- 1Department of Neurology and Department of Neuroscience, The First Affiliated Hospital of Xiamen University, School of Medicine, Xiamen University, Xiamen, China
- 2Fujian Key Laboratory of Brain Tumors Diagnosis and Precision Treatment, Xiamen, China
- 3Xiamen Key Laboratory of Brain Center, Xiamen, China
- 4Xiamen Medical Quality Control Center for Neurology, Xiamen, China
- 5Fujian Provincial Clinical Research Center for Brain Diseases, Xiamen, China
- 6Xiamen Clinical Research Center for Neurological Diseases, Xiamen, China
- 7Department of Neonatology, The First Affiliated Hospital of Xiamen University, Xiamen, China
Background: Increasing evidence has suggested that alterations in the gut microbiome are correlated with autoimmune neurologic disorders, yet the causal relationship between them has yet to be established.
Methods: From the published genome-wide association study (GWAS) summary statistics, we obtained data on the gut microbiota and three autoimmune neurologic disorders (Multiple Sclerosis, Guillain-Barré Syndrome, and Myasthenia Gravis). We then implemented a two-sample Mendelian Randomization (MR) to determine the causal relationship between the gut microbiota and the diseases. To validate the results, we conducted a series of sensitivity analyses. Finally, to verify the direction of causality, a reverse-causality analysis was done.
Results: We discovered that a higher relative abundance of the genus Ruminococcus2 (OR: 1.213, 95% CI: 1.006–1.462, p = 0.043, PFDR = 0.048) and the genus Roseburia (OR: 1.255, 95% CI: 1.012–1.556, p = 0.038, PFDR = 0.048) were associated with a higher risk of MS. Furthermore, the higher the abundance of the class Mollicutes (OR: 3.016, 95% CI: 1.228–7.411, p = 0.016, PFDR = 0.021), the genus Eubacterium (hallii group) (OR: 2.787, 95% CI: 1.140–6.816, p = 0.025, PFDR = 0.025), and the phylum Tenericutes (OR: 3.016, 95% CI: 1.228–7.411, p = 0.016, PFDR = 0.021) was linked to a greater probability of GBS. Additionally, the higher the abundance of the genus Ruminococcaceae UCG005 (OR: 2.450, 95% CI: 1.072–5.598, p = 0.034, PFDR = 0.036), the genus Holdemania (OR: 2.437, 95% CI: 1.215–4.888, p = 0.012, PFDR = 0.024), genus Lachnoclostridium (OR: 3.681, 95% CI: 1.288–10.521, p = 0.015, PFDR = 0.025) and the genus Eubacterium (ruminantium group) (OR: 2.157, 95% CI: 1.211–3.843, p = 0.003, PFDR = 0.016) correlated with a greater chance of MG occurrence. No SNPs were identified as outliers through sensitivity analysis. Then, the results of the reverse MR analysis did not indicate any reverse causality.
Conclusion: Our findings demonstrate a causal relationship between the gut microbiota and three autoimmune neurologic disorders, providing novel insights into the mechanisms of these autoimmune neurologic disorders that are mediated by gut microbiota.
1 Introduction
Autoimmune neurologic disorders can affect both the central and peripheral nervous systems, such as Multiple Sclerosis (MS), Guillain-Barré Syndrome (GBS), and Myasthenia Gravis (MG) (Vass, 2012). Over the past few decades, the incidence of these diseases has risen steadily, and they have caused a heavy burden to patients and society due to their high mortality and disability rates (Rubin et al., 2018). Therefore, it is imperative to discover potential causal risk factors for autoimmune neurologic disorders.
Despite a lack of understanding of the exact causes of autoimmune neurologic disorders, genetics, environmental factors, and their interactions are believed to play a major role in their development (Diaz-Marugan et al., 2023; Kapoor et al., 2023). Evidence has been mounting that the gut microbiota is closely related to the host’s health and is implicated in the cause of a variety of complex human diseases, including autoimmune diseases (Wang et al., 2023). The gut microbiota is an intricate and ever-changing community of ecological microbes that inhabit the human digestive tract, including bacteria, archaea, viruses, parasites, and other microorganisms (Lozupone et al., 2012). The communication between the microbiota and the nervous system is likely facilitated by the microbiota-gut-brain axis, which involves a variety of pathways such as the immune system, the vagus and enteric nerves, and molecules or metabolites derived from the microbiota (Nunez et al., 2021). Alterations in the composition of gut microbiota, including decreased species diversity and increased presence of pathogens, have been observed in several autoimmune neurologic disorders. For example, an investigation found considerable disparities in the concentrations of operational taxa among MG patients, specifically Firmicutes, Bacteroides, and Actinobacteria (Kapoor et al., 2023). Reports showed that MS patients have a decreased presence of Parabacteroides and Butyricicoccus (Cekanaviciute et al., 2017; Diaz-Marugan et al., 2023), while Amuciniphila, Eggerthellalenta, Bifidobacterium adolescentis, and Ruminococcus taxa are more abundant. Additionally, a strong connection was observed between GBS and Campylobacter jejuni infection (Allos, 1997). Nevertheless, it is still unknown whether there is a causal association between the gut microbiota and autoimmune neurologic disorders.
Conventionally, Randomized Controlled Trials (RCTs) can potentially help establish a causal relationship. However, due to certain limitations such as technological constraints and ethical concerns, these trials can be difficult to implement. As an alternative, Mendelian Randomization (MR) analysis can provide a viable solution. Through the use of genetic variations that are present before the onset of any disease, MR reduces the potential of confounding factors, reverse causation, or other biases from affecting the accuracy of the results. By leveraging the Genome-Wide Association Study (GWAS), Single Nucleotide Polymorphisms (SNPs) associated with the exposure can be used as Instrumental Variables (IVs) in a two-sample MR analysis to determine the causal relationship between the exposure and the outcome (Davey Smith and Hemani, 2014; Bowden and Holmes, 2019).
In this study, we conducted a MR analysis using publicly available GWAS data to explore the potential causal relationship between the gut microbiota and three autoimmune neurologic disorders: MS, MG, and GBS. Our research may provide a novel perspective on developing new treatment strategies, such as probiotic therapy and dietary modulations for autoimmune neurologic disorders.
2 Methods
2.1 Study design
This study used publicly available data from a GWAS summary to identify suitable IVs for MR analysis to investigate the causal relationship between gut microbiota and three autoimmune neurologic disorders: MS, MG, and GBS (Figure 1). Three assumptions were strictly adhered to in order to ensure the accuracy of the results: (Vass, 2012) the IVs should relate to the exposure; (Rubin et al., 2018) they should not be related to any confounding factors; (Diaz-Marugan et al., 2023) their effects on outcomes should only be through the exposure. Ethical approval and informed consent have been provided in the original studies.
