Spatial structure of in situ reflectance in coastal and inland waters: implications for satellite validation
- 1Plymouth Marine Laboratory, Plymouth, United Kingdom
- 2Marine and Environmental Science Centre, Faculdade de Ciências da Universidade de Lisboa, Lisboa, Portugal
Validation of satellite-derived aquatic reflectance involves relating meter-scale in situ observations to satellite pixels with typical spatial resolution ∼ 10–100 m within a temporal “match-up window” of an overpass. Due to sub-pixel variation these discrepancies in measurement scale are a source of uncertainty in the validation result. Additionally, validation protocols and statistics do not normally account for spatial autocorrelation when pairing in situ data from moving platforms with satellite pixels. Here, using high-frequency autonomous mobile radiometers deployed on ships, we characterize the spatial structure of in situ Rrs in inland and coastal waters (Lake Balaton, Western English Channel, Tagus Estuary). Using variogram analysis, we partition Rrs variability into spatial and intrinsic (non-spatial) components. We then demonstrate the capacity of mobile radiometers to spatially sample in situ Rrs within a temporal window broadly representative of satellite validation and provide spatial statistics to aid satellite validation practice. At a length scale typical of a medium resolution sensor (300 m) between 5% and 35% (median values across spectral bands and deployments) of the variation in in situ Rrs was due to spatial separation. This result illustrates the extent to which mobile radiometers can reduce validation uncertainty due to spatial discrepancy via sub-pixel sampling. The length scale at which in situ Rrs became spatially decorrelated ranged from ∼ 100–1,000 m. This information serves as a guideline for selection of spatially independent in situ Rrs when matching with a satellite image, emphasizing the need for either downsampling or using modified statistics when selecting data to validate high resolution sensors (sub 100 m pixel size).
1 Introduction
Satellite observations of aquatic reflectance are used to derive water quality parameters such as photosynthetic pigment concentration, light availability, and infer the transport of suspended solids. These are used to delineate aquatic habitats and to investigate biogeochemical processes in aquatic ecosystems. Satellite radiance recorded at the top of the atmosphere requires correction for atmospheric scattering and absorption to provide consistent observation of water colour. Accurate derivation of the water-leaving radiance is challenging as the aquatic signal component is often
The atmospheric correction of satellite radiometry is particularly challenging in coastal and inland waters, due to their optical complexity relative to ocean waters where optical properties are closely coupled to the phytoplankton component. Inland and coastal waters also have pronounced diversity in their particulate constituents, and therefore the shape of reflectance spectra (Spyrakos et al., 2018; Lehmann et al., 2023). A wide turbidity range, particularly at rivers and in relatively shallow areas with soft substrate, can cause near-infrared (NIR) reflectance to depart significantly from zero, making the atmospheric correction harder to constrain than in the open ocean (Siegel et al., 2000). Near land, the top-of atmosphere radiance is further perturbed by light reflected from neighbouring land pixels and scattered into the field of view of the sensor, also known as the adjacency effect (Otterman and Fraser, 1979; Bulgarelli et al., 2014). Additionally, near the coast, there are complex aerosol mixtures that make selection of an appropriate aerosol model very challenging (e.g., Montes et al., 2022). Current satellite radiometer systems used for inland and coastal waters include those designed for ocean colour observation with a spatial resolution up to 300 m and daily revisit time, as well as land imagers with fewer and broader spectral bands but higher spatial resolution (up to 10 m) and longer revisit times up to 5 days at the equator. There are several atmospheric correction processors developed for these systems that are undergoing validation with in situ Rrs (e.g., Barnes et al., 2019; Warren et al., 2019; Pahlevan et al., 2021). Due to dependencies between water type and satellite Rrs accuracy (Pahlevan et al., 2021), it is desirable to perform satellite validation across a range of sites representing optical variability in water and atmospheric composition.
In addition to the atmospheric correction, the differing spatial scales of satellite and in situ reflectance are sources of uncertainty within a validation analysis (Salama and Su, 2011; Lee et al., 2012; Salama et al., 2022). Historically, pixel sizes for satellite aquatic remote sensing sensors were ∼ 100–1,000 m2 (Groom et al., 2019), which is far greater than the meter-scale footprint of in situ Rrs. For example, ESA Sentinel-3 OLCI (Ocean Land Color Instrument) has a pixel size 300 m. More recently, higher resolution sensors such as ESA Sentinel-2 Multi Spectral Imager (MSI), which has pixel size 10–60 m, have been used for remote sensing of coastal and inland waters (Warren et al., 2019). Mobile (typically shipborne) and fixed radiometric platforms are both used to collect in situ Rrs, and the different sampling strategies impact on how spatial discrepancy with the satellite pixel can be accounted for. Validation from fixed platforms, for example, the Aeronet-OC network (Zibordi et al., 2009; 2022), is reliant on selecting spatially homogeneous sites and applying filters for spatial homogeneity (Concha et al., 2021). Mobile radiometers deployed for along-track sampling on research vessels and ships-of-opportunity have the benefit that sub-pixel averaging can quantify sub-pixel variability in the presence of horizontal heterogeneity (Brewin et al., 2016), depending on vessel speed and instrument frequency. Deploying mobile radiometers on ships-of-opportunity is a particularly attractive solution to obtaining extensive spatial sampling of inland and coastal water bodies at a low operational cost.
In addition to repeat spatial sampling within an individual pixel, mobile radiometers are also more likely to sample data within multiple satellite pixels within a match-up time window. In principle, this capacity allows for many different match-up pairs to be identified from a single satellite scene. However, validation metrics are typically based on an assumption of statistically independent observation pairs (Loew et al., 2017). Consequently, it is desirable to test for spatial autocorrelation - the statistical dependence of in situ Rrs within neighbouring pixels - prior to performing the match-up analysis. Spatial autocorrelation (in the context of match-up analysis) has received surprisingly little attention within the ocean colour research community, in part due to the relative scarcity of Rrs transects. Research into the scale-dependence of variability and spatial autocorrelation through variography is, however, relatively common in other areas of satellite validation; for example, forest (Román et al., 2009) and glacier (Ryan et al., 2017) albedo. Variography has also successfully been applied to optical water properties in different scientific contexts; for example, spatially resolving sediment plumes (Aurin et al., 2013) and investigation of the spatial structure of planktonic marine ecosystems at the mesoscale (∼10–100 km) (Glover et al., 2018).
In this study we quantify the spatial structure of in situ Rrs from mobile radiometric deployments in coastal and inland waters (Lake Balaton, Western English Channel, Tagus Estuary) over repeat transects which range from ∼ 1 km - ∼ 35 km in length. The overall goal is to provide insight on how spatial structure of in situ Rrs impacts on satellite validation and provide recommendations on how data from mobile radiometers can best be used in the future. We do not perform an explicit match-up analysis with satellite data, but instead apply variogram analysis to in situ data selected within a time interval broadly representative of a match-up window. We partition variation of in situ Rrs into spatial and intrinsic (non-spatial) components, and provide statistics across different spectral bands and deployments. We assess the variation in in situ Rrs at a length scale representative of a medium-resolution satellite sensor such as OLCI (300 m), which enables us to assess how the mobile radiometer is able to reduce in situ Rrs variability via sub-pixel sampling. We then assess the autocorrelation length of in situ Rrs; a quantity which serves as a criterion for selecting independent pixel match-ups for validation from a single scene. This study informs the process of working towards fully automated satellite validation services, specifically highlighting the role of spatial statistics when using data from mobile autonomous radiometric systems.
2 Satellite validation of remote-sensing reflectance
To motivate the study, we first present a brief review of Rrs satellite validation practice, focusing on spatial and temporal collocation. Satellite validation is defined as the process of evaluating, by independent means, the accuracy of satellite-derived data products and quantifying their uncertainties by comparison with in situ reference data (Justice et al., 2000; Loew et al., 2017). In aquatic remote sensing in situ measurements of Rrs (defined as the ratio of water-leaving radiance to downwelling planar irradiance just above the water surface), or the related variable of normalized-water leaving radiance, provide reference data for validation of satellite products (Bailey and Werdell, 2006; Zibordi et al., 2009; Qin et al., 2017; Warren et al., 2019; Pahlevan et al., 2021). Rrs from satellite sensors is normally multi-spectral, consisting of measurements in discrete spectral bands. Rrs from in situ sensors is typically hyperspectral and convolved with the spectral response function of the satellite sensor when performing validation. For convenience, we generally do not explicitly notate the wavelength-dependence of Rrs and other radiometric quantities.
2.1 Match-up criteria and validation metrics
The ocean colour community has applied a range of criteria to match-up analysis which differ primarily in quality control thresholds and spatiotemporal collocation criteria (Bailey and Werdell, 2006; Zibordi et al., 2009; Concha et al., 2021). Prior to matching with in situ data, satellite Rrs are often locally averaged (e.g., using a moving 3 × 3 average of neighbouring pixels). This spatial window, the ‘satellite extract’, is also used to test for spatial homogeneity in Rrs via the local coefficient of variation and to filter out noisy or heterogeneous regions (Bailey and Werdell, 2006). The time window is kept sufficiently short to limit spatiotemporal discrepancies between the in situ and remote sample population, or long to produce sufficient data to allow statistical analysis. The length of the temporal window about a satellite overpass that is used to select in situ Rrs has varied from ±0.5 h (Ilori et al., 2019) (highly dynamic environments) to ±1 day or greater (Kutser, 2012; Warren et al., 2019) (inland waters). Match-up windows for coastal environments typically range from ±1 h to ±3 h (refer to Table 3 in Concha et al. (2021)). Recent IOCCG recommendations for dynamic regions are ±1 h (IOCCG, 2019). If there are repeated in situ Rrs measurements within a pixel within the match-up window, these are typically averaged before being defined as a match-up pair. In addition, a sequence of quality control filters are applied to both in situ Rrs (e.g., restrictions on viewing angles, wind speed, and levels of sunglint), and the satellite-derived reflectance (e.g., restrictions on the pixel classification).
