- 1Department of Emergency Medicine, Jining No.1 People’s Hospital, Jining, China
- 2Wuxi Mental Health Center, Wuxi, China
- 3Department of Oncology, Affiliated Hospital of Xuzhou Medical University, Xuzhou, China
- 4Department of Gastroenterology, The Second Affiliated Hospital of Xuzhou Medical University, Xuzhou, China
- 5Department of Gastroenterology, Xuzhou First People’s Hospital, Xuzhou, China
- 6Jiangsu Normal University, Xuzhou, China
- 7Department of Gastroenterology, Jining First People’s Hospital, Jining, China
Objective: Gastric cancer is a prevalent gastrointestinal malignancy worldwide. In this study, a prognostic model was developed for gastric cancer patients who underwent radical gastrectomy using machine learning, employing advanced computational techniques to investigate postoperative mortality risk factors in such patients.
Methods: Data of 295 patients with gastric cancer who underwent radical gastrectomy at the Department of General Surgery of Affiliated Hospital of Xuzhou Medical University (Xuzhou, China) between March 2016 and November 2019 were retrospectively analyzed as the training group. Additionally, 109 patients who underwent radical gastrectomy at the Department of General Surgery Affiliated to Jining First People’s Hospital (Jining, China) were included for external validation. Four machine learning models, including logistic regression (LR), decision tree (DT), random forest (RF), and gradient boosting machine (GBM), were utilized. Model performance was assessed by comparing the area under the curve (AUC) for each model. An LR-based nomogram model was constructed to assess patients’ clinical prognosis.
Results: Lasso regression identified eight associated factors: age, sex, maximum tumor diameter, nerve or vascular invasion, TNM stage, gastrectomy type, lymphocyte count, and carcinoembryonic antigen (CEA) level. The performance of these models was evaluated using the AUC. In the training group, the AUC values were 0.795, 0.759, 0.873, and 0.853 for LR, DT, RF, and GBM, respectively. In the validation group, the AUC values were 0.734, 0.708, 0.746, and 0.707 for LR, DT, RF, and GBM, respectively. The nomogram model, constructed based on LR, demonstrated excellent clinical prognostic evaluation capabilities.
Conclusion: Machine learning algorithms are robust performance assessment tools for evaluating the prognosis of gastric cancer patients who have undergone radical gastrectomy. The LR-based nomogram model can aid clinicians in making more reliable clinical decisions.
Introduction
Gastric cancer (GC) is believed to be the fifth most common cancer and the third most common cause of death worldwide.Notably, China and Japan are at the forefront, collectively accounting for 75% of Asian cases (1, 2). Despite being one of the most common treatment modalities for gastric cancer, surgical intervention alone has failed to elevate the overall 5-year survival rate beyond 50%. Thus, the quest for precise clinical assessments holds paramount clinical importance for the diagnosis and management of affected patients (3). One widely embraced approach in clinical research involves amassing clinical data to construct prognostic models. Within this domain, gastric cancer model studies have proliferated, offering the promise of better-informed clinical decision-making (4, 5). In addition to clinicopathological data, these models incorporate hematologic inflammatory markers and the widely utilized carcinoembryonic antigen (CEA). The association between inflammation and its impact on the occurrence, progression, metastasis, and prognosis of cancer patients, as revealed by blood-based metrics, has become a burgeoning area of research interest (6, 7). The principle underlying the utilization of CEA as a serum tumor marker is well-established in clinical practice. This marker finds extensive utility in the early screening of various tumors. Furthermore, its early elevation is recognized as an independent risk factor associated with the poorer prognosis of gastric cancer (8).
Machine learning stands as a precision algorithm within the context of artificial intelligence, uniquely poised to decipher vast and intricate medical datasets. Its capacity to construct clinical prediction models makes it an invaluable tool in the realm of healthcare, offering crucial assistance in diagnosis and prognostication (9). The development of clinical predictive models typically involves the processing and optimization of large datasets within a training set. Subsequently, these models undergo rigorous testing using external validation set data, a pivotal step in establishing their external validity and, by extension, their applicability to diverse patient populations (10, 11). Cancer, marked by its complexity and heterogeneity, emerges as a particularly promising frontier for machine learning applications in medical research. The significance of clinical data available empowers early cancer detection, facilitates ongoing monitoring of disease progression, and supports the optimization of treatment strategies (9, 12).
Patients and methods
Patients’ enrollment
This retrospective analysis involved a total of 295 gastric cancer patients who underwent radical gastrectomy at the Department of General Surgery, Affiliated Hospital of Xuzhou Medical University (Xuzhou, China), between March 2016 and November 2019. These patients constituted the training group. Additionally, 109 gastric cancer patients who underwent radical gastrectomy at the Department of General Surgery of Jining First People’s Hospital (Jining, China) were included as the verification group. The inclusion criteria were as follows: (1): patients newly diagnosed with gastric cancer, for whom comprehensive medical records were available; (2) cases where primary radical resection of gastric cancer was conducted at the respective hospitals, with subsequent confirmation of gastric adenocarcinoma; (3) absence of any prior anti-tumor therapies, including radiotherapy or chemotherapy, before surgical intervention. The exclusion criteria were as follows: (1) patients with concurrent malignancies; (2) patients presenting preoperative complications of other infectious diseases, blood system disorders, autoimmune conditions, or any other medical conditions that could potentially influence inflammatory markers; (3) cases who had recently received or were currently undergoing anti-inflammatory or immunosuppressive treatments; (4) patients subjected to preoperative blood transfusion therapy; (5) patients with severe liver or kidney dysfunction; (6) cases featuring incomplete clinical data or visitor information. Further details are illustrated in Figure 1.
Outcome measures
The primary outcome event for this study was the survival status of patients at the three-year post-radical gastrectomy. Follow-up procedures involved telephonic or outpatient monitoring. The survival rate was determined from the date of admission to either the date of decease or the specified deadline for follow-up.
Research purpose
This study concentrated on evaluating the three-year survival outcomes of patients who underwent radical gastrectomy. A total of 404 gastric cancer patients from two medical centers were included in the study. A machine learning algorithm was employed to develop a clinical prediction model aimed at identifying the prognostic risk factors for postoperative patients. The creation of a visual nomogram model, based on these risk factors, can aid healthcare professionals in conducting risk assessments.
