus the advantages of polarization. Imaging multi-wavelength multi-angle polarimeters were introduced to the community with POLarization and Directionality of the Earth's Reflectances (POLDER)/PARASOL (Deuzé et al., 2000, 2001; Chen et al., 2020). Future imaging polarimeters will improve upon the POLDER/PARASOL technology with better polarization accuracy, hyperspectral and hyper-angle capabilities, decreases in pixel size, and increases in the number of wavelengths with polarization and still allow for imaging. Super polarimeters with all of this technology in a single instrument are not necessary. Individual missions will tailor their instrument characteristics to meet particular mission needs, but these types of instrument advancement will be available for mission design. The Multi-viewing, Multi-channel, Multi-polarisation imaging mission (3MI) is the European follow-on to POLDER/PARASOL that will fly on EUMETSAT's second-generation polar-orbiting Meteorological Observing satellite series (MetOp SG) (Fougnie et al., 2018). The first of this series is planned for launch in 2024. The HyperAngle Rainbow Polarimeter 2 (HARP2) and the Spectro-polarimeter for Planetary Exploration One (SPEXone) will both fly on NASA's Plankton, Aerosol, Clouds, ocean Ecosystem (PACE) mission to be launched in 2024 (Werdell et al., 2019), and the Multi Angle Imager for Aerosols (MAIA: Diner et al., 2018) is scheduled for launch soon after. China has the Directional Polarized Camera (DPC) already in orbit aboard the Daqi-1 (DQ-1) satellite, with similar sensors repeated to launch until 2033. Finally, India is planning an aerosol mission, Nanosatellite for Earth Monitoring and Observation-Aerosol Monitoring (NEMO-AM), which will carry a multiangle polarimeter, the Multi Angle Dual Polarisation Imaging sensor (MADPI).
Similar instruments, with extended wavelength ranges, are planned for NASA's Atmospheric Observing System (AOS, 2023) launching later this decade. Beyond the AOS, we expect more hyperspectral capability, broader wavelength ranges including the thermal infrared, finer spatial resolution, broader swaths, and more angles. Twenty years from now these imaging multi-angle and hyperspectral polarimeters will be the norm for passive aerosol-characterizing sensors. Simple single-view multi-wavelength radiometers will still be flying through the 2030s, as they offer products across multiple disciplines, i.e., the Visible InfraRed Suite (VIIRS), Ocean and Land Colour Instrument (OLCI), MultiSpectral Instrument (MSI), and Medium Resolution Spectral Imager (MERSI), but the cutting edge aerosol characterization will have moved to the polarimeters by the end of the 2030s. The exception for radiometers will be the geostationary fleet of multispectral radiometers that we discuss below.
In the thermal infrared, single-view instruments will still have a role (DeSouza-Machado, 2010; Klüser et al., 2011). Hyperspectral thermal infrared spectrometers such as the Atmospheric Infrared Sounder (AIRS) have been used to detect and quantify dust aerosol optical depth (AOD) and layer height beginning with the Aqua mission (DeSouza-Machado et al., 2006) and advancing to other hyperspectral sounders in this spectral range, e.g., the Infrared Atmospheric Sounding Interferometer (IASI) (Clarisse et al., 2019). The community has recently directed greater focus to dust's contribution to Earth's energy balance through its perturbation of the radiation field at infrared wavelengths (Zheng et al., 2022; Song et al., 2022). New technology for hyperspectral thermal infrared spectrometers will improve signals and reduce spatial footprints and are our best hope for achieving mineral speciation of airborne dust from space.
Along with advances in passive remote sensing imagers, we expect new capabilities in active lidars. The straightforward backscatter lidar in space (CALIOP) has demonstrated the unique aerosol information that only a lidar can provide. By 2043 standard aerosol missions will include lidars, and these lidars will surpass CALIOP with additional wavelengths, high-spectral-resolution lidar (HSRL) and/or Raman capability, as well as multiple beams to expand coverage. Ground-based and airborne lidars with these enhanced capabilities are demonstrating that, like polarimetry, the information content from enhanced lidar design allows for aerosol characterization in ways previously unattainable (Müller et al., 2014). Post-CALIOP lidars flying now or expected in the near future include the Chinese Aerosol and Carbon Detection Lidar (ACDL; Ke et al., 2022) and the European ATmospheric backscatter LIDar (ATLID; Groß et al., 2015). Both have HSRL capability. These are small steps forward, and we understand the expense of launching high-capability lidars in space, as the HSRL planned for NASA's AOS mission has recently been descoped because of cost. However, we remain optimistic. By 2043, these modest steps into lidar capability expansion could grow to achieve the ideal for aerosol characterization (Müller et al., 2014). This means that there could be at least one space payload offering three wavelengths of backscattering, two wavelengths of extinction profiles using HSRL technology, and one wavelength providing depolarization. Aerosol scientists have been advocating for this array of lidar payload capability for more than a decade, and despite the next set of planned aerosol missions falling short, we foresee the dream becoming reality in 20 years.
The lessons we learned from the past 20 years in terms of the advantages of formation flying will guide mission planning far into the future. Like the A-Train of the 2000s and 2010s, missions will be constructed to purposely match passive polarimeters with active lidars in ways that promote synthesized data processing, algorithms, science, and applications. The workhorse Sun-synchronous polar-orbiting mission that has been the mainstay of aerosol characterization will be supplemented with inclined orbits that have been more typical of precipitation-centered missions but that have much to offer for aerosols. Inclined orbits allow for a multitude of geometries and time-of-day samplings of the aerosol system over the course of weeks as well as multiple coincidences a day, with other satellites looking simultaneously at the same target at the ground. This provides a look into aerosol situations never encountered by MODIS, MISR, or their ilk. Part of this expanding constellation of aerosol observations from satellites will be geostationary (GEO) imagers. Capability from GEO platforms has already evolved from the two or three broad uncalibrated wavelengths that were ubiquitous at the time of Terra launch into multi-wavelength moderate-resolution sensors that mimic most of MODIS' capabilities. By 2043, these modern-day Advanced Baseline Imagers (ABIs) and the Advanced Himawari Imager (AHI), FengYu (FY), and Meteosat Third Generation (MTG) will be upgraded with spectrometers as per the NASA–NOAA joint Geostationary Extended Observations (GEO-XO) program (Frost et al., 2020). At that time we expect studies in progress to adapt polarimeters to GEO orbits. Other GEO missions include the Geostationary Extended Observations Imager (GXI), Flexible Combined Imager (FCI), Geostationary High speed Imager (GHI), and Advanced Geostationary Radiation Imager (AGRI). Lidar at that altitude may still be pending. With the Equator crowded with observatories in GEO orbit, overlap between sensors provides both a means to cross-calibrate and also multi-angle looks at each scene to increase the information content for aerosol retrieval (Bian et al., 2021).
Table 1 presents a partial list of planned space missions from the national agencies of the US, Europe, and China that have identified an aerosol objective. The list is not comprehensive by any measure, as many missions not specifically designed to characterize aerosol find a means to do so anyway. For example, the Earth surface Mineral dust source Investigation (EMIT) mission will advance characterization of dust aerosol by identifying the mineral content of dust on the ground before the particles are airborne. EMIT will help with aerosol characterization but is not included here because it is not designed to retrieve airborne aerosol parameters. Table 1 is also missing a comprehensive list of missions because many additional countries are finding resources to launch space observatories as space becomes more democratized, as we will discuss below. India, Japan, and Korea are already planning for future aerosol-capable missions in the next decades.
Table 1Partial list of planned missions from major space agencies in the US, China, and Europe and one each from India and South Korea being aerosol-objective or aerosol-capable without a specific objective. Compiled from WMO OSCAR (2011–2023).
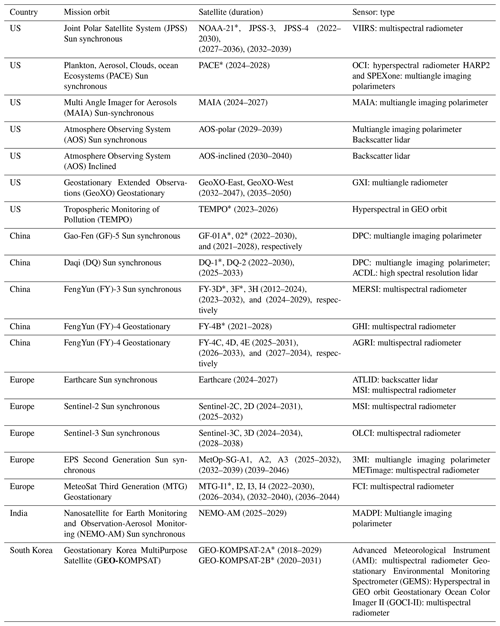
∗ Already launched and in orbit.
