- 1Department of Physical Medicine and Rehabilitation, Harvard Medical School, Boston, MA, United States
- 2Spaulding Rehabilitation Hospital and Spaulding Research Institute, Charlestown, MA, United States
- 3Home Base, A Red Sox Foundation and Massachusetts General Hospital Program, Charlestown, MA, United States
- 4Department of Psychology, University of Kentucky, Lexington, KY, United States
Objective: Low scores on neuropsychological tests are considered objective evidence of mild cognitive impairment. In clinical practice and research, it can be challenging to identify a cognitive deficit or mild cognitive impairment in high-functioning people because they are much less likely to obtain low test scores. This study was designed to improve the methodology for identifying mild cognitive impairment in adults who have above average or superior intellectual abilities.
Method: Participants completed the National Institutes of Health Toolbox for the Assessment of Neurological and Behavioral Function Cognition Battery (NIHTB-CB). The sample included 384 adults between the ages of 20 and 85 who had completed either a 4-year college degree or who scored in the above average, superior, or very superior range on a measure of intellectual functioning, the Crystallized Composite score. Algorithms were developed, based on the absence of high scores and the presence of low scores, for identifying mild cognitive impairment.
Results: Base rate tables for the presence of low scores and the absence of high scores are provided. The base rate for people with high average crystalized ability obtaining any one of the following, 5 scores <63rd percentile, or 4+ scores <50th percentile, or 3+ scores ≤ 25th percentile, or 2+ scores ≤ 16th percentile, is 15.5%.
Conclusions: Algorithms were developed for identifying cognitive weakness or impairment in high-functioning people. Research is needed to test them in clinical groups, and to assess their association with clinical risk factors for cognitive decline and biomarkers of acquired neurological or neurodegenerative diseases.
Introduction
Deficit measurement is the sine qua non of clinical neuropsychology. Low scores on neuropsychological tests are used to define a cognitive deficit or mild cognitive impairment (Heaton et al., 1991, 2004; Reitan and Wolfson, 1993; Petersen et al., 1999; Dubois et al., 2007). However, if many tests are administered, most healthy adults and older adults will obtain one or more low scores (Palmer et al., 1998; Axelrod and Wall, 2007; Schretlen et al., 2008; Binder et al., 2009; Brooks et al., 2009a, 2010, 2011). In fact, for healthy adults of average intelligence, with no known form of cognitive impairment, it is common to obtain up to 20–25% of their test scores, across a battery of tests, at or below one standard deviation (SD) from the mean (Brooks et al., 2009a, 2011). Even within a single cognitive domain, such as memory or executive function, it is common for healthy children, adults, and older adults to obtain one or more low test scores (Brooks et al., 2008, 2009b,c; Karr et al., 2017, 2018; Cook et al., 2019). This makes it challenging to accurately identify mild cognitive impairment (Petersen et al., 1999; Albert et al., 2011) or mild neurocognitive disorder, based on the Diagnostic and Statistical Manual of Mental Disorders, Fifth Edition (DSM-5) criteria (American Psychiatric Association, 2013), because these diagnostic criteria require test performance that is greater than one SD from the mean, but the criteria do not specify exactly how that is determined—such as whether one or more test scores in this range are required.
There is a strong association between higher intelligence and higher neuropsychological test scores (Warner et al., 1987; Tremont et al., 1998; Horton, 1999; Steinberg et al., 2005a,b). In clinical practice and research, it can be challenging to identify a cognitive deficit or mild cognitive impairment in high-functioning people because they are much less likely to obtain low test scores (Brooks et al., 2009a, 2011), and a much greater change in functioning needs to occur, as a result of a neurological disease, before they perform one or more SDs below the normative mean. Therefore, in some high-functioning people, it might be the absence of high scores, more so than the presence of low scores, that reveals their cognitive decline.
This study was designed to improve the methodology for identifying mild cognitive impairment in adults who have above average or superior intellectual abilities. The adult standardization sample for the National Institutes of Health Toolbox for the Assessment of Neurological and Behavioral Function (Gershon et al., 2010, 2013) Cognition Battery (NIHTB-CB) (Weintraub et al., 2013) was used to develop algorithms for defining cognitive impairment. This 30-min battery is comprised of seven tests measuring attention, working memory, language, processing speed, and executive functioning. The algorithms incorporate concepts from recent studies illustrating that the absence of high scores is uncommon in high-functioning people (Karr and Iverson, 2020; Karr et al., 2020). For example, considering the five fluid scores from the NIH Toolbox, only 17–19% of adults with above average or superior intelligence will have no above average fluid cognition scores (Karr and Iverson, 2020). Therefore, in high-functioning people, cognitive deficits might be reflected by the presence of low scores, the absence of high scores, or both. This study will combine criteria relating to both low scores (Holdnack et al., 2017) and high scores (Karr and Iverson, 2020) to propose a new method for identifying mild cognitive impairment in adults with above average or superior intellectual abilities.
Method
Participants
The normative sample for the NIHTB-CB (Gershon, 2016) includes 1,021 adult participants between the ages of 20 and 89, of whom 843 completed all seven tests. Previously published studies reported low and high score base rates using the entire NIHTB-CB normative sample, including those with pre-existing neurodevelopmental, psychiatric, substance use, and neurological disorders (Holdnack et al., 2017; Karr and Iverson, 2020), whereas these base rates were re-calculated for the current study including only those participants who did not report any of these pre-existing conditions. Participants were excluded from analysis if they reported (a) a pre-existing neurodevelopmental disorder, including a specific learning disability (n = 9), attention-deficit/hyperactivity disorder (n = 16), Asperger's syndrome (n = 1), or a developmental delay (n = 1); (b) a psychiatric or substance use disorder, including a serious emotional disturbance (n = 8), bipolar disorder or schizophrenia (n = 8), depression or anxiety (n = 92), alcohol abuse (n = 3), drug abuse (n = 5), or a hospitalization due to emotional problems (n = 7); or (c) a neurological disorder, including epilepsy or seizures (n = 5), traumatic brain injury (n = 1), multiple sclerosis (n = 1), a stroke or transient ischemic attack (n = 7), or a history of brain surgery (n = 8). Some participants had more than one of these conditions. This resulted in a final sample of 730 participants (age: M = 47.4 years, SD = 17.6, range: 18 to 85; 35.6% men, 64.4% women; education: M = 14.2 years, SD = 2.5). The racial and ethnic breakdown of the sample was as follows: 63.1% White, 17.7% African American, 9.7% Latinx, 4.0% Asian or Pacific Islander, 1.6% Multiracial, 1.0% Native American, 1.0% Afro-Latinx, and 1.9% not provided. A subsample of these participants (n = 687) had sufficient data to calculate demographic-adjusted T scores (age: M = 47.8 years, SD = 17.6, range: 18–85; 35.7% men, 64.3% women; education: M = 14.3 years, SD = 2.5). The racial and ethnic breakdown of that sample was as follows: 67.1% White, 18.6% African American, 10.3% Latinx, and 3.9% Asian.