Following data selection and filtering, a range of statistical metrics are then used to assess the uncertainties of the system of remote sensor and atmospheric correction against in situ Rrs (Bailey and Werdell, 2006; Zibordi et al., 2009; Concha et al., 2021). Common validation metrics are, for each sensor waveband, the root-mean-square error (RMSE), mean bias (δ), percentage bias (ψ), defined by:
where yi notates the remote observation (a pixel or local average of pixels), xi notates an in situ match-up (one or many observations averaged over a pixel), and N the number of match-up pairs. The bias metrics can also be defined for absolute differences and/or median averages. Regression methods including ordinary least-squares, Type 2 (Qin et al., 2017) and Deming regression (Warren et al., 2019)) are also used in match-up statistics, in each case, generating correlation, slope and intercept parameters.
In applying validation metrics, Eqs 1–3, underlying statistical assumptions (e.g., requirements on stationary, Gaussianity, linearity, independence of residuals) should be considered (Loew et al., 2017). The assumption of independence of residuals (which applies to all of the metrics above) is particularly relevant to this study, which considers spatial autocorrelation of Rrs. Specifically, the presence of spatial autocorrelation leads to correlation in the residuals, which results in negative/positive residuals tending to occur together. In turn, this leads to an overestimation of the number of independent match-up pairs (i.e., the value of N), therefore impacting on the validity of the statistics.
2.2 Decomposition of sources of uncertainty
To isolate either spatial or temporal sources of uncertainty, previous studies (Salama and Stein, 2009; Salama et al., 2022) have used the following error decomposition
where Δtot is the total retrieval uncertainty, Δd is the ‘derivation uncertainty’, Δs is the spatial discrepancy uncertainty, and Δt is the temporal discrepancy uncertainty. Δtot could be expressed, for example, by δ or ψ in Eqs 2 and 3. Δd represents all non spatiotemporal sources of uncertainty, including sensor calibration, algorithmic uncertainty in atmospheric correction, and normalisation of observation geometry. Δs represents uncertainty due to differences in spatial representation; i.e., uncertainty associated with relating meter-scale in situ measurements with a satellite pixel. Δt represents uncertainty due to differences in the temporal representation; i.e., uncertainty associated with the time difference between the satellite overpass and the in situ measurement. Eq. 4 is approximate, in the sense that the three sources of uncertainty are modelled as independent.
Most Rrs validation studies do not consider Δs and Δt explicitly, and instead aim to minimize their impact via selecting spatially homongenous sample sites and sufficiently short time windows about the satellite overpass (Concha et al., 2021). This is generally done so that the validation accuracy metrics can be used to compare different atmospheric correction schemes (i.e., the assumed dominant sub-component of Δd). In this study, we instead focus on spatial statistics which relate to the Δs term in Eq. 4. Specifically, via characterization of the scale-dependence of Rrs variability, we consider how mobile radiometers can reduce Δs via sub-pixel sampling. Additionally, via characterization of the autocorrelation length, we provide pixel separation distances for selection of spatially independent in situ Rrs.
3 Data
3.1 Radiometric measurement system
In situ Rrs were obtained using the autonomous Solar-tracking Radiometry platform (So-Rad), developed at Plymouth Marine Laboratory (Wright and Simis, 2021; Jordan et al., 2022), and based on the earlier system described by Simis and Olsson (2013). So-Rad obtains Rrs via three synchronised individual spectroradiometer measurements of downwelling irradiance (Ed), sky radiance (Ls) and total upwelling radiance (Lt). A key feature of So-Rad is that the Ls and Lt sensors are mounted to an azimuthally rotating motor which enables optimization of the azimuthal viewing angles with respect to solar azimuth and ship heading. The Ls sensor is at viewing zenith of 40°, with the Lt sensor in the corresponding specular direction. To ensure an unobstructed field of view, the Ed sensor is ideally mounted in an elevated position with unobstructed view of the sky.
The Ed, Lt, and Ls instruments used in So-Rad were TriOS RAMSES ARC (radiance) and ACC (irradiance), calibrated annually at Tartu Observatory (Estonia). The calibrated spectral range of all sensors was 320–950 nm, the spectral resolution was ∼ 10 nm, and the spectral sample spacing was 3.3 nm. The temporal sample spacing between sets of Ed, Lt, and Ls measurements was nominally 15 s. So-Rad was automatically set to record data for solar zenith angles
3.2 Reflectance processing
The retrieval of in situ Rrs used the 3C (3 glint component) algorithm (Groetsch et al., 2017), following the parameterization in Jordan et al. (2022). 3C reconstructs Rrs by inputting a set of Ed, Lt, and Ls spectra into a spectral optimization procedure that incorporates models for solar irradiance (Gregg and Carder, 1990) and the inherent optical properties of water (Albert and Mobley, 2003). The 3C Rrs equation is of the form
where the air-water reflectance factor (ρs) and spectral offset (Δ(λ)) are both solved for within the spectral optimization. 3C is particularly useful in automated, stand-alone deployments, as it bounds how physically realistic a solution is via an optimization residual parameter (Pitarch et al., 2020). It is also effective in non-ideal conditions; e.g., glint affected data or higher wind speeds (Groetsch et al., 2020).
The quality control for Rrs follows the previous steps for the 3C algorithm (Groetsch et al., 2017) as described in Jordan et al. (2022) and provided through the monda (MONocle Data Analysis) Python package (Simis et al., 2022). The key steps are as follows. First, a set of radiometric filters were applied to measured Ed(λ), Ls(λ), and Lt(λ); for example, setting a minimum value on the spectral maximum of Ed(λ) (500 mW m−2nm−1). Second, we removed glint affected spectra when Lt(λ)/Ed(λ) exceeded an empirical threshold of 0.025 sr−1 on the interval 850–900 nm. Third, filtering was applied based on the convergence and residuals of the 3C algorithm optimization.
3.3 Field deployments
The study consists of deployments at three different water bodies. In each case, a So-Rad system was mounted onboard a ship-of-opportunity undergoing operational tasks:
1. Lake Balaton (Hungary) between 28 May and 5 July 2019 onboard the car ferry connecting Tihany and Szántód. The coverage consists of an approximately 1 km transect between the North and South shores of the lake, with the round trip taking approximately 40 min and with minor variation in the exact route over the course of the day.
2. The Western (English) Channel, United Kingdom, between 25 April and 13 October 2021 onboard the Plymouth Marine Laboratory research vessel Quest. The coverage typically consists of weekly transects from the harbour in Plymouth to the L4 buoy and once per month extending to the E1 buoy, approximately 16 km and 37 km from the shore respectively. Radiometric data from Quest, with similar spatial sampling to this study, is presented by Martinez-Vicente et al. (2013).
3. The Tagus Estuary in Lisbon (Portugal) between 29 June and 27 November 2021 onboard the Lisboat sight-seeing ferry. The coverage consists of counter-clockwise circuits of the estuary inlet channel that are approximately 20 km in total transect length.
Example reflectance spectra and coverage maps from each deployment are shown in Figure 1. All three deployments have reflectance peaks between 550 and 600 nm which is typical of inland and nearshore marine water. Lake Balaton (Figures 1A,B) has the highest absolute reflectance values, whilst the Western Channel (Figures 1C,D) has the lowest. The Western Channel dataset spans a larger geographical area and has larger distance between observations due to ship speed. It has the greatest apparent variation in spectral shape and amplitude, and the spectral shape is less constrained towards shorter wavelengths compared to the other sites. All transects correspond to data collected from a 4 h time window (Tagus Estuary) or 6 h time window (Lake Balaton and the Western Channel) centred at 12 noon (approximate solar maximum) in the local time zone as used in the variograms.
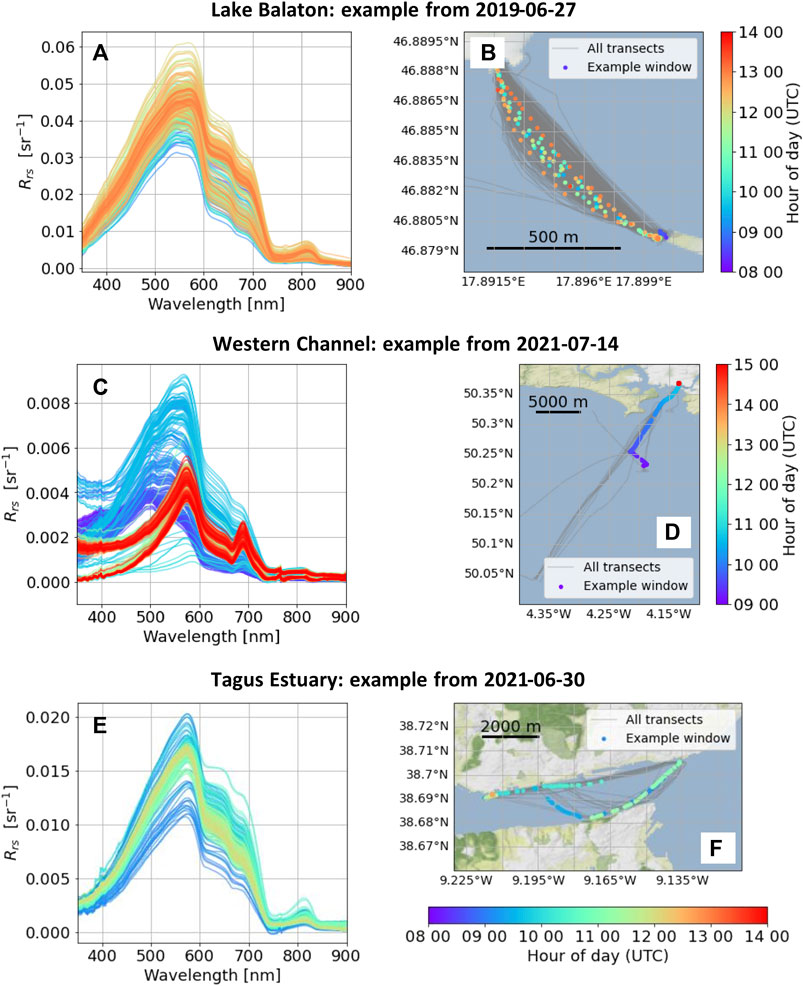
FIGURE 1. Example reflectance (Rrs) spectra and ship transects from each deployment. Top row (A, B) Lake Balaton. Centre row (C, D) Western Channel. Bottom row (E, F) Tagus Estuary. The Rrs spectra correspond to a time window of data as used in the variogram analysis (6 h for Lake Balaton and the Western Channel and 4 h for the Tagus Estuary) centred around noon in the local time zone. The Rrs spectra are referenced to the points and colour bar in the coverage maps.
