Phase of firing as a local window for efficient neuronal computation: tonic and phasic mechanisms in the control of theta spike phase
- 1 Department of Brain and Cognitive Engineering, Korea University, Seoul, South Korea
- 2 Department of Physiology, Anatomy and Genetics, University of Oxford, Oxford, UK
- 3 Physiology Laboratory, Department of Physiology, Development and Neuroscience, University of Cambridge, Cambridge, UK
Introduction
The nature of the neural code remains a central issue of contention in neuroscience. Firing rate based schemes have dominated thinking for most of the past century, but there is a growing acceptance that temporal patterns of neuronal activity have an important role to play, at least in some systems and circumstances. Neuronal oscillations provide a central pillar in the evidence supporting temporal coding, perhaps because temporal codes can ultimately be understood only in the context of population activity and oscillations are at once experimentally accessible and analytically tractable.
In the many roles proposed for oscillatory activity, a uniting theme is the control of spike timing, which can broadly be considered on two timescales. On the one hand, fast oscillations may be important in promoting precise synchronization of activity across cells, by providing millisecond windows of enhanced spike probability. Slow oscillations, on the other hand, can provide a broader temporal scaffold, against which other inputs, both tonic and phasic, can determine spike timing on the order of milliseconds to tens of milliseconds. Again, this could be important for synchronization of activity, but equally, could be used to control spike order, for coding, or plasticity purposes, or could be used to desynchronize discrete assemblies, enabling parallel processing. Here, we describe the cellular mechanisms underlying this broader timescale process in the hippocampus, specifically focusing on the effect of tonic and phasic inputs on the control of spike timing in single hippocampal neurons during theta oscillations and the implications for information coding and storage.
Tonic Input: Rate-to-Phase Transform
Probably the best studied example of oscillation-based coding is the phenomenon of hippocampal place cell phase precession in rodents (O’Keefe and Recce, 1993). Place cells are so-called because their activity increases when an animal passes through a limited spatial location, the place field, analogous to the receptive field familiar from sensory systems (O’Keefe, 1976). The hippocampus is notable for having strong theta-frequency oscillatory activity, and when place cell activity is examined relative to the phase of this oscillation, a systematic relation between spatial location and phase of firing is apparent. On entry to the place field, spikes occur at a certain restricted phase of the oscillation. As the animal traverses the place field, the phase of firing advances monotonically, leading to a strong correlation between spatial location and phase of firing in a given cell (O’Keefe and Recce, 1993). How is this phase code established? Numerous models at both cellular and network levels have been proposed to account for phase precession (Burgess and O’Keefe, 1996; Tsodyks et al., 1996; Kamondi et al., 1998; Harris et al., 2002; Mehta et al., 2002; Lengyel et al., 2003), and recordings of the intracellular dynamics during phase precession of place cells in area CA1 of hippocampus are beginning to constrain these models (Harvey et al., 2009), but the underlying mechanisms remain unclear. It emerges that a very simple biophysical mechanism, described below, can capture core features of the phenomenon. While it is entirely likely that more elaborate mechanisms are at play in establishing the detailed behavior of place cells, this simple mechanism nonetheless provides an excellent starting point for developing insight into the fundamental computations available in an oscillatory neural system.
A key insight is that phase of firing is correlated with firing rate (Harris et al., 2002), at least for the initial period of entry into the place field (Huxter et al., 2003). Increasing firing rate typically implies increased depolarizing drive to a cell and so an obvious question is, what is the predicted effect of increased drive on the phase of firing for a cell receiving oscillatory input?
An intuitive account serves well in this case. For a fixed amplitude of membrane potential oscillation in the cell (whether internally generated or imposed by external inputs), as depolarizing drive increases, eventually a level of input is reached at which the peak of the oscillation just reaches threshold, and so firing occurs at that phase (Figure 1A). As depolarizing drive increases further, the membrane potential reaches threshold slightly earlier on the oscillation, yielding a phase advance. This intuition can be confirmed fairly easily using an analytical description of this system for a leaky integrate-and-fire (IF) model, at least to a first approximation, treating only a single spike on each cycle of the oscillation (for a more complete analytical account of oscillatory cycle locking behaviors, see Coombes and Bressloff, 1999; Gerstner and Kistler, 2002; McLelland and Paulsen, 2009; ). That real neurons demonstrate this behavior has been confirmed experimentally, both in vitro (Figure 1B; McLelland and Paulsen, 2009) and in vivo (Kamondi et al., 1998; Margrie and Schaefer, 2003). The analytical description is useful in providing a starting point to understand the effects of parameter changes (oscillatory input or intrinsic cell properties) on system behavior (Figure 1C). Several points are worth emphasizing (for a more detailed explanation, see McLelland and Paulsen, 2009):
(1) This transform from rate (of inputs) to phase is not reliant on any special properties of the cells, but is automatically implemented in even very simple neuron models (single-compartment leaky IF). Any such neuron with an externally imposed membrane potential oscillation must behave like this (stronger inputs yield earlier phases of firing, or vice versa: early phases imply strong inputs) unless specific mechanisms are in place to override this (e.g., synaptic inputs are of sufficient amplitude or multiple inputs sufficiently synchronous that a spike is driven directly, irrespective of the phase of the ongoing oscillation).
(2) This mechanism can only yield up to 180° of phase advance. As intuition suggests, and models and experiments support, increased drive not only advances the phase of the first spike on every cycle, it also allows spikes to occur at later phases. As such, for levels of input sufficient to advance the first spike by nearly 180°, there may be minimal separation between the first spike in a given cycle and the last spike in the preceding cycle, so that (a) for coding purposes it may be difficult to distinguish first and last spikes and (b) the post-spike conductances from a late phase spike may interfere with phase-locking of the subsequent early phase spike, such that the cell drops out of the phase-locked regime and chaotic firing patterns ensue.