The tag line for technology in 2043 will be more, more, more! Each sensor will be collecting orders of magnitude more data than MODIS does today. Polarized measurements require 3 times the data per wavelength than a simple radiometer to account for the three angles of polarization. Multiply that increase by the number of view angles beyond the single MODIS-like view, and we are likely now producing 15 to 60 times the number of data being produced today. The implementation of hyperspectral sensors or improvements in pixel size while maintaining broad swaths to view the entire globe further increases the data volume. Advancing lidars with additional wavelengths, HSRL, multiple beams, and quicker pulses also magnifies data volume quickly. A part of the technology evolution will be the need for spacecraft and especially spacecraft electronics, data systems, and transmission to keep up with data volumes. Optical transmission will replace radio frequencies, and infrastructure in space will serve as a way for stations to stage downlinking to overworked ground stations. For example, today the Ka band is the radio frequency of choice for satellite missions. NASA is promoting “new and improved” Ka-band technology available for licensing that achieves a data rate of 130 megabits per second (130 Mbps) (NASA Technology Solution Communications, 2023). In parallel, NASA recently announced the achievement of 200 gigabytes per second (Gbps) with a space-to-ground optical link (NASA Press Release, 2023), i.e., 3 orders of magnitude more data coming down with an optical system. On Earth, computing power will be fully cloud-based and ever expanding to hold the proliferation of collected data.
Today, the majority of aerosol retrieval algorithms interpret the signal measured by the satellite sensor in terms of radiative transfer through the atmosphere. The process is governed by this equation:
ρTOA is the solar radiance reflected by the Earth system measured at the top of the atmosphere by the satellite sensor, ρa is the contribution by the atmosphere alone, ρs is the reflectance of Earth's surface that transmits through the atmosphere with transmittance, T, and s is the spherical albedo with the (1−sρs) term representing multiple scattering between the atmosphere and Earth's surface. Each term is for specific wavelength, Sun, and sensor geometries, but that notation has been omitted for simplicity. The terms depend on surface properties (ρs and s) and the amount and optical properties of the gases and aerosols in the atmosphere (ρa and T). By making physically based assumptions about some surface, gas, and aerosol properties and calculating ρTOA, the calculated ρTOA can be compared with the measured ρTOA and assumptions adjusted until the calculated and measured ρTOA values are the same to within an acceptable error, consistent with measurement uncertainty.
The simplest physically based algorithms use one wavelength, one geometry at a time. These simple retrievals keep all assumptions constant except for the optical measure of aerosol loading, the AOD. Thus, the retrievals use one piece of information to retrieve one parameter, the AOD. By minimizing the difference between measured and calculated ρTOA simultaneously for more than one wavelength, more than one angle, or more than one polarization state, more information is introduced, and additional assumptions can become free parameters, leading to retrievals of aerosol parameters beyond AOD. For example, the MODIS dark target retrieval is based on the knowledge that independent, multispectral observations provide more information. However, not all wavelengths are completely independent, so that seven MODIS wavelengths from 0.47 to 2.1 µm yield, at most, three pieces of information (Tanré et al., 1996). Likewise, additional geometries and/or polarization states can be introduced, freeing even more parameters for retrieval. The simplest algorithms have employed radiative transfer models run in the forward direction with assumed aerosol, gas, and surface parameters, resulting in a look-up table (LUT) stored for future use. The retrieval can then move quickly through the LUT, interpolating between entries, and return the retrieved parameters in an operational setting.
Today, as information content increases and computer power grows to meet demand, LUTs are being supplemented by other methods to solve the radiative transfer equation. Techniques are being used to simultaneously retrieve multiple aerosol, gas, and surface parameters. These more flexible algorithms provide an alternative framework for retrieval, but they are still physically based on radiative transfer. As we march towards 2043, the LUT methods will be permanently jettisoned. The reason is that radiative transfer will become so sophisticated with better representation of aerosol optical properties that we would need an infinitely large LUT. Optimal estimation avoids LUT limitations but requires an initial guess and the risk of finding local minima. With parallel improvements (and greater sampling) of ground-based and airborne observations including in situ sampling, more properties can be constrained. The in situ measurements are especially important for characterizing irregularly shaped particles. Algorithms will be designed to ingest data from multiple collocated sensors, especially combinations of passive and active sensors (Liu et al., 2017) and combinations of spaceborne and ground-based sensor observations (Lopatin et al., 2013; Li et al., 2019). We expect joint retrievals of aerosol and trace gases, aerosol and land properties, aerosol and ocean color properties, and aerosol–gas–surface retrievals. Eventually there will be sufficient information by 2043 for retrieved properties to include vertical profiles of aerosol extinction and particle number concentration (Schlosser et al., 2022) as well as retrievals over clouds (Torres et al., 2012; Jethva et al., 2013) and over all land surface types, including snow and ice (Shi et al., 2019; Mei et al., 2020; Zhang et al., 2023). Characterization will include particle spectral absorption (Xu et al., 2021) and make progress towards constraining particle composition (Kacenelenbogen et al., 2022).
By 2043, although radiative transfer and scattering processes will be better understood, completely physically based algorithms will be rare. Development of machine-learning (ML) retrieval algorithms will accelerate, such that there will be algorithms that produce a full array of aerosol characteristics by pumping satellite-measured quantities through ML-derived models. These models have no physical basis and do not rely on the radiative transfer equation (Eq. 1). Instead, the models are created by training on a formulation dataset that matches satellite-measured radiances with measured aerosol characteristics. For example, today we can train a ML model using satellite measurements collocated with AERONET observations (Lary et al., 2009; Di Noia and Hasekampf, 2018; Kang et al., 2022). In this way we can obtain aerosol characteristics such as AOD that match AERONET with great accuracy and precision (Lary et al., 2009), but we lose all physical understanding of how that AOD is retrieved. The challenge for exclusive ML methods like these cited will be the identification of accurate measurements of aerosol characteristics, other than AOD, in sufficient quantities to train the ML models. However, ML algorithms will not recognize a physical situation that falls outside of its training dataset, and they still need to accommodate measurement uncertainty.
There are other uses of ML in aerosol remote sensing besides directly obtaining aerosol parameters from satellite observations. Within a physically based algorithm there are many assumptions that can be aided by ML methods. For example, running a radiative transfer model is computationally expensive. A ML model derived from radiative transfer runs will be more accurate than current interpolations of LUTs and save time in an operational setting (Gao et al., 2021; Ukkonen, 2022). In other work, ML methods are used to classify specific aerosol types, such as dust by training on other satellite datasets (CALIPSO) (Lee et al., 2021). ML is used to bias-correct aerosol products derived using standard methods (Lipponen et al., 2022), and it can help with interpolation, constraining non-retrievable aerosol optical properties, cloud (Wei et al., 2020) or snow masking, and surface reflectance determination (Su et al., 2020). In 2043 aerosol retrieval algorithms will continue to be physically based, but when examined carefully, they will contain many elements of indispensable ML within.
The biggest noticeable change over the next 20 years will be the gradual extinction of “satellite aerosol products”. The entire purpose of what we know now as a satellite data product will be to feed major assimilation systems that produce a complete representation of the global aerosol system within the context of the complex Earth system (Zhang et al., 2008; Benedetti et al., 2009; Gelaro et al., 2017). We already see the widespread acceptance of assimilation products, as noted by the number of citations earned by key papers describing each system. For example, using Clarivate's Web of Science database (Clarivate, referenced January 2023) and searching “satellite AND aerosol”, we find the first returned reference to be Holben et al. (1998), the seminal AERONET paper with 5265 citations accumulated over 24 years. Next up is Gelaro et al. (2017), the fundamental reference for the Modern-Era Retrospective Analysis for Research and Applications, Version 2 (MERRA-2) assimilation products, with 3270 citations accumulated over only 9 years. We understand that not all citations to MERRA-2 will involve applications to MERRA-2 aerosol products and that having a single fundamental reference concentrates users' citations to a single paper, but the rate of acceptance of MERRA-2 and other systems' assimilation products over the past 5 years is astounding. See Fig. 1.
Assimilation systems are analogous to the science fiction concept of a “cyborg”, defined by the Oxford Dictionary of Science Fiction as “A hybrid being: half human, half machine (a contraction of `cybernetic organism')” (Prucher, 2006). In our context, the assimilation systems are a hybrid of half observations, half models. Satellites measure electromagnetic radiation that is processed through aerosol retrieval algorithms to determine aerosol parameters. Assimilation systems intercept parameters from the retrieval algorithms during processing to ingest those data into a global model that adjusts model representation of aerosol parameters to agree with the observations. Some assimilation systems draw from the aerosol retrieval algorithms close to the satellite observations, making use of radiance or reflectance directly (Randles et al., 2017). Some systems ingest the final retrieval products such as AOD (Zhang et al., 2008). In all the cases the global model is drawn closer to actual measurements at regular time steps and is expected to represent the actual aerosol system better than a model that is initialized and run forward from aerosol source inputs. The advantage of assimilation aerosol products is that users can access aerosol parameters for any time period, at any location, and at any altitude. The parameters will have the benefit of ALL available data from ALL satellites simultaneously. Users will not need to patch together products from different algorithms. The model does that for you and presents the results in an Earth system governed by physical restraints.
The disadvantages of assimilation are that the resulting aerosol field can be variable, changing abruptly with model time step or with the availability of different aerosol input fields. For example, there will be much more data available during the day than at night, which may create day–night discontinuities. Furthermore, by drawing the model nearer to the observed variables, there can be arbitrary introduction or subtraction of aerosol mass. This can create biases in mass budget parameters, such as mass deposition. Furthermore, model parameterizations such as chemical transformation and interaction with clouds remain uncertain and likely will remain uncertain in 2043. Nevertheless, assimilation datasets will become the norm for the majority of aerosol data product consumers. The assimilation systems will run in the cloud and will be called on to produce the cyborg data at a user's will.