3.4 Data gridding and selection from time window
Prior to the variogram analsyis (Section 4), the hyperspectral in situ Rrs spectra were downsampled to discrete spectral bands centred on 443, 560, 665, and 783 nm using a Gaussian weighting based on the full width at half maximum of the MSI spectral response function. The band centres correspond to the chlorophyll-a absorption maximum (443 nm), the chlorophyll-a reference/absorption minimum (560 nm), the second chlorophyll-a absorption maximum/suspended sediment band (665 nm) and an NIR band used for atmospheric correction (783 nm). OLCI has similar band centres at 443 nm, 560 nm, 665 nm. Rrs within each spectral band was then resampled to a regular 20 m grid, taking the mean Rrs when there were multiple measurements within each grid cell. The gridding represents a practical lower bound on the length scale for comparisons to be made between in situ and satellite Rrs, and follows how in situ Rrs has been gridded for MSI validation (Warren et al., 2019). The gridding also regularizes the sampling for the variograms as longer time series when the ship is stationary are averaged to a single measurement.
Our variogram analysis considers the spatial dependence of Rrs variability, but neglects (explicit) temporal variation. For each variogram computation, in situ Rrs were selected on a daily basis from a time window centred about 12 noon in the local time zone, allowing ±3 h from noon for the Lake Balaton and Western Channel deployments, and ±2 h for the more dynamic Tagus Estuary. The time window lengths are a trade-off so that data can be adequately sampled to generate the variograms, whilst being sufficiently short to be (broadly) comparable to a satellite match-up window (Section 2.1). The variograms are computed from multiple pairwise in situ measurements, so the associated timescale differs from the single match-up pairs that are used in satellite validation. The distribution of pairwise time separation therefore gives an alternative quantification of the time difference between Rrs measurements within the variogram (Figures 2A–C). The distributions have a strong positive skew and median time separations of 1.29 decimal hours (Lake Balaton), 0.90 decimal hours (Western Channel), 1.05 decimal hours (Tagus Estuary). The Western Channel has the lowest pairwise time separation, as the transects are typically collected over a fraction of the allowed time window (e.g., Figure 1D).
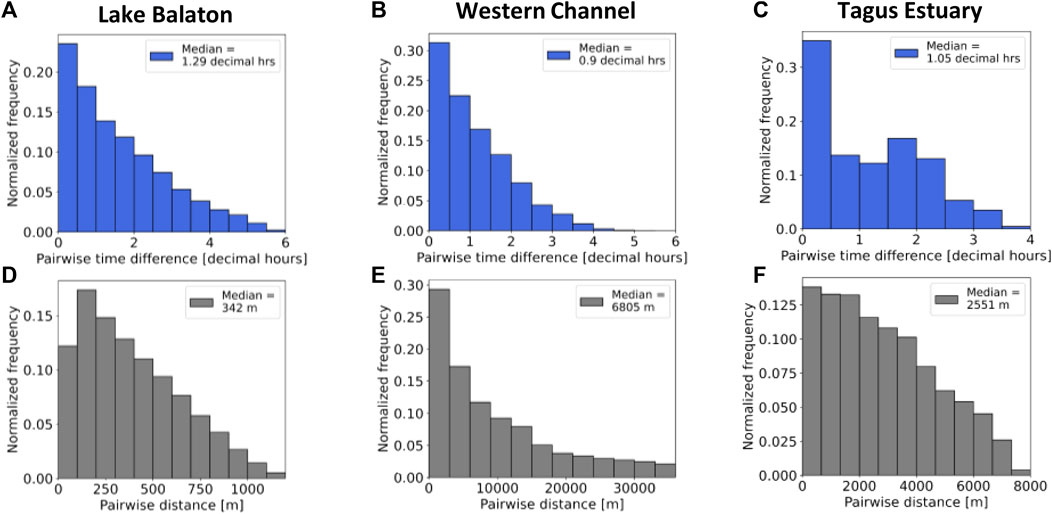
FIGURE 2. Top row (A–C): Normalized frequency distributions of pairwise time separation for each deployment. Bottom row (D–F): Normalized frequency distributions of pairwise point-separation distance for each deployment.
Additionally, the distribution of pairwise point separations, which are an input to the variogram analysis, are shown for each deployment in Figures 2D–F. The maximum point separations are ∼ 1,200 m for Lake Balaton, ∼ 35,000 m for the Western Channel, and ∼ 8,000 m for the Tagus Estuary.
4 Materials and methods
4.1 Overview of variogram analysis
The variogram is a commonly used graphical method to characterize variation in a geographic quantity as a function of the separation distance between measurements, and is described in geostatistics textbooks (e.g., Cressie, 1993) aswell as ocean colour studies (e.g., Glover et al., 2018). The variogram enables partitioning of variance into a structural component that is associated with spatial separation and an intrinsic component not associated with spatial separation. The variogram also enables characterization of the autocorrelation length, which is the characteristic length scale at which a quantity ceases to be spatially correlated with neighbouring observations.
Variogram analysis expresses the semivariance (γ) as a function of separation distance (h), which is also referred to as the lag or ground-sample distance (GSD). For in situ Rrs, computation of the semivariance uses an equation of the form
where Rrs(xi) is an in situ reflectance observation at geographic location xi and, N(h) is the total number of measurement pairs at distance h (Glover et al., 2018). γ(h) is computed separately for each spectral band. The summation in Eq. 6 applies across the set of all pairwise point separations, and pivots about each data point in turn, sampling from a circular annulus with thickness δh (the lag bin width). The radial sampling method can be applied to both distributed data (as occurs for the variable paths of the Lake Balaton car ferry, Figure 1A) and purely transect-like data (as is the case for the Western Channel deployment). Eq. 6 assumes that γ(h) depends only on h but not on the spatial location. In other words, γ(h) represents an average quantity computed over the extent of the geographic survey, unless spatial windowing is applied. As it graphs the semivariance, the variogram is sometimes referred to as a semivariogram (Bachmaier and Backes, 2011). Eq. 6 is applied after quality control of Rrs (see Section 3.2). Numerical details on the computation of γ(h) and fitting procedure are provided in Section 4.3.
γ(h) in Eq. 6 can be interpreted as representing the variance in Rrs at separation distance h (refer to Bachmaier and Backes (2011) for an explanation how the formula relates to a conventional expression for the variance). In the context of in situ Rrs, γ(h) has units sr−2. In satellite validation of Rrs it is preferable to express uncertainty in units of sr−1 (i.e., expressed as a standard deviation of Rrs). We therefore consider graphs of
4.2 Interpreting the root-variogram and spatial structure of in situ Rrs
This section describes the features of the root-variogram and how these relate to spatial properties of Rrs. A schematic example from Lake Balaton is shown in Figure 3 for Rrs(560). The root-variogram (Figure 3A) shows empirical computations (points) and fitted curves (solid lines), with the computations and fitting procedure described in Section 4.3. The root-variogram has three fitted parameters:
where k is a constant. In the data analysis we also consider the root-variogram normalized by the survey mean
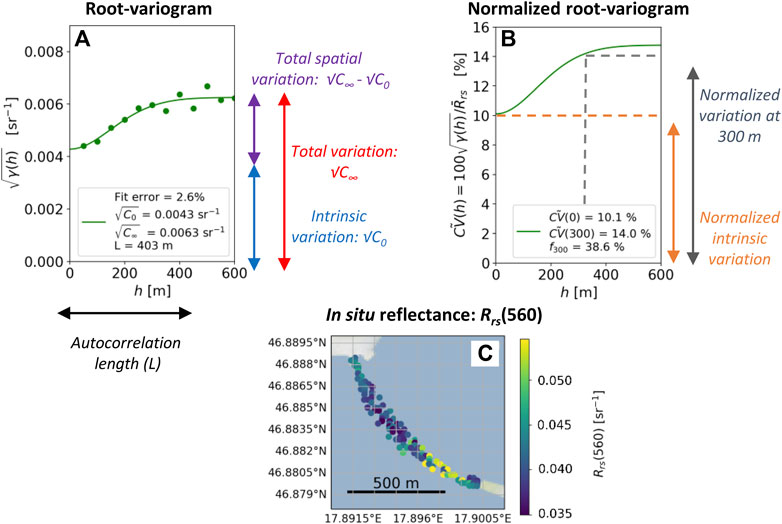
FIGURE 3. Annotated examples of (A) root-variogram,
Intrinsic variability represents variation in Rrs that is not due to measurement point separation. In the root-variogram, intrinsic variation is quantified by
Spatial variability represents variation in Rrs that occurs due to measurement point separation. The quantity
where the numerator,
Spatial autocorrelation. The autocorrelation length L quantifies the separation distance at which Rrs measurements cease to be spatially correlated with the original location. This is also the distance at which further increasing measurement point separation does not increase the variation in Rrs. In general, the definition of L can vary between variogram models and correspondingly the decay constant k in Eq. 7 can also take different definitions (Mälicke, 2022). Here we set k = 3 which corresponds to the fitted curve reaching ∼ 98% of the difference between the sill value
4.3 Computation and fitting of the variograms
Prior to the variogram analysis, geographic coordinates were converted to Universal Transverse Mercator coordinates using the WGS 84 ellipsoid. The variogram computations were performed using the Python geostatistics module (Mälicke 2022) using the inbuilt variogram function to compute γ(h) via Eq. 6. The computations select Rrs data from 12 discretized lag (h) bins. Following recommendations that the maximum lag must be order half the maximum point separation or less (Mälicke, 2022), the maximum possible lag of 600 m was used for Lake Balaton, corresponding to a bin width of δh = 50 m. For the other deployments a maximum lag of 1,500 m was used, corresponding to a bin width of δh = 125 m.
