- 1Translational Health Science and Technology Institute, NCR Biotech Science Cluster, Faridabad, India
- 2Jawaharlal Nehru University, Delhi, India
Emerging antimicrobial resistance (AMR) among Gram-positive pathogens, specifically in Staphylococcus aureus (S. aureus), is becoming a leading public health concern demanding effective therapeutics. Metabolite modulation can improve the efficacy of existing antibiotics and facilitate the development of effective therapeutics. However, it remained unexplored for drug-resistant S. aureus (gentamicin and methicillin-resistant), primarily due to the dearth of optimal metabolite extraction protocols including a protocol for AMR-associated metabolites. Therefore, in this investigation, we have compared the performance of the two most widely used methods, i.e., freeze-thaw cycle (FTC) and sonication cycle (SC), alone and in combination (FTC + SC), and identified the optimal method for this purpose. A total of 116, 119, and 99 metabolites were identified using the FTC, SC, and FTC + SC methods, respectively, leading to the identification of 163 metabolites cumulatively. Out of 163, 69 metabolites were found to be associated with AMR in published literature consisting of the highest number of metabolites identified by FTC (57) followed by SC (54) and FTC + SC (40). Thus, the performances of FTC and SC methods were comparable with no additional benefits of combining both. Moreover, each method showed biasness toward specific metabolite(s) or class of metabolites, suggesting that the choice of metabolite extraction method shall be decided based on the metabolites of interest in the investigation.
1. Introduction
Antimicrobial resistance (AMR) among bacterial pathogens has become a leading cause of morbidity and mortality and hence a global public health concern demanding immediate action to develop strategies to combat such antimicrobial resistant difficult-to-treat bacterial infections (Dhingra et al., 2020). This recent trend indicates the emerging prevalence of Multidrug-resistant (MDR) is not only among Gram-negative (i.e., Klebsiella pneumoniae, Acinetobacter baumannii, and Pseudomonas aeruginosa, etc.) but also Gram-positive bacterial pathogens [primarily methicillin and gentamicin-resistant Staphylococcus aureus (MRSA)] making them also difficult to treat (Bassetti et al., 2019; Mulani et al., 2019). Tremendous efforts are being made to understand the pathogen and disease biology and develop relevant diagnostics and therapeutics for the effective treatment of Gram-negative pathogens; however, the same has been comparatively limited for Gram-positive pathogens (Vazquez-Guillamet and Kollef, 2014; Breijyeh et al., 2020).
World Health Organization (WHO) and the centers for disease control and prevention (CDC) have placed MRSA under a list of serious threat causing drug-resistant pathogens (WHO, 2021). MRSA alone has been responsible for more than 100,000 deaths worldwide in 2019 globally (Oxford, U.o, 2019). In India, the prevalence of MRSA has been recorded to be around 30–70% with high mortality rates among patients developing MRSA bacteremia (Antimicrobial Resistance, C, 2022). Originally, MRSA was common in the healthcare setting contributing to nosocomial/hospital-acquired (HA-MRSA) infections like those associated with surgical procedures, indwelling catheters, or prosthetic devices (Mehta et al., 2020). However, over the last decade, there has been an upsurge of community-associated MRSA (CA-MRSA) infections also like bone, joint and skin infections (Masimen et al., 2022). These HA- and CA-S. aureus infections are spread through direct contact with an infected wound or contaminated hands and can be fatal if remains untreated (Masimen et al., 2022). Further, limited therapeutic options and an increasing rate of emergence of AMR even against the available last-resort antibiotics have worsened the problem. Altogether, S. aureus infection is associated with a greater occurrence of complications, longer hospital stays, duration of therapy, as well as higher costs of treatment (Lee et al., 2018).
Therefore, understanding the molecular changes driving antibiotic resistance among Gram-positive bacteria, specifically S. aureus, has become critically important. This can be achieved using various new-generation biological tools, such as genomic, transcriptomic, proteomic, and metabolomics. However, metabolomics allows the identification and quantification of metabolites that are the end product of any genomic- and proteomic-based biological activity of an organism at a given point of time and hence provide a characteristic chemical fingerprint of a specific cellular process (Xiao et al., 2012). Recently, studies have shown that modulation of the pathogen’s metabolome can be used to deal with the emerging problem of AMR. One recent example is the conjugating antibiotics with small metabolites like aminoglycosides with fructose and fumarate, resulting in increased potency of aminoglycosides against S. aureus and Escherichia coli (Rosenberg et al., 2020). A central metabolite of the energy-generation pathway, pyruvate, has been reported to be associated with the virulence and pathogenicity of S. aureus, indicating it as a potential target for controlling infection (Harper et al., 2018). Further exogenous administration of L-valine, L-leucine, L-isoleucine, and L-proline in S. aureus bloodstream infection animal models has also been shown to have anti-infective effects (Pang et al., 2020). A decrease in intracellular ATP levels has been linked to the development of the S. aureus persister phenotype making them resistant to antibiotics (Conlon et al., 2016). However, existing knowledge of target(s) for regulating metabolomic modulation to treat resistant infections is still limited, owing to limited attempts to investigate the comprehensive metabolome of S. aureus (Kumar et al., 2022). This may potentially be due to the unavailability of the appropriate protocols and pipeline for investigating the metabolome of the Gram-positive bacteria, S. aureus (specifically drug-resistant strains), including the very first step of extracting AMR-associated metabolites.
Therefore, the goal of this study was to determine the optimal method for extraction of AMR-associated metabolites from S. aureus because the method of metabolite extraction dictates the possible range of metabolites that can be detected in the sample and thus has a large impact on the potential outcome(s) of the metabolomic studies. Most of the metabolomic investigations employ FTC or SC-based methods for extracting bacterial metabolites; however, their efficacy for extraction of AMR-associated metabolite from Gram-positive bacteria has not been investigated yet (Conlon et al., 2016). In this study, we have employed both methods, i.e., FTC and SC individually as well as their combination FTC followed by SC (FTC + SC) to extract the metabolites from the S. aureus pathogen. Further, Electrospray Ionization-Liquid Chromatography-Mass Spectrometry/Mass Spectrometry (ESI-LC–MS/MS; Orbitrap Fusion Tribrid, Thermo-Scientific), a highly sensitive and advanced mass-spectrometer was used to potentially increase the metabolomic coverage. Finally, the list of identified metabolites was subjected to an intensive literature search for finding the potential association of identified metabolites with AMR. Altogether, this information was utilized to identify the optimal metabolite extraction method for investigating AMR-associated metabolites.