(3) The system works best when the period of the oscillation is substantially longer than the membrane time constant of the cell (and, ideally, the dynamics of post-spike conductances). In this regime, the trough of the oscillation is of sufficient duration for all membrane potential perturbations from the preceding cycle to decay, so that only the tonic level of drive and the oscillation itself determine the timing of the first spike in the subsequent cycle. Thus the system can support a range of firing rates without this perturbing the monotonic phase advance of the first spike per cycle. Further, in this regime, the system is remarkable in not needing to iterate toward a cycle-locked phase: the “correct” phase will be achieved in the first cycle following a change in tonic input, or at the latest, the second cycle.
(4) This transform can operate for faster oscillations (Tiesinga et al., 2002), but in that case the regime is slightly different. The threshold input current is likely to yield a firing rate below the frequency of the oscillation, and spikes in that state will not be phase-locked. Only when drive is sufficient to yield a cycle-locked firing rate will phase-locking and phase precession with increased drive be implemented, and in that regime, the phase of firing on a given cycle is strongly dependent on the phase of firing in the preceding cycle (thus the system has to iterate toward the “correct” phase, and is also more susceptible to noisy perturbations of timing).
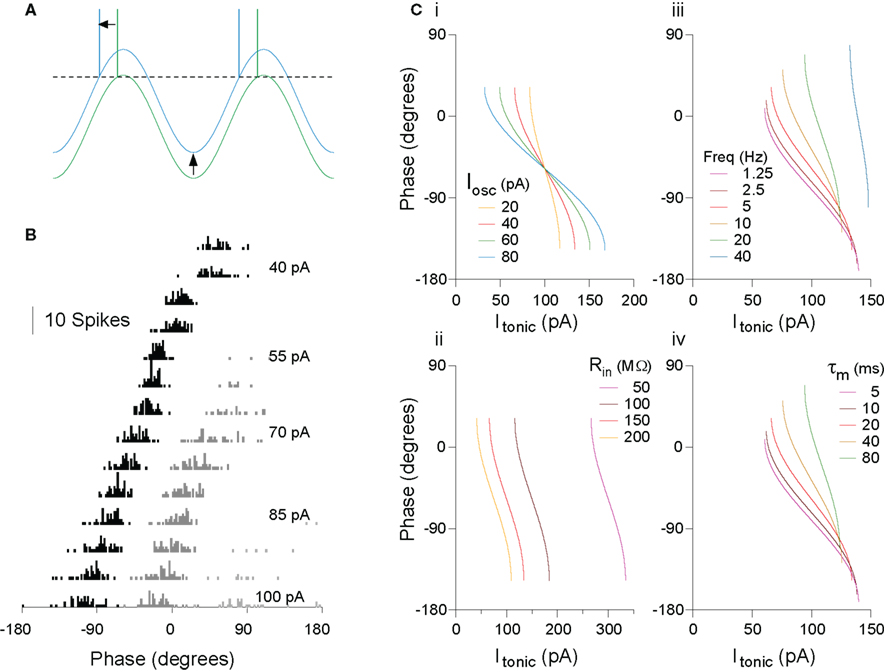
Figure 1. The rate-to-phase transform: Increasing tonic excitation produces progressive spike phase advancement. (A) The transform can be understood at an intuitive level. For a constant amplitude of membrane potential oscillation, as the level of tonic excitatory drive to the cell increases, a level is reached at which the peak of the oscillation is just suprathreshold (green trace). Further increases in excitatory drive lead to earlier crossing of threshold, that is, spike phase advance (blue trace). (B) Real cells implement this transform. Spike phase histogram from hippocampal pyramidal cells in vitro, receiving current injection to simulate a theta-frequency (5 Hz) oscillation of physiologically relevant amplitude (5 mV), and a range of levels of tonic drive. The first spike per cycle (black) advances systematically with increasing drive, and this process is not interrupted as secondary (dark gray) and even tertiary (light gray) spikes are recruited in each cycle. (C) The rate-to-phase transform can be described analytically, to a first approximation, facilitating an understanding of the way in which oscillation and cell parameters will affect the phase–current (φ–I) curve. (i) Changes in oscillation amplitude yield a horizontal scaling of the curve. (ii) Changes to cell input resistance yield only a sideways shift. (iii) Oscillation frequency and (iv) cell membrane time constant have equal but opposite effects (doubling oscillation frequency has the same effect as halving membrane time constant). Note also that, for some parameter ranges, relatively large changes in time constant (e.g., 5–20 ms here) can have fairly small effect on the slope of the φ–I curve. As a result, a constant change in the level of input across a group of cells could yield a similar phase-shift in each cell, independent of their individual conductance states. Modified from McLelland and Paulsen (2009).
Phasic Input: Phase-to-Phase Transform
We have outlined how tonic input can control spike phase relative to slow (e.g., theta-frequency) oscillatory input. However, real synaptic input can never truly be considered tonic, even in the unlikely event that the rate of synaptic events is constant. Thus, the system described above can present only an approximate starting point for the understanding of spike phase control against slow oscillations, albeit, we hope, a useful one. A neuron in an intact network at any given time point is likely to receive myriad additional synaptic inputs simultaneously with those arising as part of the ongoing network oscillation (Buzsaki, 2002). Since these inputs arrive during the oscillation, naturally, they can be considered in terms of their phase relative to the cycle of the oscillation, that is, as phasic inputs. The theoretical treatment of phasic inputs is intrinsically more complex in nature, and can take into account differing synaptic origin, type, location, and amplitude, all of which could influence synaptic integration and the overall spike output, and consequently the spike phase. Here we will discuss how phasic inputs could influence temporal coding during theta-frequency oscillations and how such a code could directly influence the plasticity and computational learning rules of the hippocampal network.