The fitting of Eq. 7 to the empirical variogram, which determines
5 Results
5.1 Variogram structure of in situ Rrs
We first illustrate how the spatial distribution of in situ Rrs relates to variogram structure, and how this can differ for different days within each deployment or between spectral bands. The examples from Lake Balaton (Figure 4) illustrate different degrees of spatial structure within the green band (Rrs(560)). The example in the top row (Figures 4A–C) has significantly more spatial variation than the bottom row (Figures 4D–F) indicated by the steeper curve and the greater difference between
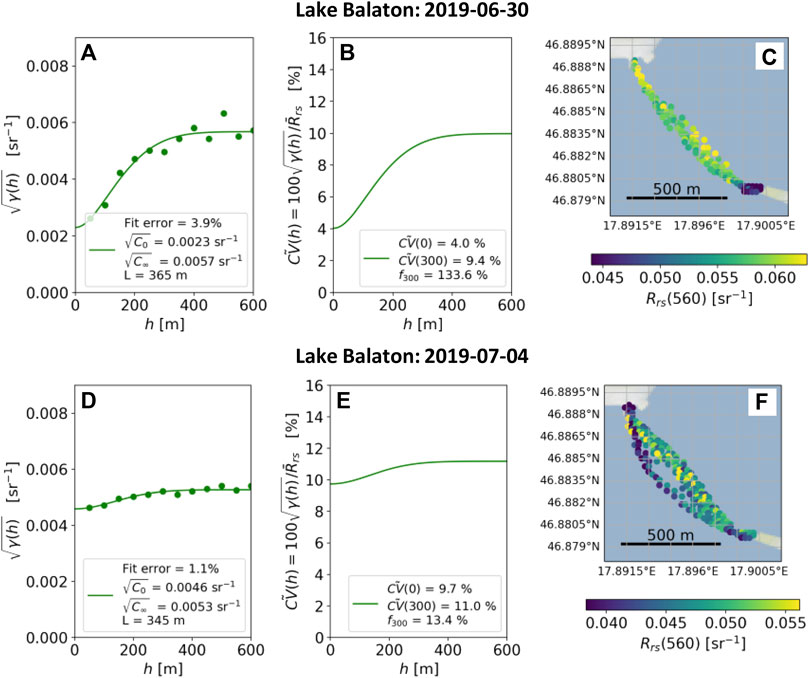
FIGURE 4. Illustrative examples of root-variograms
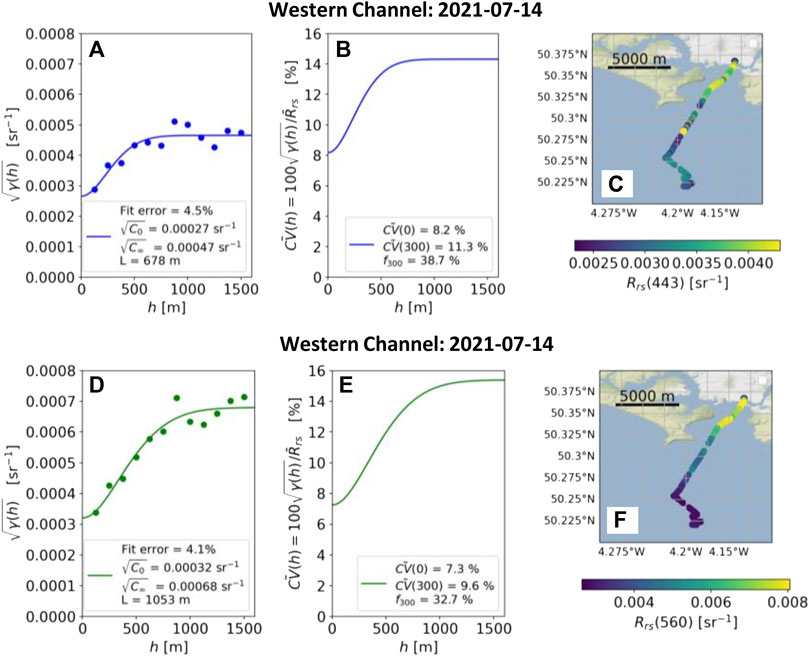
FIGURE 5. Illustrative examples of root-variograms
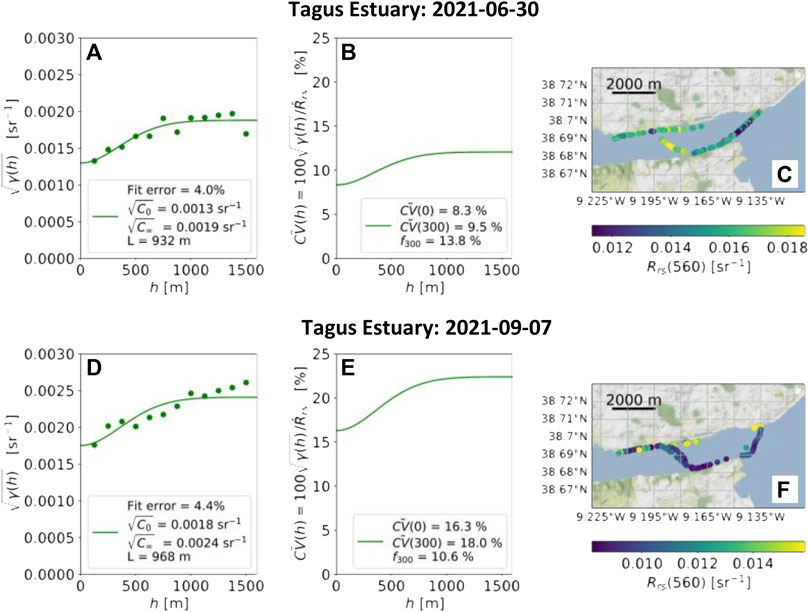
FIGURE 6. Illustrative examples of root-variograms
Figures 7, 8 show summaries of root-variograms
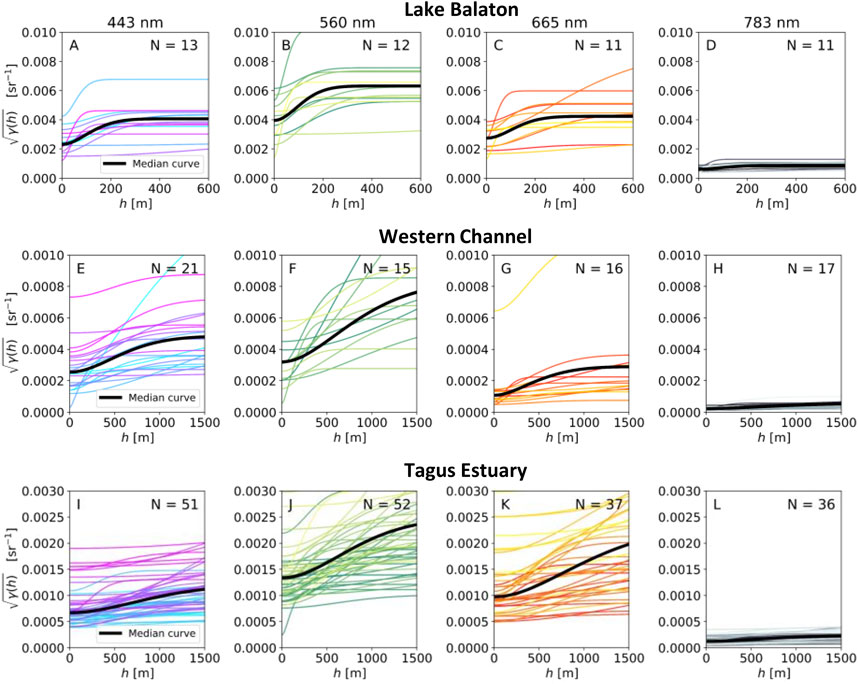
FIGURE 7. Summary of root-variograms
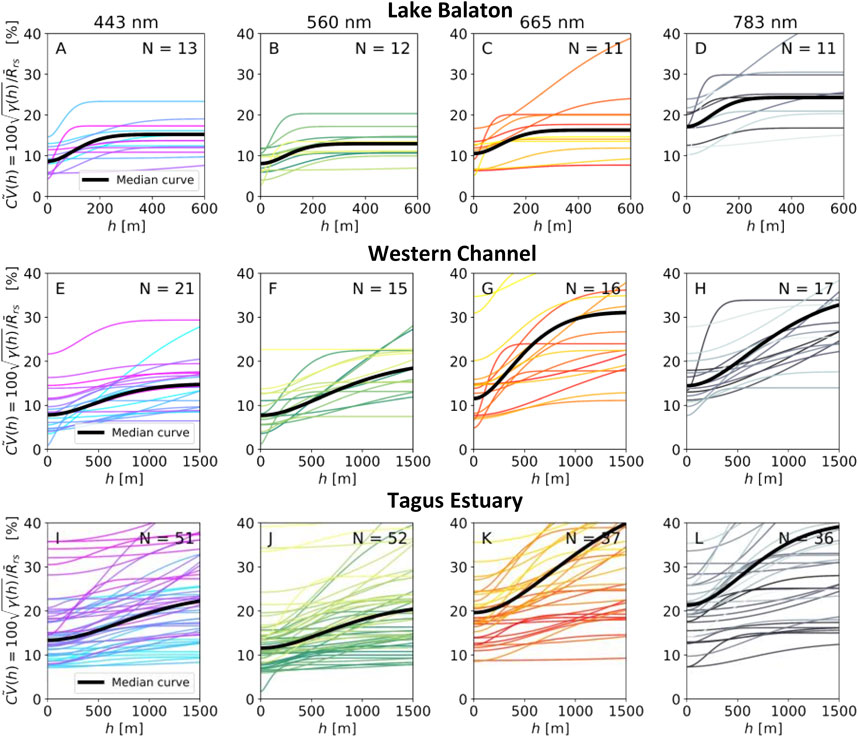
FIGURE 8. Summary of normalized root-variograms
The diversity of curves in each panel in Figures 7, 8 show that a range of variogram structure exists for each deployment and spectral band. However, some clear trends are present. Notably, the variogram curves from Lake Balaton have a markedly different shape than the other deployments, with the sill being reached at shorter length scales than the other deployments (note the different horizontal axis scale in Figure 7). This difference in variogram structure represents that spatial variation in Rrs occurs over a shorter length scale in Lake Balaton than other deployments. Additionally, the Tagus Estuary generally has a greater spread of variogram curves and higher relative variability than the Lake Balaton and Western Channel deployments.