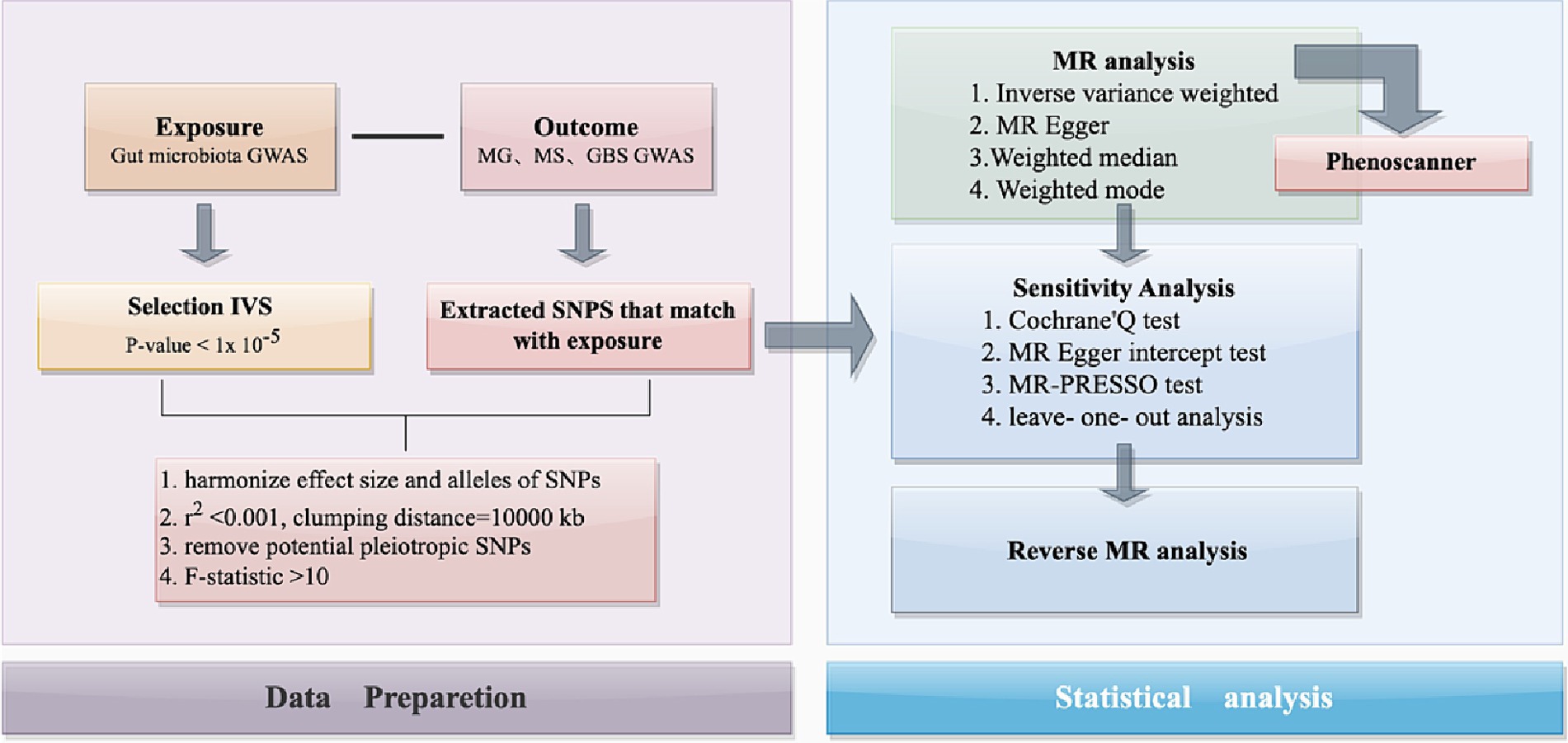
Figure 1. The flowchart of the present study. The whole workflow of MR analysis. MR, Mendelian randomization; MS, Multiple Sclerosis; GBS, Guillain-Barré Syndrome; MG, Myasthenia Gravis.
2.2 Data sources
2.2.1 Exposure data
The MiBioGen consortium1 conducted a large-scale GWAS study to obtain summary statistics of the gut microbiome, comprising data from 24 cohorts from different countries and a total of 18,340 individuals (Kurilshikov et al., 2021). A total of 211 microbial community data were analyzed, encompassing 9 phyla, 16 classes, 20 orders, 35 families, and 131 genera. After removing 17 unknown groups, 194 microbial taxa were taken into consideration.
2.2.2 Outcome data
The outcome data generated comes from a publicly accessible database, which contains a comprehensive description of the data in the original source. The MS data was from a GWAS conducted by the International Multiple Sclerosis Genetics Consortium (IMSGC) in 2019, involving 115,803 individuals, with 47,429 of them being MS patients and 68,374 being healthy controls (International Multiple Sclerosis Genetics Consortium, 2019). The Finnish database provided GWAS data for MG and GBS. There were 232 cases and 217,056 controls for MG, making a total of 217,288 individuals, and 213 cases and 215,718 controls for GBS, resulting in 215,931 individuals. To ensure consistency in the study, all outcome GWAS data used were from individuals of European ethnicity. All GWAS data utilized in the analysis is detailed in Table 1.
2.3 Instrumental variable selection (IVs)
To confirm the causal relationship between the gut microbiome and autoimmune neurologic disorders, we utilized quality control modalities to select the relevant IVs. Initially, SNPs that had a significant correlation with the gut microbiome were chosen to be IVs. Given that only a minor proportion of the gut microbiome exhibits more than three independent SNPs at a genome-wide significant threshold with a p < 5 × 10−8, we opted to raise the p-value to 1 × 10−5 to obtain an adequate number of IVs and yield more comprehensive outcomes. Secondly, SNPs with a minor allele frequency (MAF) of less than 0.01 were not included. Thirdly, to avoid IVs with linkage disequilibrium (LD), the SNPs chosen in our study were assessed against the European 1000 Genomes Project reference panel, with the requirements of r2 < 0.001 and clump distance >10,000 kb. According to the formula F = (R2 denotes the fraction of variance explained by genetic variation in the exposure, N represents the sample size, and K is the number of SNPs.) (Li et al., 2022), if the F-statistic>10, it can be deduced that there is no remarkable weak tool bias. Phenoscanner is employed to recognize potential confounding elements (Zhang et al., 2023).