Of the samples described above, 394 met at least one of the following criteria: (a) having 16 or more years of education, (b) obtaining an age-adjusted Crystallized Composite Standard Score of 110 or greater, or (c) obtaining a demographic-adjusted Crystallized Composite T score of 57 or greater. The average age of this sample was 47.0 years (SD = 17.2) and the sample includes 38.1% men and 61.9% women. Their average education was 15.7 years (SD = 2.2). The racial and ethnic breakdown of the sample was as follows: 66.8% White, 16.5% African American, 10.2% Latinx, 4.6% Asian, 0.8% Multiracial, 0.3% Afro-Latinx, and 0.3% Native American. This sample was used to prepare algorithms for identifying cognitive impairment in high-functioning people.
Measures
The NIHTB-CB includes seven tests, from which three composites are derived by averaging normalized scores: the Total Composite, the Crystallized Composite, and the Fluid Composite. The Total Composite is derived from all seven tests, whereas the other composites are derived from a subset of scores. The Crystallized Composite is composed of two tests: Picture Vocabulary and Oral Reading Recognition. These tests have been shown to correlate with tests of word reading and receptive vocabulary (Gershon et al., 2014), which correlate with intelligence and are commonly used as estimates of premorbid intellectual functioning. In this study, the Crystallized Composite score serves as our estimate of a person's level of intelligence. The Fluid Composite is composed of five tests: a measure of working memory, the List Sorting Working Memory (Tulsky et al., 2014); a measure of episodic memory, Picture Sequence Memory (Dikmen et al., 2014); a measure of processing speed, Pattern Comparison Processing Speed (Carlozzi et al., 2014); and measures of inhibitory control and cognitive flexibility, Flanker Inhibitory Control and Attention and Dimensional Change Card Sort, respectively (Zelazo et al., 2014). Detailed descriptions of each test are reported elsewhere (Holdnack et al., 2017).
Procedures
The normative data for the English-language NIHTB-CB was collected as part of a national norming study involving recruitment of a sample of children, adolescents, and adults representative of the U.S. population per 2010 U.S. Census data (Beaumont et al., 2013). The adult sample consisted of community-dwelling adults who were capable of following test instructions in English and provided informed consent prior to participation. The fully deidentified normative data are publicly available for download for secondary analysis (Gershon, 2016). The secondary analyses of these deidentified data were deemed not human subjects research and were approved by the Partners Human Research Committee (Protocol #: 2020P000504).
Statistical Analyses
For the NIHTB-CB, age-adjusted scores are standardized as Standard Scores (SS; M = 100, SD = 15) and the demographic-adjusted scores are standardized as T scores (M = 50, SD = 10) with adjustments for age, gender, education, and race/ethnicity (Casaletto et al., 2015). The following cutoffs were used to define performances at or below specific percentiles: ≤ 25th percentile (SS ≤ 90 or T ≤ 43), ≤ 16th percentile (SS ≤ 85 or T ≤ 40), ≤ 9th percentile (SS ≤ 80 or T ≤ 36), ≤ 5th percentile (SS ≤ 76 or T ≤ 34), and ≤ 2nd percentile (SS ≤ 70 or T ≤ 30). Of note, no whole number T score corresponds to the exact 9th percentile, and a T ≤ 36 was selected because it corresponds to the lowest whole number T score typically interpreted as borderline or unusually low in clinical practice. The following cutoffs were used for defining scores at or above certain cutoffs: ≥50th percentile (SS≥100 or T≥50), ≥63rd percentile (SS≥105 or T≥53), ≥75th percentile (SS≥110 or T≥57), ≥84th percentile (SS≥115 or T≥60), ≥91st percentile (SS≥120 or T≥64), ≥95th percentile (SS≥124 or T≥66), and ≥98th percentile (SS≥130 or T≥70). Of note, a T score of 53 was selected as the closest whole number T score to the 63rd percentile and a T score of 64 was selected as the closest whole number T score to the 91st percentile, but they align more closely with the 62nd percentile and 92nd percentile, respectively. The percentile cutoffs for defining low and high scores are consistent with previous research on multivariate base rates using the NIHTB-CB (Holdnack et al., 2017; Karr and Iverson, 2020). Although all the above cutoffs, collectively, are described as high score base rates, performances falling ≥50th and ≥63rd percentiles are not typically interpreted as high in clinical practice, but they are useful for determining whether an absence of scores above these cutoffs is unusual for high functioning individuals, and potentially indicative of cognitive impairment.
Results
Base Rates of Low Scores
The base rates of low scores on the NIHTB-CB, for the total sample and stratified by years of education and Crystallized Composite, are presented in Table 1. Base rates are presented for several different cutoff scores, including ≤ 25th, ≤ 16th, ≤ 9th, ≤ 5th, and ≤ 2nd percentiles for both age-adjusted normative scores and demographic-adjusted normative scores. Using age-adjusted norms, people with higher levels of education and above average or superior scores on the Crystallized Composite, obtained fewer low scores. For example, using the 16th percentile as the cutoff for a low score, the base rates of having one or more low scores, by subgroup, were as follows: education = 12 years, 49.2%; education = 16 or more years, 36.0%; crystallized composite = 110–119, 22.7%; and crystallized composite = 120 or greater, 19.2%.