Phase response curves (PRCs) have been adopted in experimental and theoretical studies as a systematic approach to formalize the impact of transient perturbations on the spiking behavior of an oscillatory neuron (Reyes and Fetz, 1993a,b; Ermentrout, 1996; Tateno et al., 2004; Netoff et al., 2005; Tsubo et al., 2007; Kwag and Paulsen, 2009a,b). In PRCs, the spike phase-shift caused by a small depolarizing or hyperpolarizing perturbation is calculated as a function of the phase of perturbation during the spike cycle (often maintained by a tonic depolarizing current). Although PRCs are mostly studied to predict the ability of neurons to synchronize their spiking activity in a network, in fact, what the PRCs are measuring is how phasic inputs influence the spike output-phase during oscillation. Such re-interpretation and application of PRCs has been made in hippocampal CA1 and CA3 pyramidal neurons (Lengyel et al., 2005; Kwag and Paulsen, 2009a,b). Depending on the timing of phasic excitatory inputs, PRCs in hippocampus showed not only spike phase advancement but also delay during oscillations (Lengyel et al., 2005; Kwag and Paulsen, 2009b). When the excitatory input was simulated on the ascending phase of theta, late in the cycle, the effect was similar to that of an increase in tonic drive, advancing spike phase. Strikingly, however, when the same excitatory input was simulated on the descending phase of the oscillation, early in the cycle, then the spike phase was delayed. Indeed, phasic inputs could delay spike phase beyond the peak of the membrane potential oscillation, thus beyond the range that can be achieved with purely tonic input. The amount of spike phase advancement and delay with phasic inputs in CA3 pyramidal neurons is dependent on the amplitude of synaptic input and, in CA1 pyramidal neurons, the synaptic pathway from which the input emanates. In addition to excitatory synaptic inputs, inhibitory synaptic inputs such as the GABAA receptor-mediated inhibitory input impinging on the soma of CA1 pyramidal neurons, are also able to bi-directionally control the spike phases, with its effect reversed compared to that with excitatory input (Kwag and Paulsen, 2009b).
As with tonic input, the ability of excitatory phasic input to advance spike phases with excitatory input is intuitive – excitatory input on the ascending phase of the oscillation causes the membrane potential to reach threshold earlier, yielding phase advance. However, how can an excitatory input on the descending phase of oscillation delay the spike phase? So far, experimental and analytical models have demonstrated that there are two different mechanisms – intrinsic and synaptic. Intrinsic membrane conductances such as the hyperpolarization-activated non-specific cation channel underlying Ih expressed in dendrites of pyramidal neurons can mediate excitation-induced spike phase delay as shown experimentally and theoretically in layer V pyramidal neurons (Goldberg et al., 2007). As we show here using a single-compartment exponential IF neuron, addition of h-conductance alone was sufficient to cause spike phase delay with excitatory input (Figure 2). Recently, Prescott and Sejnowski (2008) argued that subthreshold conductances such as the voltage-activated M-type K+ current (IM) could also improve spike-time coding. Thus, voltage-dependent intrinsic neuronal conductances can contribute to the fine-tuning of the phase of spike firing.
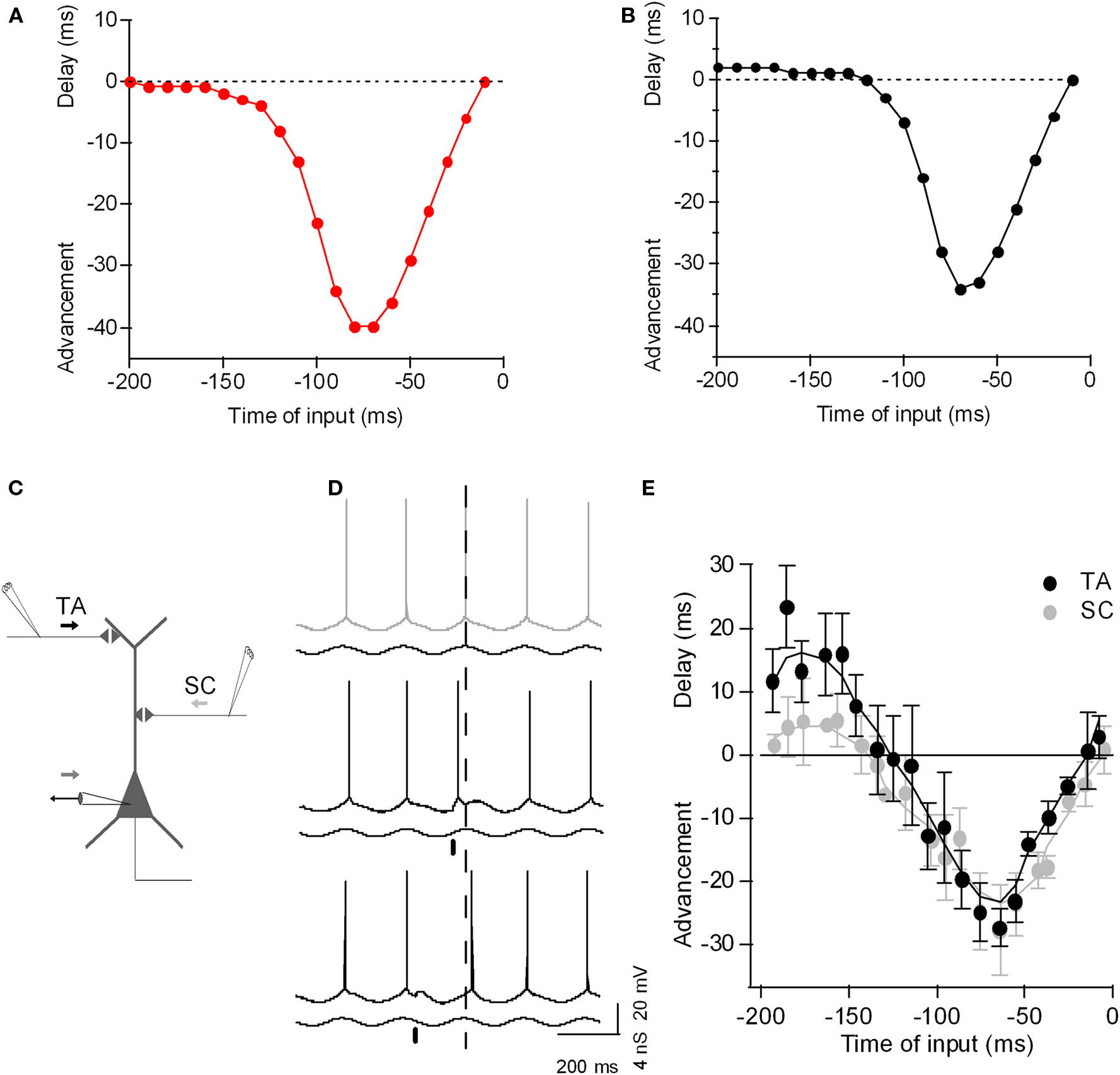