2.4 Statistical analysis
2.4.1 MR analysis
We applied a two-sample MR method to investigate the causal link between microbiome characteristics and three autoimmune neurological disorders. Four methods commonly used for features with multiple IVs are Inverse-Variance Weighted (IVW) tests, Weighted Modes (Hartwig et al., 2017), Weighted Median Estimator (WME) (Bowden et al., 2016), and MR-Egger regression (Hartwig et al., 2017). Evidence from prior studies has shown that the IVW method produces more meaningful results than other approaches (Burgess et al., 2013), thus this study primarily utilizes the IVW method. With the other methods providing supplementary explanations. The OR and 95% confidence interval (CI) were used to demonstrate the effect size. The Weighted Modes, WME method and the MR-Egger test were employed as additional approaches for MR analysis in the study. The Benjamini–Hochberg (BH) method was applied to calculate a false discovery rate p-value (PFDR) in order to adjust for multiple tests. Results were considered significant if the FDR < 0.05 (Korthauer et al., 2019; Luo et al., 2023).
2.4.2 Sensitivity analysis
Cochran’s Q statistic (Greco et al., 2015) was employed to determine heterogeneity, with p < 0.05 indicating the presence of heterogeneity. MR-Egger intercept test and MR-PRESSO test can be used to determine if horizontal pleiotropy is present (Verbanck et al., 2018). In our study, when the MR-PRESSO global test indicated significant horizontal pleiotropy, we removed outliers with p < 0.05 and re-evaluated the remaining SNPs using the IVW analysis. And the MR-Egger regression intercept has a p-value >0.05, indicating no horizontal pleiotropy. Moreover, to evaluate if a single SNP was responsible for the causality in the two sample MR analysis, we performed a leave-one-out sensitivity analysis on the identified significant outcomes.
2.4.3 Reverse MR analysis
To investigate if MS, MG, and GBS, the three neuroimmune diseases, are caused by gut microbiota, we performed a reverse MR analysis utilizing their related SNPs as IVs. We applied MS, MG, and GBS as exposures and the microbiome identified in MR analysis as the outcomes and then employed sensitivity analysis to assess the reliability of the results. Furthermore, the MR Steiger directionality test (Hemani et al., 2017) was utilized to identify if there was a directional causality between the exposure and the outcome.
To explore the causal relationship between the gut microbiome and MS, MG, and GBS, the “Two-Sample MR” R package and “MR-PRESSO” R package were used to carry out a MR study (Yavorska and Burgess, 2017).
3 Results
3.1 SNP selection from gut microbiome
Among the 194 taxa included in our study, the SNPs that were identified for each microbial taxa are provided (Supplementary Table S1). Details for each SNP include the effect allele, the other allele, the beta, the standard error, the p-value, and the F-value. Our query of the SNPs on PhenoScanner did not reveal any SNPs associated with the confounders specified (Supplementary Table S2).
3.2 Causal effects of the gut microbiome on the three autoimmune neurologic disorders
3.2.1 MS
Results from the IVW-MR analysis indicated that four microbial taxa (genus Ruminococcaceae UCG003: OR: 0.824, 95% CI: 0.680–0.999, p = 0.048, PFDR = 0.048; genus Ruminiclostridium5: OR: 0.695, 95% CI: 0.554–0.871, p = 0.002, PFDR = 0.006; genus Anaerotruncus: OR: 0.726, 95% CI: 0.592–0.890, p = 0.002, PFDR = 0.006; order Burkholderiales: OR: 0.800, 95% CI: 0.653–0.981, p = 0.032, PFDR = 0.048) were found to have a negative correlation with MS. Two microbial taxa (genus Ruminococcus 2: OR: 1.213, 95% CI: 1.006–1.462, p = 0.043, PFDR = 0.048; genus Roseburia: OR: 1.255, 95% CI: 1.012–1.556, p = 0.038, PFDR = 0.048) were positively correlated with MS (Figure 2 and Table 2). All of them with p < 0.05 showed significant results. The MR-Egger analysis revealed a positive correlation between the genus Ruminococcaceae UCG003 (OR: 1.159, 95% CI: 0.601–2.235, p = 0.043, PFDR = 0.048) and MS, however, the result was not statistically significant as the p > 0.05.
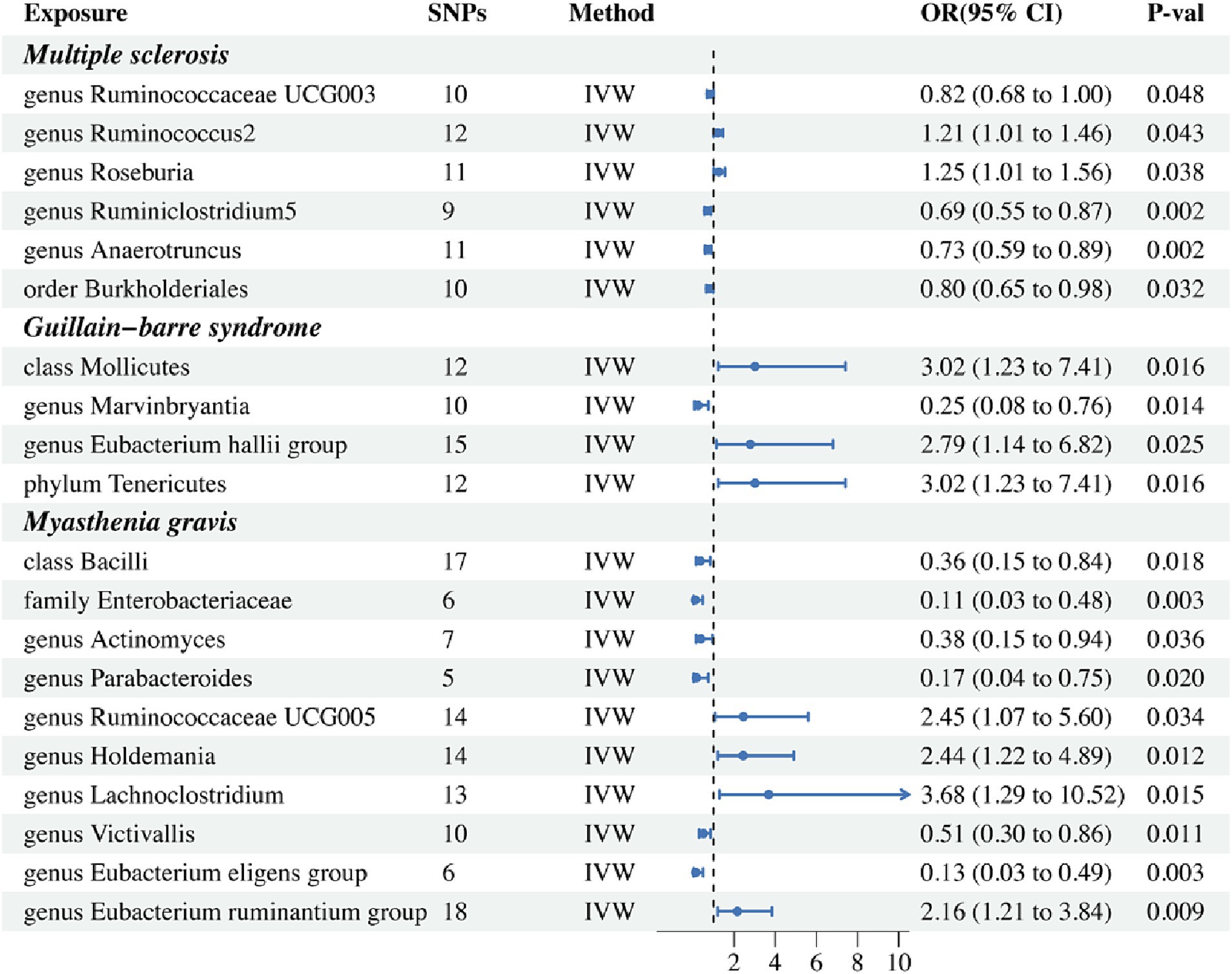
Figure 2. Forest plot of IVW analysis results of the effect of gut microbiome on MS, GBS, MG. IVW, Inverse variance weighted.