Risk factors
Concerning the study subjects, clinical data were collected, including patient’s name, age, gender, and clinicopathological information. This included data on blood parameters, tumor location, maximum tumor size, TNM stage, lymph node involvement, nerve vessel invasion, method of gastrectomy, tumor differentiation grade, along with specific blood markers including neutrophil count, monocyte count, lymphocyte count, and CEA level. Peripheral venous blood samples were obtained from fasting cases on the next morning. The collected indices were then incorporated into the Lasso regression model. The Lasso model employs a technique that can shrink the coefficients of unimportant variables to 0, promoting feature selection. Following the establishment of inclusion and exclusion criteria, the relevant data were fed into the Lasso model, enabling the complete elimination of the weight associated with the least important variables. This process allows for data screening and complexity adjustment while fitting the generalized linear model. Consequently, the Lasso model ensures the accuracy of variables in the subsequent development of the machine learning model.
Statistical analysis
Continuous variables were presented as mean ± standard deviation, and categorical variables were expressed as ratio. To create the machine learning and nomogram models, the process was initiated by applying a Lasso regression model to identify the key risk factors linked to the 3-year survival status of patients, as depicted in Figure 1. Subsequently, these relevant risk factors were integrated into machine learning algorithms, leading to the development of logistic regression (LR), decision tree (DT), random forest (RF), and gradient boosting machine (GBM) models. Model performance was assessed by comparing the area under the curve (AUC) of each model. Ultimately, a LR model was selected to construct a nomogram, enhancing the interpretability and visibility of the results.
Feature selection and machine learning performance evaluation
To reduce model complexity and eliminate redundant or irrelevant data in the training group, we applied the Lasso regression model to screen the variables, as illustrated in Figures 2A, B. Besides, 4 machine learning models (LR, DT, RF, and GBM), as illustrated in Figures 3–6 were used in this study. LR is a classification algorithm that seeks to establish a relationship between a feature and the probability of a specific outcome. It possesses the advantage of not presupposing the data distribution and presents results in a probabilistic format, making it appropriate for numerous probability-assisted decision-making tasks. Nonetheless, LR proves ineffective for handling nonlinear data and exhibits heightened sensitivity to imbalances in multicollinearity datasets (13, 14). DT is primarily used for classification tasks, and decision trees start from a root node to identify the initial decision point in a dataset and contain features that best divide the dataset into distinct classes. DT is well-suited for handling irrelevant features, offering a model that is easy to understand and explain. They can be visualized and analyzed, facilitating a clear interpretation of the underlying rules. Additionally, DT is effective in dealing with missing data (15). RF, as an extension of the DT method, combines multiple DTs, with the majority vote among the trees determining the final class prediction of the model. RF incurs a substantial training cost, and the decision-making process of the model is susceptible to the specific division of feature values (16, 17). GBM is a boosting technique utilized as a numerical optimization algorithm for minimizing loss functions and constructing additive models. It proves effective for small-scale datasets, excelling in the processing of multi-classification tasks and accommodating incremental training. Additionally, GBM demonstrates good inclusiveness for handling missing data. However, its performance diminishes when dealing with high-dimensional feature spaces. The effectiveness of GBM in classification tasks is also reliant on the division of feature attributes, making it more sensitive to the expression form of input data (18, 19).
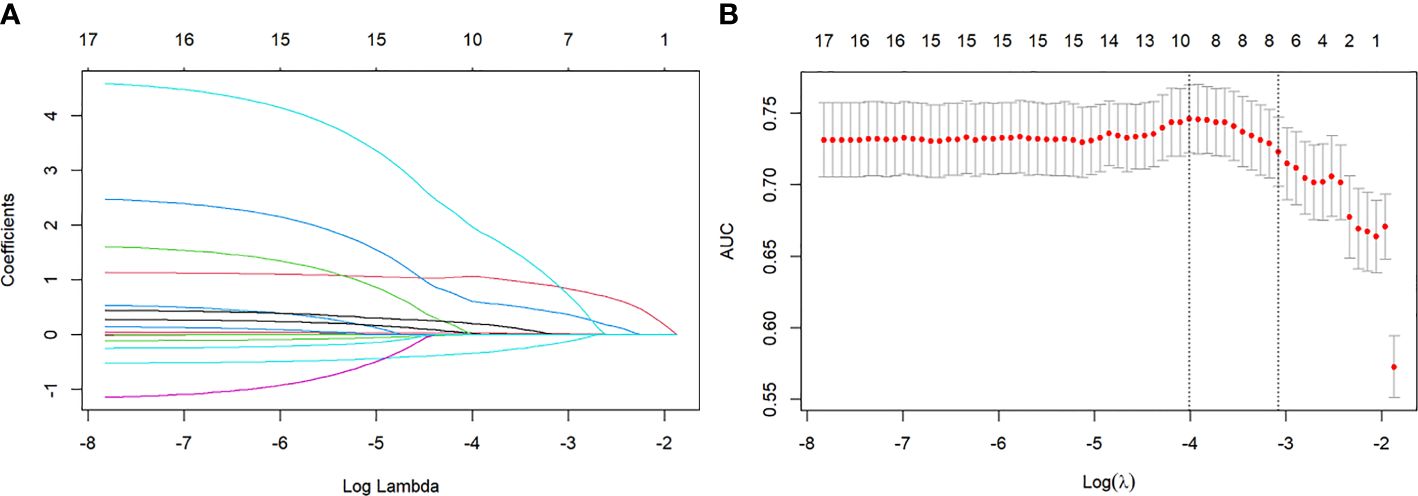
Figure 2 (A) Lasso regression coefficient path diagram. Lasso regression variables were used for dimensionality reduction to further screen the relevant variables. (B) Lasso regression cross validation. Using ten-fold cross-validation, the λ value with the smallest cross-validation error is used as the optimal solution of the model.