Figure 2. The phase-to-phase transform: Phasic input bi-directionally controls the postsynaptic spike timing during theta-frequency oscillation. (A) Plot of spike-time advancement and delay as a function of the time of phasic input in a simple model (a single-compartment exponential integrate-and-fire model, as described by Fourcaud-Trocme et al. (2003), gL 10 nS, EL −70 mV, C 200 pF, VT −55 mV, ΔT 3 mV, Vreset −60 mV). An oscillation was driven by sinusoidal inhibitory conductance of 0–10 nS at 5 Hz, and a tonic depolarizing current of 120 pA was applied, bringing the neuron past threshold. Excitatory phasic current input with a duration of 10 ms and amplitude of 80 pA was given once per theta cycle and the corresponding spike phase-shift was plotted against the timing of the phasic input. (B) As (A), but with 4 nS of h-channel conductance included (parameters as described by Golding et al., 2005). Excitatory phasic current now yields a delay in spike phase if applied 200–120 ms before the peak of the oscillation. (C) Diagram of experimental set-up: CA1 hippocampal pyramidal neuron with recording electrode at the soma and extracellular electrode stimulating SC input (SC) and TA input (TA). (D) Example voltage traces recorded from CA1 pyramidal neuron during theta oscillation induced by conductance clamp (black trace; minimum inhibitory conductance upward). Without any synaptic perturbation, the neuron spikes near the peak of the oscillation (gray, dashed line). When TA input is stimulated on the ascending phase of the oscillation (black bar), the postsynaptic spike is advanced (black trace). When TA input is stimulated on the descending phase of the oscillation (black bar), the postsynaptic spike is delayed (black trace). (E) Plot of spike-time advancement and delay as a function of the time of TA stimulation (black) or SC stimulation (light gray). Time zero is the average spike-time without TA stimulation. Data are means ± SD of 10 postsynaptic spike-times for each TA stimulation time. Adapted from Kwag and Paulsen (2009b).
However, spike phase delay can also depend on synaptic conductances. For example, (Kwag and Paulsen, 2009b) compared PRCs for different input-pathways to hippocampal CA1 pyramidal cells: intra-hippocampal CA3 inputs and extra-hippocampal entorhinal input (Kwag and Paulsen, 2009b). While phase advancement effects were similar, they found synaptic input-dependence of spike phase delay. Intra-hippocampal CA3 input (the Schaffer collateral input) synapsing onto the proximal dendrites of CA1 pyramidal cells induced only a small degree of spike phase delay whereas temporoammonic pathway (TA) input from layer III of the entorhinal cortex (EC) synapsing onto the distal dendrites of CA1 cells produced much larger spike phase delay. The increased spike phase delay with extra-hippocampal input from the EC is due to the preferential activation of GABAB receptor-mediated inhibition when stimulating TA input, since it was reduced by blockade of GABAB receptor-mediated inhibition (Kwag and Paulsen, 2009b). Whether spike phase delay has any specific physiological and computational function in vivo is as yet unclear but recent experimental evidence suggests that delayed spike phases may code environmental novelty in CA1 pyramidal neurons (Lever et al., 2010).
One intriguing aspect of phasic input is that it can cause bidirectional spike phase-shifts without changing the firing rate. This supports the idea that rate and temporal codes may co-exist, at least partially independently. The variables that could enrich the diversity of such temporal codes include amplitude, synaptic input-pathway, and types of inputs, to name a few. All in all, while we can consider the mean level of synaptic input to behave approximately according to the principles described for tonic input above, that is, increased input will advance spike phase, the potential remains for phasic inputs to both advance and delay postsynaptic spike phase dependent on the timing of the input.
Phase Code Efficiency
Thus far we have described mechanisms by which the phase of firing can be controlled. Just how efficient are these mechanisms? Here, we consider the simple example of the rate-to-phase transform for tonic inputs.