2. Materials and methods
2.1. Harvesting of Staphylococcus aureus cell pellets
The primary culture of S. aureus (ATCC®33592) was inoculated in 5 ml Mueller Hinton Broth (MHB), followed by incubation for approximately 16 h at 37°C at 220 rpm. The secondary culture was inoculated (1% from primary culture) in fresh MHB and incubated till the exponential growth phase (Optical density, OD600nm:~0.6–0.7, i.e., 4 h post-inoculation) was achieved. Approximately 107 cells (OD-based measurement) were harvested using centrifugation and washing with LC–MS grade cold water and kept at −80°C. The cell pellets were generated for 6 independent biological replicates. Since the cellular architecture and composition of member pathogens among a species are highly expected to be similar, a single strain of S. aureus was used for the study.
2.2. Bacterial metabolism quenching and extraction
For metabolite extraction, the stored cell pellet was thawed for 10 min on ice, followed by resuspension in 500 μl of mass spectrometry (MS) grade chilled methanol (Sigma). To evaluate the metabolites extraction efficiency of three extraction methods, i.e., FTC, SC, FTC + SC, 13C-labeled L-valine (40 ng/ml), an internal standard, was added to each sample before metabolite extraction (Kumar et al., 2022). For the freeze-thaw cycle-based metabolite extraction method, the resuspended cells were subjected to repeated freeze-thaw cycles (10 min at −80°C followed by 10 min on ice) three times. For the sonication-based metabolite extraction method, the mixture was sonicated for 2 min at 35 A° (10-s on-and-off cycles). Similarly, for FTC + SC, the resuspended cell pellet was subjected to a freeze-thaw cycle three times and followed by a sonication cycle as described above. The sample mixture following FTC, SC, or FTC + SC methods was collected as supernatant after centrifugation at 12,500 RPM for 10 min (4°C), aliquoted and stored at −80°C till further analysis. The metabolites were extracted using each method (FTC, SC and FTC + SC) from 6 independent biological replicate samples.
2.3. Separation and quantitation of metabolites using ESI-LC–MS/MS
The standard workflow for metabolite separation and measurement using ESI-LC–MS/MS was as followed. An aliquot (100 μl) of the sample was vacuum dried and the pellet was resuspended in 3:17, methanol: water mixture (25ul), followed by vortexing for 15 min, and 10 min centrifugation at 11000 rpm at 4°C. Data acquisition was done on C18 Reversed Phase HPLC Columns (C18) and Hydrophilic interaction LC (HILIC) columns with positive and negative mode separately. The collected metabolites were separated using C18 (HSS T3) and HILIC (XBridge BEH Amide) columns on UPLC ultimate 3,000 maintained at 40°C and 35°C temperature, respectively. A gradient of mobile phase A consisting of water +0.1% formic acid and mobile phase B (methanol + 0.1% formic acid) was used as a mobile phase for the C18 column. For the HILIC column, 20 mM ammonium acetate in the water of pH 9.0 (mobile phase A) and 100% acetonitrile (mobile phase B) were used for separation. For separation in the reverse phase, a gradient of 1% mobile phase B to 99% mobile phase B over 14 min (flow rate of 0.3 ml/min) was set and for the HILIC column, 85% mobile phase B to 10% B over 16 min (flow rate of 0.35 ml/min) was used. 5ul sample was injected into the column and for data acquisition. The Orbitrap Fusion Tribrid Mass Spectrometer (Thermo-Scientific) equipped with heated electrospray ionization (HESI) source was used for processing the sample using the following settings: 4000 positive mode spray voltage, 35,000 V for negative mode, 60–900 m/z mass range, AGC (Automatic gain control) was targeted at 100,000 ions. For data acquisition, 120,000 resolutions in MS1 mode and 30,000 resolutions in data-dependent MS2 scan mode were used. For MS, 50 ms was used as the maximum injection time while for MS/MS, an AGC target of 20,000 ions and a maximum injection time of 60 ms was used.
2.4. Metabolites identification and data analysis
The untargeted workflow of Progenesis QI software for metabolomics from Water Corporation was used at default settings for acquiring the data and its analysis. The MetaScope plugin of Progenesis QI metabolites was used for matching the mass, fragmented ions pattern and retention time of identified compounds with a list of 950 metabolites from our in-house library followed by an online spectral library search to confirm the identified metabolites. Peak detected in ≥4 replicates (out of 6 independent biological replicates) with intensity ≥100 (confidence interval->95%) receives an identification by spectral match. Further, ±1 min and 5 ppm retention time error for MS and MS/MS with fragmentation pattern match were also considered as a criterion for identifying metabolites (Supplementary Table 1).
For data analysis, the list of identified metabolites in HILIC and C18 (both positive and negative mode) was combined, and duplicates were removed (Supplementary Table 2). MetaboAnalyst 5.0 was used for statistical and functional analysis like principal component analysis (PCA) and heat map analysis. Outlier intensities of metabolite(s) were excluded and the data were loaded in a matrix and statistical analysis was performed using peak intensities thereby using a statistical filter of interquartile range. Sample normalization was performed using “normalization by sum” methods followed by data transformation using log transformation (base 10) thereby scaling the data using Pareto scaling (mean-centered and divided by the square root of the standard deviation of each variable) (shown in MetaboAnalyst report, i.e., Supplementary Data sheet 3). Univariate analysis was performed using one-way analysis of variance (ANOVA) and post-hoc tests using a value of p (FDR) cutoff of ≤0.05. 2-D PCA was performed displaying 95% confidence regions to observe the inner clusters and find the apparent outliers. Hierarchical clustering heat maps were prepared using t-test/one-way ANOVA and Euclidean distance measure and Ward clustering method.
For quantitation, the peak intensity average of replicates for each metabolite extraction method (SC, FTC, and FTC + SC) was considered and used to identify differences among the extraction method. Chemical class-based analysis and functional categorization of metabolites were done using Metabolomics Workbench.1
2.5. Identification of AMR-associated metabolites
A majority of antibiotics work by targeting cellular processes like (1) cell wall synthesis, (2) cell metabolism, (3) nucleotide biosynthesis and/or (4) protein synthesis. So, it is evident that establishing and identifying metabolites associated with these processes can help us in understanding the metabolic fingerprint and mechanism of antibiotic resistance. Therefore, a literature search to identify the role of each metabolite in nucleotide synthesis, protein synthesis, cell wall biosynthesis, cell proliferation (potential representative of resistant phenotypes as they can grow even in presence of selective pressure, i.e., antibiotics) and cell death (representative drug sensitive bacterial population) was performed using Google Scholar and PubMed and the resulting information was utilized to fetch the AMR-associated metabolites and followed by data interpretation.