We note that satellite-retrieved aerosol products can interact with models without being assimilated. They can serve as validation and identify and characterize aerosol point sources such as smoke and volcanic aerosols. Using satellite-derived datasets as separate, independent data can improve parameterization of aerosol processes within models. Such processes include chemical transformation and aerosol–cloud interactions. Such independent constraints on models have important implications for climate prediction and air quality applications (Kahn et al., 2022).
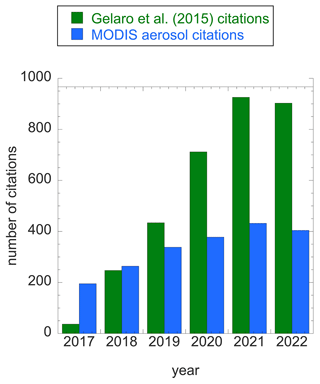
Figure 1Number of citations received by Gelaro et al. (2017), the fundamental reference for the NASA MERRA-2 assimilation system products (green) and the combination of citations received by Levy et al. (2013), Hsu et al. (2013), and Lyapustin et al. (2018), representing the Dark Target, Deep Blue, and MAIAC aerosol algorithm products for MODIS (blue). Source: Clarviate Web of Science database. The point is to note the rapid acceleration of MERRA-2 citations as an indicator of the speed of the research community's acceptance of assimilation products, while traditional retrieval products such as MODIS aerosol products maintain a consistent presence in the research community. We anticipate that, over the next 20 years, assimilation product use will continue to grow while use of standard products will plateau and decrease.
A gold rush to space has begun and will intensify over the next 20 years as space becomes crowded with a variety of sensors in various Earth orbits. During this period we will realize a true democracy in space as non-traditional players such as the space agencies from the developing world and from a wide range of commercial sector companies join traditional national agencies in blanketing the skies with satellites and sensors. While only a fraction of these satellite sensors will be Earth-viewing and aerosol-capable, the result will yield a tremendous volume of data useful for aerosol retrieval.
Satellites will come in all sizes. Traditional agencies will still launch large, expensive, and robust flagship missions with multiple platforms and multiple sensors on each platform. The flagship missions will continue demonstrating lower risk, higher accuracy, greater reliability, and longevity. The flagship missions will be supplemented by and integrated with constellations of smaller satellites and nano-sized cubesats. The smaller satellites will be orders of magnitude less expensive and thus riskier and with more limited capability than the larger observatories. However, the multitude of the smaller units renders the loss of any particular unit insignificant to the greater objectives. With so many satellites flying, observing Earth, and returning measurements for aerosol retrieval, each satellite will be seeking a particular niche. All orbit types will be covered, including polar-orbiting, inclined orbit, and geosynchronous, and near-real-time observations will be achieved.
The challenge of so many data sources is the maintenance of data quality. There will be a lot of data, and there will be a lot of bad data. Eventually the scientific and user communities will organize to set standards and protocols to regulate data quality. Such standards will rely on the cohesion of the data communities for enforcement. An example of community establishing standards that are enforced through peer review are the protocols published by the International Ocean Colour Coordinating Group (IOCCG) for satellite ocean color sensor validation. Not all data will be free and open access. Commercialization of data products will aid in enforcement of standards, as different entities will be competing to sell their products based on many characteristics, including quality. Evaluation of that quality may move into the hands of independent companies or coordinating groups. Commercial entities that launch satellites intend to produce specialized data products for specific customers. Sometimes these customers will be national agencies looking for input into their assimilation systems. At other times commercial entities will produce their own assimilation system cyborg products and sell those results to their customers.
Calibration will be a continuing concern with the proliferation of small and nano-satellite data flooding the market. As stated above, there will be a lot of bad data, much of them due to poor calibration of each individual sensor, as evidenced by today's fleet of mostly poorly calibrated small satellite sensors. This will change. Calibration takes many forms and includes radiometric, polarimetric, geometric, and spectral components, and each component requires its own intense scrutiny that not all satellite-launching entities are ready to provide. To mitigate some of the chaos, a commercial pre- and post-launch calibration industry will spring up. These companies will offer pre-launch calibration facilities and take responsibility for a sensor's calibration, even verifying that calibration using vicarious methods once the sensor is in orbit. Eventually, either governmental or space industry bodies will move to a certification process similar to the Underwriters Laboratories (UL) or Conformite Europeenne (CE) marks common today to denote adherence to a specified set of standards for electronic systems. There will also be a need for in-space calibration options, such as was proposed for the Climate Absolute Radiance and Refractivity Observatory (CLARREO) mission. CLARREO would maintain highly accurate, traceable reflectance measurements from Earth and the Moon through its lifetime, which could be transferred to other instruments in orbit.
Validation of aerosol products is another issue. With the democratization of space, the leading national space agencies will no longer hold a monopoly on space access. Ironically, instead, the government agencies that pioneered aerosol characterization from space will take the lead on suborbital measurements to support the validation side of producing high-quality aerosol products. Government-sponsored field experiments and ground networks will provide the basis for the entire international community, including the commercial sector, to evaluate their products and improve their algorithms.
Today's basis for validation of aerosol datasets is the ground-based AERONET network (Holben et al., 1998; Giles et al., 2019). The AERONET concept will continue for decades into the future, but the technology itself will evolve. Instruments will move from finite wavelength bands to hyperspectral spectrometers and will continue AERONET's current trend into the shortwave infrared. Originally, AERONET instruments had invested in polarimetry, which turned out to be a capability ahead of its time. The community has caught up, and AERONET will expand the polarimetric capabilities of their networks and incorporate polarimetry into their retrievals. Data capacity, storage, and transmission will expand, as will the network itself. AERONET's subsidiary, the Marine Aerosol Network (MAN), will continue to collect data on ocean-going cruises and ships of opportunity. The MAN instruments will also upgrade their technology and data capabilities. The AERONET retrieval that produces reliable aerosol characterization in addition to AOD, including particle size distribution, single scattering albedo, complex refractive indices, and non-sphericity, will become a more important asset over time as aerosol satellite remote sensing advances in information content, requiring evaluation of satellite-retrieved products that include a wide range of aerosol parameters. However, we note that, while the uncertainty in AERONET AOD is sufficiently small to offer true validation to a collocated satellite AOD product, the uncertainty of AERONET inversion products (particle size distribution, single scattering albedo, etc.) is not. The AERONET inversion products are themselves subject to assumptions and caveats and may have greater error than certain satellite parameters. Still, the inversion products provide a vital service in the evaluation of satellite products, providing a standardized framework of high-quality, widely distributed aerosol characterization for nearly immediate comparisons with satellite products.
The AERONET concept of a widespread, high-quality, and aerosol-observing ground network will proliferate, as we are already witnessing with the Interagency Monitoring of Protected Visual Environments (IMPROVE: Malm and Hand, 2007), the European Aerosol Research Lidar Network (EARLINET: Pappalardo et al., 2014), the Aerosol, Cloud and Trace gases Research Initiative (ACTRIS, 2023), the Surface Particulate Matter Network (SPARTAN: Snider et al., 2015), and other networks. The different networks offer different types of aerosol characterization necessary to keep up with the expansion of retrieved aerosol parameters from space. In situ measurements of aerosol aerodynamic properties will continue to be essential for the assessment of the aerosol health impact and to link aerodynamic and optical measurements, which form the basis for satellite products. Ground lidar networks (Welton et al., 2001; Pappalardo et al., 2014) assisted by improved airport ceilometers will expand high temporal characterization of the aerosol vertical profile. As satellite retrieval algorithms upgrade their radiative transfer, refine their assumptions, incorporate ML, and become cyborgs, so will the ground network retrieval algorithms. More and more often validation datasets will include the resulting aerosol characterization obtained by simultaneous retrieval of several collocated instruments. Ideally this will include passive measurements with collocated lidar and ground-level or balloon-borne in situ measurements.
Field experiments featuring aircraft-borne measurements will be indispensable for validating the satellite observations at the radiance or polarization level (Level 1) and the retrieved aerosol particle properties in regions and situations of high scientific interest or societal relevance. Aircraft fly in three dimensions, sampling situations between network stations and providing vertical profiles of parameters where lidar is absent. Field experiments will also be essential for confirming ground-based validation sites, as now these sites will be producing a suite of retrieved parameters that require their own validation. We will find that field experiment measurements will be the only truly independent dataset for validation. With the abundance of suborbital data, quality again becomes an issue, and national agencies will be the only community players with the resources to maintain high-quality, long-term validation datasets.
At the opposite end of the spectrum will be the proliferation of low-cost citizen science networks, including the use of smartphone imagery. These commercial networks will expand across populated areas and will contribute to characterizing the global aerosol system simply by the abundance of available observations. However, there will not be the same oversight to maintain the quality of these datasets, and users will need to calibrate the data from citizen scientist measurements with government-maintained and quality-assured sources.