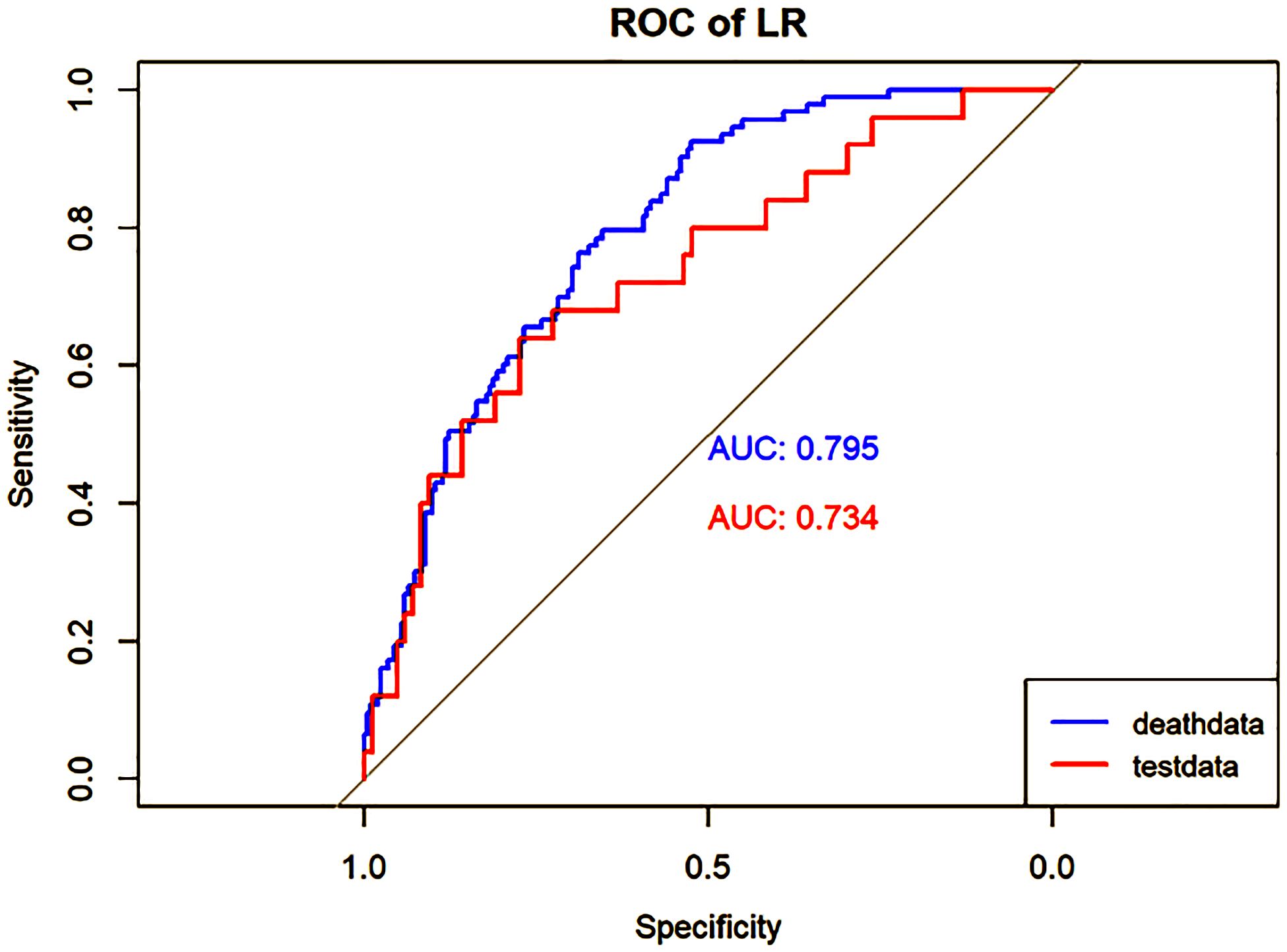
Figure 3 Performance of the LR model. The AUC, Sen and Spe of the training and internal validation sets were exhibited in figure, respectively. ROC, receiver operating characteristic; AUC, area under the curve; Sen, sensitivity; Spe, specificity. Blue line: Training set. Red line: Validation set.
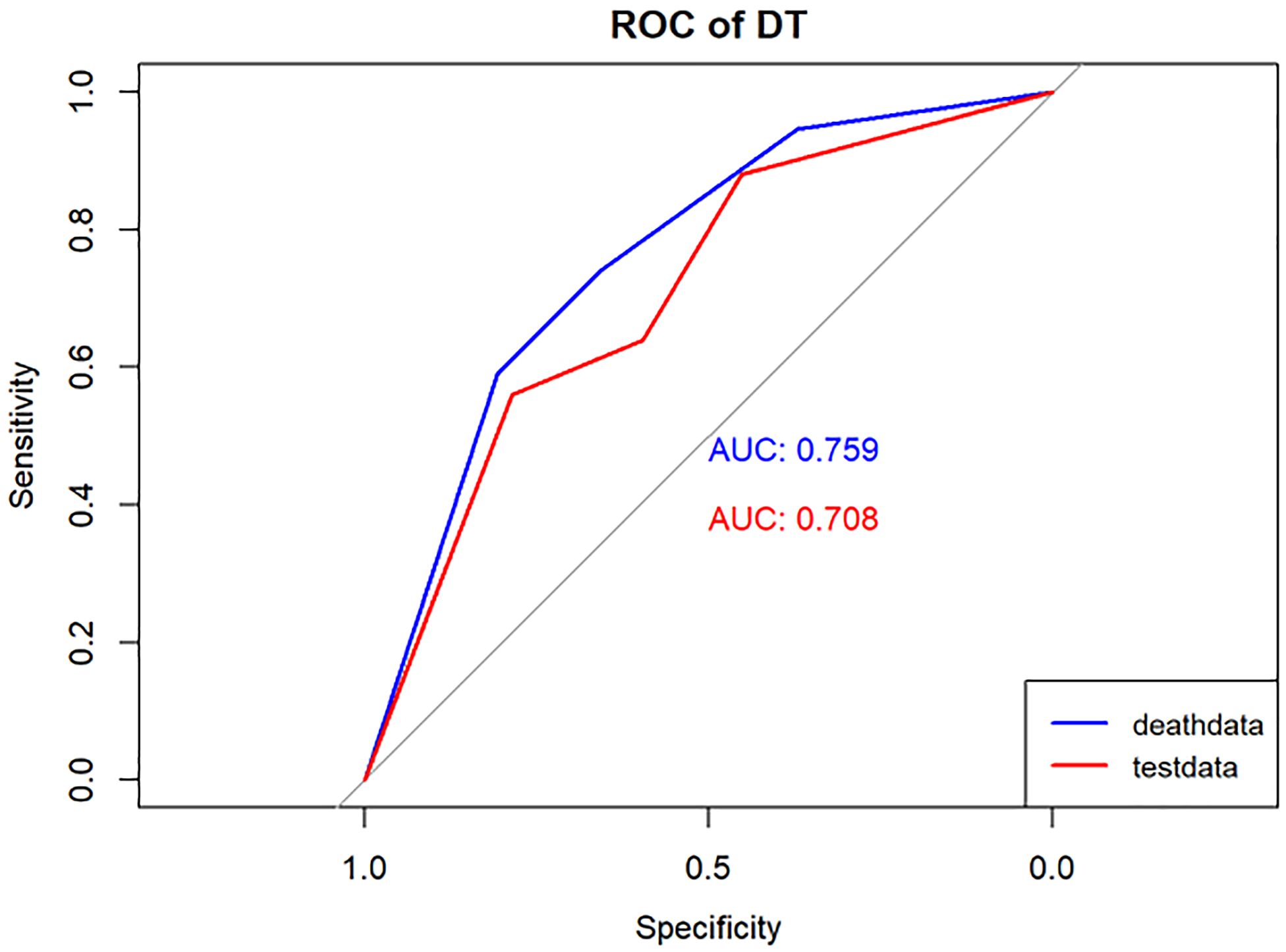
Figure 4 Performance of the DT model. The AUC, Sen and Spe of the training and internal validation sets were exhibited in figure, respectively. ROC, receiver operating characteristic; AUC, area under the curve; Sen, sensitivity; Spe, specificity. Blue line: Training set. Red line: Validation set.