3. Results
3.1. Identification of metabolites
Metabolites extracted from S. aureus using three methods, FTC, SC, and their combination FTC + SC, were separated using UPLC ultimate 3,000 (C18and HILIC column), detected and quantified using an Orbitrap Fusion Mass Spectrometer and identified using the untargeted workflow of Progenesis QI software for metabolomics (Water Corporation). The list of identified non-polar and polar metabolites from the C18 and HILIC columns, respectively, was merged to get a collated list of metabolites extracted from each method-FTC, SC, and FTC + SC (Table 1).

Table 1. Number of identified metabolites with respect to different metabolite extraction methods and chromatographic surfaces (polar/non-polar).
The data of identified metabolites were subjected to PCA analysis to identify the distribution of the metabolomic profiles generated using FTC, SC and FTC + SC methods (Table 1). Noticeable differences between the three extraction protocols (3 distinct clusters) were observed indicating the characteristic fingerprint of each method (Figure 1A). The separation and clustering in PCA analysis also indicated the biasness of the individual method for different classes of metabolites and the quality of the sample generated for metabolomics experiments, respectively. Overall, a total of 116, 119, and 99 metabolites were identified using the FTC, SC, and FTC + SC methods of metabolite extraction, respectively, leading to the identification of 163 metabolites cumulatively (Tables 1, 2).
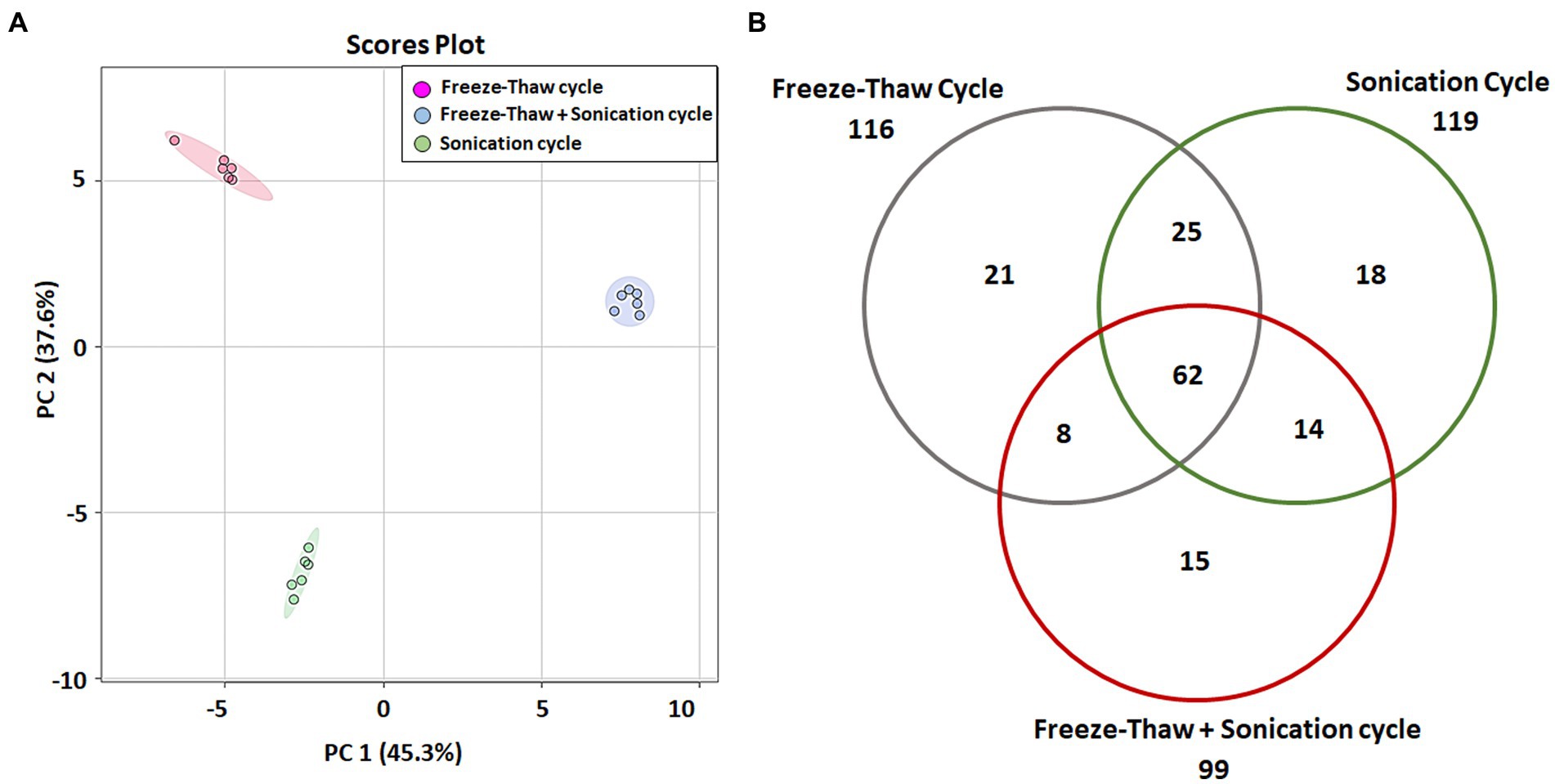
Figure 1. Multi-variate analysis of identified metabolites. (A) Principal component analysis (PCA) showing score plots of the metabolic differences between 3 methods (consisting a total 6 independent biological replicates for each method), red: freeze-thaw Cycle (FTC), green: Sonication Cycle (SC), blue: freeze-thaw Cycle followed by Sonication Cycle (FTC + SC). All three methods clustered distinctly having replicates of each method together indicating the consistency of sample preparation and downstream analysis. (B) Venn diagram representing unique and common metabolites among the three different methods of metabolite extraction [freeze-thaw cycle (FTC), Sonication Cycle (SC) and freeze-thaw cycle followed by Sonication Cycle (FTC + SC)]. A total of 62 metabolites were common among all 3 methods, whereas 21, 18, and 15 were uniquely identified in FTC, SC, and FTC + SC, respectively, indicating the potential biasness of the individual methods. Data from n ≥ 4 (out of six independent biological replicates were used in this analysis). One-way ANOVA was used to select the significant metabolites, i.e., 163 total metabolites (cutoff value of p ≤ 0.05) for the analysis.