One approach to this question is to estimate the mutual information between the input signal (level of tonic excitation) and the output (phase of firing). Such an estimation serves two purposes. Firstly, when considering phase control as a coding mechanism directly (e.g., a certain phase implies a certain sensory stimulus or situation; Jensen and Lisman, 2000), it allows comparison with other candidate coding schemes, such as the rate code. Secondly, without explicitly considering phase as a direct coding mechanism, oscillatory control of spike timing could support various computational mechanisms (e.g., Hopfield, 1995), the implementation of which depends on the extent to which relative timing across a pair or group of neurons can be precisely controlled, whether the goal be synchronization for increased downstream synaptic effect, or control of spike timing for coding or plasticity purposes. This kind of precision in relative spike timing is precisely what is measured by the mutual information estimate, and so that framework can provide a useful means of building a general understanding of the system. Without entering into the detail of the calculation (Borst and Theunissen, 1999; McLelland and Paulsen, 2009), really just two factors determine the mutual information:
(1)Variance of output-phases for a given input level. Temporal coding schemes in general have the theoretical advantage over rate codes that the encoding variable is continuous. Thus, if the system were noise free and if spike timing could be detected with arbitrary accuracy, then the code could be infinitely accurate. Obviously, for real neurons, this is not the case – even in vitro, in the absence of synaptic noise, intrinsic conductance noise results in a range of output-phases for a given level of excitatory drive to a cell. As the variance of this distribution increases, the mutual information decreases (intuitively, comparing the responses to two levels of input, as the overlap in the distributions of these responses increases, the inputs become less discriminable, Figure 3A). For real neurons, the nature of the spike generating mechanism is such that spike timing precision increases with the steepness of approach to threshold.
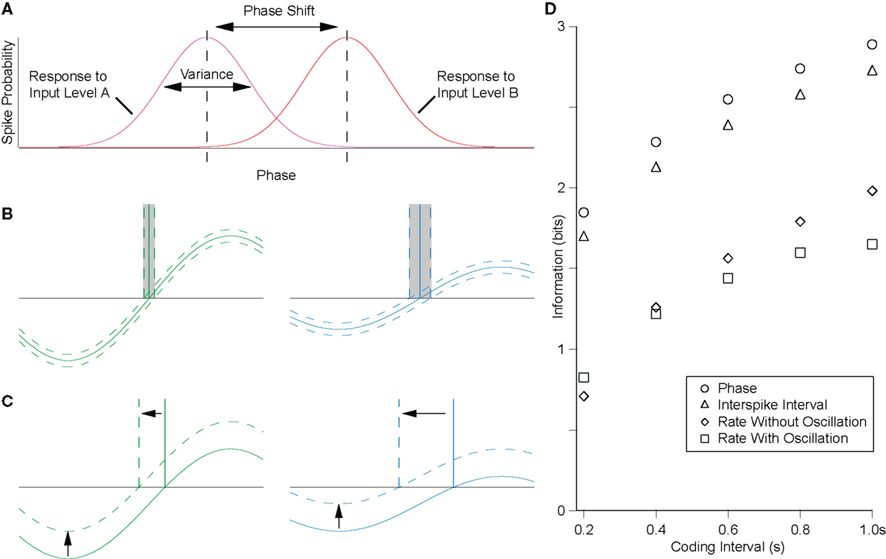
Figure 3. The precision of the rate-to-phase transform can be quantified by the mutual information between the tonic input level and the phase of firing. (A) For the rate-to-phase transform, mutual information increases with two main factors: (i) decreasing the variance in response phase for a given level of tonic input, and (ii) increasing the shift in phase for a given change in the level of tonic input, that is, the slope of the φ–I curve. These factors are both strongly influenced by oscillation amplitude, as follows. (B) The gradient of the membrane potential on approach to threshold influences the susceptibility to noise. For a fixed amplitude of membrane potential noise (dotted lines around the membrane potential trace), increasing oscillation amplitude will decrease response phase variance, increasing mutual information. (C) For precisely the same underlying reasons, increasing the oscillation amplitude will decrease the phase-shift for a given change in tonic input, decreasing the mutual information. (D) The efficiency of the rate-to-phase transform was estimated in hippocampal pyramidal cells in vitro, for a physiologically relevant amplitude (5 mV) of theta-frequency (5 Hz) oscillation. Mutual information was significantly higher for the phase of firing than for the rate of firing, and similar to that for the interspike interval where no oscillation was present (McLelland and Paulsen, 2009).
(2) Slope of the φ/I curve. As well as the variance of phase distributions, the second factor determining mutual information in this system is the phase-shift for a given change in input level. If this shift were large, then even with large response variance, different levels of input would remain discriminable (Figure 3A).
Consider the effect of oscillation parameters on this system. Larger amplitude oscillations will yield lower variance for a given level of input (Figure 3B) but less phase advance for any change in input level (Figures 3C and 1C). In terms of the mutual information, these are competing effects, and ultimately the net effect will depend on the specific system, and the level and temporal structure of any noise therein. Increasing the frequency of oscillations would tend to have the same effect as increasing oscillation amplitude, in that membrane potential gradient tends to increase. However, because the membrane is a low pass filter, oscillation amplitude tends to decrease as frequency increases, offsetting this effect.
The full calculation also has to take into account the distribution of inputs encountered, but the above description should capture the essence of mutual information during oscillations. Thus one can imagine that competing demands determine optimal oscillation amplitude and frequency, to which must be added the effects of the intrinsic properties of the cells involved. At the same time, oscillation frequency is constrained by the demands of the computational task itself.