We have described a vibrant global measurement system focused on aerosol remote sensing as we move forward into the next 20 years. This picture assumes a continuing need for aerosol characterization, which will be driven by pressing science questions and societal priorities. What will be the aerosol science questions of the coming decades?
Quantifying changes to the aerosol system will be essential as we continue to struggle to catch up to climate change. By 2043 we will have 70 years of Total Ozone Measuring System (TOMS), 60 years of AVHRR and 40 years of MODIS and VIIRS aerosol time series. While most of these time series offer insight into global and regional changes to aerosol loading, inferring changes to aerosol types and particle properties will be especially important.
We will still be working towards understanding aerosol–cloud–precipitation processes, how these have changed in time, and how these processes interact with atmospheric dynamics.
The role of aerosols in transporting nutrients around the globe and depositing them into terrestrial and oceanic ecosystems will continue to be a pressing issue in the coming decades. Likewise, the role of aerosols in transporting pathogens will still be under investigation in the coming years.
Maintaining healthy air quality for all the world's populations will continue to call for understanding of global aerosol distributions and characterization in a way that will require satellite remote sensing.
Underlying these issues will be the goal of using the characterization of the global aerosol system to make our planet healthier and environmentally just.
Despite today's assessment that we are “data-rich”, by 2043 we will look back at 2023 as a primitive data desert. Aerosol products that are actually cyborgs produced by assimilation systems will represent the global aerosol, producing near-real-time representation of aerosol fields at fine temporal and spatial scales. Assimilation aerosol product users will forget that clouds (real clouds, not data clouds) exist, as all the aerosol fields will be interpolated through traditionally cloudy scenes by the models. Likewise, assimilation aerosol data fields will have no gaps due to difficult surfaces such as Sun glint, snow, or ice. However, the challenge will be the quality checking and synthesis of so many data. There will be commercial aerosol data ambassadors who, for a fee, will create custom data packages from the overwhelming supply of public and private data product sources.
Today's retrieval algorithms will appear quaint to aerosol remote sensing scientists in 2043. The days of a single inversion applied to a single sensor will be in decline. Even physically based algorithms will be designed to invert the observations from multiple sensors and platforms simultaneously. This includes joint inversions between satellite and ground-based sensors to produce full characterization of atmospheric constituents (aerosols and gases) as well as complete characterization of the surface beneath whether that surface is a cloud, the ocean, or a snow field. The caveat with multi-sensor inversions is the difficulty of determining the propagation of error and uncertainty from sensors of various capabilities and calibrations.
Few people will care about aerosol optical depth. The assimilation systems will offer aerosol characterizations in forms most useful for individual users. Air quality managers will automatically obtain particulate mass concentration. Aerosol–cloud–precipitation scientists will obtain vertically resolved number concentration or heating rates due to aerosol absorption. Those interested in aerosol radiative forcing will find numbers (Wm−2) coming automatically out of some assimilation system. There will be attempts to produce deposition mass flux, but the error bars will still be large. Likewise, attempts to reliably retrieve chemical composition directly from remote sensing will likely fail, although aerosol type will be constrained very well. The only interest in aerosol optical depth will be users working towards continuity with the old sensors for long-term trend analysis or as a critical first step in validating a retrieval.
In 2043 validation networks must be in place, and major research efforts will switch from algorithm development to designing and implementing a strategy that reserves sufficient independent data for validation. If the assimilation is producing a cyborg vertically resolved distribution of particle number concentration near or in clouds, there needs to be a validation strategy to confirm and put error bars on that product. Investment will be needed to develop the instruments and platforms to implement a validation strategy. True validation of some difficult parameters such as vertically resolved particle number validation may be measured only infrequently during dedicated field campaigns. In some ways validation in 2043 may look like a step backward from what aerosol scientists have come to expect today. Instead of 1 million collocations that we achieve now when we validate AOD with AERONET, for some retrieved aerosol parameters, we will be satisfied with 10 to 50 validation points hard-earned in the field.
Where will the funding come from? From the same tax base that has propelled major space agency missions in the past but now directed more to assimilation systems, validation, quality assurance, and buying data from commercial entities who can sell it cheaper than the agencies can produce themselves. In addition, there will be private concerns, investors, and customers, who see value in data products tailored to particular concerns: the insurance industry, the agricultural industry, and local air quality jurisdictions.
In summary, 2043 will be an exciting time for aerosol remote sensing. There will be plenty of data for answering science questions and plenty of work to do to ensure the integrity of those data. We are sorry to say, though, that we cannot promise flying hover boards as seen in the Back To The Future movies (Zemeckis and Gale, 1989, Schildhause, 2014), even in 2043.
No computer code was developed for this Opinion. The one figure was plotted using commercial software package Kaleidagraph 5.01 from Synergy Software.
Citation data for the one figure (Fig. 1) were accessed from Clarivate Web of Science. Clarivate is a commercial entity and is not open source. The Web of Science accessible through the subscriptions of many research and academic institutions provides the quickest and easiest method to attribute the number of citations of particular articles. In this Opinion, the quick access and analysis of the citation statistics made a general point not essential to the main conclusions of the Opinion. Any reader interested in comparing similar citation results could satisfy their curiosity by using any citation database accessible to them. The authors are confident that the same general point can be made about the rise in the citations of assimilation datasets versus the relatively stable number of citations of traditional satellite aerosol products over the same time period.
The manuscript resulted from discussions between the three authors over a time span of years. LR wrote the initial draft. RL and JVM edited this draft and added their own insights. LR took responsibility for addressing reviewers' comments, with RL and JVM editing her responses and subsequent versions of the Opinion.
LR and JVM hold ownership stakes in GRASP SAS in addition to their primary employment at the University of Maryland Baltimore County. GRASP SAS is involved in the commercial small satellite industry and in producing aerosol remote sensing products from its own satellites and from publicly available satellite measurements. GRASP SAS's subsidiary, Airphoton Inc., designs, builds, and sells ground-based instruments for aerosol measurements and payloads for space.
Publisher’s note: Copernicus Publications remains neutral with regard to jurisdictional claims made in the text, published maps, institutional affiliations, or any other geographical representation in this paper. While Copernicus Publications makes every effort to include appropriate place names, the final responsibility lies with the authors.
This article is part of the special issue “20 years of Atmospheric Chemistry and Physics”. It is not associated with a conference.
The authors thank both reviewers, Ralph Kahn and Zhanqing Li, for their very thoughtful comments that caused us to push harder towards a defensible opinion. Many of the opinions of these reviewers were incorporated into our opinion in its final form, and we are proud to acknowledge them both by name.
This paper was edited by Xiaohong Liu and James Allan and reviewed by Ralph Kahn and Zhanqing Li.
ACTRIS (Aerosol, Cloud and Trace gases Research Initiative): Aerosol, Cloud and Trace gases Research Initiative webpage, https://www.actris.eu (last access: 29 May 2023), 2023.
AOS (Atmospheric Observation System): Atmospheric Observing System webpage, https://aos.gsfc.nasa.gov/mission.htm (last access: 5 February 2023), 2023.
ASDC (Atmospheric Science Data Center): Atmospheric Science Data Center webpage, https://asdc.larc.nasa.gov/about (last access: 5 February 2023), 2023.
Bellouin, N., Jones, A., Haywood, J., and Christopher, S. A.: Updated estimate of aerosol direct radiative forcing from satellite observations and comparison against the Hadley Centre climate model, J. Geophys. Res., 113, D10205, https://doi.org/10.1029/2007JD009385, 2008.
Benedetti, A., Morcrette, J. J., Boucher, O., Dethof, A., Engelen, R. J., Fisher, M., Flentje, H., Huneeus, N., Jones, L., Kaiser, J. W., and Kinne, S.: Aerosol analysis and forecast in the European centre for medium-range weather forecasts integrated forecast system: 2. Data assimilation, J. Geophys. Res.-Atmos., 114, D13205, https://doi.org/10.1029/2008JD011115, 2009.
Bian, Q., Kreidenweis, S., Chiu, J. C., Miller, S. D., Xu, X., Wang, J., Kahn, R. A., Limbacher, J. A., Remer, L. A., and Levy, R. C.: Constraining Aerosol Phase Function Using Dual-View Geostationary Satellites, J. Geophys. Res.-Atmos., 126, e2021JD035209, https://doi.org/10.1029/2021JD035209, 2021.
Chen, C., Dubovik, O., Fuertes, D., Litvinov, P., Lapyonok, T., Lopatin, A., Ducos, F., Derimian, Y., Herman, M., Tanré, D., Remer, L. A., Lyapustin, A., Sayer, A. M., Levy, R. C., Hsu, N. C., Descloitres, J., Li, L., Torres, B., Karol, Y., Herrera, M., Herreras, M., Aspetsberger, M., Wanzenboeck, M., Bindreiter, L., Marth, D., Hangler, A., and Federspiel, C.: Validation of GRASP algorithm product from POLDER/PARASOL data and assessment of multi-angular polarimetry potential for aerosol monitoring, Earth Syst. Sci. Data, 12, 3573–3620, https://doi.org/10.5194/essd-12-3573-2020, 2020.