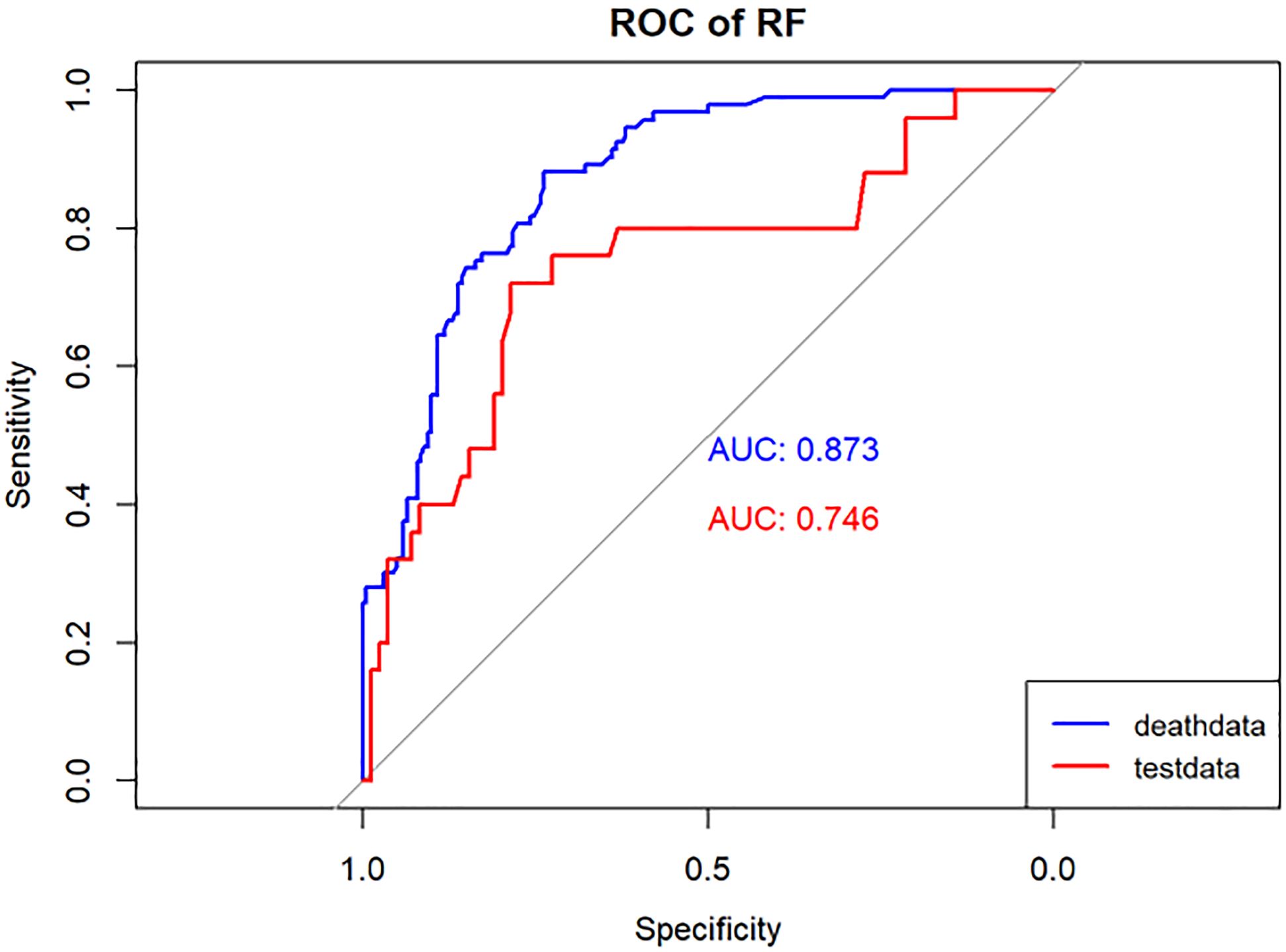
Figure 5 Performance of the RF model. The AUC, Sen and Spe of the training and internal validation sets were exhibited in figure, respectively. ROC, receiver operating characteristic; AUC, area under the curve; Sen, sensitivity; Spe, specificity. Blue line: Training set. Red line: Validation set.