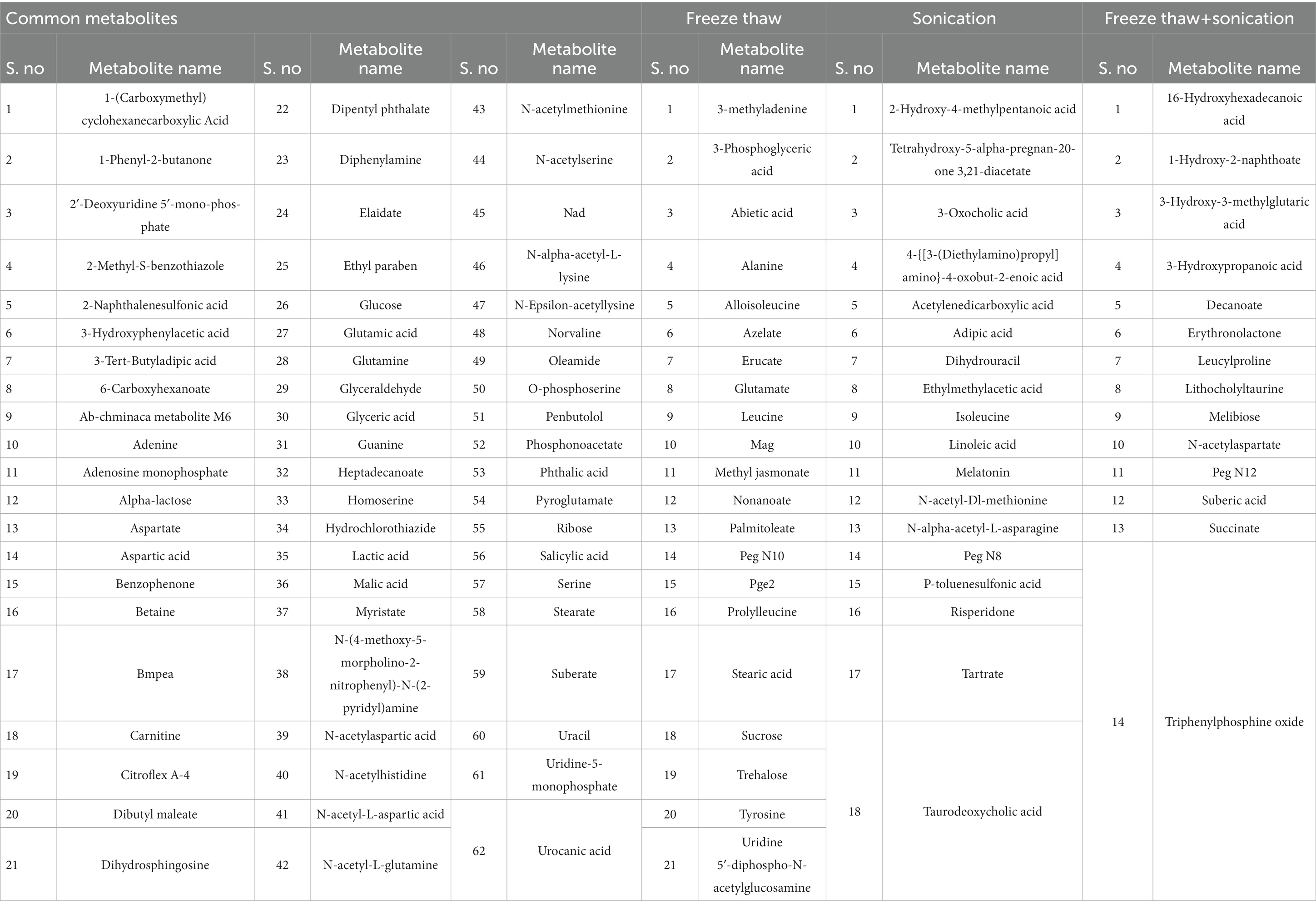
Table 2. List of identified metabolites specific to metabolite extraction protocols (FTC, SC, and FTC + SC) as well as commonly identified metabolites among all methods.
Overall, 62 metabolites were common among all three methods. FTC and SC yielded 25 common metabolites, 14 were common between FTC + SC and SC methods, and eight metabolites were identified by both FTC and FTC + SC methods (Figure 1B). Whereas 21 metabolites were uniquely identified in the FTC method followed by 18 unique metabolites by the sonication method, and only 15 unique metabolites by FTC + SC (Table 2).
3.2. Chemical classification of identified metabolites
All the metabolite extraction methods (FTC, SC, and FTC + SC) predominantly enabled the identification of different chemical classes of amino acids followed by saturated fatty acids and dicarboxylic acid [Figures 1–4 (Supplementary File-4)]. Similar pattern was observed in uniquely identified metabolites.
The dominant class of metabolites identified as amino acids (29% by FTC and 27% by SC and FTC + SC) followed by saturated fatty acids. Interestingly, the class “Naphthalene carboxylic acid” was uniquely identified in the FTC + SC method only (Figure 2). However, uniquely identified metabolites from individual methods showed biases toward specific chemical classes/subclass of metabolites (Figure 2).
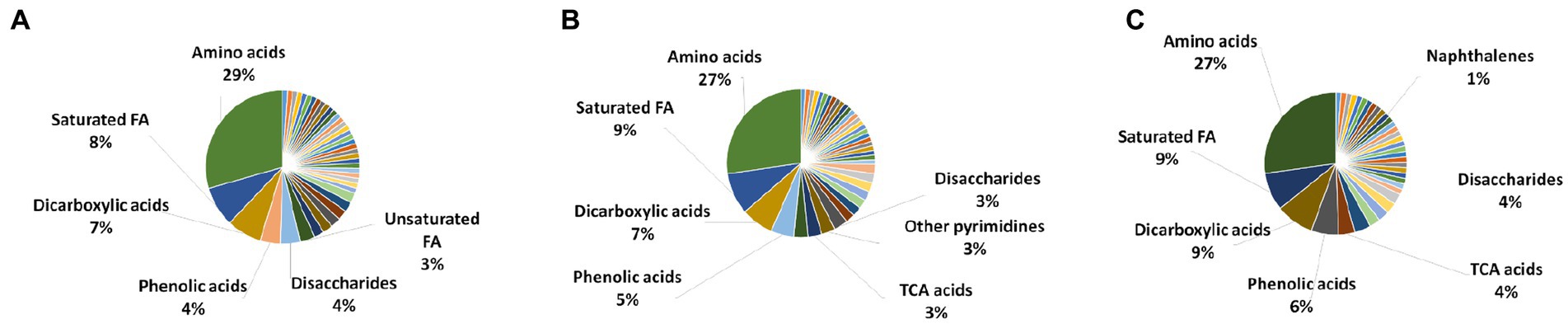
Figure 2. Chemical class analysis of identified metabolites. Pie-Chart of metabolite chemical class composition, identified using three different metabolite extraction methods n ≥ 4 (out of 6 independent experiments, selected using one-way ANOVA test with cutoff value of p ≤ 0.05) biological replicates were utilized to analyze the chemical class distribution. (A) freeze-thaw Cycle; (B) sonication Cycle; and (C) freeze-thaw Cycle followed by Sonication Cycle. The major metabolite class identified using each method was amino acids, followed by saturated fatty acids, dicarboxylic acids and phenolic acids (only major classes are shown in the figure). FA, fatty acids; TCA acids, trichloroacetic acids.