Christopher, S. A. and Zhang, J.: Shortwave aerosol radiative forcing from MODIS and CERES observations over the oceans, Geophys. Res. Lett., 29, 6–1, https://doi.org/10.1029/2002GL014803, 2002.
Clarivate: Web of Science, https://clarivate.com/webofsciencegroup/solutions/web-of-science/ (last access: 13 February 2024), 2023.
Clarisse, L., Clerbaux, C., Franco, B., Hadji-Lazaro, J., Whitburn, S., Kopp, A. K., Hurtmans, D., and Coheu, P.-F.: A decadal data set of global atmospheric dust retrieved from IASI satellite measurements, J. Geophys. Res.-Atmos., 124, 1618–1647, https://doi.org/10.1029/2018JD029701, 2019.
DeSouza-Machado, S. G., Strow, L. L., Hannon, S. E., and Motteler, H. E.: Infrared dust spectral signatures from AIRS, Geophys. Res. Lett., 33, L03801, https://doi.org/10.1029/2005GL024364, 2006.
DeSouza-Machado, S. G., Strow, L. L., Imbiriba, B., McCann, K., Hoff, R. M., Hannon, S. E., Martins, J. V., Tanré, D., Deuzé, J. L., Ducos, F., and Torres, O.: Infrared retrievals of dust using AIRS: Comparisons of optical depths and heights derived for a North African dust storm to other collocated EOS A-Train and surface observations, J. Geophys. Res.-Atmos., 115, D15201, https://doi.org/10.1029/2009JD012842, 2010.
Deuzé, J. L., Goloub, P., Herman, M., Marchand, A., Perry, G., Susana, S., and Tanré, D.: Estimate of the aerosol properties over the ocean with POLDER, J. Geophys. Res. Atmos., 105, 15329–15346, https://doi.org/10.1029/2000JD900148, 2000.
Deuzé, J. L., Bréon, F. M., Devaux, C., Goloub, P. H., Herman, M., Lafrance, B., Maignan, F., Marchand, A., Nadal, F., Perry, G., and Tanré, D.: Remote sensing of aerosols over land surfaces from POLDER-ADEOS-1 polarized measurements, J. Geophys. Res.-Atmos., 106, 4913–4926, https://doi.org/10.1029/2000JD900364, 2001.
Diner, D. J., Beckert, J. C., Reilly, T. H., Bruegge, C. J., Conel, J. E., Kahn, R. A., Martonchik, J. V., Ackerman, T. P., Davies, R., Gerstl, S. A., and Gordon, H. R.: Multi-angle Imaging SpectroRadiometer (MISR) instrument description and experiment overview, IEEE Tran. Geosci. Rem. Sens., 36, 1072–1087, https://doi.org/10.1109/36.700992, 1998.
Diner, D. J., Boland, S. W., Brauer, M., Bruegge, C., Burke, K. A., Chipman, R., Di Girolamo, L., Garay, M. J., Hasheminassab, S., Hyer, E., and Jerrett, M.: Advances in multiangle satellite remote sensing of speciated airborne particulate matter and association with adverse health effects: from MISR to MAIA, J. Appl. Rem. Sens., 12, 042603–042603, https://doi.org/10.1117/1.JRS.12.042603, 2018.
Di Noia, A. and Hasekamp, O. P.: Neural networks and support vector machines and their application to aerosol and cloud remote sensing: A review, Springer Series in Light Scattering: Volume 1: Multiple Light Scattering, Rad. Transf. Remote Sens., 279–329, https://doi.org/10.1007/978-3-319-70796-9_4, 2018.
Engel-Cox, J. A., Holloman, C. H., Coutant, B. W., and Hoff, R. M.: Qualitative and quantitative evaluation of MODIS satellite sensor data for regional and urban scale air quality, Atmos. Environ., 38, 2495–2509, https://doi.org/10.1016/j.atmosenv.2004.01.039, 2004.
Fougnie, B., Marbach, T., Lacan, A., Lang, R., Schlüssel, P., Poli, G., Munro, R., and Couto, A. B.: The multi-viewing multi-channel multi-polarisation imager–Overview of the 3MI polarimetric mission for aerosol and cloud characterization, J. Quant. Spec. Rad. Trans., 219, 23–32, doi.org/10.1016/j.jqsrt.2018.07.008, 2018.
Frost, G. J., Kopacz, M., Kondragunta, S., Ahmadov, R., Al Saadi, J. Andrews, A., Barnet, C., Breeze, V., Christopoulos, J., Cooper, O., Crawford, A., Flynn, L., Gaudel, A., Martin, C., McDonald, B., McQueen, J., Paulot, F., Pavolonis, M., Petropavlovskikh, I., Pierce, R. B., Rosenlof, K. H., Saylor, R., Schmit, T., Stajner, I., Stanitski, D., and Szykman, J.: A Value Assessment of an Atmospheric Composition Capability on the NOAA Next-Generation Geostationary and Extended Orbits (GEO-XO) Missions, NOAA technical report OAR CPO, 8, https://doi.org/10.25923/1s4s-t405, 2020.
Gao, M., Franz, B. A., Knobelspiesse, K., Zhai, P.-W., Martins, V., Burton, S., Cairns, B., Ferrare, R., Gales, J., Hasekamp, O., Hu, Y., Ibrahim, A., McBride, B., Puthukkudy, A., Werdell, P. J., and Xu, X.: Efficient multi-angle polarimetric inversion of aerosols and ocean color powered by a deep neural network forward model, Atmos. Meas. Tech., 14, 4083–4110, https://doi.org/10.5194/amt-14-4083-2021, 2021.
Gao, Y., Kaufman, Y. J., Tanré, D., Kolber, D., and Falkowski, P. G.: Seasonal distributions of aeolian iron fluxes to the global ocean, Geophys. Res. Lett., 28, 29–32, https://doi.org/10.1029/2000GL011926, 2001.
Gelaro, R., McCarty, W., Suarez, M., Todling, R., Molod, A., Takacs, L., Randles, C.A., Darmenov, A., Bosilovich, M. G., Reichle, R., Wargan, K., Coy, L., Cullather, R., Draper, C., Akella, S., Buchard, V., Conaty, A., da Silva A. M., Gu, W., Kim, G.-K., Koster, R., Lucchesi, R., Merkova, D., Nielsen, J. E., Partyka, G., Pawson, S., Putnam, W., and Rienecker, M., Schubert, S. D., Sienkiewicz, M., Zhao, B.: The Modern-Era Retrospective Analysis for Research and Applications, Version 2 (MERRA-2), J. Climate, 30, 5419–5454, https://doi.org/10.1175/JCLI-D-16-0758.1, 2017.
Giles, D. M., Sinyuk, A., Sorokin, M. G., Schafer, J. S., Smirnov, A., Slutsker, I., Eck, T. F., Holben, B. N., Lewis, J. R., Campbell, J. R., Welton, E. J., Korkin, S. V., and Lyapustin, A. I.: Advancements in the Aerosol Robotic Network (AERONET) Version 3 database – automated near-real-time quality control algorithm with improved cloud screening for Sun photometer aerosol optical depth (AOD) measurements, Atmos. Meas. Tech., 12, 169–209, https://doi.org/10.5194/amt-12-169-2019, 2019.
Groß, S., Freudenthaler, V., Wirth, M., and Weinzierl, B.: Towards an aerosol classification scheme for future EarthCARE lidar observations and implications for research needs, Atmos. Sci. Lett., 16, 77–82, https://doi.org/10.1002/asl2.524, 2015.
Gupta, P. and Christopher, S. A.: Particulate matter air quality assessment using integrated surface, satellite, and meteorological products: Multiple regression approach, J. Geophys. Res., 114, D14205, https://doi.org/10.1029/2008JD011496, 2009a.
Gupta, P. and Christopher, S. A.: Particulate matter air quality assessment using integrated surface, satellite, and meteorological products: 2. A neural network approach, J. Geophys. Res., 114, D20205, https://doi.org/10.1029/2008JD011497, 2009b.
Hammer, M. S., van Donkelaar, A. Li, C., Lyapustin, A., Sayer, A. M., Hsu, N. C., Levy, R. C., Garay, M. J., Kalashnikova, O. V., Kahn, R. A., Brauer, M., Apte, J. S., Henze, D. K., Zhang, L., Zhang, Q., Ford, B., Pierce, J. R., and Martin, R. V.: Global estimates and long-term trends of fine particulate matter concentrations (1998–2018), Environ. Sci. Technol., 54, 7879–7890, https://doi.org/10.1021/acs.est.0c01764, 2020.
Jethva, H. T., Torres, O., Remer, L., and Bhartia, P. K.: A color ratio method for simultaneous retrieval of aerosol and cloud optical thickness of above-cloud absorbing aerosols from passive sensors: Application to MODIS measurements, IEEE T. Geosci. Remote Sens., 51, 3870, https://doi.org/10.1109/TGRS.2012.2230008, 2013.
Holben, B. N., Eck, T. F., Slutsker, I., Tanre, D., Buis, J. P., Setzer, A., Vermote, E., Reagan, J. A., Kaufman, Y. J., Nakajima, T., Lavenu, F., Jankowiak, I., and Smirnov, A.: AERONET – A federated instrument network and data archive for aerosol characterization, Remote Sens. Environ., 66, 1–16, https://doi.org/10.1016/S0034-4257(98)00031-5, 1998.