It is notable that, for a physiologically relevant amplitude of theta-frequency oscillation, spike phase was found to encode significantly more information about the level of tonic input than was encoded by spike rate (Figure 3D; McLelland and Paulsen, 2009). This echoes the finding of increased accuracy in position reconstruction from hippocampal place cell activity when spike phase is taken into account, as compared to the spike rate alone (Jensen and Lisman, 2000). Similarly, in the auditory system, it has recently been reported that the phase of spike patterns relative to low-frequency rhythms encoded additional information and was notably robust to noise (Kayser et al., 2009).
Phase Code for Storing Information
In addition to being a more efficient way of encoding information, precisely controlled spike times may contribute to information storage in a network as changes in synaptic weights via spike timing-dependent plasticity (STDP; Song et al., 2000). In STDP, timing-dependent long-term potentiation (tLTP) of synaptic weight occurs when a presynaptic neuron spikes before the postsynaptic neuron, whereas timing-dependent long-term depression (tLTD) occurs if this order is reversed, both within a time scale of a few tens of milliseconds (Markram et al., 1997; Bi and Poo, 1998). Thus, phasic control of spike phases during oscillation might serve an important function in naturally organizing spikes into time windows conducive to STDP (Paulsen and Sejnowski, 2000; Song et al., 2000). Under such a scenario, a mechanism that could control the spike phase could also control hippocampal information processing as well as plasticity. This has been demonstrated in the hippocampus where the spike phase control mechanism has been directly utilized to control the sign of STDP during oscillation (Kwag and Paulsen, 2009b). Pre-before-post pairing of CA3 and CA1 neurons would normally induce tLTP whereas post-before-pre pairing of these neurons could induce tLTD (Bi and Poo, 1998; Debanne et al., 1998; Kwag and Paulsen, 2009b). However, the timing of direct activation of TA input could control the CA1 firing phase relative to the theta oscillation, and consequently control the sign of plasticity locally in the hippocampus. The switch in plasticity was purely due to the change in spike timing thus the change in the pairing order of pre- and postsynaptic spike times (Figure 4). Simple computational modeling suggests that TA input, with its ability to bi-directionally control spike phases, is special in potentially being able to enforce both tLTP and tLTD in the hippocampal network (Figure 4), whereas SC input, with less ability to phase delay the spike, would promote tLTD in the network (Kwag and Paulsen, 2009b). As entorhinal TA input is suggested to channel most external sensory information into the local hippocampal circuit, the control of STDP by TA input might have implications for how external sensory information could be encoded as spike phases relative to local hippocampal theta and eventually be stored in the local hippocampal network as synaptic weight changes. Thus, under the phasic input-controlled phase coding regime, the change in spike timing may contribute to the control of neuronal computation during oscillation without changing the rate code (Kwag and Paulsen, 2009b). Interestingly, there is a temporal offset between preferred firing phases of entorhinal input and hippocampal CA1 neurons in vivo and such innate temporal delay could render subdivisions of the entorhinal–hippocampal system computationally relatively independent (Mizuseki et al., 2009).
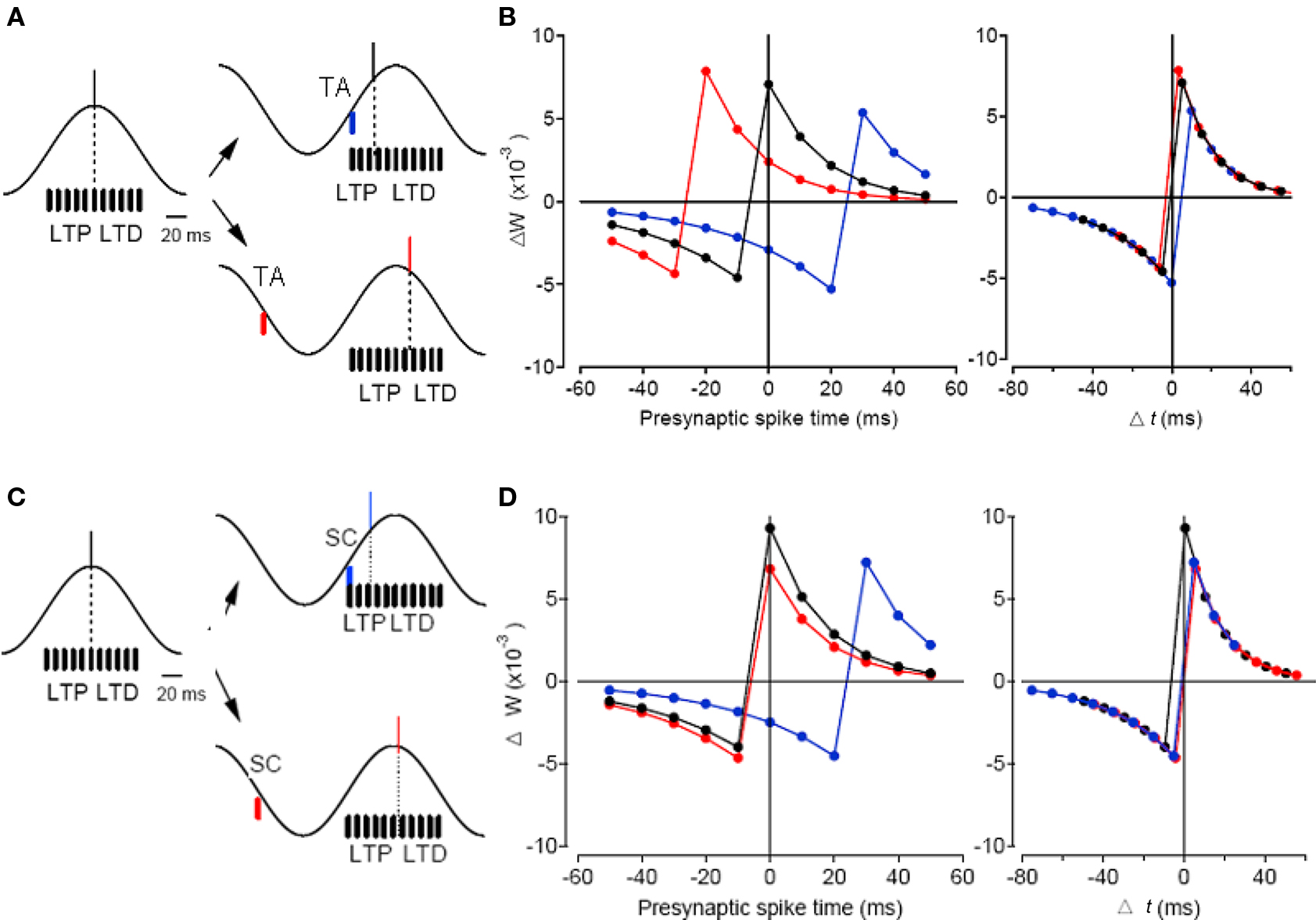