3.3. Differential abundance of identified metabolites from FTC, SC, and FTC + SC method
The difference in intensity of metabolites was observed with different methods of extraction (Figure 3 and Supplementary Figure 5). A few metabolites were observed to be more intense in a specific method compared to others (Figure 4). For example, Alloisoleucine, nonanoate, palmitoleate, leucine, sucrose, tyrosine, glutamate, and prolylleucine were more abundant in the FTC method. Fucose, risperidone, hexazinone, and isoleucine were found to be more abundant in the SC method. Decanoate, succinate, leucylproline, and melibiose were found to be higher in intensity in FTC + SC. A few metabolites were identified by two methods. For example, maltose, suberate, pentadecanoic acid, palmitoleic acid, arachidate, fipronil, adenosine, and urocanate were more abundant in FTC and SC. Phenylalanine, pyruvic acid, sebacic acid, arabinose, palatinose, fumaric acid, laurate, palmitic acid, and serine were found to be more abundant in FTC + SC and SC methods. Guanine, azelaic acid, linoleate, adenine, and uracil were highly abundant in FTC and FTC + SC methods. Whereas a few metabolites like carnitine and glucose were identified by all three methods. This altogether indicates the biasness of metabolite extraction methods toward particular metabolite(s).
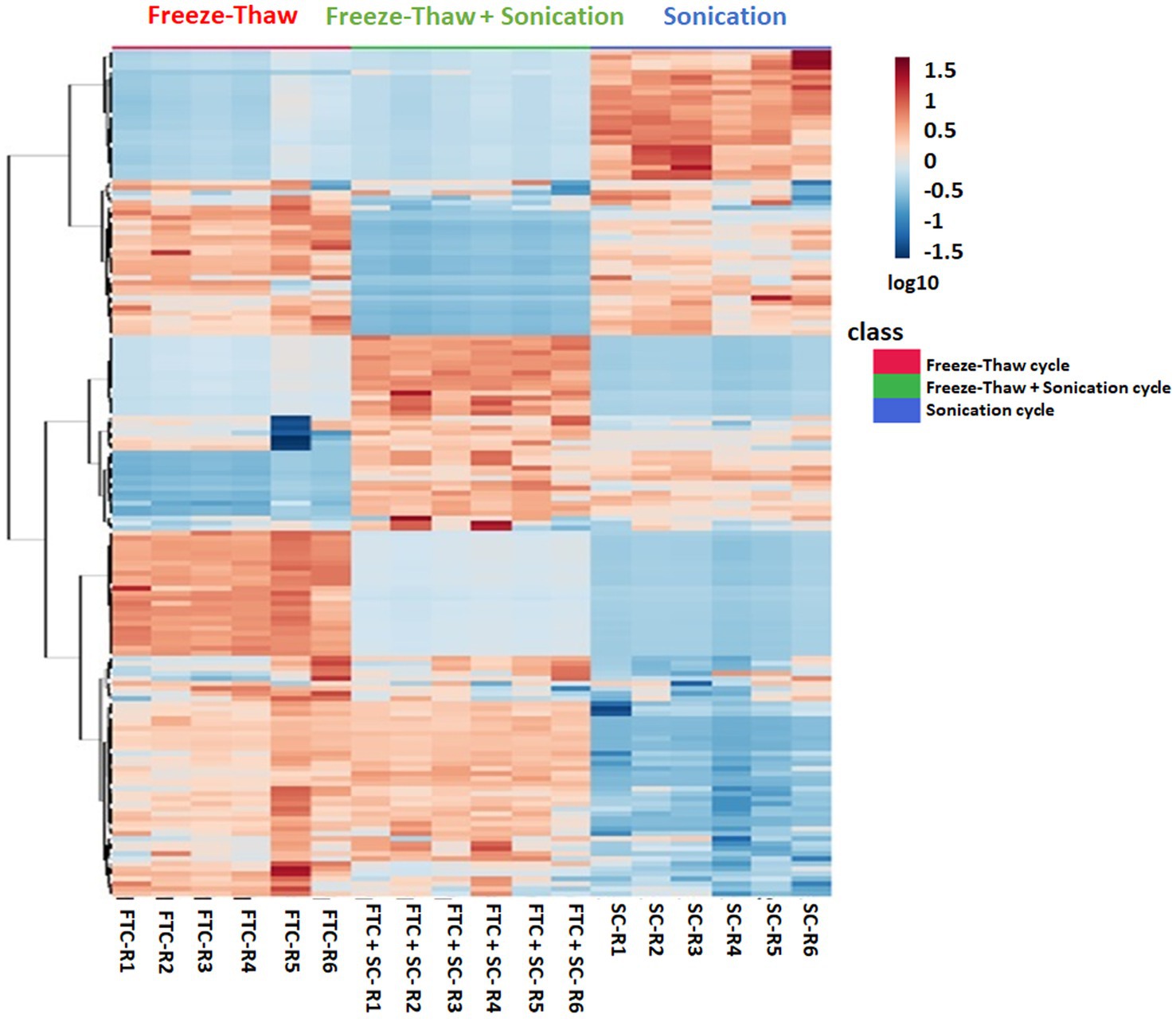
Figure 3. Heatmap profile depicting the relative expression levels of 163 metabolites (selected using one-way ANOVA test with cutoff value of p ≤ 0.05) from all three different extraction methods, i.e., freeze-thaw Cycle (FTC), Sonication Cycle (SC) and freeze-thaw Cycle followed by Sonication Cycle (FTC + SC) as indicated. Each column represents a specific biological replicates sample and row represent the metabolite. The raw intensities of metabolites from n ≥ 4 (from six independent biological replicates) were parsed by Pareto scaling (mean-centered and divided by the square root of the standard deviation of each variable) and rendered using the MetaboAnalyst 5.0 software. The clustering of the rows is based on Euclidean distance measure and Ward clustering method. A few metabolite clusters were observed to be more intensely expressed (indicated by Red) in a specific method compared to others showing biasness of the method(s). The information of the members in these clusters was utilized to explore their AMR-related biological function. The color is representing log10 transformed metabolite intensities (red: highest; blue: lowest).