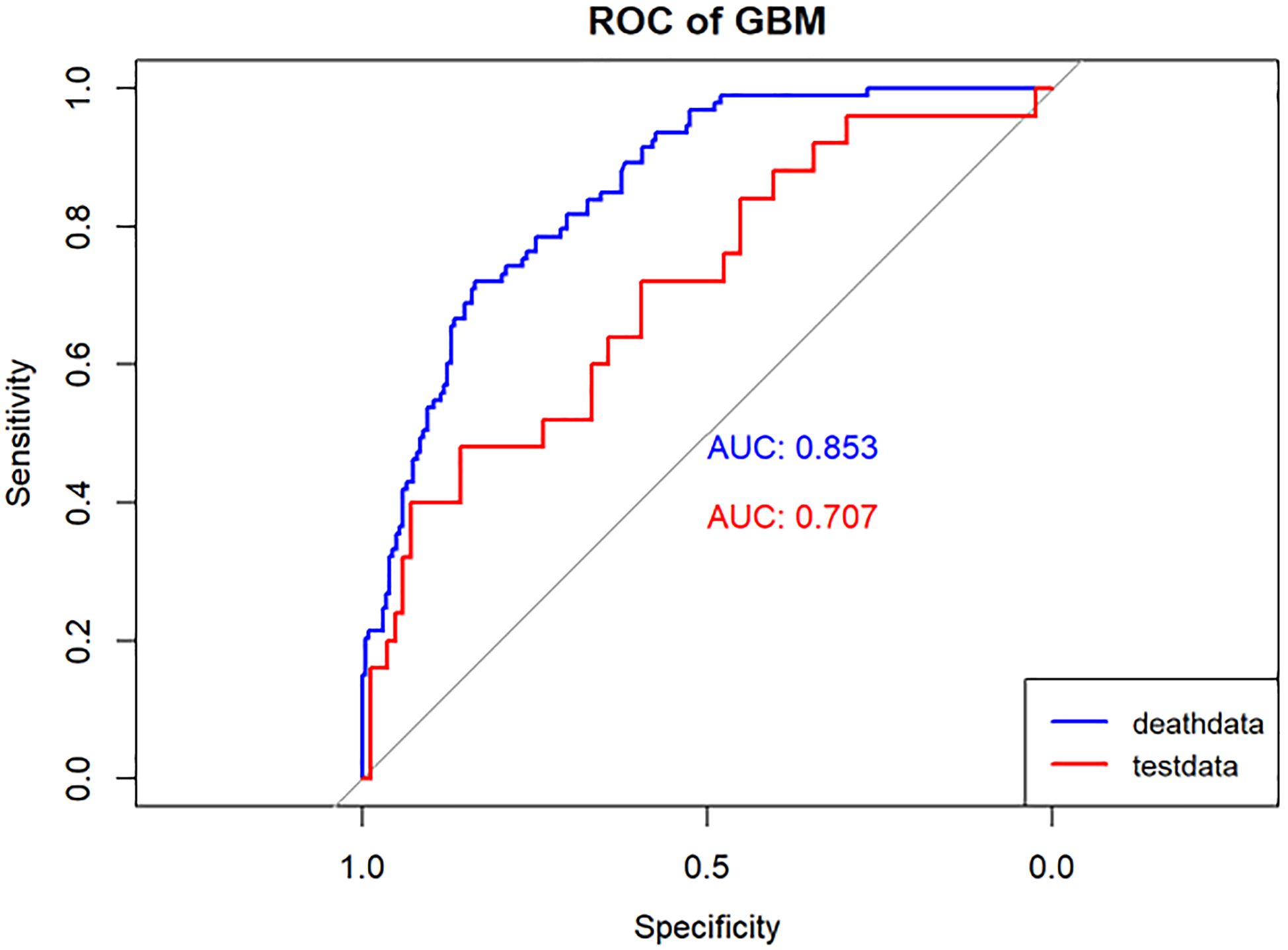
Figure 6 Performance of the GBM model. The AUC, Sen and Spe of the training and internal validation sets were exhibited in figure, respectively. ROC, receiver operating characteristic; AUC, area under the curve; Sen, sensitivity; Spe, specificity. Blue line: Training set. Red line: Validation set.
Model performance was evaluated using various metrics, including accuracy, recall, and the area under the ROC curve, a primary indicator for binary classification performance, ranging from 0 to 1, with higher values signifying superior performance. Additionally, for models with two outcomes, we reported the area under the accuracy-recall curve, which illustrates the trade-off between true accuracy and positive predicted values, as well as the F1 score, defined as the harmonic mean of precision and recall. The models underwent 10-fold cross-validation on the training set and were subsequently tested on the test set, as shown in Tables 1 and 2.
Nomogram
LR was employed to construct a nomogram model for predicting the risk of mortality following radical gastrectomy, utilizing eight variables incorporated into the model. Lines 2 through 9 in the nomogram represent the risk scores associated with individual patients, as shown in Figure 7. The cumulative score serves as an indicator for assessing patients’ prognoses, with higher scores signifying an increased risk level and a poorer prognosis.