Figure 4. Simple spike timing-based learning model demonstrating modulation of STDP sign by TA input and SC input. (A) Simplified schematic of SC inputs distributed near the peak of theta (black bars) and the effect of postsynaptic spike-time (dashed line) during theta-frequency oscillation (black trace) on the enforcement of STDP sign. Without any TA input activated, the postsynaptic spike occurs near the peak of oscillation (black line). When TA input is activated on the ascending phase of the oscillation (blue bar), it advances the postsynaptic spike (blue line, top right panel) and enforces LTD, whereas when TA input is activated on the descending phase of the oscillation (red bar), it delays the postsynaptic spike (red line, bottom right panel) and enforces LTP. (B) Synaptic weight change plotted against the time of SC input relative to the peak of theta oscillation (left) and against Δt (right) without TA input (black), with TA advancing the spike (blue), and with TA delaying the spike (red). (C,D) Same as (A,B) but with SC input used as phasic input. Note that SC input activated on the ascending phase of the oscillation (blue bar) advances the postsynaptic spike (blue line, top right panel) and enforces LTD whereas SC input activated on the descending phase of the oscillation (red bar) slightly delays the postsynaptic spike (red line, bottom right panel) and the LTP enforcement effect is small. Adapted from Kwag and Paulsen (2009b).
Conclusion
Mounting experimental evidence suggests that phase codes are important in hippocampal processing but the establishment of such phase codes and their roles in information and memory processing are still unclear. Here we have discussed two modes by which phase can be controlled in CA1 hippocampal neurons during oscillations – a rate-to-phase transform for tonic inputs and, building on the same framework, a phase-to-phase transform for phasic inputs. Phase codes are notable for their efficiency in information encoding, and also intrinsically provide a means by which phase-coded information can be stored as changes in synaptic weights. Although the exact nature of the neural code is yet to be uncovered, it is likely that both rate and phase codes co-exist and the mechanisms we have described here suggest how phase coding may yield efficiency in hippocampal network computations.
Acknowledgment
The authors’ research was supported by the BBSRC and the Wellcome Trust. Jeehyun Kwag was supported by the World Class University (WCU) program through the National Research Foundation of Korea funded by the Ministry of Education, Science, and Technology (R31-10008).
References
Bi, G. Q., and Poo, M. M. (1998). Synaptic modifications in cultured hippocampal neurons: dependence on spike timing, synaptic strength, and postsynaptic cell type. J. Neurosci. 18, 10464–10472.
Borst, A., and Theunissen, F. E. (1999). Information theory and neural coding. Nat. Neurosci. 2, 947–957.
Burgess, N., and O’Keefe, J. (1996). Neuronal computations underlying the firing of place cells and their role in navigation. Hippocampus 6, 749–762.
Coombes, S., and Bressloff, P. C. (1999). Mode locking and Arnold tongues in integrate-and-fire neural oscillators. Phys. Rev. E 60, 2086–2096.
Debanne, D., Gahwiler, B. H., and Thompson, S. M. (1998). Long-term synaptic plasticity between pairs of individual CA3 pyramidal cells in rat hippocampal slice cultures. J. Physiol. 507(Pt 1), 237–247.
Ermentrout, B. (1996). Type I membranes, phase resetting curves, and synchrony. Neural Comput. 8, 979–1001.
Fourcaud-Trocme, N., Hansel, D., van Vreeswijk, C., and Brunel, N. (2003). How spike generation mechanisms determine the neuronal response to fluctuating inputs. J. Neurosci. 23, 11628–11640.
Goldberg, J. A., Deister, C. A., and Wilson, C. J. (2007). Response properties and synchronization of rhythmically firing dendritic neurons. J. Neurophysiol. 97, 208–219.
Golding, N. L., Mickus, T. J., Katz, Y., Kath, W. L., and Spruston, N. (2005). Factors mediating powerful voltage attenuation along CA1 pyramidal neuron dendrites. J. Physiol. 568, 69–82.
Harris, K. D., Henze, D. A., Hirase, H., Leinekugel, X., Dragoi, G., Czurko, A., and Buzsaki, G. (2002). Spike train dynamics predicts theta-related phase precession in hippocampal pyramidal cells. Nature 417, 738–741.
Harvey, C. D., Collman, F., Dombeck, D. A., and Tank, D. W. (2009). Intracellular dynamics of hippocampal place cells during virtual navigation. Nature 461, 941–946.
Hopfield, J. (1995). Pattern recognition computation using action potential timing for stimulus representation. Nature 376, 33–36.
Huxter, J., Burgess, N., and O’Keefe, J. (2003). Independent rate and temporal coding in hippocampal pyramidal cells. Nature 425, 828–832.