5.2 Variogram statistics for spatial structure of in situ Rrs
Figure 9 shows frequency distributions for
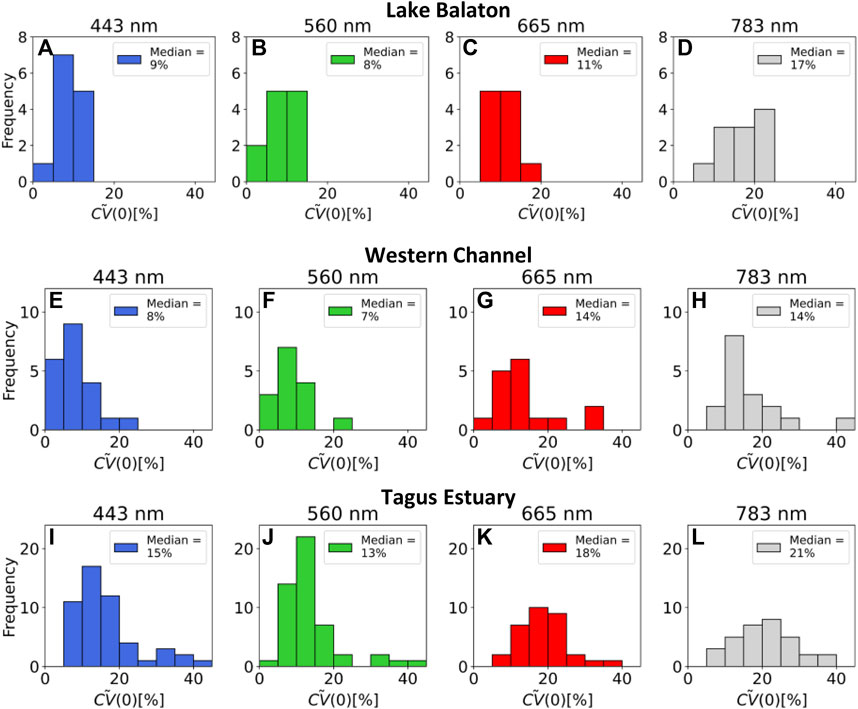
FIGURE 9. Frequency distributions of the intrinsic variation in Rrs as measured by
Figure 10 shows frequency distributions for f300, Eq. 8, for each deployment and spectral band. This parameter represents the fraction of Rrs variability due to measurement point separation at 300 m (chosen due to the pixel size of OLCI). The values of f300 are significantly higher for Lake Balaton than the other deployments, with median values
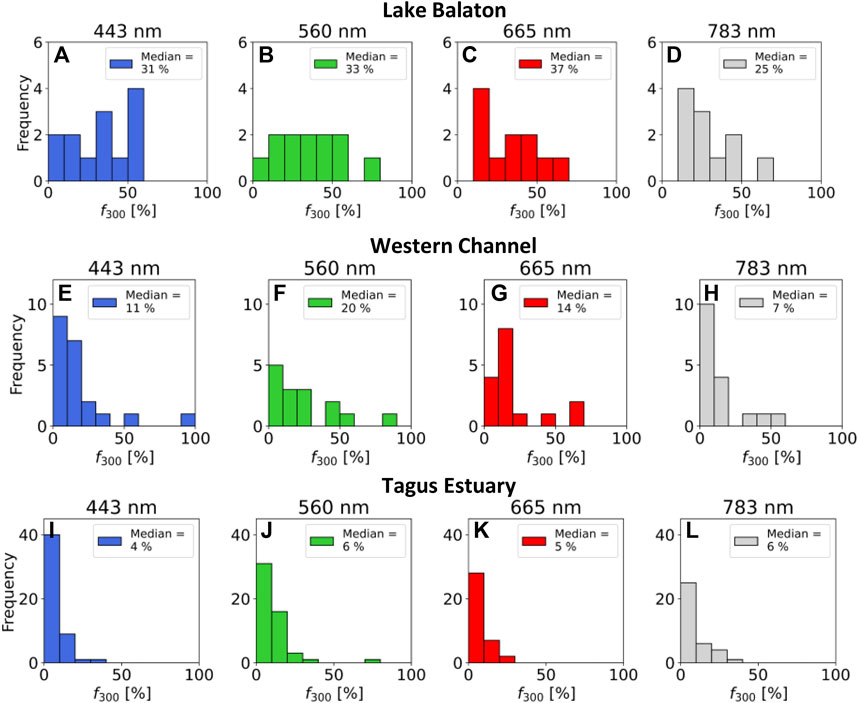
FIGURE 10. Frequency distributions of spatial variation in Rrs as measured by
Figure 11 shows frequency distributions for the autocorrelation length, L. Lake Balaton has lower values of L than the other deployments, with median values between 250 and 300 m. The Western Channel has median values of L between 1,000 and 500 m, with the Tagus Estuary between 1,800 and 2,500 m. In all panels the frequency distributions for L are broad, indicating that the spatial autocorrelation structure of in situ Rrs is changeable throughout the timescale of the deployment.
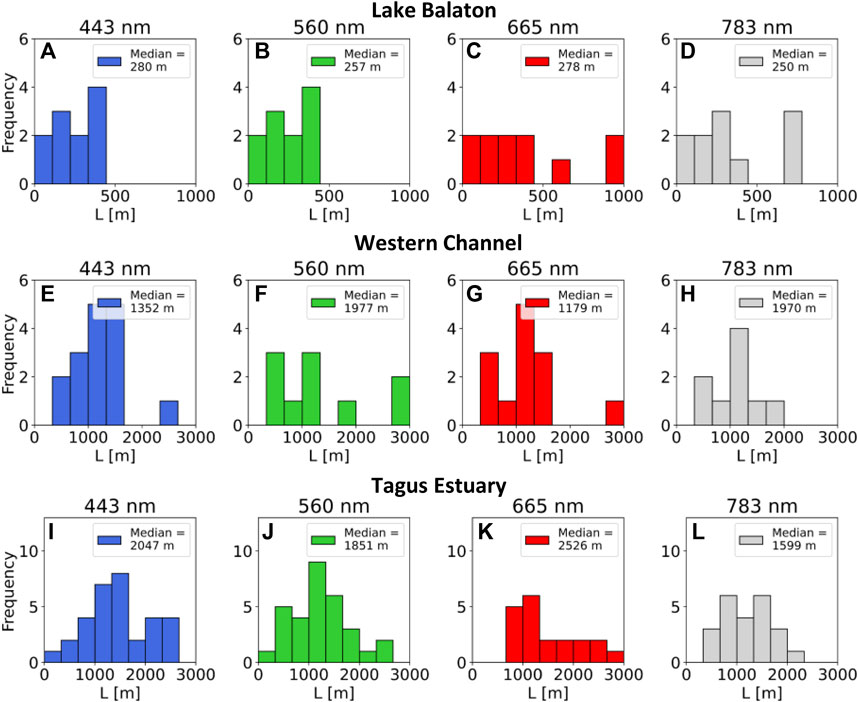
FIGURE 11. Frequency distributions of variogram autocorrelation lengths (L). Top row (A–D) Lake Balaton. Middle row (E–H) Western Channel. Bottom row (I–L) Tagus Estuary. This parameter represents the maximum distance at which in situ Rrs is considered spatially correlated with neighbouring observations. Outliers (L > 1,000 m in the top row and L > 3,000 m in the middle and bottom rows) are not shown.