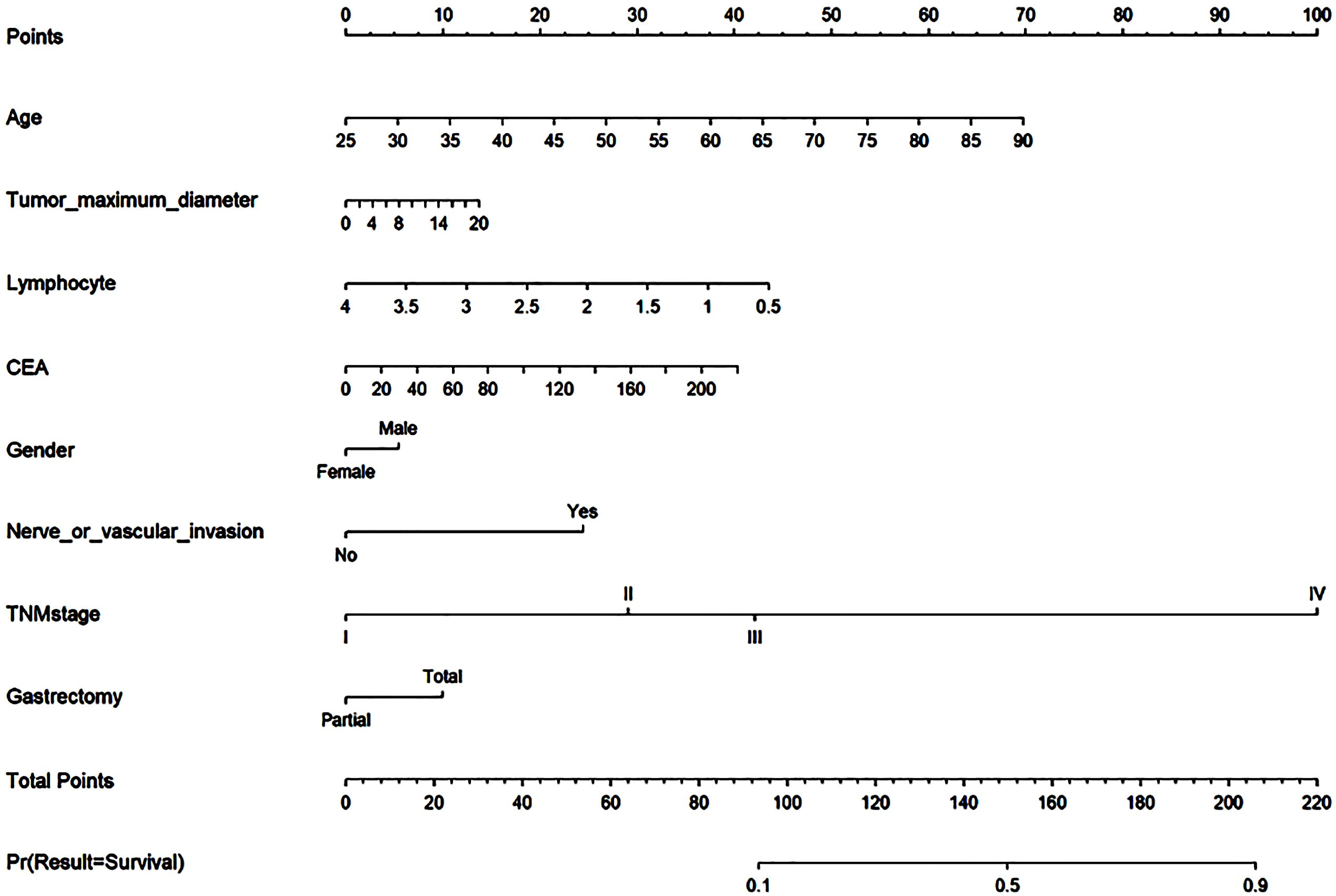
Figure 7 Nomogram. Lines 2 through 9 in the nomogram represent the risk scores associated with individual patients. The cumulative score serves as an indicator for assessing patients’ prognoses, with higher scores signifying an increased risk level and a poorer prognosis.
Results
Patients’ baseline characteristics
Patients’ baseline characteristics are presented in Table 3. The training group consisted of 295 patients, among whom 93 (73 males and 20 females) passed away within 3 years. The validation group comprised 109 patients, with 25 fatalities (14 males and 11 females). In the training group, variables, such as age, maximum tumor diameter, TNM stage, lymph node metastasis, nerve or vascular invasion, type of gastrectomy, lymphocyte count, and CEA level exhibited statistically significant differences between patients who survived and those who succumbed. Conversely, there were no statistically significant differences in gender, tumor differentiation, tumor site, neutrophil count, and monocyte count. In the validation group, significant differences were found in maximum tumor diameter, TNM stage, lymph node metastasis, and nerve or vascular invasion, while other variables did not exhibit significant differences.
Discussion
Machine learning employs computer algorithms to identify intricate relationships or patterns within extensive datasets. It accomplishes this by performing numerous operations using pre-existing algorithms to recognize and analyze data. Through iterative adjustments to these algorithms, machine learning strives to achieve optimal performance, resulting in the creation of models that establish connections between multiple variables and target variables (20). In essence, supervised machine learning is tasked with identifying associations between input and output data, enabling the prediction of outcomes based on patients’ data (21). Machine learning represents a fundamental shift in healthcare, where computers glean insights from patient data without the need for explicit programming of specific tasks. This approach possesses the advantages of enhanced capacity, objectivity, and repeatability when handling large datasets, thereby ensuring data reliability (22, 23). It has the potential to enhance the quality of early diagnosis, disease progression monitoring, and the ability to predict patient-specific outcomes in orthopedics, such as prognosis, risk of complications, and implant longevity (24). These advantages promote the sharing of decision-making information between healthcare professionals and patients, facilitating effective planning and rational utilization of healthcare services (25, 26). In addition, the model can be periodically retrained to improve prediction accuracy over time (27).
In the present study, Lasso regression was employed to identify 8 risk factors associated with postoperative mortality in gastric cancer patients. Additionally, we established four machine learning models to assess patient prognosis and created nomograms to evaluate prognosis based on LR. Lasso regression effectively filtered out non-statistically significant variables during the variable screening process, thereby reducing data redundancy and enhancing the model’s accuracy and reliability by using fewer variables. This approach to developing clinical models has found applications in various medical domains (28, 29). The models’ performance was assessed using the ROC curve, with metrics, such as AUC values, sensitivity, specificity, and accuracy. Table 1 illustrates that all four models exhibit commendable accuracy, indicating the robust diagnostic capability of the machine learning models for predicting postoperative prognosis in gastric cancer patients. Table 2 further validates these findings in the verification group, demonstrating the models’ strong external applicability. Collectively, these results underscore the effectiveness of machine learning models in accurately reflecting postoperative outcomes in gastric cancer surgery (30, 31).
The postoperative prognosis histogram provides an intuitive representation of prognostic risk in gastric cancer patients. Figure 7 illustrates specific scores assigned to variables including age, gender, lymphocyte count, maximum tumor diameter, CEA level, nerve or vascular invasion, TNM stage, and gastrectomy method. In the previous study, Hu used traditional methods to establish clinical models to prove positive LNs, tumor size, adjacent organs invasion, vascular invasion, CA125, the depth of invasion, and HER2 status is the reason that affects radical gastrectomy (32). In the model established by our machine learning algorithm, age and gender are also proved to be the factors that affect the prognosis of radical gastrectomy, which exactly proves that the machine learning algorithm has more powerful computing power.
A nomogram serves as a valuable tool for stratifying the risk of patients, enabling clinicians to assess their conditions effectively. This model assigns scores to various characteristic variables, allowing clinicians to evaluate a patient’s status based on these characteristics. Higher scores on the nomogram indicate an increased susceptibility to risk and a less favorable prognosis. Consequently, patients with distinct scores can benefit from tailored treatment strategies, ensuring a more personalized approach to their healthcare. For instance, determining whether to administer chemotherapy to postoperative gastric cancer patients is typically based on clinical recommendations for patients in stage 1b to stage 3. However, the decision regarding when to initiate chemotherapy for stage 1b to stage 3 patients can be informed by the risk score derived from the histogram. Among patients at the same stage, those with higher scores may be advised to pursue additional treatments. This approach effectively stratifies patients based on their individual conditions, facilitating personalized diagnosis and treatment.