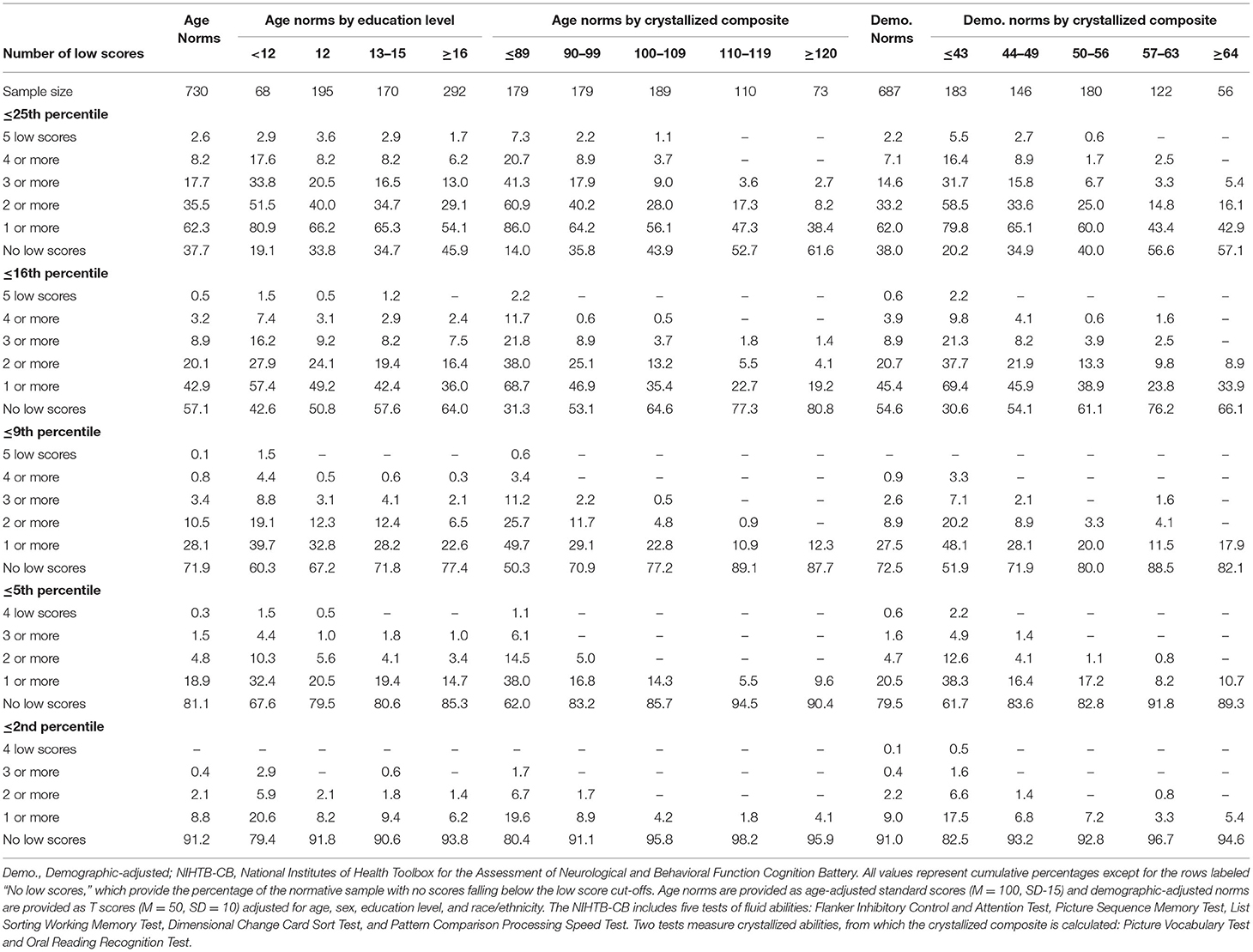
Table 1. Base rates of low scores on NIHTB-CB fluid measures in the normative sample without pre-existing neurobehavioral, psychiatric, substance use, or neurological conditions, with stratifications by education and crystallized ability level.
Base Rates of High Scores
The base rates of high scores on the NIHTB-CB, for the total sample and stratified by education and level of intellectual functioning, are presented in Table 2. Base rates are presented for several different cutoff scores, including ≥50th, ≥63rd, ≥75th, ≥84th, ≥91st, ≥95th, and ≥98th percentiles for both age-adjusted normative scores and demographic-adjusted normative scores. Using age-adjusted norms, people with higher levels of education and above average or superior scores on the Crystallized Composite obtained more high scores. For example, using the 84th percentile as the cutoff for a high score, the base rates of having two or more high scores, by subgroup, were as follows: education = 12 years, 20.0%; education = 16 or more years, 27.1%; crystallized composite = 110-119, 33.6%; and crystallized composite = 120 or greater, 45.2%. Using the 95th percentile as the cutoff for a high score, the base rates of having one or more high scores, by subgroup, were as follows: education = 12 years, 19.5%; education = 16 or more years, 29.1%; crystallized composite = 110-119, 34.5%; and crystallized composite = 120 or greater, 42.5%.