Hsu, N. C., Tsay, S. C., King, M. D., and Herman, J. R.: Deep blue retrievals of Asian aerosol properties during ACE-Asia, IEEE T. Geosci. Remote Sens., 44, 3180–3195, https://doi.org/10.1109/TGRS.2006.879540, 2006.
Hsu, N. C., Jeong, M. J., Bettenhausen, C., Sayer, A. M., Hansell, R., Seftor, C. S., Huang, J., and Tsay, S. C.: Enhanced Deep Blue aerosol retrieval algorithm: The second generation, J. Geophys. Res.-Atmos., 118, 9296–9315, https://doi.org/10.1002/jgrd.50712, 2013.
Kacenelenbogen, M. S. F., Tan, Q., Burton, S. P., Hasekamp, O. P., Froyd, K. D., Shinozuka, Y., Beyersdorf, A. J., Ziemba, L., Thornhill, K. L., Dibb, J. E., Shingler, T., Sorooshian, A., Espinosa, R. W., Martins, V., Jimenez, J. L., Campuzano-Jost, P., Schwarz, J. P., Johnson, M. S., Redemann, J., and Schuster, G. L.: Identifying chemical aerosol signatures using optical suborbital observations: how much can optical properties tell us about aerosol composition?, Atmos. Chem. Phys., 22, 3713–3742, https://doi.org/10.5194/acp-22-3713-2022, 2022.
Kahn, R. A. and Gaitley, B. J.: An analysis of global aerosol type as retrieved by MISR, J. Geophys. Res.-Atmos., 120, 4248–4281, https://doi.org/10.1002/2015JD023322, 2015.
Kahn, R. A., Banerjee, P., MacDonald, D., and Diner, D.: Sensitivity of multiangle imaging to aerosol optical depth and to pure-particle size distribution and composition over ocean, J. Geophys. Res., 103, 32195–32213, https://doi.org/10.1029/98JD01752, 1998.
Kahn, R. A., Banerjee, P., and McDonald, D.: The Sensitivity of Multiangle Imaging to Natural Mixtures of Aerosols Over Ocean, J. Geophys. Res., 106, 18219–18238, https://doi.org/10.1029/2000JD900497, 2001.
Kahn, R. A., Li, W.-H., Moroney, C., Diner, D. J., Martonchik, J. V., and Fishbein, E.: Aerosol source plume physical characteristics from space-based multiangle imaging, J. Geophys. Res., 112, D11205, https://doi.org/10.1029/2006JD007647, 2007.
Kahn, R. A., Chen, Y., Nelson, D. L., Leung, F.-Y., Li, Q., Diner, D. J., and Logan, J. A.: Wildfire smoke injection heights: Two perspectives from space, Geophys. Res. Lett., 35, L04809, https://doi.org/10.1029/2007GL032165, 2008.
Kahn, R. A., Andrews, E., Brock, C.A., Chin, M., Feingold, G., Gettelman, A., Levy, R. C., Murphy, D. M., Nenes, A., Pierce, J. R., Popp, T., Redemann, J., Sayer, A. M., da Silva, A. M., Sogacheva, L., and Stier, P.: Reducing aerosol forcing uncertainty by combining models with satellite and within-the-atmosphere observations: A three-way street, Rev. Geophys., 61, e2022RG000796, https://doi.org/10.1029/2022RG000796, 2022.
Kang, Y., Kim, M., Kang, E., Cho, D., and Im, J.: Improved retrievals of aerosol optical depth and fine mode fraction from GOCI geostationary satellite data using machine learning over East Asia, ISPRS J. Photogramm., 183, 253–268, https://doi.org/10.1016/j.isprsjprs.2021.11.016, 2022.
Kaufman, Y., Tanré, D,. and Boucher, O.: A satellite view of aerosols in the climate system, Nature, 419, 215–223, https://doi.org/10.1038/nature01091, 2002.
Kaufman, Y. J., Tanré, D., Remer, L. A., Vermote, E. F., Chu, A., and Holben, B. N.: Operational remote sensing of tropospheric aerosol over land from EOS moderate resolution imaging spectroradiometer, J. Geophys. Res., 102, 17051–17067, https://doi.org/10.1029/96JD03988, 1997.
Kaufman, Y. J., Koren, I., Remer, L. A., Rosenfeld, D., and Rudich, Y.: The effect of smoke, dust, and pollution aerosol on shallow cloud development over the Atlantic Ocean, P. Natl. Acad. Sci. USA, 102, 11207–11212, https://doi.org/10.1073/PNAS.0505191102, 2005.
Ke, J., Sun, Y., Dong, C., Zhang, X., Wang, Z., Lyu, L., Zhu, W., Ansmann, A., Su, L., Bu, L., Xiao, D. Wang, S., Chen, S., Liu, J., Chen, W., and Liu, D.: Development of China's first space-borne aerosol-cloud high-spectral-resolution lidar: retrieval algorithm and airborne demonstration PhotoniX, 3, p. 17, https://doi.org/10.1186/s43074-022-00063-3, 2022.
Klüser, L., Martynenko, D., and Holzer-Popp, T.: Thermal infrared remote sensing of mineral dust over land and ocean: a spectral SVD based retrieval approach for IASI, Atmos. Meas. Tech., 4, 757–773, https://doi.org/10.5194/amt-4-757-2011, 2011.
Koren, I., Kaufman, Y. J., Rosenfeld, D., Remer, L. A., and Rudich, Y.: Aerosol invigoration and restructuring of Atlantic convective clouds, Geophys. Res. Lett., 32, L14828, https://doi.org/10.1029/2005GL023187, 2005.
Koren, I., Martins, J. V., Remer, L. A., and Afargan, H.: Smoke Invigoration Versus Inhibition of Clouds over the Amazon, Science, 321, 946–949, https://doi.org/10.1126/science.1159185, 2008.
LAADS DAAC (Level-1 and Atmosphere Archive & Distribution System Distributed Active Archive Center): LAADS DAAC web page, https://ladsweb.modaps.eosdis.nasa.gov/about/ (last access: 5 February 2023), 2023.
Lary, D. J., Remer, L. A., MacNeill, D., Roscoe, B., and Paradise, S.: Machine learning and bias correction of MODIS aerosol optical depth, IEEE Geosci. Remote Sens. Lett., 6, 694–698, https://doi.org/10.1109/LGRS.2009.2023605, 2009.
Lee, J., Shi, Y. R., Cai, C., Ciren, P., Wang, J., Gangopadhyay, A., and Zhang, Z.: Machine learning based algorithms for global dust aerosol detection from satellite images: inter-comparisons and evaluation, Remote Sens., 13, 456, https://doi.org/10.3390/rs13030456, 2021.
Levy, R. C., Remer, L. A., Mattoo, S., Vermote, E. F., and Kaufman, Y. J.: Second-Generation Operational Algorithm: Retrieval of Aerosol Properties over Land from Inversion of Moderate Resolution Imaging Spectroradiometer Spectral Reflectance, J. Geophys. Res.-Atmos., 112, D13211, https://doi.org/10.1029/2006JD007811, 2007.
Levy, R. C., Mattoo, S., Munchak, L. A., Remer, L. A., Sayer, A. M., Patadia, F., and Hsu, N. C.: The Collection 6 MODIS aerosol products over land and ocean, Atmos. Meas. Tech., 6, 2989–3034, https://doi.org/10.5194/amt-6-2989-2013, 2013.
Li, L., Dubovik, O., Derimian, Y., Schuster, G. L., Lapyonok, T., Litvinov, P., Ducos, F., Fuertes, D., Chen, C., Li, Z., Lopatin, A., Torres, B., and Che, H.: Retrieval of aerosol components directly from satellite and ground-based measurements, Atmos. Chem. Phys., 19, 13409–13443, https://doi.org/10.5194/acp-19-13409-2019, 2019.
Li, Z., Lau, W. K.-M., Ramanathan, V., Wu, G., Ding, Y., Manoj, M. G., Liu, J., Qian, Y., Li, J., Zhou, T., Fan, J., Rosenfeld, D., Ming, Y., Wang, Y., Huang, B., Wang, B., Xu, X., Lee, S.-S. , Cribb, M. C., Zhang, F., Yang, X., Zhao, C., Takemura, T., Wang, K., Xia, X., Yin, Y., Zhang, H., Guo, J., Zhai, P. M., Sugimoto, N., Babu, S. S., and Brasseur, G. P.: Aerosol and monsoon interactions in Asia, Rev. Geophys., 54, 866–929, https://doi.org/10.1002/2015RG000500, 2016.
Lipponen, A., Reinvall, J., Väisänen, A., Taskinen, H., Lähivaara, T., Sogacheva, L., Kolmonen, P., Lehtinen, K., Arola, A., and Kolehmainen, V.: Deep-learning-based post-process correction of the aerosol parameters in the high-resolution Sentinel-3 Level-2 Synergy product, Atmos. Meas. Tech., 15, 895–914, https://doi.org/10.5194/amt-15-895-2022, 2022.