The model identified 8 risk factors for postoperative death in gastric cancer patients using Lasso regression. In addition, 4 machine learning models were developed to assess patient prognosis and nomograms were established based on LR to predict patients’ outcomes. Lasso regression effectively filtered out irrelevant factors, reducing data redundancy, and enhancing model accuracy and reliability with fewer variables. This approach has been applied in various medical fields.
Limitation
There are certain limitations in this study. The retrospective nature of the study may introduce subjective and selective biases,The reliability and validity of the data are limited, and we cannot completely eliminate the possibility of selection bias. Moreover, despite being a two-center study, the sample size remains relatively limited. Further validation with large-scale research is essential to confirm the model’s external applicability.
Conclusions
In conclusion, age, gender, lymphocyte count, maximum tumor diameter, CEA level, nerve or vascular invasion, TNM stage, and gastrectomy method could serve as risk factors influencing the postoperative survival of gastric cancer patients. The machine learning model, established through Lasso regression, demonstrated promising performance and reliability. The nomogram model, which is based on the LR model, provides a practical tool for individualized diagnosis and treatment in clinical settings.
Data availability statement
The raw data supporting the conclusions of this article will be made available by the authors, without undue reservation.
Ethics statement
The studies involving humans were approved by Affiliated Hospital of Xuzhou Medical University. The studies were conducted in accordance with the local legislation and institutional requirements. The ethics committee/institutional review board waived the requirement of written informed consent for participation from the participants or the participants’ legal guardians/next of kin because this study protocol was approved by the Ethics Committee.
Author contributions
TLu: Writing – original draft. ML: Writing – review & editing. HL: Methodology, Writing – review & editing. DS: Writing – review & editing. ZW: Data curation, Writing – review & editing. YG: Data curation, Writing – review & editing. YF: Data curation, Writing – review & editing. QC: Supervision, Writing – review & editing. TLi: Investigation, Writing – review & editing.
Funding
The author(s) declare that no financial support was received for the research, authorship, and/or publication of this article.
Conflict of interest
The authors declare that the research was conducted in the absence of any commercial or financial relationships that could be construed as a potential conflict of interest.
The handling editor, QZ, declared a shared parent affiliation with the author ML at the time of review.
Publisher’s note
All claims expressed in this article are solely those of the authors and do not necessarily represent those of their affiliated organizations, or those of the publisher, the editors and the reviewers. Any product that may be evaluated in this article, or claim that may be made by its manufacturer, is not guaranteed or endorsed by the publisher.
References
1. Leowattana W, Leowattana P, Leowattana T. Immunotherapy for advanced gastric cancer. World J Methodol (2023) 13(3):79–97. doi: 10.5662/wjm.v13.i3.79
2. Guan WL, He Y, Xu RH. Gastric cancer treatment: recent progress and future perspectives. J Hematol Oncol (2023) 16(1):57. doi: 10.1186/s13045-023-01451-3
3. Liu HN, Qu PF. Chinese guidelines for diagnosis and treatment of gastric cancer 2018. Chin J Cancer Res (2019) 31(5):707–73. doi: 10.21147/j.issn.1000-9604.2019.05.01
4. Wang J, Qin D, Tao Z, Wang B, Xie Y, Wang Y, et al. Identification of cuproptosis-related subtypes, construction of a prognosis model, and tumor microenvironment landscape in gastric cancer. Front Immunol (2022) 13:1056932. doi: 10.3389/fimmu.2022.1056932
5. Li H, Lin D, Yu Z, Li H, Zhao S, Hainisayimu T, et al. A nomogram model based on the number of examined lymph nodes-related signature to predict prognosis and guide clinical therapy in gastric cancer. Front Immunol (2022) 13:947802. doi: 10.3389/fimmu.2022.947802
6. Sung H, Ferlay J, Siegel RL, Laversanne M, Soerjomataram I, Jemal A, et al. Global Cancer Statistics 2020: GLOBOCAN estimates of incidence and mortality worldwide for 36 cancers in 185 countries. CA Cancer J Clin (2021) 71(3):209–49. doi: 10.3322/caac.21660
7. Cupp MA, Cariolou M, Tzoulaki I, Aune D, Evangelou E, Berlanga-Taylor AJ. Neutrophil to lymphocyte ratio and cancer prognosis: an umbrella review of systematic reviews and meta-analyses of observational studies. BMC Med (2020) 18(1):360. doi: 10.1186/s12916-020-01817-1
8. Feng F, Tian Y, Xu G, Liu Z, Liu S, Zheng G, et al. Diagnostic and prognostic value of CEA, CA19-9, AFP and CA125 for early gastric cancer. BMC Cancer (2017) 17(1):737. doi: 10.1186/s12885-017-3738-y
9. McMaster C, Bird A, Liew DFL, Buchanan RR, Owen CE, Chapman WW, et al. Artificial intelligence and deep learning for rheumatologists. Arthritis Rheumatol (2022) 74(12):1893–905. doi: 10.1002/art.42296
10. Mainali G. Artificial intelligence in medical science: perspective from a medical student. JNMA J Nepal Med Assoc (2020) 58(229):709–11. doi: 10.31729/jnma.5257
11. Liu PR, Lu L, Zhang JY, Huo TT, Liu SX, Ye ZW. Application of artificial intelligence in medicine: an overview. Curr Med Sci (2021) 41(6):1105–15. doi: 10.1007/s11596-021-2474-3
12. Takeshima H. Deep learning and its application to function approximation for MR in medicine: an overview. Magn Reson Med Sci (2022) 21(4):553–68. doi: 10.2463/mrms.rev.2021-0040