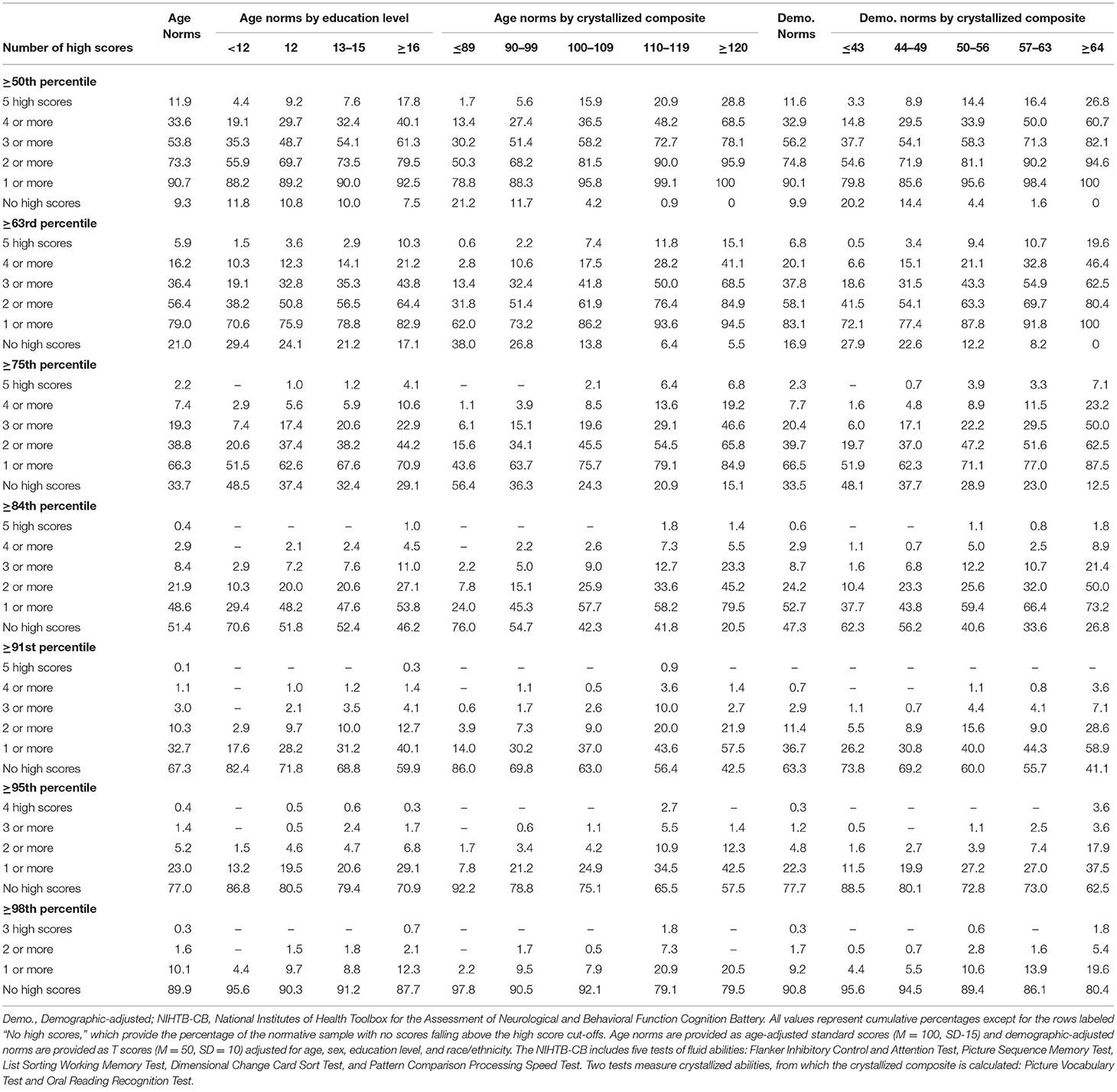
Table 2. Base rates of high scores on NIHTB-CB fluid measures in the normative sample without pre-existing neurobehavioral, psychiatric, substance use, or neurological conditions, with stratifications by education and crystallized ability level.
As seen in Table 2, it is uncommon to obtain no scores ≥50th percentile or ≥63rd percentile, which occurred in only 9.3 and 21.0% of the total sample, respectively, using age-adjusted norms. The absence of scores at or above these cutoffs was very uncommon in individuals of high average crystallized ability, occurring in only 0.9 and 6.4%, respectively, using age-adjusted norms. Using demographic-adjusted norms, 91.8% of those with high average crystallized ability and 100% of individuals with superior crystallized ability obtained at least 1 score ≥63rd percentile.
Algorithms for Identifying Cognitive Impairment
The algorithms in Table 3 rely on age-adjusted normative scores. We have computed the base rate of each component of the algorithm separately, and then the base rate for the entire algorithm. For Algorithm A, for example, the base rate for people with high average crystalized ability obtaining any one of the following, 5 scores <63rd percentile, or 4+ scores <50th percentile, or 3+ scores ≤ 25th percentile, or 2+ scores ≤ 16th percentile, is 15.5%. As such, having a performance patten on the NIHTB-CB consistent with that algorithm would correspond to 1 SD below the mean for people with high average intellectual abilities. For Algorithm D, the base rate for people with university degrees obtaining 4+ scores ≤ 25th percentile or 2+ scores ≤ 5th percentile is 7.5%. As such, a performance consistent with that algorithm is ~1.5 SDs below the mean for people with university degrees.
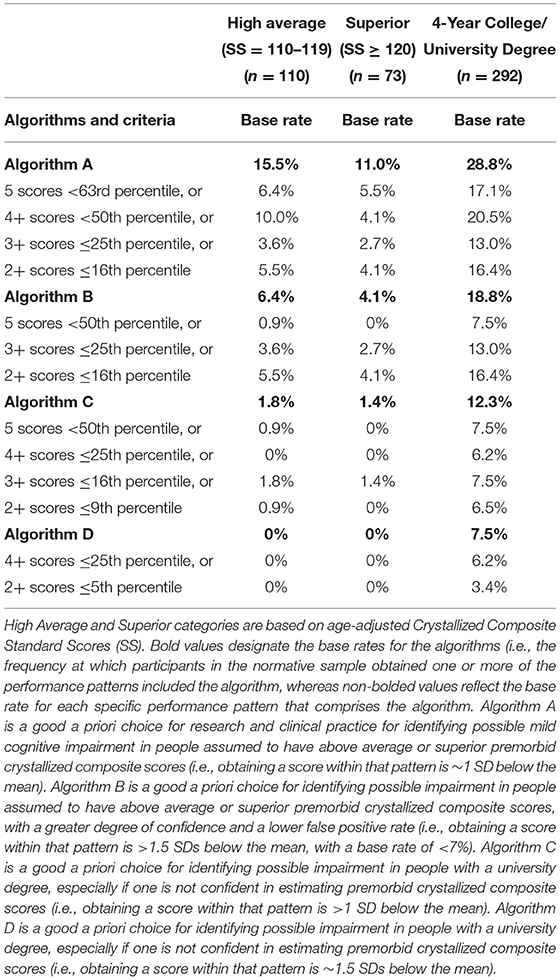
Table 3. Primary algorithms for identifying cognitive impairment in high functioning adults on the NIH toolbox cognition battery using age-adjusted normative scores.
Algorithms for identifying cognitive impairment using demographic-adjusted normative scores are presented in Table 4. For Algorithm D, the base rate for people with high average or superior crystalized ability obtaining any one of the following, 5 scores <50th percentile, or 3+ scores ≤ 25th percentile, or 2+ scores ≤ 9th percentile, is 6.6 and 5.4%, respectively. As such, a performance pattern on the NIHTB-CB consistent with that algorithm is >1.5 SDs below the mean for people with university degrees.