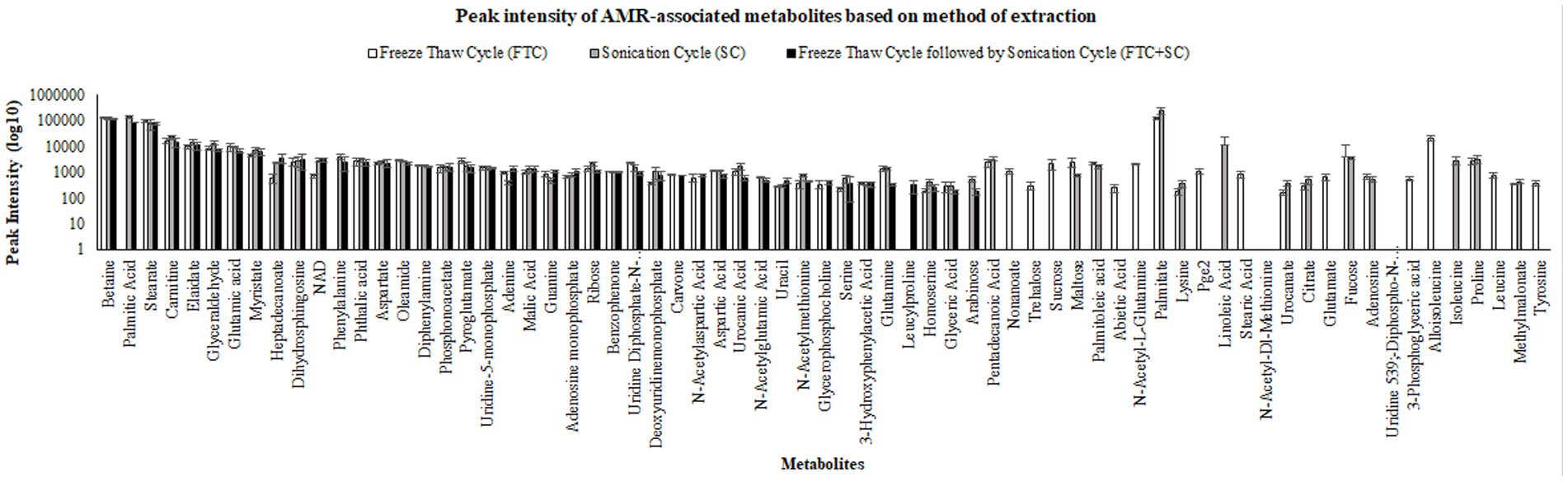
Figure 4. Pictorial representation of peak intensities of identified metabolites (selected using one-way ANOVA, means ± SD, with cutoff value of p ≤ 0.05) using three different metabolite extraction methods (FTC, SC and FTC + SC). The data represents the metabolites (intensity) from the n ≥ 4 (out of six independent biological replicate-Supplementary Table 1) samples. FTC, freeze-thaw cycle; SC, sonication cycle; FTC + SC, freeze-thaw cycle followed by sonication cycle.
3.4. AMR-associated metabolites
Out of 163, a total of 69 metabolites were found to be related with AMR-associated phenotypes (cell proliferation and cell death) (Table 3). Of these, 57, 54, and 40 AMR-associated metabolites were found in metabolite samples extracted from FTC, SC or FTC + SC method, whereas 12, 7, and 2 were unique to each method (Table 3).
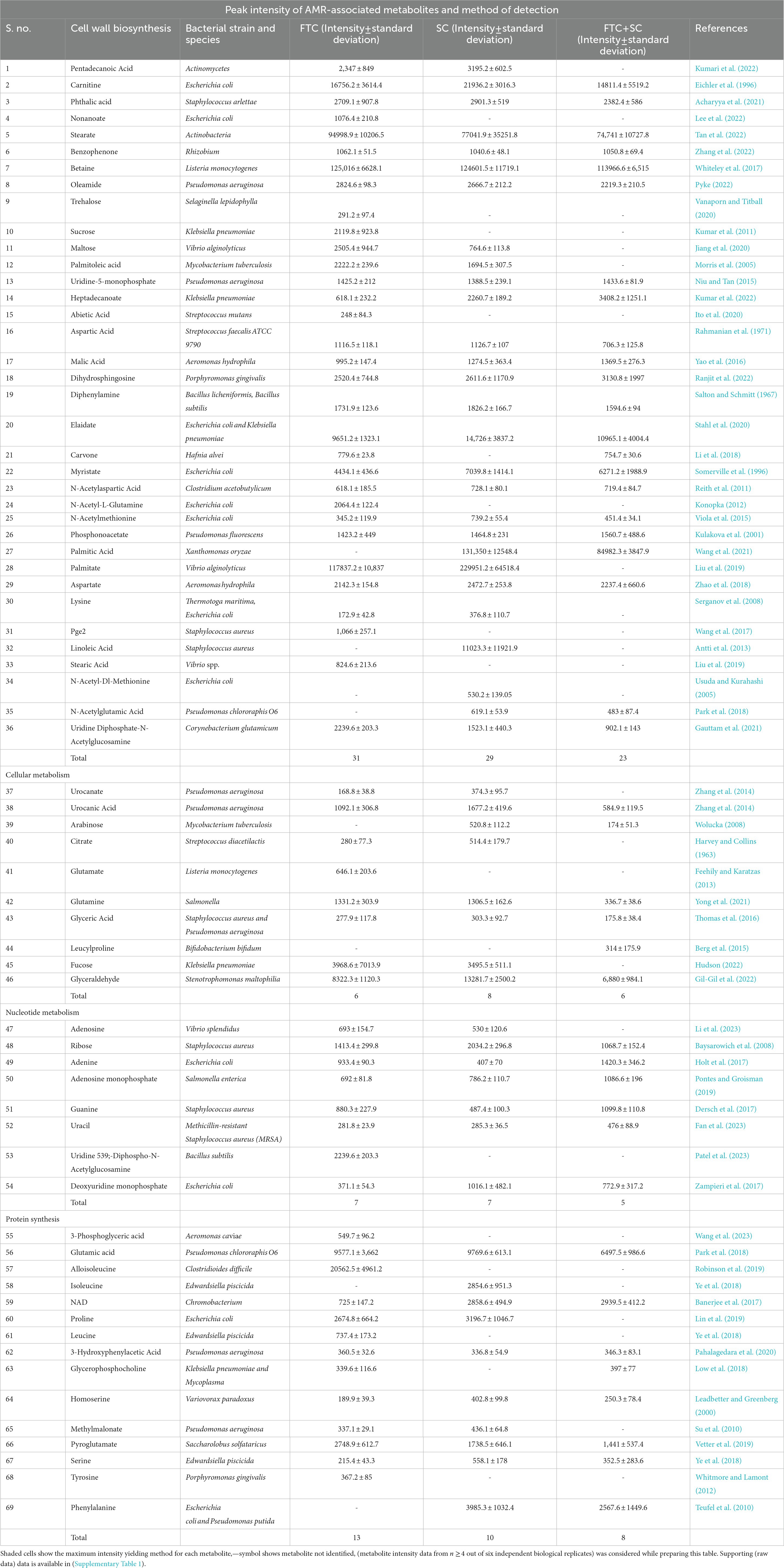
Table 3. Comparative overview of identified antimicrobial resistance (AMR)-associated metabolites among three different methods of metabolite extraction used in the study.