13. Zhou CM, Wang Y, Yang JJ, Zhu Y. Predicting postoperative gastric cancer prognosis based on inflammatory factors and machine learning technology. BMC Med Inform Decis Mak (2023) 23(1):53. doi: 10.1186/s12911-023-02150-2
14. Song X, Liu X, Liu F, Wang C. Comparison of machine learning and logistic regression models in predicting acute kidney injury: A systematic review and meta-analysis. Int J Med Inform (2021) 151:104484. doi: 10.1016/j.ijmedinf.2021.104484
15. Koga S, Zhou X, Dickson DW. Machine learning-based decision tree classifier for the diagnosis of progressive supranuclear palsy and corticobasal degeneration. Neuropathol Appl Neurobiol (2021) 47(7):931–41. doi: 10.1111/nan.12710
16. Collin FD, Durif G, Raynal L, Lombaert E, Gautier M, Vitalis R, et al. Extending approximate Bayesian computation with supervised machine learning to infer demographic history from genetic polymorphisms using DIYABC Random Forest. Mol Ecol Resour (2021) 21(8):2598–613. doi: 10.1111/1755-0998.13413
17. Choi RY, Coyner AS, Kalpathy-Cramer J, Chiang MF, Campbell JP. Introduction to machine learning, neural networks, and deep learning. Transl Vis Sci Technol (2020) 9(2):14. doi: 10.1167/tvst.9.2.14
18. Cha GW, Moon HJ, Kim YC. Comparison of random forest and gradient boosting machine models for predicting demolition waste based on small datasets and categorical variables. Int J Environ Res Public Health (2021) 18(16):8530. doi: 10.3390/ijerph18168530
19. Senders JT, Staples P, Mehrtash A, Cote DJ, Taphoorn MJB, Reardon DA, et al. An online calculator for the prediction of survival in glioblastoma patients using classical statistics and machine learning. Neurosurg (2020) 86(2):E184–92. doi: 10.1093/neuros/nyz403
20. Obermeyer Z, Emanuel EJ. Predicting the future - big data, machine learning,and clinical medicine. N Engl J Med (2016) 375:1216e9. doi: 10.1056/NEJMp1606181
21. Bayliss L, Jones LD. The role of artificial intelligence and machine learning inpredicting orthopaedic outcomes. Bone Joint J (2019) 101-b:1476e8. doi: 10.1302/0301-620X.101B12.BJJ-2019-0850.R1
22. Devries Z, Hoda M, Rivers CS, Maher A, Phan P. Development ofan unsupervised machine learning algorithm for the prognosticationofwalking ability in spinal cord injury patients. Spine J (2019) 20:213–24. doi: 10.1016/j.spinee.2019.09.007
23. Bien N, Rajpurkar P, Ball RL, Irvin J, Lungren MP. Deep-learning-assisted diagnosis for knee magnetic resonance imaging: developmentand retrospective validation of MRNet. PloS Med (2018) 15:e1002699. doi: 10.1371/journal.pmed.1002699
24. Wu EQ, Deng PY, Qu XY, Tang Z, Sheng RSF. Detecting fatiguestatus ofpilots based on deep learning network using eeg signals. IEEE Trans Cognit DevSyst (2020) 13:575–85. doi: 10.1109/TCDS.2019.2963476
25. Anajemba JH, Iwendi C, Mittal M, Tang Y. (2020). Improved advanceencryption standard with a privacy database structure for IoT nodes, in: 2020 IEEE 9th Int Conf Commun Syst Netw Technol Gwalior, India Vol. 13. pp. 575–85. doi: 10.1109/CSNT48778.2020.9115741
26. Tang Z, Zhu R, Lin P, He J, Wang H, Huang Q, et al. A hardware friendlyunsupervised memristive neural network with weight sharing mechanism. Neurocomput (2019) 332:193–202. doi: 10.1016/j.neucom.2018.12.049
27. Tang Z, Zhu R, Hu R, Chen Y, Chang S. A multilayer neural networkmerging image preprocessing and pattern recognition by integrating diffusionand drift memristors. IEEE Trans Cognit Dev Syst (2020) 24:625–85. doi: 10.1109/TCDS.2020.3003377
28. Chen DL, Cai JH, Wang CCN. Identification of key prognostic genes of triple negative breast cancer by LASSO-based machine learning and bioinformatics analysis. Genes (Basel) (2022) 13(5):902. doi: 10.3390/genes13050902
29. Han H, Chen Y, Yang H, Cheng W, Zhang S, Liu Y, et al. Identification and verification of diagnostic biomarkers for glomerular injury in diabetic nephropathy based on machine learning algorithms. Front Endocrinol (Lausanne) (2022) 13:876960. doi: 10.3389/fendo.2022.876960
30. Hu X, Yang Z, Chen S, Xue J, Duan S, Yang L, et al. Development and external validation of a prognostic nomogram for patients with gastric cancer after radical gastrectomy. Ann Transl Med (2021) 9(23):1742. doi: 10.21037/atm-21-6359
31. Lin JX, Lin JP, Xie JW, Wang JB, Lu J, Chen QY, et al. Prognostic importance of the preoperative modified systemic inflammation score for patients with gastric cancer. Gastric Cancer (2019) 22(2):403–12. doi: 10.1007/s10120-018-0854-6
Keywords: machine learning, gastric cancer, prognosis, clinical model, nomogram model
Citation: Lu T, Lu M, Liu H, Song D, Wang Z, Guo Y, Fang Y, Chen Q and Li T (2024) Establishment of a prognostic model for gastric cancer patients who underwent radical gastrectomy using machine learning: a two-center study. Front. Oncol. 13:1282042. doi: 10.3389/fonc.2023.1282042
Received: 23 August 2023; Accepted: 21 December 2023;
Published: 11 April 2024.
Edited by:
Qun Zhang, Nanjing Medical University, ChinaReviewed by:
Liang Chao, Ningbo University, ChinaChangwei Lin, Central South University, China
Christian Cotsoglou, Ospedale di Vimercate - ASST Brianza, Italy
Peiqiang Yan, Harvard Medical School, United States
Fan Zhang, Duke University, United States
Yiyi Ji, Duke University, United States
Copyright © 2024 Lu, Lu, Liu, Song, Wang, Guo, Fang, Chen and Li. This is an open-access article distributed under the terms of the Creative Commons Attribution License (CC BY). The use, distribution or reproduction in other forums is permitted, provided the original author(s) and the copyright owner(s) are credited and that the original publication in this journal is cited, in accordance with accepted academic practice. No use, distribution or reproduction is permitted which does not comply with these terms.
*Correspondence: Daqing Song, 19552153365@163.com
†These authors have contributed equally to this work and share first authorship