Liu, X., Stamnes, S., Burton, S, Ferrare, R., Hostetler, C., Chemyakin, E., Mueller, D., and Cairns, B.: A Combined Polarimeter and Lidar Optimal Estimation Algorithm to Improve Aerosol Microphysical Property Retrievals, NASA Technical Reports Server, Document ID 20200009791, https://ntrs.nasa.gov/citations/20200009791, 2017.
Lopatin, A., Dubovik, O., Chaikovsky, A., Goloub, P., Lapyonok, T., Tanré, D., and Litvinov, P.: Enhancement of aerosol characterization using synergy of lidar and sun-photometer coincident observations: the GARRLiC algorithm, Atmos. Meas. Tech., 6, 2065–2088, https://doi.org/10.5194/amt-6-2065-2013, 2013.
Lyapustin, A., Wang, Y., Korkin, S., and Huang, D.: MODIS Collection 6 MAIAC algorithm, Atmos. Meas. Tech., 11, 5741–5765, https://doi.org/10.5194/amt-11-5741-2018, 2018.
Malm, W. C. and Hand, J. L.: An examination of the physical and optical properties of aerosols collected in the IMPROVE program, Atmos. Environ., 41, 3407–3427, https://doi.org/10.1016/j.atmosenv.2006.12.012, 2007.
Martonchik, J. V., Diner, D. J., Kahn, R. A., Ackerman, T. P., Verstraete, M. M., Pinty, B., and Gordon, H. R.: Techniques for the retrieval of aerosol properties over land and ocean using multiangle imaging, IEEE T. Geosci. Remote Sens., 36, 1212–1227, https://doi.org/10.1109/36.701027, 1998.
Martonik J. V., Kahn, R. A., and Diner, D. J.: Retrieval of Aerosol Properties over Land Using MISR Observations, in: Aerosol Remote Sensing Over Land, edtied by: Kokhanovsky, A. A. and de Leeuw, G., Satellite Springer, Berlin, 267-293, ISBN 978-3-540-69396-3, 2009.
Mei, L., Vandenbussche, S., Rozanov, V., Proestakis, E., Amiridis, V., Callewaert, S., Vountas, M., and Burrows, J. P.: On the retrieval of aerosol optical depth over cryosphere using passive remote sensing, Remote Sens. Environ., 241, 111731, https://doi.org/10.1016/j.rse.2020.111731, 2020.
Müller, D., Hostetler, C. A., Ferrare, R. A., Burton, S. P., Chemyakin, E., Kolgotin, A., Hair, J. W., Cook, A. L., Harper, D. B., Rogers, R. R., Hare, R. W., Cleckner, C. S., Obland, M. D., Tomlinson, J., Berg, L. K., and Schmid, B.: Airborne Multiwavelength High Spectral Resolution Lidar (HSRL-2) observations during TCAP 2012: vertical profiles of optical and microphysical properties of a smoke/urban haze plume over the northeastern coast of the US, Atmos. Meas. Tech., 7, 3487–3496, https://doi.org/10.5194/amt-7-3487-2014, 2014.
NASA Press Release: NASA, Partners Achieve Fastest Space-to-Ground Laser Comms Link, https://www.nasa.gov/feature/ames/tbird-milestone (last access: 13 February 2024), 2023.
NASA Technology Solution Communications: Radiation-Hardened, High- Data-Rate Ka-Band Modulator and Transmitter, https://partnerships.gsfc.nasa.gov/conradchallenge/tops/GSC-TOPS-49.pdf (last access: 13 February 2024), 2023.
Niu, F. and Li, Z.: Systematic variations of cloud top temperature and precipitation rate with aerosols over the global tropics, Atmos. Chem. Phys., 12, 8491–8498, https://doi.org/10.5194/acp-12-8491-2012, 2012.
Pappalardo, G., Amodeo, A., Apituley, A., Comeron, A., Freudenthaler, V., Linné, H., Ansmann, A., Bösenberg, J., D'Amico, G., Mattis, I., Mona, L., Wandinger, U., Amiridis, V., Alados-Arboledas, L., Nicolae, D., and Wiegner, M.: EARLINET: towards an advanced sustainable European aerosol lidar network, Atmos. Meas. Tech., 7, 2389–2409, https://doi.org/10.5194/amt-7-2389-2014, 2014.
Peng, J., Li, Z., Zhang, H., Liu, J., and Cribb, M.: Systematic changes in cloud radiative forcing with aerosol loading for deep clouds in the tropics, J. Atmos. Sci., 73, 231–249, https://doi.org/10.1175/JAS-D-15-0080.1, 2016.
Prucher, J.: The Oxford Dictionary of Science Fiction, Oxford University Press, ISBN-13 9780195305678, https://doi.org/10.1093/acref/9780195305678.001.0001 (last access: 29 May 2023), 2006.
Randles, C. A., Da Silva, A. M., Buchard, V., Colarco, P. R., Darmenov, A., Govindaraju, R., Smirnov, A., Holben, B., Ferrare, R., Hair, J., and Shinozuka, Y.: The MERRA-2 aerosol reanalysis, 1980 onward. Part I: System description and data assimilation evaluation, J. Climate, 30, 6823-6850, https://doi.org/10.1175/JCLI-D-16-0609.1, 2017.
Remer, L. A. and Kaufman, Y. J.: Aerosol direct radiative effect at the top of the atmosphere over cloud free ocean derived from four years of MODIS data, Atmos. Chem. Phys., 6, 237–253, https://doi.org/10.5194/acp-6-237-2006, 2006.
Remer, L. A., Kaufman, Y. J., Tanre, D., Mattoo, S., Chu, D. A., Martins, J. V., Li, R. R., Ichoku, C., Levy, R. C., Kleidman, R. G., Eck, T. F., Vermote, E., and Holben, B. N.: The MODIS aerosol algorithm, products and validation, J. Atmos. Sci., 62, 947–973, https://doi.org/10.1175/JAS3385.1, 2005.
Remer, L. A., Kleidman, R. G., Levy, R. C., Kaufman, Y. J., Tanré, D., Mattoo, S., Martins, J. V., Ichoku, C., Koren, I., Yu, H., and Holben, B. N.: Global aerosol climatology from the MODIS satellite sensors, J. Geophys. Res., 113, D14S07, https://doi.org/10.1029/2007JD009661, 2008.
Salomonson, V. V., Barnes, W. L., Maymon, P. W., Montgomery, H. E., and Ostrow, H.: MODIS: Advanced facility instrument for studies of the Earth as a system, IEEE T. Geosci. Remote, 27, 145–153, https://doi.org/10.1109/36.20292, 1989.
Shi, Z., Xing, T., Guang, J., Xue, Y., and Che, Y.: Aerosol Optical Depth over the Arctic Snow-Covered Regions Derived from Dual-Viewing Satellite Observations, Remote Sens., 11, 891, https://doi.org/10.3390/rs11080891, 2019.
Schildhause, C.: “Back to the Future II's Art Director Tells Us How They Developed The Film's Somewhat Misguided Predictions”, Uproxx, https://uproxx.com/movies/back-to-the-future-ii-predictions-art-director/ (last access: 19 February 2024), May 2023.
Schlosser, J. S., Stamnes, S., Burton, S. P., Cairns, B., Crosbie, E., Van Diedenhoven, B., Diskin, G., Dmitrovic, S., Ferrare, R., Hair, J. W., Hostetler, C. A., Hu, Y., Liu, X., Moore, R. H., Shingler, T., Shook, M. A., Thornhill, K. L., Winstead, E., Ziemba, L., and Sorooshian, A.: Polarimeter + Lidar − Derived Aerosol Particle Number Concentration, Front. Remote Sens., 3, 885332, https://doi.org/10.3389/frsen.2022.885332, 2022.
Snider, G., Weagle, C. L., Martin, R. V., van Donkelaar, A., Conrad, K., Cunningham, D., Gordon, C., Zwicker, M., Akoshile, C., Artaxo, P., Anh, N. X., Brook, J., Dong, J., Garland, R. M., Greenwald, R., Griffith, D., He, K., Holben, B. N., Kahn, R., Koren, I., Lagrosas, N., Lestari, P., Ma, Z., Vanderlei Martins, J., Quel, E. J., Rudich, Y., Salam, A., Tripathi, S. N., Yu, C., Zhang, Q., Zhang, Y., Brauer, M., Cohen, A., Gibson, M. D., and Liu, Y.: SPARTAN: a global network to evaluate and enhance satellite-based estimates of ground-level particulate matter for global health applications, Atmos. Meas. Tech., 8, 505–521, https://doi.org/10.5194/amt-8-505-2015, 2015.
Song, Q., Zhang, Z., Yu, H., Kok, J. F., Di Biagio, C., Albani, S., Zheng, J., and Ding, J.: Size-resolved dust direct radiative effect efficiency derived from satellite observations, Atmos. Chem. Phys., 22, 13115–13135, https://doi.org/10.5194/acp-22-13115-2022, 2022.
Su, T., Laszlo, I., Li, Z., Wei, J., and Kalluri, S.: Refining aerosol optical depth retrievals over land by constructing the relationship of spectral surface reflectances through deep learning: application to Himawari-8, Remote Sens. Environ., 251, 112093, https://doi.org/10.1016/j.rse.2020.112093, 2020.