4. Discussion
Infections caused by Gram-positive bacteria have become a serious public health threat causing high morbidity and mortality (Jubeh et al., 2020). Limited treatment options and rapid development of resistance to even the last-line antibiotics is the main reason for deaths, especially in the case of S. aureus (MRSA infections) (Chambers and Deleo, 2009; Alos, 2015). Besides enormous efforts, very few has been discovered over the last few decades, and this necessitates identifying new strategies to combat the emerging problem of AMR (WHO, 2020). Thus, understanding the molecular changes driving AMR among S. aureus becomes very important. Metabolomics can decode the real biochemical state of any organism and help in analyzing the emergence and/or spread of AMR phenomena (Pinu et al., 2019). To date metabolomics has been employed to identify new metabolic pathways (Deng et al., 2020), identification of bacterial species/strains (Zhang and Zhu, 2022), study the influence of external factors on bacteria (Tang, 2011), and in some cases reported to be of diagnostic use to detect bacterial infections (Fernández-García et al., 2018). However, so far, very few studies and efforts have been made to understand the spread and/or emergence of AMR and this may be primarily due to the unavailability of appropriate protocols and pipelines for investigating global and AMR-associated metabolites. As of now, even the performance of existing protocols for extracting AMR-associated intracellular metabolites from Gram-positive pathogen, S. aureus, are not established and hence the optimal method for the purpose remains unknown.
4.1. Analysis of metabolite extraction method and identified metabolites
The metabolites were extracted from S. aureus (gentamicin and methicillin resistant) using the two most commonly used protocols, i.e., FTC, and SC, alone and in the combination [FTC followed by SC (FTC + SC)] and identified using ESI-LC–MS/MS (Orbitrap Fusion Tribrid Mass Analyzer), a highly sensitive and advanced mass-spectrometer to potentially achieve increased metabolomic coverage. Further, we have used 2 different Liquid Chromatography (LC) columns, i.e., HILIC and C18 which are specific for separating compounds with different physico-chemical properties were also used. Generally, HILIC columns are very well known for separating polar amino acids, organic acids, sugars, phosphorylated sugars, nucleobases, nucleotides, phosphorylated metabolites, hydrophilic vitamins, and coenzymes (Galeano Garcia et al., 2019). C18 columns are usually employed to separate semi-polar and non-polar compounds like alkaloids, flavonoids, phenolic acids, and other glycosylated species (Liu et al., 2019). Data analysis from individual LC columns (HILIC and C18) revealed that C18 enabled a higher number of metabolite identification compared to HILIC among all the metabolite extraction methods used (Table 1). This may potentially be because of poor retention of very polar metabolites during chromatographic separation and hence is in line with published literature (Harrieder et al., 2022). Only a few metabolites were common among the metabolites identified using C18 and HILIC columns. A total of 163 metabolites were cumulatively identified using all chromatographic surfaces and extraction methods. The SC method showed the highest number of metabolites though comparable with FTC among the total identified metabolites (116 by FTC and 119 by SC out of a total of 163 metabolites). The most likely reason for low yield in FTC + SC methods might be the degradation of already extracted metabolites during sonication. Among 163 metabolites, 21, 18, and 15 were uniquely identified in FTC, SC, and FTC + SC methods indicating the potential bias of the method toward specific metabolite(s) (Table 2). Chemical class-based functional categorization and analysis revealed that amino acids were the major chemical class of metabolites identified by all methods. The FTC method was observed to be more biased toward saturated fatty acids (17%), and disaccharides (17%) (Supplementary Figure 2). The SC method showed biasness toward hexoses (2%), C24 bile acids (2%), and pyrimidines (3%) (Supplementary Figure 3). Disruption of the peptidoglycan layer (mesh-like network of amino acids and sugar) during metabolite extraction might be the possible reason for yielding a high number of amino acids, dipeptides, sugar derivatives like hexoses, disaccharides, and sugar acids (Vollmer et al., 2008). However, a combination of FTC + SC methods showed an enrichment of hydroxy fatty acids (28%) (Supplementary Figure 4). This altogether indicates the potential bias of each method for investigating specific metabolites or classes of metabolites. Therefore, the method of metabolite extraction shall primarily be chosen based on the specific metabolite(s) of interest or classes of metabolites. A few attempts have been made by researchers to establish the metabolome of this clinically relevant Gram-positive pathogen, S. aureus. Recently, 109 metabolites (RN450) and 107 (450 M) metabolites were identified using cold methanol and vortexing (vigorously for ~1 min) based metabolite extraction method with HPLC coupled with a TSQ Quantiva Triple Quadrupole mass spectrometer in (methicillin-resistant S. aureus) MRSA and (methicillin sensitive S. aureus) MSSA strains, respectively, after exposure to a sublethal dose of antibiotic (ampicillin, kanamycin, norfloxacin) (Schelli et al., 2017). However, the number of identified metabolites was low compared to our study potentially due to the use of only the HILIC column, one extraction method, i.e., vortexing (instead of freeze-thaw and vortexing or sonication) and the low-sensitive equipment (Schelli et al., 2017). Another attempt to differentiate MSSA and MRSA biofilm and planktonic phenotypes using an (Nuclear magnetic resonance spectroscopy) NMR-based metabolomics study has been reported. They have identified a total of 120 metabolites (Ammons et al., 2014). Although the reason for identifying a lower number of metabolites in this study might be because of the technology used, NMR is already known to be less sensitive than ESI-LC–MS/MS (Ammons et al., 2014). Among them, 19 and 26 common metabolites were identified when compared to our study, respectively, (Table 4 and Figure 5) (Ammons et al., 2014; Schelli et al., 2017). Similarly, a total of 173 metabolites were identified in MRSA and MSSA using a combination of hydrophilic interaction liquid chromatography and PFP columns (pentafluorophenyl-propyl) coupled with high-resolution mass spectrometry (Aros-Calt et al., 2019). The possible reason for yielding a higher number of metabolites in comparison to our study may be due to a bigger library size compared to our in-house library (~950 metabolites). Notably, none of the above-discussed studies have mentioned the identified AMR-associated metabolites.