We note that the results for f300 in Figure 10 and for L in Figure 11 hold for both unnormalized
6 Discussion
6.1 Comparison with previous variographic analyses of ocean colour and inland water quality
The spatial structure of Rrs (or related ocean colour variables) has been assessed in numerous past studies, based on satellite images (Yoder et al., 1987; Aurin et al., 2013; Glover et al., 2018), airborne (Bissett et al., 2004; Davis et al., 2007; Moses et al., 2016) and shipborne (Moses et al., 2016) radiometric platforms. The key novelty of our study is quantification of the spatial structure of in situ Rrs from autonomous radiometric systems deployed in the context of satellite validation. In coastal regions, reported autocorrelation lengths range from ∼ 1–100 km (Davis et al., 2007; Aurin et al., 2013; Moses et al., 2016), consistent with the Western Channel and Tagus estuary deployments in our study (Figure 11). For example, in near-coastal waters Moses et al. (2016) established that Rrs variability can increase rapidly when measured on scales of 10–100 m (up to a ∼ three-fold increase in the local coefficient of variation from a point measurement) with typical autocorrelation lengths being kilometer-scale. In Monterey Bay, California, Davis et al. (2007) showed autocorrelation lengths to be
Spatial structure analysis has also been applied to a diversity of chlorophyll measurements (which strongly correlates with Rrs) in inland water bodies. Using a chlorophyll index derived from airborne optical data Hedger et al. (2001) investigated spatial correlation in two Scottish Lochs (Loch Ness, and Loch Awe) reporting autocorrelation lengths between 500 and 1,300 m. Using discrete in situ Chlorophyll-a measurements, Yenilmez et al. (2014) reported autocorrelation length 1,200 m for the Porsuk Dam Reservoir in Turkey, and Anttila et al. (2008) reported autocorrelation lengths of 945 m and 1,357 m in Lake Vesijärvi, Finland. These autocorrelation lengths are slightly higher than the ferry transect we analysed in Lake Balaton (Figures 11A–D) suggesting that ∼ 1 km is a typical length scale of autocorrelation in inland waters.
There are examples of ocean colour variogram structure being more complex than the monotonically increasing Gaussian model used in this study. For example, Aurin et al. (2013) revealed the presence of ‘sub-sills’ (i.e., where the variogram levels out, then rises again), associated with sediment plumes. As we applied a goodness-of-fit filter to the Gaussian model, variograms that deviated significantly from a monotonic increase were filtered out. In comparing variograms between different ocean colour studies, it remains important to note that the transect-like data from shipborne or airborne sampling result in non-uniform spatial sampling, whereas a satellite image will be approximately uniform.
6.2 Opportunities and challenges of using mobile radiometers in satellite validation
Mobile radiometers deployed on ships-of-opportunity enable high-frequency spatial sampling of in situ Rrs in coastal and inland waters. In the context of satellite validation, the obvious advantage to using a mobile system (versus a fixed-platform deployment) is that it enables sampling of variation in in situ Rrs within a satellite pixel and across optical gradients. Quantifying within-pixel variability is important as nearshore waters are dynamic across short spatiotemporal scales which means that selecting spatially homogeneous sites for satellite validation is not always possible or desirable. Sampling in situ Rrs across optical gradients is particularly desirable to validate the response of algorithms over a wide biogeochemical concentration range. A specific challenge in improving validation practice from moving platforms is to account for spatial autocorrelation when selecting in situ data for match-ups with the same satellite image. In a wider context of data integration, it is also desirable to relate point-like in situ reflectance data to optical image data taken at different scales; ranging from drone flight imagery to high and moderate resolution imagery from satellites.
To quantify pixel-scale variation in in situ Rrs within our 4 or 6 h time windows, we introduced the parameter f300 which quantifies the percentage of in situ Rrs variation due to spatial separation at 300 m (representative of the medium resolution OLCI sensor). The advantage of using a mobile radiometric system to sample sub pixel variation was particularly clear for the Lake Balaton deployment, where f300 was greater than 30% across all spectral bands (median values in Figure 10). This was likely because spatial coherence of in situ Rrs was preserved throughout the time window. On the other hand, the Western Channel and Tagus Estuary deployments are more dynamic systems, which likely results in lower spatial coherence and lower values of f300. In tidal systems, fixed-monitoring stations are also able to sample spatial variation due to the flow of water (Salama et al., 2022), representing an alternative strategy to reduce uncertainty in a validation analysis.
Ultimately, it is the average value of in situ Rrs sampled within the extent of a satellite pixel that is compared to the satellite observation, and numerically relates to Δs in Eq. 4. The spatial sampling of the mobile radiometer will reduce the standard error on the pixel mean by a factor proportional to
In general, accounting for the spatial autocorrelation of in situ Rrs in validation of remotely-sensed reflectance becomes more important as the resolution of the satellite sensor increases. This is because neighbouring measurements become more likely to be located in adjacent pixels, rather than being averaged within the same pixel. If multiple match-up pairs are used from the same image, a simple approach to account for spatial autocorrelation is to downsample the match-up pairs, based on the autocorrelation length, L. Using Lake Balaton as an example, with a median value of L between 250 and 300 m, match-up pairs for high resolution sensors such as MSI could be downsampled to a 300 m spacing, thus reducing the effect of spatial autocorrelation, and thus providing a suitable number of match-up pairs. As the Lake Balaton autocorrelation lengths were generally comparable to the OLCI pixel scale downsampling is not necessary for OLCI match-up pairs from this area of Lake Balaton. For the other two deployments, downsampling match-up pairs to a separation distance of ∼ 2–3 km would be effective to reduce the effects of spatial autocorrelation.
Due to the breadth of the L distributions in Figure 11, and the range of reported water autocorrelation lengths in Section 6.1, it is desirable that downsampling uses the specific variogram on the day of data collection to set the separation distance for the downsampling of in situ Rrs. However, recognising that coincidence of remote and in situ observations is a rarity, as a rule of thumb ∼ 1 km is likely to be an appropriate separation distance for inland waters, with 1 km a minimum distance for coastal waters, being aware that sediment features may have much longer scales of autocorrelation (Aurin et al., 2013). Additionally, spatial structure analysis could be applied to climatologically-averaged ocean colour products to produce a map representing a first-order approximation of spatial autocorrrelation. Downsampling represents just one approach to account for spatial autocorrelation in validation statistics. Alternatively, validation statistics could be modified for spatial autocorrelation; for example, spatially-weighted regression methods (Anselinm and Bera, 1998).
As chlorophyll-a concentration often controls the majority of the variability in Rrs, it typically results in a high degree of co-variance between spectral bands (e.g., Cael et al., 2023). This is reflected in the variogram statistics in Section 5.2 which show similarity between spectral bands, although band differences can exist (e.g., as shown for the Western Channel in Figures 10, 11) when there is variation in the spectral shape of Rrs (Figure 1C). We anticipate that variogram statistics could have the most pronounced differences between spectral bands in optically complex waters, where higher concentrations of suspended sediment and CDOM are present. We therefore recommend continued evaluation of variogram statistics across reflectance bands in coastal and inland waters, until more general conclusions can be drawn on differences between spectral bands.
6.3 Relationship between intrinsic variation and uncertainty of Rrs
In this study, we used the metric
Measures of Rrs variability provide only a proxy for uncertainty. Formal uncertainty assessment from fiducial reference measurements refers to a component-by-component propagation of sources of uncertainty, traceable to metrology standards (Ruddick et al., 2019; Banks et al., 2020). Individual sources of uncertainty include radiometric calibration, sensor characteristics (e.g., straylight, non-linearity, thermal response), and the uncertainty of the Rrs estimation method, which is impacted by environmental conditions: i.e., cloud cover and windspeed (surface roughness). Environmental sources of uncertainty are typically half of the total uncertainty budget (Lin et al., 2022), with higher windspeeds and scattered cloud leading to higher uncertainties. Variable environmental conditions are therefore likely to be a dominant factor influencing the spread of
6.4 Limitations of variogram analysis and future work
Due to computing the variogram over a 4 or 6 h time window, there are temporal sources of variation that are present in the analysis. These include variation in Rrs due to changing solar zenith and relative azimuth (Mobley, 1999), as well as natural temporal fluctuations in water constituent concentrations. Casting the analysis in terms of the normalized water leaving radiance, which adjusts for Rrs variable zenith angle and atmospheric attenuation (Gordon and Wang, 1994), would give a more accurate picture of how the water properties vary spatially. Additionally, the variogram structure will be impacted by temporal variation. For example, in the Tagus Estuary deployment, the relatively high variation in in situ Rrs at shorter length scales (Figure 9) is hypothesised to be partially influenced by the ship revisiting a location where Rrs changed temporally due to tidal influence (see neighbouring transects collected at different times in Figure 1F). Temporal Rrs differences between neighbouring transects is also likely to have an impact on the spatial structure analysis from Lake Balaton.
An important caveat to the variogram analysis is that the ship transects do not uniformly sample across the survey area, or each pixel. Therefore, the variogram structure is anticipated to be specific to the path of the ship within each deployments, and is likely to be very different for alternative ship paths in the same water body. Additionally, due to the quality control of in situ Rrs (Section 3.2) the effective temporal sampling frequency is also subject to change, because not all observations taken along a transect are suitable to derive estimates of Rrs.
A future research direction is to extend the “static” variogram analysis to the temporal dimension, and hence enable spatial and temporal autocorrelation to be considered in a unified way. It is likely this will require the data sampling strategy to be developed around the analysis method; i.e., obtaining repeat in situ measurements at a set of fixed locations that can be used to robustly window the variograms. Alternatively, a sequence of images from geostationary satellites which have hourly revisit times (e.g., Choi et al., 2012) could be used to gain insight.