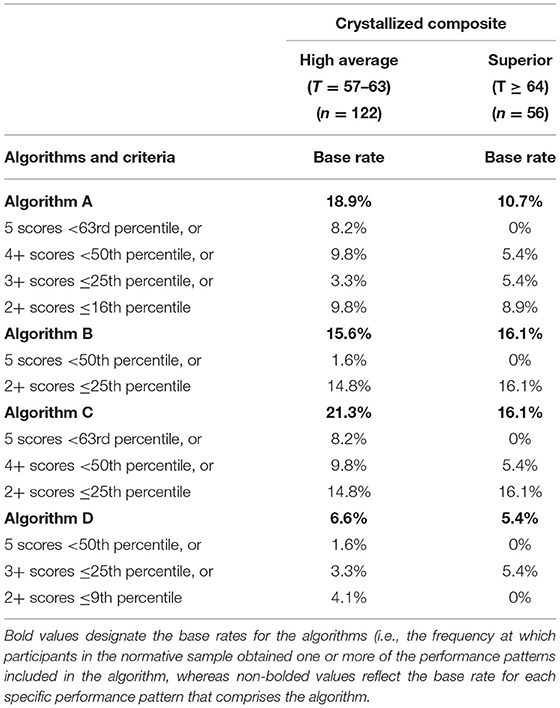
Table 4. Primary algorithms for identifying cognitive impairment in high functioning adults on the NIH toolbox cognition battery using demographic-adjusted normative scores.
Discussion
A longstanding approach to identifying cognitive deficits, or mild cognitive impairment, is to select a cutoff for defining a low score and applying that cutoff to all people—such as scoring 1 SD (Taylor and Heaton, 2001; Busse et al., 2006) or 1.5 SDs (Lopez et al., 2006; Tabert et al., 2006) below the mean. This approach underlies many studies relating to mild cognitive impairment in older adults (Jak et al., 2009; Ganguli et al., 2011; Petersen et al., 2014; Weissberger et al., 2017) and for identifying mild neurocognitive disorder according to the DSM-5 (American Psychiatric Association, 2013). This approach is also common in clinical practice. A one-size fits all approach to identifying cognitive deficits, however, is not appropriate because there are major individual differences in cognitive abilities that must be considered when defining a deficit or impairment, especially a person's level of intellectual functioning and educational history. People with below average intellectual functioning are expected to obtain a large number of low neuropsychological test scores and people with above average or superior intellectual functioning obtain far fewer low test scores (Binder et al., 2009; Brooks et al., 2009b, 2011). This was also true in the present study, as seen in base rates of age-adjusted low scores presented in Table 1, Figure 1. Each year of education corresponds to a one to five point increase in IQ score (Ritchie and Tucker-Drob, 2018), so that people with higher levels of education are expected to obtain fewer low neuropsychological test scores (Brooks et al., 2013). This, too, was illustrated in the present study, as seen in Table 1, whereby those with more years of education also obtained fewer age-adjusted low scores. These individual differences in education and intellectual functioning can only be partially mitigated by using demographic-adjusted normative data, which adjust for education. The differences in base rates of low scores across levels of intelligence were smaller when using demographic-adjusted normative scores compared to age-adjusted normative scores, but still present.
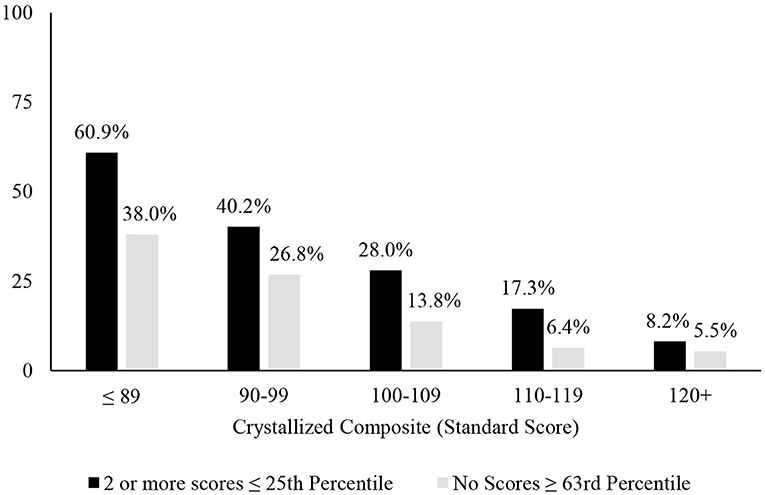
Figure 1. Association between level of intellectual ability and patterns of scores. Percentages of people showing the pattern of scores stratified by their level of intellectual functioning. It is common for people with below average intellectual abilities to have 2 or more fluid scores ≤ 25th and uncommon for people with superior intellectual abilities to have 2 or more below average fluid test scores. Similarly, it is common for people with below average intellectual abilities to have no fluid test scores ≥63rd percentile and it is very uncommon for people with above average or superior intellectual abilities to have no fluid test scores ≥63rd percentile.
Tables 1, 2 allow clinicians and researchers to determine how common it is to have low scores and high scores on the NIHTB-CB. The base rates of low scores presented in this article differ from those previously published (Holdnack et al., 2017) because we excluded people with health conditions that might have an adverse effect on cognition and Holdnack and colleagues collapsed those with high average and superior crystallized composite scores into a single group. The high score base rate tables presented in this article differ modestly from those previously published (Karr and Iverson, 2020), because participants with neurodevelopmental, psychiatric, substance use, and neurological disorders were excluded in the current analyses, but were included in the prior study.
The algorithms provided in Tables 3, 4 are ready to be applied in clinical studies. Researchers and clinicians should be aware that when using base rate analyses, in research and clinical practice, if multiple algorithms are applied sequentially or simultaneously the base rates increase. For Algorithm A in Table 3, for example, when applying each component of the algorithm the base rates range from 2.7 to 5.5%, but when applying all components, the base rate is 11.0% in people with superior intellectual abilities.
Limitations
There are limitations associated with using the NIHTB-CB for identifying cognitive weaknesses, deficits, or impairments. First, the battery is relatively brief. Second, it includes brief measures for some important constructs, such as memory, that lack process-oriented test scores often used to identify different dementias. Although the NIHTB-CB does include an auditory verbal learning test as a supplementary measure, the normative data for this measure is very limited, and does not have the demographic adjustments automatically applied to the core seven tests (Casaletto et al., 2015). Finally, those in the normative sample did not undergo effort testing during the standardization of the battery, meaning that if there were participants with low effort on testing, they could not be identified.