Jensen, O., and Lisman, J. E. (2000). Position reconstruction from an ensemble of hippocampal place cells: contribution of theta phase coding. J. Neurophysiol. 83, 2602–2609.
Kamondi, A., Acsady, L., Wang, X. J., and Buzsaki, G. (1998). Theta oscillations in somata and dendrites of hippocampal pyramidal cells in vivo: activity-dependent phase-precession of action potentials. Hippocampus 8, 244–261.
Kayser, C., Montemurro, M. A., Logothetis, N. K., and Panzeri, S. (2009). Spike-phase coding boosts and stabilizes information carried by spatial and temporal spike patterns. Neuron 61, 597–608.
Kwag, J., and Paulsen, O. (2009a). Bidirectional control of spike timing by GABA(A) receptor-mediated inhibition during theta oscillation in CA1 pyramidal neurons. Neuroreport 20, 1209–1213.
Kwag, J., and Paulsen, O. (2009b). The timing of external input controls the sign of plasticity at local synapses. Nat. Neurosci. 12, 1219–1221.
Lengyel, M., Kwag, J., Paulsen, O., and Dayan, P. (2005). Matching storage and recall: hippocampal spike timing-dependent plasticity and phase response curves. Nat. Neurosci. 8, 1677–1683.
Lengyel, M., Szatmary, Z., and Erdi, P. (2003). Dynamically detuned oscillations account for the coupled rate and temporal code of place cell firing. Hippocampus 13, 700–714.
Lever, C., Burton, S., Jeewajee, A., Wills, T. J., Cacucci, F., Burgess, N., and O’Keefe, J. (2010). Environmental novelty elicits a later theta phase of firing in CA1 but not subiculum. Hippocampus 20, 229–234.
Margrie, T., and Schaefer, A. (2003). Theta oscillation coupled spike latencies yield computational vigour in a mammalian sensory system. J. Physiol. 546, 363–374.
Markram, H., Lubke, J., Frotscher, M., and Sakmann, B. (1997). Regulation of synaptic efficacy by coincidence of postsynaptic APs and EPSPs. Science 275, 213–215.
McLelland, D., and Paulsen, O. (2009). Neuronal oscillations and the rate-to-phase transform: mechanism, model and mutual information. J. Physiol. 587, 769–785.
Mehta, M. R., Lee, A. K., and Wilson, M. A. (2002). Role of experience and oscillations in transforming a rate code into a temporal code. Nature 417, 741–746.
Mizuseki, K., Sirota, A., Pastalkova, E., and Buzsaki, G. (2009). Theta oscillations provide temporal windows for local circuit computation in the entorhinal–hippocampal loop. Neuron 64, 267–280.
Netoff, T. I., Acker, C. D., Bettencourt, J. C., and White, J. A. (2005). Beyond two-cell networks: experimental measurement of neuronal responses to multiple synaptic inputs. J. Comput. Neurosci. 18, 287–295.
O’Keefe, J. (1976). Place units in the hippocampus of the freely moving rat. Exp. Neurol. 51, 78–109.
O’Keefe, J., and Recce, M. L. (1993). Phase relationship between hippocampal place units and the EEG theta-rhythm. Hippocampus 3, 317–330.
Paulsen, O., and Sejnowski, T. J. (2000). Natural patterns of activity and long-term synaptic plasticity. Curr. Opin. Neurobiol. 10, 172–179.
Prescott, S. A., and Sejnowski, T. J. (2008). Spike-rate coding and spike-time coding are affected oppositely by different adaptation mechanisms. J. Neurosci. 28, 13649–13661.
Reyes, A. D., and Fetz, E. E. (1993a). Effects of transient depolarizing potentials on the firing rate of cat neocortical neurons. J. Neurophysiol. 69, 1673–1683.
Reyes, A. D., and Fetz, E. E. (1993b). Two modes of interspike interval shortening by brief transient depolarizations in cat neocortical neurons. J. Neurophysiol. 69, 1661–1672.
Song, S., Miller, K. D., and Abbott, L. F. (2000). Competitive Hebbian learning through spike-timing-dependent synaptic plasticity. Nat. Neurosci. 3, 919–926.
Tateno, T., Harsch, A., and Robinson, H. P. (2004). Threshold firing frequency–current relationships of neurons in rat somatosensory cortex: type 1 and type 2 dynamics. J. Neurophysiol. 92, 2283–2294.
Tiesinga, P. H. E., Fellous, J. M., Jose, J. V., and Sejnowski, T. J. (2002). Information transfer in entrained cortical neurons. Network 13, 41–66.
Tsodyks, M. V., Skaggs, W. E., Sejnowski, T. J., and McNaughton, B. L. (1996). Population dynamics and theta rhythm phase precession of hippocampal place cell firing: a spiking neuron model. Hippocampus 6, 271–280.
Citation: Kwag J, McLelland D and Paulsen O (2011) Phase of firing as a local window for efficient neuronal computation: tonic and phasic mechanisms in the control of theta spike phase. Front. Hum. Neurosci. 5:3. doi: 10.3389/fnhum.2011.00003
Received: 11 June 2010;
Paper pending published: 07 September 2010;
Accepted: 06 January 2011;
Published online: 02 February 2011.
Copyright: © 2011 Kwag, McLelland and Paulsen. This is an open-access article subject to an exclusive license agreement between the authors and Frontiers Media SA, which permits unrestricted use, distribution, and reproduction in any medium, provided the original authors and source are credited.
*Correspondence: jkwag@korea.ac.kr; douglas.mclelland@dpag.ox.ac.uk; op210@cam.ox.ac.uk