3.2.2 GBS
In addition, we conducted a causal analysis to determine if there is a correlation between gut microbiome and GBS. We found that in the IVW-MR method, there were three microbial taxa (class Mollicutes: OR: 3.016, 95% CI: 1.228–7.411, p = 0.016, PFDR = 0.021; genus Eubacterium (hallii group): OR: 2.787, 95% CI: 1.140–6.816, p = 0.025, PFDR = 0.025; phylum Tenericutes: OR: 3.016, 95% CI: 1.228–7.411, p = 0.016, PFDR = 0.021) which were positively correlated with GBS, and the genus Marvinbryantia (OR: 0.247, 95% CI: 0.081–0.755, p = 0.014, PFDR = 0.021) was inversely associated with GBS (Figure 2 and Table 2). The outcomes of the analysis were all significant. The MR-Egger method revealed a positive correlation between the genus Marvinbryantia (OR: 1.382, 95% CI: 0.016–121.625, p = 0.891, PFDR = 0.891) and GBS, though it was not considered significant.
3.2.3 MG
The IVW-MR analysis of the gut microbiome and MG, showed that six microbial taxa (class Bacilli: OR: 0.358, 95% CI: 0.153–0.838, p = 0.018, PFDR = 0.025; family Enterobacteriaceae: OR: 0.111, 95% CI: 0.026–0.477, p = 0.003, PFDR = 0.016; genus Actinomyces: OR: 0.377, 95% CI: 0.152–0.937, p = 0.036, PFDR = 0.036; genus Parabacteroides: OR: 0.166, 95% CI: 0.037–0.752, p = 0.020, PFDR = 0.025; genus Victivallis: OR: 0.509, 95% CI: 0.302–0.857, p = 0.011, PFDR = 0.024; genus Eubacterium (eligens group): OR: 0.130, 95% CI: 0.034–0.495, p = 0.003, PFDR = 0.016) were negatively correlated with MG; four microbial taxa (genus Ruminococcaceae UCG005: OR: 2.450, 95% CI: 1.072–5.598, p = 0.034, PFDR = 0.036; genus Holdemania: OR: 2.437, 95% CI: 1.215–4.888, p = 0.012, PFDR = 0.024; genus Lachnoclostridium: OR: 3.681, 95% CI: 1.288–10.521, p = 0.015, PFDR = 0.025; genus Eubacterium (ruminantium group): OR: 2.157, 95% CI: 1.211–3.843, p = 0.003, PFDR = 0.016) were positively associated with MG. The results of all microbial taxa were significant (p < 0.05) (Figure 2 and Table 2). Results from the MR-weighted median analysis indicated that four microbial taxa (genus Actinomyces: OR: 0.276, 95% CI: 0.092–0.827, p = 0.022, PFDR = 0.073; genus Holdemania: OR: 2.582, 95% CI: 1.021–6.529; genus Victivallis: OR: 0.397, 95% CI: 0.195–0.808, p = 0.045, PFDR = 0.092; genus Eubacterium (eligens group): OR: 0.110, 95% CI: 0.20–0.616, p = 0.012, PFDR = 0.06) had a significant impact. The results of the MR-Egger analysis for the genus Victivallis are contrary to those of IVW-MR, yet not statistically significant. All other MR-Egger analyses and MR estimates of the weighted median were in agreement with the IVW-MR analysis but had no significance (p > 0.05). The results of analyses of all gut microbiota and the three autoimmune neurologic disorders have been included in the Supplementary Table S3.
3.3 Sensitivity analyses
We employed Cochran’s Q test to detect heterogeneity and MR-PRESSO test and MR-Egger intercept to identify horizontal pleiotropy. The results of the test (p > 0.05) showed no evidence of heterogeneity or horizontal pleiotropy (Table 3). Moreover, four methods were implemented to measure the outcomes of MR analysis, and a scatter plot was formulated for the three diseases (Figure 3). Our main results were further substantiated through a leave-one-out analysis (Supplementary Figure S1).