7 Summary and conclusion
This study quantified the spatial structure of in situ Rrs from mobile radiometers deployed in coastal and inland water bodies. The overarching aim was to inform how spatial statistics can be used to aid in satellite validation practice where ship-transect data is used. A first focus was quantifying how mobile radiometers can reduce variability via sub-pixel sampling and a second focus was quantifying spatial autocorrelation, and thereby informing in situ data selection for match-up analysis.
There were pronounced differences in spatial structure between the deployments, within each deployment, as well as more subtle differences between spectral bands. At a 300 m length scale (typical pixel size of a medium resolution ocean colour satellite sensor) we showed that typically 5%–35% of the total variation in in situ Rrs was due to the spatial separation of measurements. For validation of medium-resolution sensors, mobile radiometers therefore provide a distinct advantage in generating more spatially representative data than a fixed platform, reducing the contribution of in situ variability in the validation process. The autocorrelation length, which informs an ideal minimum separation distance for in situ Rrs in validation, ranged from ∼ 100–1,000 m. Consequently, validation of high-resolution sensors (sub 100 m pixel size) requires either downsampling of in situ data to ensure spatial independence or for validation statistics to take spatial autocorrelation into account.
In the future, we anticipate that spatial statistics will become increasingly important for both validation and data integration of aquatic reflectance across multiple sensor systems, including spaceborne, shipborne, airborne, and ground-based platforms.
Data availability statement
The datasets presented in this study can be found in online repositories. The names of the repository/repositories and accession number(s) can be found below: https://pypi.org/project/monda/; https://github.com/tjor/SR_variograms.
Author contributions
TJ, SS, and NS contributed to conception and design of the study. TJ led the data analysis with contributions from SS and NS. SS, VM-V, GS, and FI designed the field deployments and collected data. TJ led the writing of the manuscript with contributions from all authors. SS obtained the primary funding and administered the project. All authors contributed to the article and approved the submitted version.
Funding
This project received primary funding from the European Union’s Horizon 2020 research and innovation programme under grant agreement No 776480 (MONOCLE: Multiscale Observation Networks for Optical monitoring of Coastal waters, Lakes and Estuaries), https://monocle-h2020.eu. TJ and VM-V were partially supported by Hyperspectral Bio-Optical Observations Sailing on Tara (HyperBOOST) (ESA contract number: 4000135756/21/I-EF). GS and FI acknowledge funding from from Horizon 2020 grant agreement No 870349 (CERTO: Copernicus Evolution: Research for harmonised and Transitional water Observation). TJ was partially funded by NERC through its Earth Observation Data Acquisition and Analysis Service (NEODAAS), award number NE/S013377/1.
Acknowledgments
We would like to thank our reviewers whose thorough and constructive comments greatly improved our publication.
Conflict of interest
The authors declare that the research was conducted in the absence of any commercial or financial relationships that could be construed as a potential conflict of interest.
Publisher’s note
All claims expressed in this article are solely those of the authors and do not necessarily represent those of their affiliated organizations, or those of the publisher, the editors and the reviewers. Any product that may be evaluated in this article, or claim that may be made by its manufacturer, is not guaranteed or endorsed by the publisher.
References
Albert, A., and Mobley, C. (2003). An analytical model for subsurface irradiance and remote sensing reflectance in deep and shallow case-2 waters. Opt. Express 11, 2873–2890. doi:10.1364/OE.11.002873
Anselinm, L., and Bera, A. K. (1998). Spatial dependence in linear regression models with an introduction to spatial econometrics. CRC Press, 237–290. chap. 7. doi:10.1201/9781482269901-36
Anttila, S., Kairesalo, T., and Pellikka, P. (2008). A feasible method to assess inaccuracy caused by patchiness in water quality monitoring. Environ. Monit. Assess. 142, 11–22. doi:10.1007/s10661-007-9904-y
Aurin, D., Mannino, A., and Franz, B. (2013). Spatially resolving ocean color and sediment dispersion in river plumes, coastal systems, and continental shelf waters. Remote Sens. Environ. 137, 212–225. doi:10.1016/j.rse.2013.06.018
Bachmaier, M., and Backes, M. (2011). Variogram or semivariogram? Variance or semivariance? Allan variance or introducing a new term? Math. Geosci. 43, 735–740. doi:10.1007/s11004-011-9348-3
Bailey, S. W., and Werdell, P. J. (2006). A multi-sensor approach for the on-orbit validation of ocean color satellite data products. Remote Sens. Environ. 102, 12–23. doi:10.1016/j.rse.2006.01.015
Banks, A. C., Vendt, R., Alikas, K., Bialek, A., Kuusk, J., Lerebourg, C., et al. (2020). Fiducial reference measurements for satellite ocean colour (FRM4SOC). Remote Sens. 12, 1322. doi:10.3390/rs12081322
Barnes, B. B., Cannizzaro, J., English, D., and Hu, C. (2019). Validation of VIIRS and MODIS reflectance data in coastal and oceanic waters: an assessment of methods. Remote Sens. Environ. 220, 110–123. doi:10.1016/J.RSE.2018.10.034
Bissett, W. P., Arnone, R. A., Davis, C. O., Dickey, T. D., Dye, D., Kohler, D. D., et al. (2004). From meters to kilometers: a look at ocean-color scales of variability, spatial coherence, and the need for fine-scale remote sensing in coastal ocean optics. Oceanography 17 (2), 32–43. doi:10.5670/oceanog.2004.45
Brewin, R. J., Dall’Olmo, G., Pardo, S., van Dongen-Vogels, V., and Boss, E. S. (2016). Underway spectrophotometry along the atlantic meridional transect reveals high performance in satellite chlorophyll retrievals. Remote Sens. Environ. 183, 82–97. doi:10.1016/j.rse.2016.05.005
Bulgarelli, B., Kiselev, V., and Zibordi, G. (2014). Simulation and analysis of adjacency effects in coastal waters: a case study. Appl. Opt. 53, 1523–1545. doi:10.1364/AO.53.001523
Cael, B. B., Bisson, K., Boss, E., and Erickson, Z. K. (2023). How many independent quantities can be extracted from ocean color? Limnol. Oceanogr. Lett. 8, 603–610. doi:10.1002/lol2.10319
Choi, J.-K., Park, Y. J., Ahn, J. H., Lim, H.-S., Eom, J., and Ryu, J.-H. (2012). Goci, the world’s first geostationary ocean color observation satellite, for the monitoring of temporal variability in coastal water turbidity. J. Geophys. Res. Oceans 117. doi:10.1029/2012JC008046
Concha, J. A., Bracaglia, M., and Brando, V. E. (2021). Assessing the influence of different validation protocols on ocean colour match-up analyses. Remote Sens. Environ. 259, 112415. doi:10.1016/j.rse.2021.112415
Cressie, N. A. C. (1993). Statistics for spatial data. Revised Edition. John Wiley and Sons, 27–104. chap. 2. doi:10.1002/9781119115151
Davis, C. O., Kavanaugh, M., Letelier, R., Bissett, W. P., and Kohler, D. (2007). “Spatial and spectral resolution considerations for imaging coastal waters,” in Coastal Ocean remote sensing. Editors R. J. Frouin, and Z. Lee (International Society for Optics and Photonics), 6680, 196–207. doi:10.1117/12.734288
Glover, D. M., Doney, S. C., Oestreich, W. K., and Tullo, A. W. (2018). Geostatistical analysis of mesoscale spatial variability and error in seaWiFS and MODIS/aqua global ocean color data. J. Geophys. Res. Oceans 123, 22–39. doi:10.1002/2017JC013023
Gordon, H. R., and Wang, M. (1994). Retrieval of water-leaving radiance and aerosol optical thickness over the oceans with seawifs: a preliminary algorithm. Appl. Opt. 33, 443–452. doi:10.1364/AO.33.000443
Gregg, W. W., and Carder, K. L. (1990). A simple spectral solar irradiance model for cloudless maritime atmospheres. Limnol. Oceanogr. 35, 1657–1675. doi:10.4319/lo.1990.35.8.1657
Groetsch, P. M. M., Foster, R., and Gilerson, A. (2020). Exploring the limits for sky and sun glint correction of hyperspectral above-surface reflectance observations. Appl. Opt. 59, 2942–2954. doi:10.1364/AO.385853
Groetsch, P. M. M., Gege, P., Simis, S. G. H., Eleveld, M. A., and Peters, S. W. M. (2017). Validation of a spectral correction procedure for sun and sky reflections in above-water reflectance measurements. Opt. Express 25, A742–A761. doi:10.1364/OE.25.00A742
Groom, S., Sathyendranath, S., Ban, Y., Bernard, S., Brewin, R., Brotas, V., et al. (2019). Satellite ocean colour: current status and future perspective. Front. Mar. Sci. 6, 1–30. doi:10.3389/fmars.2019.00485
Hedger, R. D., Atkinson, P. M., and Malthus, T. J. (2001). Optimizing sampling strategies for estimating mean water quality in lakes using geostatistical techniques with remote sensing. Lakes Reservoirs Sci. Policy Manag. Sustain. Use 6, 279–288. doi:10.1046/j.1440-1770.2001.00159.x