It is important to appreciate, in clinical practice and research, that we used the Crystallized Composite score as an estimate of longstanding intellectual abilities. If research participants have a neurological disorder, or they have sustained a moderate-severe traumatic brain injury, their Crystallized Composite score might underestimate their longstanding, premorbid, intellectual functioning. This is only problematic for our algorithms if the under-estimate results in a change in the person's estimated premorbid intellectual category—such as moving from high average to average. The differences in base rates between those with estimated superior abilities vs. high average abilities are modest. The real potential problem is for examinees who obtain an age-adjusted Crystallized Composite score between 106 and 109, for example, and the researcher or clinician has good reason to suspect that their longstanding premorbid composite score was likely to be 110 or higher. Research is needed to determine if a small upward adjustment in obtained Crystallized Composite scores, for people who score a few points lower than the high average classification range, improves the diagnostic accuracy of these algorithms in people with neurological conditions.
Conclusions
In conclusion, the identification of mild cognitive deficits in high-functioning people is challenging in clinical practice and research. High-functioning people are less likely to obtain low neuropsychological test scores than people of average intelligence (Brooks et al., 2011, 2013; Holdnack et al., 2017; Karr et al., 2017, 2018). It is possible that some high functioning people with psychiatric or neurological disorders might not obtain any low scores within a cognitive domain, and if so, it might be the absence of above average scores, not the presence of low scores, that reveals their cognitive deficits. Future research is needed to determine whether a cognitive impairment classification based on these algorithms corresponds to risk factors for, or biomarkers of, clinical conditions known to affect cognitive functioning.
Data Availability Statement
Publicly available datasets were analyzed in this study. This data can be found at: https://doi.org/10.7910/DVN/FF4DI7.
Ethics Statement
The secondary analyses of these deidentified data were deemed not human subjects research and were approved by the Partners Human Research Committee (Protocol #: 2020P000504). Written informed consent for participation was not required for this study in accordance with the national legislation and the institutional requirements.
Author Contributions
GI conceptualized the study, assisted with the literature review, helped conceptualize the analyses, drafted sections of the manuscript, edited the manuscript, and approved the final manuscript. JK assisted with the literature review, helped conceptualize the analyses, conducted the analyses, drafted sections of the manuscript, edited the manuscript, and approved the final manuscript. Both authors contributed to the article and approved the submitted version.
Funding
GI acknowledges philanthropic support from the Third Option Foundation and the Spaulding Research Institute. The above mentioned entities were not involved in the study design, interpretation of data, the writing of this article, or the decision to submit it for publication.
Conflict of Interest
GI has received research support from test publishing companies in the past, including PAR, Inc., ImPACT Applications, Inc., and CNS Vital Signs. He receives royalties for one neuropsychological test (Wisconsin Card Sorting Test-64 Card Version). He serves as a scientific advisor for NanoDx®, Sway Operations, LLC, and Highmark, Inc. He has a clinical and consulting practice in forensic neuropsychology, including expert testimony, involving individuals who have sustained mild TBIs. He has received research funding from several test publishing companies, including ImPACT Applications, Inc., CNS Vital Signs, and Psychological Assessment Resources (PAR, Inc.). He has received research funding as a principal investigator from the National Football League, and subcontract grant funding as a collaborator from the Harvard Integrated Program to Protect and Improve the Health of National Football League Players Association Members. He acknowledges unrestricted philanthropic support from ImPACT Applications, Inc., the Mooney-Reed Charitable Foundation, and the National Rugby League.
The remaining author declares that the research was conducted in the absence of any commercial or financial relationships that could be construed as a potential conflict of interest.
Publisher's Note
All claims expressed in this article are solely those of the authors and do not necessarily represent those of their affiliated organizations, or those of the publisher, the editors and the reviewers. Any product that may be evaluated in this article, or claim that may be made by its manufacturer, is not guaranteed or endorsed by the publisher.
References
Albert, M. S., DeKosky, S. T., Dickson, D., Dubois, B., Feldman, H. H., Fox, N. C., et al. (2011). The diagnosis of mild cognitive impairment due to Alzheimer's disease: Recommendations from the National Institute on Aging-Alzheimer's Association workgroups on diagnostic guidelines for Alzheimer's disease. Alzheimer's Dement. 7, 270–279. doi: 10.1016/j.jalz.2011.03.008
American Psychiatric Association (2013). Diagnostic and Statistical Manual of Mental Disorders, 5th Edn. Washington, DC: American Psychiatric Association.
Axelrod, B. N., and Wall, J. R. (2007). Expectancy of impaired neuropsychological test scores in a non-clinical sample. Int. J. Neurosci. 117, 1591–1602. doi: 10.1080/00207450600941189
Beaumont, J. L., Havlik, R., Cook, K. F., Hays, R. D., Wallner-Allen, K., Korper, S. P., et al. (2013). Norming plans for the NIH Toolbox. Neurology 80, S87–S92. doi: 10.1212/WNL.0b013e3182872e70
Binder, L. M., Iverson, G. L., and Brooks, B. L. (2009). To err is human: “Abnormal” neuropsychological scores and variability are common in healthy adults. Arch. Clin. Neuropsychol. 24, 31–46. doi: 10.1093/arclin/acn001
Brooks, B. L., Holdnack, J. A., and Iverson, G. L. (2011). Advanced clinical interpretation of the WAIS-IV and WMS-IV: prevalence of low scores varies by level of intelligence and years of education. Assessment 18, 156–167. doi: 10.1177/1073191110385316
Brooks, B. L., Iverson, G. L., Feldman, H. H., and Holdnack, J. A. (2009b). Minimizing misdiagnosis: Psychometric criteria for possible or probable memory impairment. Dement. Geriatr. Cogn. Disord. 27, 439–450. doi: 10.1159/000215390
Brooks, B. L., Iverson, G. L., and Holdnack, J. A. (2013). “Understanding and using multivariate base rates with the WAIS-IV/WMS-IV,” in WAIS-IV, WMS-IV, and ACS: Advanced Clinical Interpretation, eds J. A. Holdnack, L. W. Drozdick, L. G. Weiss, and G. L. Iverson (Amsterdam: Elsevier Science), 75–102.