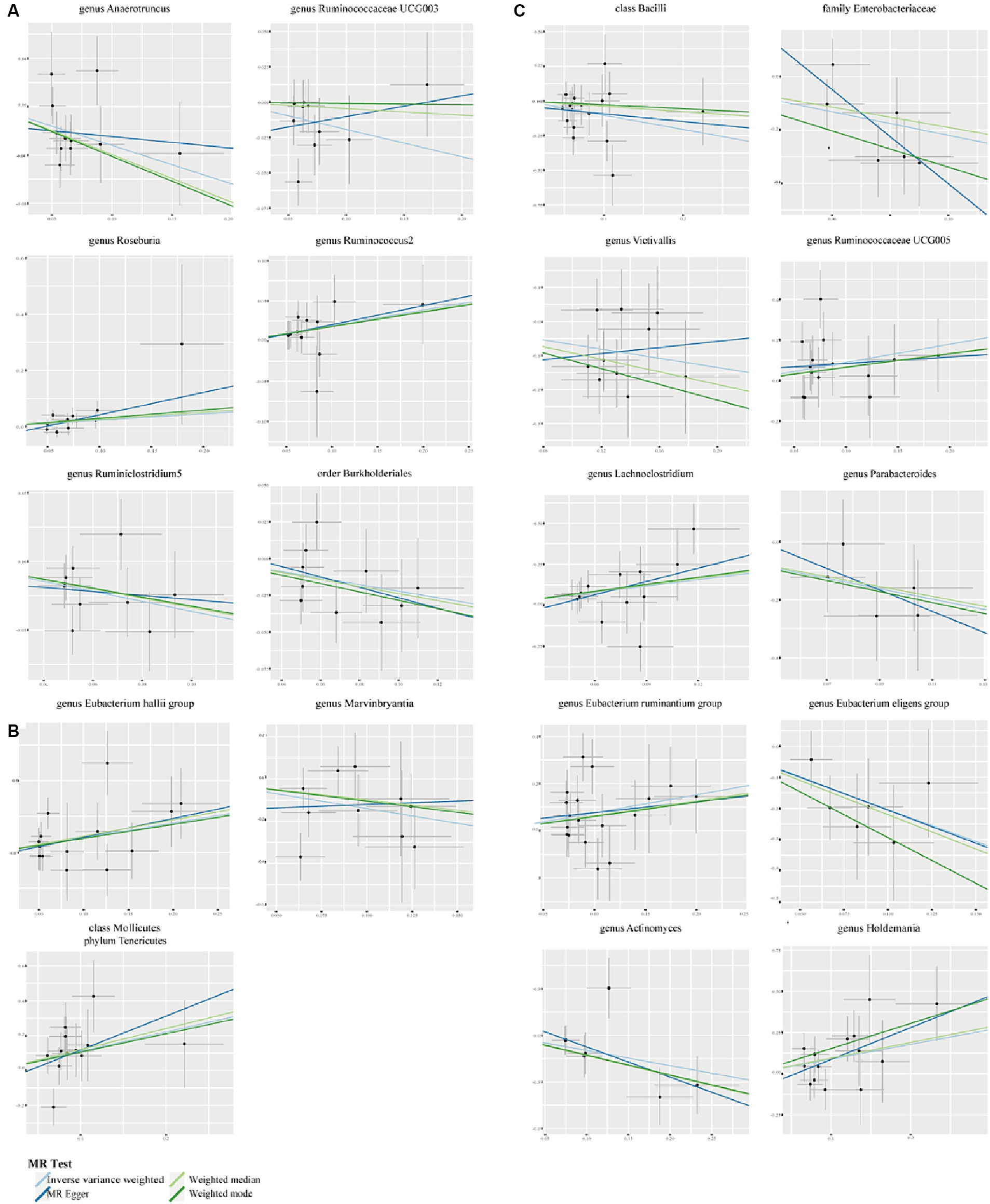
Figure 3. Scatter plots to show the associations of the gut microbiota against the three autoimmune neurologic disorders. (A) The effect of gut microbiota on MS. (B) The effect of gut microbiota on GBS. (C) The effect of gut microbiota on MG.
3.4 Reverse MR analysis
Taking into account cross-validation, we conducted reverse MR analyses for gut microbiota and three autoimmune neurologic disorders. The IVW-MR analysis findings indicated that there was no connection between the three autoimmune neurologic disorders, and the identified bacterial characteristics in a reverse causality fashion. The results of Cochran’s Q test indicated that there was no meaningful discrepancy among the incorporated independent variables. The MR-Egger intercept did not reveal any substantial horizontal pleiotropy (Table 4). Weighted Modes, Weighted Median Estimator, and MR-Egger results are presented in Supplementary Table S4.
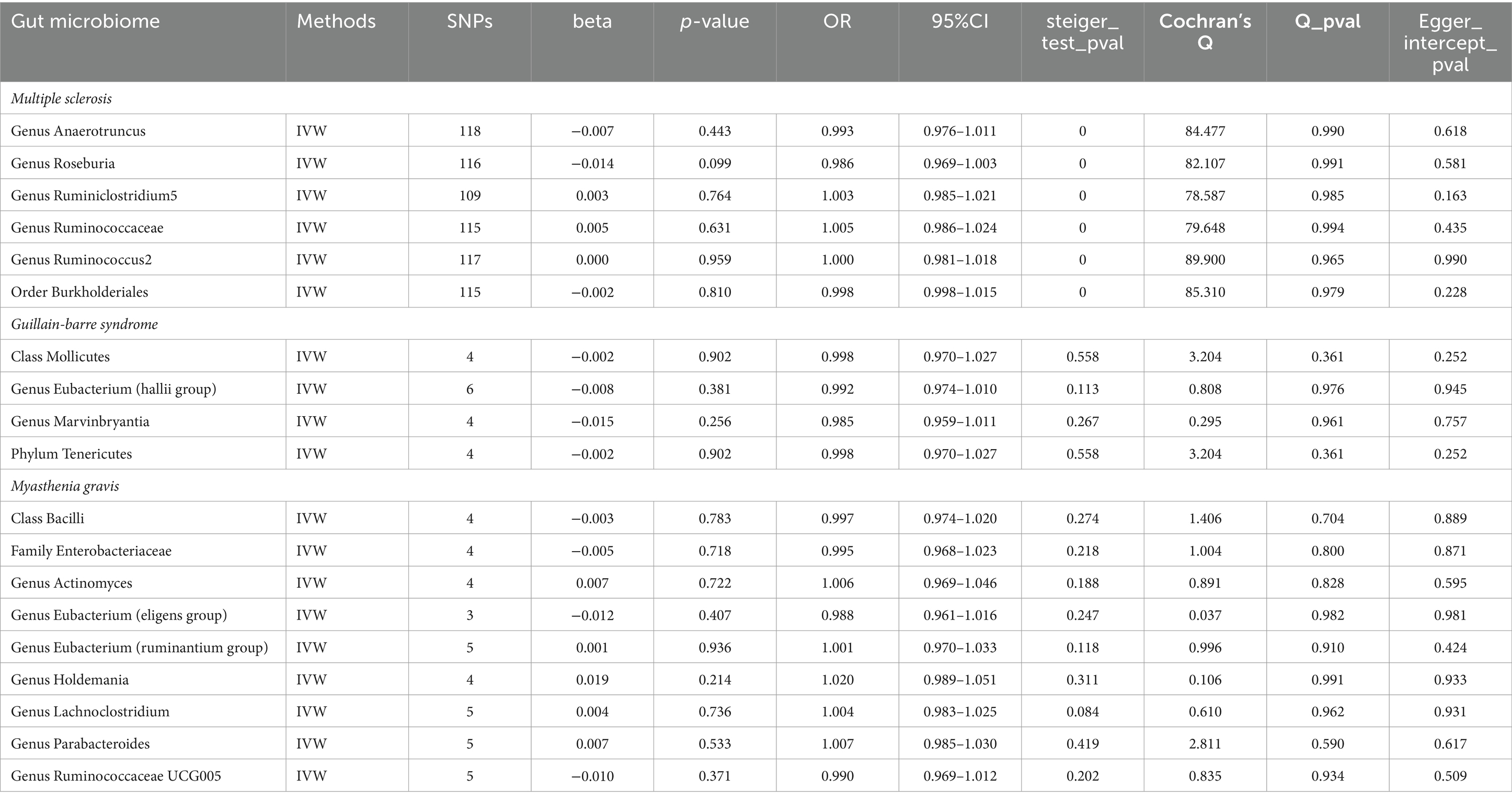
Table 4. Reverse MR results of causal links between the three autoimmune neurologic disorders and gut microbiome.