Tanré, D., Herman, M., and Kaufman, Y. J.: Information on the aerosol size distribution contained in the solar reflected spectral radiances, J. Geophys. Res., 101, 19043–19060, https://doi.org/10.1029/96JD00333, 1996.
Tanré, D., Kaufman, Y. J., Herman, M., and Mattoo, S.: Remote sensing of aerosol properties over oceans using the MODIS/EOS spectral radiances, J. Geophys. Res., 102, 16971–16988, https://doi.org/10.1029/96JD03437, 1997.
Torres, O., Jethva, H. T., and Bhartia, P. K.: Retrieval of Aerosol Optical Depth above Clouds from OMI Observations: Sensitivity Analysis and Case Studies, J. Atmos. Sci., 69, 1037–1053, https://doi.org/10.1175/JAS-D-11-0130.1, 2012.
Ukkonen, P.: Exploring pathways to more accurate machine learning emulation of atmospheric radiative transfer, J. Adv. Model. Earth Syst., 14, e2021MS002875, https://doi.org/10.1029/2021MS002875, 2022.
Val Martin, M., Kahn, R. A., and Tosca, M. G.: A Global Analysis of Wildfire Smoke Injection Heights Derived from Space-Based Multi-Angle Imaging, Remote Sens., 10, 1609, https://doi.org/10.3390/rs10101609, 2018.
Van Donkelaar, A., Martin, R. V., and Park, R. J.: Estimating ground-level PM2.5 using aerosol optical depth determined from satellite remote sensing, J. Geophys. Res., 111, D21201, https://doi.org/10.1029/2005JD006996, 2006.
Van Donkelaar, A., Martin, R. V., Brauer, M., Hsu, N. C., Kahn, R. A., Levy, R. C., Lyapustin, A., Sayer, A. M., and Winker, D. M.: Global estimates of fine particulate matter using a combined geophysical-statistical method with information from satellites, models, and monitors, Environ. Sci. Technol., 50, 3762–3772, https://doi.org/10.1021/acs.est.5b05833, 2016.
Voss, K. K. and Evan, A. T.: A new satellite-based global climatology of dust aerosol optical depth, J. Appl. Meteorol. Clim. 59, 83–102, doi.org/10.1175/JAMC-D-19-0194.1, 2020.
Wei, J., Huang, W., Li, Z., Sun, L., Zhu, X., Yuan, Q., Liu, L., and Cribb, M. C.: Cloud detection for Landsat imagery by combining the random forest and superpixels extracted via energy-driven sampling segmentation approaches, Remote Sens. Environ., 248, 112005, https://doi.org/10.1016/j.rse.2020.112005, 2020.
Welton, E. J., Campbell, J. R., Spinhirne, J. D., and Scott III, V. S.: Global monitoring of clouds and aerosols using a network of micropulse lidar systems. Proc. SPIE 4153, P. Soc. Photo-Opt. Ins., Vol. 4153, https://doi.org/10.1117/12.417040, 2001.
Werdell, P. J., Behrenfeld, M. J., Bontempi, P. S., Boss, E., Cairns, B., Davis, G. T., Franz, B. A., Gliese, U. B., Gorman, E. T., Hasekamp, O., Knobelspiesse, K. D., Mannino, A., Martins, J. V., McClain, C. R., Meister, G., and Remer, L. A.: The Plankton, Aerosol, Cloud, ocean Ecosystem mission: status, science, advances, B. Am. Meteorol. Soc., 100, 1775–1794, doi.org/10.1175/BAMS-D-18-0056.1, 2019.
WMO OSCAR: World Meterological Organization Observing Systems Capability Analysis and Review Tool, https://space.oscar.wmo.int (last access: 13 February 2024), 2011–2023.
Xu, F., Gao, L., Redemann, J., Flynn, C. J., Espinosa, W. R., da Silva, A. M., Stamnes, S., Burton, S. P., Liu, X., Ferrare, R., Cairns, B., and Dubovik, O.: A combined Lidar-Polarimeter inversion approach for aerosol remote sensing over ocean, Front. Remote Sens., 2, 620871, https://doi.org/10.3389/frsen.2021.620871, 2021.
Yu, H., Kaufman, Y. J., Chin, M., Feingold, G., Remer, L. A., Anderson, T. L., Balkanski, Y., Bellouin, N., Boucher, O., Christopher, S., DeCola, P., Kahn, R., Koch, D., Loeb, N., Reddy, M. S., Schulz, M., Takemura, T., and Zhou, M.: A review of measurement-based assessments of the aerosol direct radiative effect and forcing, Atmos. Chem. Phys., 6, 613–666, https://doi.org/10.5194/acp-6-613-2006, 2006.
Yu, H., Remer, L. A., Kahn, R. A., Chin, M., and Zhang, Y.: Satellite perspective of aerosol intercontinental transport: From qualitative tracking to quantitative characterization, Atmos. Res., 124, 73–100, https://doi.org/10.1016/j.atmosres.2012.12.013, 2013.
Yu, H., Tan, Q., Chin, M. Remer, L. A., Kahn, R. A., Bian, H., Kim, D., Zhang, Z., Yuan, T., Omar, A. H., Winker, D. M., Levy, R. C., Kalashnikova, O., Crepeau, L., Capelle, V., and Chédin, A. : Estimates of African dust deposition along the trans-Atlantic transit using the decadelong record of aerosol measurements from CALIOP, MODIS, MISR, and IASI, J. Geophys. Res.-Atmos., 124, 7975–7996, https://doi.org/10.1029/2019JD030574, 2019.
Yu, H., Yang, Y., Wang, H., Tan, Q., Chin, M., Levy, R. C., Remer, L. A., Smith, S. J., Yuan, T., and Shi, Y.: Interannual variability and trends of combustion aerosol and dust in major continental outflows revealed by MODIS retrievals and CAM5 simulations during 2003–2017, Atmos. Chem. Phys., 20, 139–161, https://doi.org/10.5194/acp-20-139-2020, 2020.
Yuan, T., Remer, L. A., Pickering, K. E., and Yu, H.: Observational evidence of aerosol enhancement of lightning activity and convective invigoration, Geophys. Res. Lett., 38, L04701, https://doi.org/10.1029/2010GL046052, 2011a.
Yuan, T., Remer, L. A., and Yu, H.: Microphysical, macrophysical and radiative signatures of volcanic aerosols in trade wind cumulus observed by the A-Train, Atmos. Chem. Phys., 11, 7119–7132, https://doi.org/10.5194/acp-11-7119-2011, 2011b.
Zemeckis, R. and Gale, B.: Back To the Future Part II. Amblin Entertainment and Universal Pictures, produced by: Bob Gale and Neil Canton, 108 minutes, 1989.
Zhang, J. and Christopher, S. A.: Longwave radiative forcing of Saharan dust aerosols estimated from MODIS, MISR, and CERES observations on Terra, Geophys. Res. Lett., 30, 2188, https://doi.org/10.1029/2003GL018479, 2003.
Zhang, J. and Reid, J. S.: A decadal regional and global trend analysis of the aerosol optical depth using a data-assimilation grade over-water MODIS and Level 2 MISR aerosol products, Atmos. Chem. Phys., 10, 10949–10963, https://doi.org/10.5194/acp-10-10949-2010, 2010.
Zhang, J., Reid, J. S., Westphal, D. L., Baker, N. L., and Hyer, E. J.: A system for operational aerosol optical depth data assimilation over global oceans, J. Geophys. Res.-Atmos., 113, D10208, https://doi.org/10.1029/2007JD009065, 2008.
Zhang, Z., Fu, G., and Hasekamp, O.: Aerosol retrieval over snow using the RemoTAP algorithm, Atmos. Meas. Tech., 16, 6051–6063, https://doi.org/10.5194/amt-16-6051-2023, 2023.
Zheng, J., Zhang, Z., Garnier, A., Yu, H., Song, Q., Wang, C., Dubuisson, P., and Di Biagio, C.: The thermal infrared optical depth of mineral dust retrieved from integrated CALIOP and IIR observations, Remote Sens. Environ., 270, 112841, https://doi.org/10.1016/j.rse.2021.112841, 2022.
- Abstract
- NASA initiates a revolution with the Terra and Aqua missions
- New technology leads to more, more, and more information
- Evolution of the algorithms
- The rise of the cyborg: blends of measurements and models
- Satellites, satellites everywhere
- Calibration and validation systems will need to keep up
- Unanswered science questions will drive the new systems
- So what will 2043 look like?
- Code availability
- Data availability
- Author contributions
- Competing interests
- Disclaimer
- Special issue statement
- Acknowledgements
- Review statement
- References
- Abstract
- NASA initiates a revolution with the Terra and Aqua missions
- New technology leads to more, more, and more information
- Evolution of the algorithms
- The rise of the cyborg: blends of measurements and models
- Satellites, satellites everywhere
- Calibration and validation systems will need to keep up
- Unanswered science questions will drive the new systems
- So what will 2043 look like?
- Code availability
- Data availability
- Author contributions
- Competing interests
- Disclaimer
- Special issue statement
- Acknowledgements
- Review statement
- References