Brooks, B. L., Iverson, G. L., Holdnack, J. A., and Feldman, H. H. (2008). Potential for misclassification of mild cognitive impairment: a study of memory scores on the Wechsler Memory Scale-III in healthy older adults. J. Int. Neuropsychol. Soc. 14, 463–478. doi: 10.1017/S1355617708080521
Brooks, B. L., Iverson, G. L., Sherman, E. M. S., and Holdnack, J. A. (2009c). Healthy children and adolescents obtain some low scores across a battery of memory tests. J. Int. Neuropsychol. Soc. 15, 613–617. doi: 10.1017/S1355617709090651
Brooks, B. L., Iverson, G. L., and White, T. (2009a). Advanced interpretation of the neuropsychological assessment battery with older adults: Base rate analyses, discrepancy scores, and interpreting change. Arch. Clin. Neuropsychol. 24, 647–657. doi: 10.1093/arclin/acp061
Brooks, B. L., Sherman, E. M. S., and Iverson, G. L. (2010). Healthy children get low scores too: prevalence of low scores on the NEPSY-II in preschoolers, children, and adolescents. Arch. Clin. Neuropsychol. 25, 182–190. doi: 10.1093/arclin/acq005
Busse, A., Hensel, A., Gühne, U., Angermeyer, M. C., and Riedel-Heller, S. G. (2006). Mild cognitive impairment: long-term course of four clinical subtypes. Neurology 67, 2176–2185. doi: 10.1212/01.wnl.0000249117.23318.e1
Carlozzi, N. E., Tulsky, D. S., Chiaravalloti, N. D., Beaumont, J. L., Weintraub, S., Conway, K., et al. (2014). NIH toolbox cognitive battery (NIHTB-CB): the NIHTB pattern comparison processing speed test. J. Int. Neuropsychol. Soc. 20, 630–641. doi: 10.1017/S1355617714000319
Casaletto, K. B., Umlauf, A., Beaumont, J., Gershon, R., Slotkin, J., Akshoomoff, N., et al. (2015). Demographically corrected normative standards for the English version of the NIH Toolbox Cognition Battery. J. Int. Neuropsychol. Soc. 21, 378–391. doi: 10.1017/S1355617715000351
Cook, N. E., Karr, J. E., Brooks, B. L., Garcia-Barrera, M. A., Holdnack, J. A., and Iverson, G. L. (2019). Multivariate base rates for the assessment of executive functioning among children and adolescents. Child Neuropsychol. 25, 836–858. doi: 10.1080/09297049.2018.1543389
Dikmen, S. S., Bauer, P. J., Weintraub, S., Mungas, D., Slotkin, J., Beaumont, J. L., et al. (2014). Measuring episodic memory across the lifespan: NIH toolbox picture sequence memory test. J. Int. Neuropsychol. Soc. 20, 611–619. doi: 10.1017/S1355617714000460
Dubois, B., Feldman, H. H., Jacova, C., DeKosky, S. T., Barberger-Gateau, P., Cummings, J., et al. (2007). Research criteria for the diagnosis of Alzheimer's disease: revising the NINCDS-ADRDA criteria. Lancet Neurol. 6, 734–746. doi: 10.1016/S1474-4422(07)70178-3
Ganguli, M., Snitz, B. E., Saxton, J. A., Chang, C. C. H., Lee, C. W., Vander Bilt, J., et al. (2011). Outcomes of mild cognitive impairment by definition: a population study. Arch. Neurol. 68, 761–767. doi: 10.1001/archneurol.2011.101
Gershon, R. C. (2016). NIH toolbox norming study. Harvard Dataverse, V4, UNF:6:bOqMnZEEG/rBz6SQyN4t2g = = [fileUNF].
Gershon, R. C., Cella, D., Fox, N. A., Havlik, R. J., Hendrie, H. C., and Wagster, M. V. (2010). Assessment of neurological and behavioural function: the NIH Toolbox. Lancet Neurol. 9, 138–139. doi: 10.1016/S1474-4422(09)70335-7
Gershon, R. C., Cook, K. F., Mungas, D., Manly, J. J., Slotkin, J., Beaumont, J. L., et al. (2014). Language measures of the NIH toolbox cognition battery. J. Int. Neuropsychol. Soc. 20, 642–651. doi: 10.1017/S1355617714000411
Gershon, R. C., Wagster, M. V., Hendrie, H. C., Fox, N. A., Cook, K. F., and Nowinski, C. J. (2013). NIH toolbox for assessment of neurological and behavioral function. Neurology 80(11 Suppl. 3), S2–S6. doi: 10.1212/WNL.0b013e3182872e5f
Heaton, R. K., Grant, I., and Matthews, C. G. (1991). Comprehensive Norms for an Extended Halstead-Reitan Battery: Demographic Corrections, Research Findings, and Clinical Applications. Lutz, FL: Psychological Assessment Resources, Inc.
Heaton, R. K., Miller, S. W., Taylor, M. J., and Grant, I. (2004). Revised Comprehensive Norms for an Expanded Halstead-Reitan Battery: Demographically Adjusted Neuropsychological Norms for African American and Caucasian Adults Professional Manual. Lutz, FL: Psychological Assessment Resources, Inc.
Holdnack, J. A., Tulsky, D. S., Brooks, B. L., Slotkin, J., Gershon, R., Heinemann, A. W., et al. (2017). Interpreting patterns of low scores on the NIH toolbox cognition battery. Arch. Clin. Neuropsychol. 32, 574–584. doi: 10.1093/arclin/acx032
Horton, A. M. N. (1999). Above-average intelligence and neuropsychological test score performance. Int. J. Neurosci. 99, 221–231. doi: 10.3109/00207459908994326
Jak, A. J., Bondi, M. W., Delano-Wood, L., Wierenga, C., Corey-Bloom, J., Salmon, D. P., et al. (2009). Quantification of five neuropsychological approaches to defining mild cognitive impairment. Am. J. Geriatr. Psychiatry 17, 368–375. doi: 10.1097/JGP.0b013e31819431d5
Karr, J. E., Garcia-Barrera, M. A., Holdnack, J. A., and Iverson, G. L. (2017). Using multivariate base rates to interpret low scores on an abbreviated battery of the Delis-Kaplan Executive Function System. Arch. Clin. Neuropsychol. 32, 297–305. doi: 10.1093/arclin/acw105
Karr, J. E., Garcia-Barrera, M. A., Holdnack, J. A., and Iverson, G. L. (2018). Advanced clinical interpretation of the Delis-Kaplan Executive Function System: multivariate base rates of low scores. Clin. Neuropsychol. 32, 42–53. doi: 10.1080/13854046.2017.1334828
Karr, J. E., Garcia-Barrera, M. A., Holdnack, J. A., and Iverson, G. L. (2020). The other side of the bell curve: multivariate base rates of high scores on the Delis-Kaplan Executive Function System. J. Int. Neuropsychol. Soc. 26, 382–393. doi: 10.1017/S1355617719001218
Karr, J. E., and Iverson, G. L. (2020). Interpreting high scores on the NIH Toolbox Cognition Battery: potential utility for detecting cognitive decline in high-functioning individuals. Neuropsychology 34, 764–773. doi: 10.1037/neu0000691
Lopez, O. L., Becker, J. T., Jagust, W. J., Fitzpatrick, A., Carlson, M. C., DeKosky, S. T., et al. (2006). Neuropsychological characteristics of mild cognitive impairment subgroups. J. Neurol. Neurosurg. Psychiatry 77, 159–165. doi: 10.1136/jnnp.2004.045567
Palmer, B. W., Boone, K. B., Lesser, I. M., and Wohl, M. A. (1998). Base rates of “impaired” neuropsychological test performance among healthy older adults. Arch. Clin. Neuropsychol. 13, 503–511.