4 Discussion
As far as we know, this is the first study to use published GWAS summary statistics to perform a two-sample MR analysis to explore the causal relationship between gut microbiota and autoimmune neurologic disorders. Through our analysis, we revealed causal relationships between certain microbial taxa and three common autoimmune neurologic disorders: MS, MG, and GBS.
Our research revealed that MS is associated with an increased presence of the genera Ruminococcus2 and Roseburia. Study has shown that individuals with a higher abundance of Ruminococcus may be more likely to suffer from demyelinating optic neuritis, implying its involvement in autoimmune illnesses (Liu et al., 2023). Moreover, alterations in the abundance of Ruminococcus and Roseburia have been reported to be closely associated with the progression of Systemic Lupus Erythematosus (a typical autoimmune disease) (Vieira et al., 2021). On the other hand, we observed that a lower risk of MS was associated with higher levels of the genus Ruminococcaceae UCG003, the genus Ruminiclostridium5, the genus Anaerotruncus, and the order Burkholderiales. The genus Ruminococcaceae can increase the production of short-chain fatty acids (SCFAs), including acetic, propionic, and butyric acids, while reducing the release of inflammatory factors (Wang et al., 2023). Additionally, Ruminiclostridium5 and Anaerotruncus have been linked to CD8+ and CD4+ T cells, and their abundance is negatively related to the levels of inflammatory TNF-α, IL-1β, and IL-6 (Mao et al., 2023; Singh et al., 2023).
In the causal relationship between gut microbiota and GBS, we revealed that an increased risk of GBS was linked to a greater abundance of the phylum Tenericutes, class Mollicutes, and genus Eubacterium (hallii group). Conversely, higher levels of the genus Marvinbryantia were associated with a lower risk of GBS. Mycoplasma, belonging to the phylum Tenericutes and class Mollicutes, is a widely known type of bacteria that causes mycoplasma pneumonia (Kuwahara et al., 2017; Gaspari et al., 2021). Previous research has established a strong correlation between Mycoplasma and the emergence of GBS, with many patients displaying a history of a mycoplasma infection before the onset of GBS. Our research has identified the genus Marvinbryantia in relation to GBS that has not been noted in prior studies. However, the exact mechanism of these bacteria leading to GBS remains poorly understood and warrants further study.
We discovered that the abundance of the genera Ruminococcaceae UCG005, Holdemania, Eubacterium (ruminantium group), and Lachnoclostridium was associated with an increased risk of MG, while a high abundance of the class Bacilli, the family Enterobacteriaceae, and the genera Parabacteroides, Victivallis, and Eubacterium (eligens group) was related to a lower risk of MG. Qiu et al. observed that the genera Clostridium, Lactobacillus, and Eubacterium had significantly diminished in healthy individuals, with only one-third remaining (Qiu et al., 2018). A decrease in the concentration of Clostridium in the body leads to a decrease in the production of SCFAs, such as butyric acid and propionic acid, which affects the immune system. Studies have indicated that lactobacilli of the Bacilli class can stimulate the production of anti-inflammatory factors such as TGF-β, IL-10, and arginase 1 by regulating the dendritic cells (Rinaldi et al., 2019). Moreover, these same lactobacilli have been found to suppress AChR-reactive lymphocyte proliferation, anti-AChR-reactive IgG levels, and pro-inflammatory cytokines such as IL-6, IL-17, IFN-γ, and TNF-α (Chae et al., 2012; Teame et al., 2020). The Enterobacteriaceae, belonging to the phylum Proteobacteria, influences the production of propionic acid, thus impacting the immune response (Laursen, 2021). Our study has highlighted the significance of the genus Holdemania and genus Parabacteroides in the risk of MG development, a subject that has not been extensively discussed in earlier research.
The gut is an impressive network of nerve cells, with more than 100 million, second only to the central nervous system. The microbiota-gut-brain axis is a communication path between the gut and the central nervous system, which encompasses the central nervous system, the hypothalamic–pituitary–adrenal axis, the neuroimmune system, the autonomic sympathetic, the enteric and vagus nerves, and the intestinal microbiota and its metabolites (Cryan et al., 2019). SCFAs, the most studied microbial metabolites, have neuroactive properties that could be related to the communication along the microbiota-gut-brain axis, either directly or indirectly (Dalile et al., 2019; Zhang et al., 2023). It is interesting to note that certain SCFA-producing bacteria, such as Ruminococcaceae UCG003, Ruminococcus2, Roseburia, Ruminiclostridium5, Lachnoclostridium, and Eubacterium, are linked to autoimmune neurologic disorders. SCFAs are reported to play an anti-inflammatory role in the parenchymal mucosa, increase the number of Tregs, inhibit the differentiation of Th17 cells, and reduce the expression of inflammatory cytokines such as IL-17, IL-21, and IL-22 (Diller et al., 2016; Scott, 2021). A decrease in SCFA levels has been linked to autoimmune and inflammatory diseases, including rheumatoid arthritis, MS, colitis, type 1 diabetes, and rheumatoid arthritis (Schiweck et al., 2022). Although probiotics have been proven to reduce the symptoms of neuroimmune diseases and enhance functional performance, the exact ways in which they protect against these diseases are yet to be determined. Future studies should adopt a comprehensive approach, incorporating multi-omics analysis, to investigate the interplay between SCFAs, gut microbiota, and neuroimmune diseases, thus enhancing our understanding of the onset and development of these diseases.
This study has several advantages. By using the MR approach, we were able to reduce the potential for confounding factors that are often seen in epidemiological studies. Additionally, we sourced genetic variants from the most comprehensive GWAS meta-analysis for human gut microbiota composition, providing the accuracy of our MR analysis. Moreover, we covered three common autoimmune neurologic disorders. Finally, the causal associations identified in our MR analysis can be used to target particular gut bacteria for the prevention and treatment of autoimmune neurologic disorders.
Nevertheless, our study has certain limitations. Firstly, to reduce the risk of error due to mixed populations, we only used sample data from European ancestry, which limits the generality of the findings to all populations. Therefore, it is important for future studies to incorporate a more diverse range of populations in order to enhance the inclusivity of our conclusions. Secondly, the screening method may cause the exclusion of many SNPs of the gut microbiota at the IV selection stage, potentially preventing the discovery of crucial results. Thirdly, the pathogenesis of the specific gut microbiota in autoimmune neurologic disorders is still unclear, and more clinical and functional studies are still needed in the future to verify the accuracy of the results.
In conclusion, this is the first comprehensive assessment of the potential bidirectional relationship between gut microbiota and autoimmune neurologic disorders. However, further studies are needed to understand how gut microbiota affect autoimmune neurologic disorders and its precise biological mechanism.
Data availability statement
The raw data supporting the conclusions of this article will be made available by the authors, without undue reservation.