Ilori, C. O., Pahlevan, N., and Knudby, A. (2019). Analyzing performances of different atmospheric correction techniques for landsat 8: application for coastal remote sensing. Remote Sens. 11, 469. doi:10.3390/rs11040469
IOCCG (2019). Uncertainties in ocean colour remote sensing. Int. Ocean. Colour. Coord. Group (IOCCG) 18. doi:10.25607/OBP-696
Jordan, T. M., Simis, S. G. H., Grötsch, P. M. M., and Wood, J. (2022). Incorporating a hyperspectral direct-diffuse pyranometer in an above-water reflectance algorithm. Remote Sens. 14, 2491. doi:10.3390/rs14102491
Justice, C., Belward, A., Morisette, J., Lewis, P., Privette, J., and Baret, F. (2000). Developments in the ’validation’ of satellite sensor products for the study of the land surface. Int. J. Remote Sens. 21, 3383–3390. doi:10.1080/014311600750020000
Kutser, T. (2012). The possibility of using the landsat image archive for monitoring long time trends in coloured dissolved organic matter concentration in lake waters. Remote Sens. Environ. 123, 334–338. doi:10.1016/j.rse.2012.04.004
Lee, Z., Hu, C., Arnone, R., and Liu, Z. (2012). Impact of sub-pixel variations on ocean color remote sensing products. Opt. Express 20, 20844–20854. doi:10.1364/OE.20.020844
Lehmann, G., Pahlevan, N., Alikas, K., Conroy, T., and Anstee, J. (2023). Gloria - a globally representative hyperspectral in situ dataset for optical sensing of water quality. Sci. Data 10, 100. doi:10.1038/s41597-023-01973-y
Lin, J., Dall’Olmo, G., Tilstone, G. H., Brewin, R. J. W., Vabson, V., Ansko, I., et al. (2022). Derivation of uncertainty budgets for continuous above-water radiometric measurements along an atlantic meridional transect. Opt. Express 30, 45648–45675. doi:10.1364/OE.470994
Loew, A., Bell, W., Brocca, L., Bulgin, C. E., Burdanowitz, J., Calbet, X., et al. (2017). Validation practices for satellite-based earth observation data across communities. Rev. Geophys. 55, 779–817. doi:10.1002/2017RG000562
Mälicke, M. (2022). Scikit-gstat 1.0: a scipy-flavored geostatistical variogram estimation toolbox written in python. Geosci. Model. Dev. 15, 2505–2532. doi:10.5194/gmd-15-2505-2022
Martinez-Vicente, V., Simis, S. G. H., Alegre, R., Land, S., and Groom, P. E. (2013). Above-water reflectance for the evaluation of adjacency effects in earth observation data: initial results and methods comparison for near-coastal waters in the western channel, UK. J. Euro. Opt. Soc. 8, 13060. doi:10.2971/jeos.2013.13060
Mobley, C. D. (1999). Estimation of the remote-sensing reflectance from above-surface measurements. Appl. Opt. 38, 7442–7455. doi:10.1364/AO.38.007442
Montes, M., Pahlevan, N., Giles, D. M., Roger, J.-C., Zhai, P.-w., Smith, B., et al. (2022). Augmenting heritage ocean-color aerosol models for enhanced remote sensing of inland and nearshore coastal waters. Front. Remote Sens. 3. doi:10.3389/frsen.2022.860816
Moses, W. J., Ackleson, S. G., Hair, J. W., Hostetler, C. A., and Miller, W. D. (2016). Spatial scales of optical variability in the coastal ocean: implications for remote sensing and in situ sampling. J. Geophys. Res. Oceans 121, 4194–4208. doi:10.1002/2016JC011767
Otterman, J., and Fraser, R. S. (1979). Adjacency effects on imaging by surface reflection and atmospheric scattering: cross radiance to zenith. Appl. Opt. 18, 2852–2860. doi:10.1364/AO.18.002852
Pahlevan, N., Mangin, A., Balasubramanian, S. V., Smith, B., Alikas, K., Arai, K., et al. (2021). Acix-aqua: a global assessment of atmospheric correction methods for landsat-8 and sentinel-2 over lakes, rivers, and coastal waters. Remote Sens. Environ. 258, 112366. doi:10.1016/j.rse.2021.112366
Pitarch, J., Talone, M., Zibordi, G., and Groetsch, P. (2020). Determination of the remote-sensing reflectance from above-water measurements with the 3c model: a further assessment. Opt. Express 28, 15885–15906. doi:10.1364/OE.388683
Qin, P., Simis, S. G., and Tilstone, G. H. (2017). Radiometric validation of atmospheric correction for meris in the baltic sea based on continuous observations from ships and aeronet-oc. Remote Sens. Environ. 200, 263–280. doi:10.1016/j.rse.2017.08.024
Román, M. O., Schaaf, C. B., Woodcock, C. E., Strahler, A. H., Yang, X., Braswell, R. H., et al. (2009). The MODIS (collection V005) BRDF/albedo product: assessment of spatial representativeness over forested landscapes. Remote Sens. Environ. 113, 2476–2498. doi:10.1016/j.rse.2009.07.009
Ruddick, K. G., Voss, K., Boss, E., Castagna, A., Frouin, R., Gilerson, A., et al. (2019). A review of protocols for fiducial reference measurements of water-leaving radiance for validation of satellite remote-sensing data over water. Remote Sens. 11, 2198. doi:10.3390/rs11192198
Ryan, J. C., Hubbard, A., Irvine-Fynn, T. D., Doyle, S. H., Cook, J. M., Stibal, M., et al. (2017). How robust are in situ observations for validating satellite-derived albedo over the dark zone of the Greenland ice sheet? Geophys. Res. Lett. 44, 6218–6225. doi:10.1002/2017GL073661
Salama, M. S., Spaias, L., Poser, K., Peters, S., and Laanen, M. (2022). Validation of sentinel-2 (MSI) and sentinel-3 (OLCI) water quality products in turbid estuaries using fixed monitoring stations. Front. Remote Sens. 2. doi:10.3389/frsen.2021.808287
Salama, M. S., and Stein, A. (2009). Error decomposition and estimation of inherent optical properties. Appl. Opt. 48, 4947–4962. doi:10.1364/AO.48.004947
Salama, M. S., and Su, Z. (2011). Resolving the subscale spatial variability of apparent and inherent optical properties in ocean color match-up sites. IEEE Trans. Geoscience Remote Sens. 49, 2612–2622. doi:10.1109/TGRS.2011.2104966
Siegel, D. A., Wang, M., Maritorena, S., and Robinson, W. (2000). Atmospheric correction of satellite ocean color imagery: the black pixel assumption. Appl. Opt. 39, 3582–3591. doi:10.1364/AO.39.003582
Simis, S., Jackson, T., Jordan, T., Peters, S., and Ghebrehiwot, S. (2022). Monda: monocle data analysis python package: so-rad. Available at: https://github.com/monocle-h2020/MONDA.
Simis, S. G. H., and Olsson, J. (2013). Unattended processing of shipborne hyperspectral reflectance measurements. Remote Sens. Environ. 135, 202–212. doi:10.1016/j.rse.2013.04.001
Spyrakos, E., O’Donnell, R., Hunter, P. D., Miller, C., Scott, M., Simis, S. G. H., et al. (2018). Optical types of inland and coastal waters. Limnol. Oceanogr. 63, 846–870. doi:10.1002/lno.10674
Wang, M., Son, S., and Shi, W. (2009). Evaluation of MODIS SWIR and NIR-SWIR atmospheric correction algorithms using seabass data. Remote Sens. Environ. 113, 635–644. doi:10.1016/j.rse.2008.11.005
Warren, M. A., Simis, S. G. H., Martinez-Vicente, V., Poser, K., Bresciani, M., Alikas, K., et al. (2019). Assessment of atmospheric correction algorithms for the sentinel-2a multispectral imager over coastal and inland waters. Remote Sens. Environ. 225, 267–289. doi:10.1016/j.rse.2019.03.018
Wright, A., and Simis, S. G. H. (2021). Construction of the solar-tracking radiometry platform (so-rad). doi:10.5281/zenodo.4485805
Yenilmez, F., Düzgün, S., and Aksoy, A. (2014). An evaluation of potential sampling locations in a reservoir with emphasis on conserved spatial correlation structure. Environ. Monit. Assess. 187, 4216. doi:10.1007/s10661-014-4216-5
Yoder, J. A., McClain, C. R., Blanton, J. O., and Oeymay, L.-Y. (1987). Spatial scales in czcs-chlorophyll imagery of the southeastern u.s. continental shelf. Limnol. Oceanogr. 32, 929–941. doi:10.4319/lo.1987.32.4.0929
Zibordi, G., Kwiatkowska, E., Mélin, F., Talone, M., Cazzaniga, I., Dessailly, D., et al. (2022). Assessment of OLCI-A and OLCI-B radiometric data products across european seas. Remote Sens. Environ. 272, 112911. doi:10.1016/j.rse.2022.112911
Keywords: satellite validation, optical radiometry, above-water reflectance, autonomous monitoring, variogram, spatial structure, water quality
Citation: Jordan TM, Simis SGH, Selmes N, Sent G, Ienna F and Martinez-Vicente V (2023) Spatial structure of in situ reflectance in coastal and inland waters: implications for satellite validation. Front. Remote Sens. 4:1249521. doi: 10.3389/frsen.2023.1249521
Received: 28 June 2023; Accepted: 20 October 2023;
Published: 09 November 2023.
Edited by:
Kevin Ruddick, Royal Belgian Institute of Natural Sciences, BelgiumReviewed by:
Juan Ignacio Gossn, European Organisation for the Exploitation of Meteorological Satellites, GermanyTim J. Malthus, Oceans and Atmosphere (CSIRO), Australia
Susanne Elizabeth Craig, National Aeronautics and Space Administration, United States
Copyright © 2023 Jordan, Simis, Selmes, Sent, Ienna and Martinez-Vicente. This is an open-access article distributed under the terms of the Creative Commons Attribution License (CC BY). The use, distribution or reproduction in other forums is permitted, provided the original author(s) and the copyright owner(s) are credited and that the original publication in this journal is cited, in accordance with accepted academic practice. No use, distribution or reproduction is permitted which does not comply with these terms.
*Correspondence: Thomas M. Jordan, tjor@pml.ac.uk