Petersen, R., Smith, G., Waring, S., Ivnik, R., Tangalos, E., and Kokmen, E. (1999). Mild cognitive impairment: clinical characterization and outcome. Arch. Neurol. 56, 303–308. doi: 10.1001/archneur.56.3.303
Petersen, R. C., Caracciolo, B., Brayne, C., Gauthier, S., Jelic, V., and Fratiglioni, L. (2014). Mild cognitive impairment: a concept in evolution. J. Intern. Med. 275, 214–228. doi: 10.1111/joim.12190
Reitan, R. M., and Wolfson, D. (1993). The Halstead-Reitan Neuropsychological Test Battery: Theory and Clinical Interpretation, 2nd Edn. Tucson, AZ: Neuropsychology Press.
Ritchie, S. J., and Tucker-Drob, E. M. (2018). How much does education improve intelligence? a meta-analysis. Psychol. Sci. 29, 1358–1369. doi: 10.1177/0956797618774253
Schretlen, D. J., Testas, S. M., Winicki, J. M., Pearlson, G. D., and Gordon, B. (2008). Frequency and bases of abnormal performance by healthy adults on neuropsychological testing. J. Int. Neuropsychol. Soc. 14, 436–445. doi: 10.1017/S1355617708080387
Steinberg, B. A., Bieliauskas, L. A., Smith, G. E., and Ivnik, R. J. (2005a). Mayo's older Americans normative studies: age- and IQ-adjusted norms for the Trail-Making Test, the Stroop test, and MAE Controlled Oral Word Association Test. Clin. Neuropsychol. 19, 329–377. doi: 10.1080/13854040590945210
Steinberg, B. A., Bieliauskas, L. A., Smith, G. E., Ivnik, R. J., and Malec, J. F. (2005b). Mayo's older Americans normative studies: age- and IQ-adjusted norms for the Auditory Verbal Learning Test and the Visual Spatial Learning Test. Clin. Neuropsychol. 19, 464–523. doi: 10.1080/13854040590945193
Tabert, M. H., Manly, J. J., Liu, X., Pelton, G. H., Rosenblum, S., Jacobs, M., et al. (2006). Neuropsychological prediction of conversion to alzheimer disease in patients with mild cognitive impairment. Arch. Gen. Psychiatry 63, 916–924. doi: 10.1001/archpsyc.63.8.916
Taylor, M. J., and Heaton, R. K. (2001). Sensitivity and specificity of WAIS-III/WMS-III domographically corrected factor scores in neuropsychological assessment. J. Int. Neuropsychol. Soc. 7, 867–874. doi: 10.1017/S1355617701777107
Tremont, G., Hoffman, R. G., Scott, J. G., and Adams, R. L. (1998). Effect of intellectual level on neuropsychological test performance: a response to Dodrill (1997). Clin. Neuropsychol. 12, 560–567. doi: 10.1076/clin.12.4.560.7238
Tulsky, D. S., Carlozzi, N., Chiaravalloti, N. D., Beaumont, J. L., Kisala, P. A., Mungas, D., et al. (2014). NIH Toolbox Cognition Battery (NIHTB-CB): list sorting test to measure working memory. J. Int. Neuropsychol. Soc. 20, 599–610. doi: 10.1017/S135561771400040X
Warner, M. H., Ernst, J., Townes, B. D., Peel, J., and Preston, M. (1987). Relationships between IQ and neuropsychological measures in neuropsychiatric populations: within-laboratory and cross-cultural replications using WAIS and WAIS-R. J. Clin. Exp. Neuropsychol. 9, 545–562. doi: 10.1080/01688638708410768
Weintraub, S., Dikmen, S. S., Heaton, R. K., Tulsky, D. S., Zelazo, P. D., Bauer, P. J., et al. (2013). Cognition assessment using the NIH Toolbox. Neurology 80(11 Suppl. 3), S54–S64. doi: 10.1212/WNL.0b013e3182872ded
Weissberger, G. H., Strong, J. V., Stefanidis, K. B., Summers, M. J., Bondi, M. W., and Stricker, N. H. (2017). Diagnostic accuracy of memory measures in Alzheimer's dementia and mild cognitive impairment: a systematic review and meta-analysis. Neuropsychol. Rev. 27, 354–388. doi: 10.1007/s11065-017-9360-6
Keywords: cognition, neuropsychological tests, psychometrics, cognitive dysfunction, NIH Toolbox
Citation: Iverson GL and Karr JE (2021) Improving the Methodology for Identifying Mild Cognitive Impairment in Intellectually High-Functioning Adults Using the NIH Toolbox Cognition Battery. Front. Psychol. 12:724888. doi: 10.3389/fpsyg.2021.724888
Received: 14 June 2021; Accepted: 12 August 2021;
Published: 08 September 2021.
Edited by:
Matteo De Marco, Brunel University London, United KingdomReviewed by:
Andrea Tales, Swansea University, United KingdomMaria Semkovska, University of Southern Denmark, Denmark
Copyright © 2021 Iverson and Karr. This is an open-access article distributed under the terms of the Creative Commons Attribution License (CC BY). The use, distribution or reproduction in other forums is permitted, provided the original author(s) and the copyright owner(s) are credited and that the original publication in this journal is cited, in accordance with accepted academic practice. No use, distribution or reproduction is permitted which does not comply with these terms.
*Correspondence: Grant L. Iverson, giverson@mgh.harvard.edu