Author contributions
MZ: Formal analysis, Methodology, Writing – original draft. JF: Formal analysis, Methodology, Writing – original draft. CZ: Formal analysis, Writing – review & editing. QL: Funding acquisition, Methodology, Writing – review & editing. JZ: Methodology, Validation, Writing – review & editing.
Funding
The author(s) declare financial support was received for the research, authorship, and/or publication of this article. This study was supported by research grants from Natural Science Foundation of Fujian Province (Grant Nos. 2022J011358 and 2022J011365), Medical and Health Key Project of Xiamen (Grant No. 3502Z20204006), and Xiamen Municipal Health Commission, Xiamen Municipal Bureau of Science and Technology (Grant No. 3502Z20209005).
Conflict of interest
The authors declare that the research was conducted in the absence of any commercial or financial relationships that could be construed as a potential conflict of interest.
Publisher’s note
All claims expressed in this article are solely those of the authors and do not necessarily represent those of their affiliated organizations, or those of the publisher, the editors and the reviewers. Any product that may be evaluated in this article, or claim that may be made by its manufacturer, is not guaranteed or endorsed by the publisher.
Supplementary material
The Supplementary material for this article can be found online at: https://www.frontiersin.org/articles/10.3389/fmicb.2024.1337632/full#supplementary-material
Footnotes
References
Allos, B. M. (1997). Association between Campylobacter infection and Guillain-Barré syndrome. J. Infect. Dis. 176, S125–S128. doi: 10.1086/513783
Bowden, J., Davey Smith, G., Haycock, P. C., and Burgess, S. (2016). Consistent estimation in Mendelian randomization with some invalid instruments using a weighted median estimator. Genet. Epidemiol. 40, 304–314. doi: 10.1002/gepi.21965
Bowden, J., and Holmes, M. V. (2019). Meta-analysis and Mendelian randomization: a review. Res. Synth. Methods 10, 486–496. doi: 10.1002/jrsm.1346
Burgess, S., Butterworth, A., and Thompson, S. G. (2013). Mendelian randomization analysis with multiple genetic variants using summarized data. Genet. Epidemiol. 37, 658–665. doi: 10.1002/gepi.21758
Cekanaviciute, E., Yoo, B. B., Runia, T. F., Debelius, J. W., Singh, S., Nelson, C. A., et al. (2017). Gut bacteria from multiple sclerosis patients modulate human T cells and exacerbate symptoms in mouse models. Proc. Natl. Acad. Sci. U. S. A. 114, 10713–10718. doi: 10.1073/pnas.1711235114
Chae, C. S., Kwon, H. K., Hwang, J. S., Kim, J. E., and Im, S. H. (2012). Prophylactic effect of probiotics on the development of experimental autoimmune myasthenia gravis. PLoS One 7:e52119. doi: 10.1371/journal.pone.0052119
Cryan, J. F., O’Riordan, K. J., Cowan, C. S. M., Sandhu, K. V., Bastiaanssen, T. F. S., Boehme, M., et al. (2019). The microbiota-gut-brain axis. Physiol. Rev. 99, 1877–2013. doi: 10.1152/physrev.00018.2018
Dalile, B., Van Oudenhove, L., Vervliet, B., and Verbeke, K. (2019). The role of short-chain fatty acids in microbiota-gut-brain communication. Nat. Rev. Gastroenterol. Hepatol. 16, 461–478. doi: 10.1038/s41575-019-0157-3
Davey Smith, G., and Hemani, G. (2014). Mendelian randomization: genetic anchors for causal inference in epidemiological studies. Hum. Mol. Genet. 23, R89–R98. doi: 10.1093/hmg/ddu328
Diaz-Marugan, L., Kantsjö, J. B., Rutsch, A., and Ronchi, F. (2023). Microbiota, diet, and the gut-brain axis in multiple sclerosis and stroke. Eur. J. Immunol. 53:e2250229. doi: 10.1002/eji.202250229
Diller, M. L., Kudchadkar, R. R., Delman, K. A., Lawson, D. H., and Ford, M. L. (2016). Balancing inflammation: the link between Th17 and regulatory T cells. Mediat. Inflamm. 2016, 1–8. doi: 10.1155/2016/6309219
Gaspari, E., Koehorst, J. J., Frey, J., Martins Dos Santos, V. A. P., and Suarez-Diez, M. (2021). Galactocerebroside biosynthesis pathways of Mycoplasma species: an antigen triggering Guillain-Barré-Stohl syndrome. Microb. Biotechnol. 14, 1201–1211. doi: 10.1111/1751-7915.13794
Greco, M. F. D., Minelli, C., Sheehan, N. A., and Thompson, J. R. (2015). Detecting pleiotropy in Mendelian randomisation studies with summary data and a continuous outcome. Stat. Med. 34, 2926–2940. doi: 10.1002/sim.6522
Hartwig, F. P., Davey Smith, G., and Bowden, J. (2017). Robust inference in summary data Mendelian randomization via the zero modal pleiotropy assumption. Int. J. Epidemiol. 46, 1985–1998. doi: 10.1093/ije/dyx102
Hemani, G., Tilling, K., and Davey, S. G. (2017). Orienting the causal relationship between imprecisely measured traits using GWAS summary data. PLoS Genet. 13:e1007081. doi: 10.1371/journal.pgen.1007081
International Multiple Sclerosis Genetics Consortium (2019). Multiple sclerosis genomic map implicates peripheral immune cells and microglia in susceptibility. Science 365:eaav7188. doi: 10.1126/science.aav7188
Kapoor, B., Gulati, M., Gupta, R., and Singla, R. K. (2023). Microbiota dysbiosis and myasthenia gravis: Do all roads lead to Rome? Autoimmun. Rev. 22:103313. doi: 10.1016/j.autrev.2023.103313
Korthauer, K., Kimes, P. K., Duvallet, C., Reyes, A., Subramanian, A., Teng, M., et al. (2019). A practical guide to methods controlling false discoveries in computational biology. Genome Biol. 20:118. doi: 10.1186/s13059-019-1716-1
Kurilshikov, A., Medina-Gomez, C., Bacigalupe, R., Radjabzadeh, D., Wang, J., Demirkan, A., et al. (2021). Large-scale association analyses identify host factors influencing human gut microbiome composition. Nat. Genet. 53, 156–165. doi: 10.1038/s41588-020-00763-1
Kuwahara, M., Samukawa, M., Ikeda, T., Morikawa, M., Ueno, R., Hamada, Y., et al. (2017). Characterization of the neurological diseases associated with Mycoplasma pneumoniae infection and anti-glycolipid antibodies. J. Neurol. 264, 467–475. doi: 10.1007/s00415-016-8371-1
Laursen, M. F. (2021). Gut microbiota development: influence of diet from infancy to toddlerhood. Ann. Nutr. Metab. 77, 21–34. doi: 10.1159/000517912
Li, P., Wang, H., Guo, L., Gou, X., Chen, G., Lin, D., et al. (2022). Association between gut microbiota and preeclampsia-eclampsia: a two-sample Mendelian randomization study. BMC Med. 20:443. doi: 10.1186/s12916-022-02657-x
Liu, Y., Fan, H., Shao, Y., Zhang, J., Zuo, Z., Wang, J., et al. (2023). Gut microbiota dysbiosis associated with different types of demyelinating optic neuritis in patients. Mult. Scler. Relat. Disord. 72:104619. doi: 10.1016/j.msard.2023.104619
Lozupone, C. A., Stombaugh, J. I., Gordon, J. I., Jansson, J. K., and Knight, R. (2012). Diversity, stability and resilience of the human gut microbiota. Nature 489, 220–230. doi: 10.1038/nature11550
Luo, M., Sun, M., Wang, T., Zhang, S., Song, X., Liu, X., et al. (2023). Gut microbiota and type 1 diabetes: a two-sample bidirectional Mendelian randomization study. Front. Cell. Infect. Microbiol. 13:1163898. doi: 10.3389/fcimb.2023.1163898
Mao, B., Guo, W., Liu, X., Cui, S., Zhang, Q., Zhao, J., et al. (2023). Potential probiotic properties of Blautia producta against lipopolysaccharide-induced acute liver injury. Probiotics Antimicrob Proteins 15, 785–796. doi: 10.1007/s12602-023-10044-y
Nunez, N., Réot, L., and Menu, E. (2021). Neonatal immune system ontogeny: the role of maternal microbiota and associated factors. How might the non-human primate model enlighten the path? Vaccines 9:584. doi: 10.3390/vaccines9060584
Qiu, D., Xia, Z., Jiao, X., Deng, J., Zhang, L., and Li, J. (2018). Altered gut microbiota in myasthenia gravis. Front. Microbiol. 9:2627. doi: 10.3389/fmicb.2018.02627
Rinaldi, E., Consonni, A., Cordiglieri, C., Sacco, G., Crasà, C., Fontana, A., et al. (2019). Therapeutic effect of Bifidobacterium administration on experimental autoimmune myasthenia gravis in Lewis rats. Front. Immunol. 10:2949. doi: 10.3389/fimmu.2019.02949
Rubin, D. B., Batra, A., Vaitkevicius, H., and Vodopivec, I. (2018). Autoimmune neurologic disorders. Am. J. Med. 131, 226–236. doi: 10.1016/j.amjmed.2017.10.033
Schiweck, C., Edwin Thanarajah, S., Aichholzer, M., Matura, S., Reif, A., Vrieze, E., et al. (2022). Regulation of CD4+ and CD8+ T cell biology by short-chain fatty acids and its relevance for autoimmune pathology. Int. J. Mol. Sci. 23:8272. doi: 10.3390/ijms23158272
Scott, D. W. (2021). Driving CARs to BARs: the winding road to specific regulatory T cells for tolerance. Front. Immunol. 12:742719. doi: 10.3389/fimmu.2021.742719
Singh, A., Ashar, H., Butcher, J. T., and Ranjan, A. (2023). Age-associated changes in the gut microbiome impact efficacy of tumor immunomodulatory treatments. Exp. Gerontol. 181:112268. doi: 10.1016/j.exger.2023.112268
Teame, T., Wang, A., Xie, M., Zhang, Z., Yang, Y., Ding, Q., et al. (2020). Paraprobiotics and postbiotics of probiotic lactobacilli, their positive effects on the host and action mechanisms: a review. Front. Nutr. 7:570344. doi: 10.3389/fnut.2020.570344
Vass, K. (2012). Current immune therapies of autoimmune disease of the nervous system with special emphasis to multiple sclerosis. Curr. Pharm. Des. 18, 4513–4517. doi: 10.2174/138161212802502288
Verbanck, M., Chen, C. Y., Neale, B., and Do, R. (2018). Detection of widespread horizontal pleiotropy in causal relationships inferred from Mendelian randomization between complex traits and diseases. Nat. Genet. 50, 693–698. doi: 10.1038/s41588-018-0099-7
Vieira, J. R. P., Rezende, A. T. O., Fernandes, M. R., and Da Silva, N. A. (2021). Intestinal microbiota and active systemic lupus erythematosus: a systematic review. Adv. Rheumatol. 61:42. doi: 10.1186/s42358-021-00201-8
Wang, Q., Lu, Q., Jia, S., and Zhao, M. (2023). Gut immune microenvironment and autoimmunity. Int. Immunopharmacol. 124:110842. doi: 10.1016/j.intimp.2023.110842
Wang, J., Ren, C., Jin, L., and Batu, W. (2023). Seabuckthorn Wuwei Pulvis attenuates chronic obstructive pulmonary disease in rat through gut microbiota-short chain fatty acids axis. J. Ethnopharmacol. 314:116591. doi: 10.1016/j.jep.2023.116591
Yavorska, O. O., and Burgess, S. (2017). MendelianRandomization: an R package for performing Mendelian randomization analyses using summarized data. Int. J. Epidemiol. 46, 1734–1739. doi: 10.1093/ije/dyx034
Zhang, D., Jian, Y. P., Zhang, Y. N., Li, Y., Gu, L. T., Sun, H. H., et al. (2023). Short-chain fatty acids in diseases. Cell Commun. Signal 21:212. doi: 10.1186/s12964-023-01219-9
Keywords: gut microbiota, Myasthenia Gravis, Multiple Sclerosis, Guillain-Barré Syndrome, Mendelian Randomization, causal relationship
Citation: Zhang M, Fang J, Zheng C, Lin Q and Zhang J (2024) Gut microbiota and autoimmune neurologic disorders: a two-sample bidirectional Mendelian randomization study. Front. Microbiol. 15:1337632. doi: 10.3389/fmicb.2024.1337632
Edited by:
Santos Garcia, Universidad Autónoma de Nuevo León, MexicoReviewed by:
Norma Heredia, Autonomous University of Nuevo León, MexicoReza Rastmanesh, American Physical Society, United States
Qing Zeng, Southern Medical University, China
Copyright © 2024 Zhang, Fang, Zheng, Lin and Zhang. This is an open-access article distributed under the terms of the Creative Commons Attribution License (CC BY). The use, distribution or reproduction in other forums is permitted, provided the original author(s) and the copyright owner(s) are credited and that the original publication in this journal is cited, in accordance with accepted academic practice. No use, distribution or reproduction is permitted which does not comply with these terms.
*Correspondence: Qing Lin, linqing2005602@aliyun.com; Jiawei Zhang, zhangjiawei8102@163.com
†These authors have contributed equally to this work