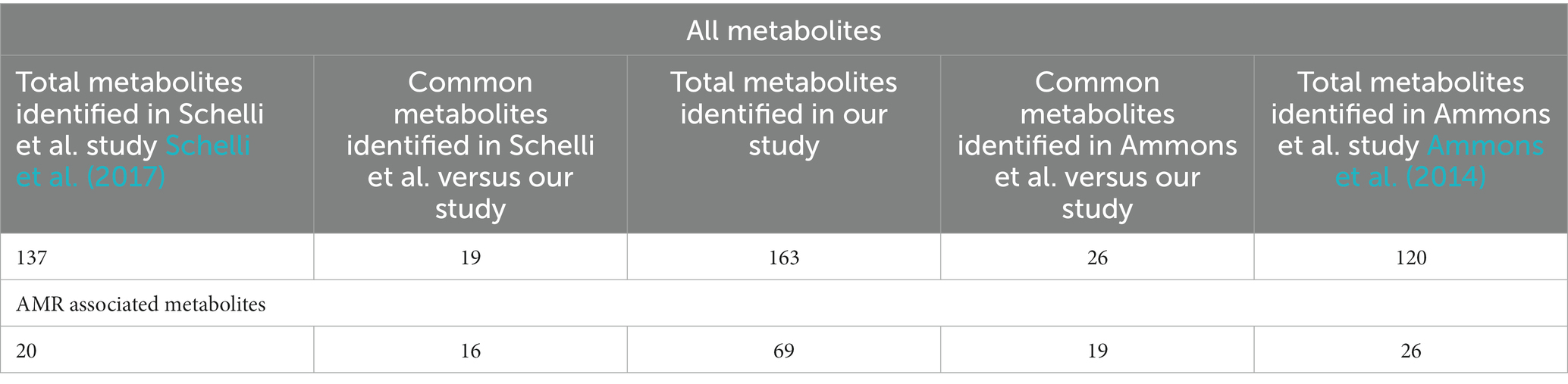
Table 4. Comparison of metabolites [total and antimicrobial resistance (AMR) associated] identified from our study versus other reported studies.
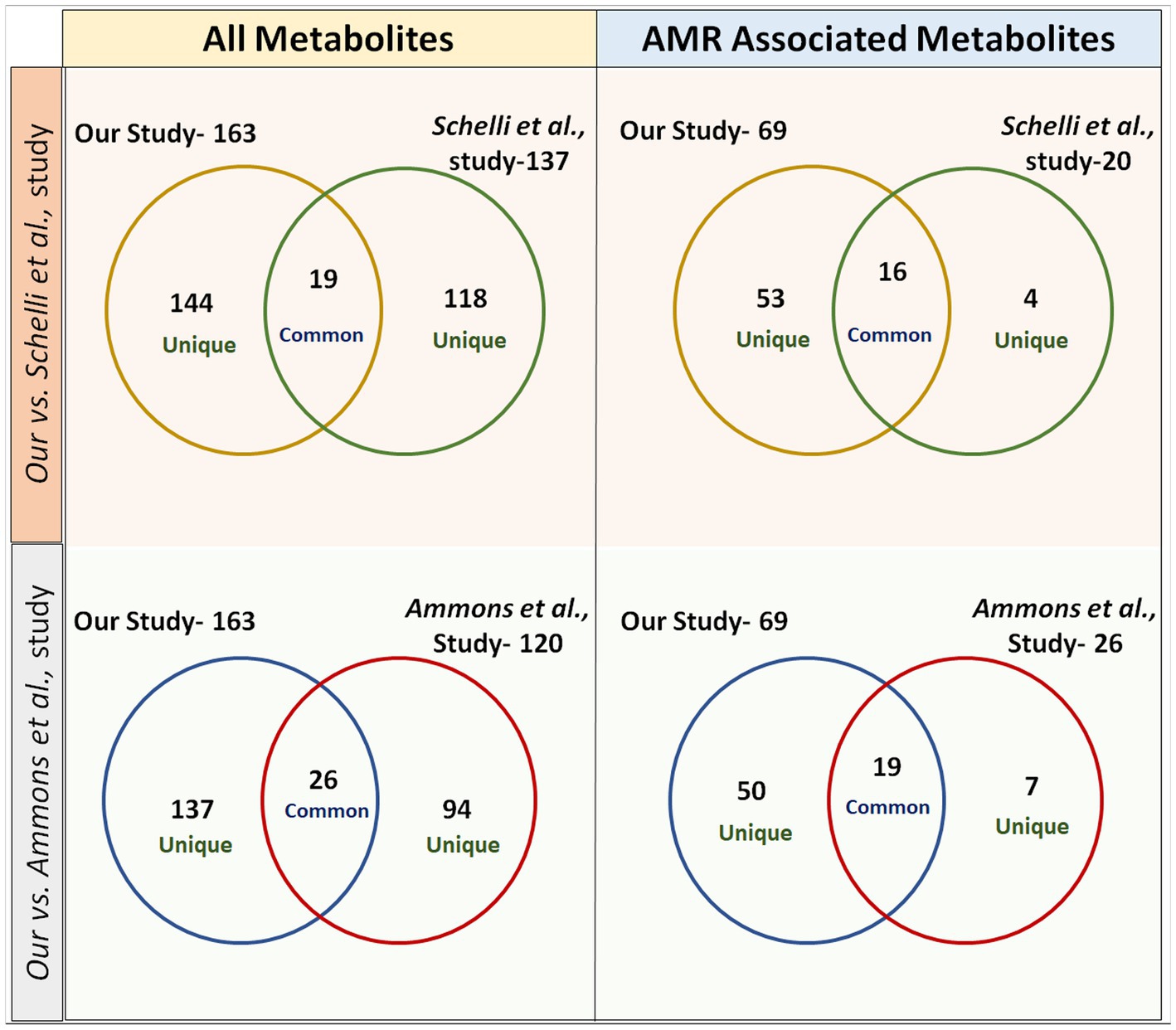
Figure 5. Venn diagrams representing a comparison of metabolites identified from our study versus previously published studies in the domain. In study-1 (Ammons et al.), 26 metabolites were found to be common in total metabolites and 19 metabolites were common in AMR-associated metabolites with our study. In study-2 (Schelli et al.), 19 metabolites were found to be common in total metabolites and 16 metabolites were common in AMR-associated metabolites with our study.
4.2. Analysis of AMR-associated metabolites
Of the identified 163 metabolites, 69 (42.0%) were observed to be associated with AMR in published literature (Table 3). Among these, the majority of identified AMR-associated metabolites were primarily linked with cell wall biosynthesis (52.1%), followed by cellular metabolism (14.4%), nucleotide biosynthesis (11.5%), and protein synthesis (21.7%) indicating the potential bias of the method of metabolite extraction or the technique. A comparison of identified AMR-associated metabolites list with previously reported study has shown only 16 and 19 common metabolites (Table 4) (Ammons et al., 2014; Schelli et al., 2017). Interestingly, the FTC method yielded a slightly higher number of AMR-associated metabolites (57 metabolites) compared to SC (54 metabolites) with 42 commonly identified metabolites. Whereas only 40 AMR-associated metabolites were identified using the FTC + SC method potentially due to the degradation of extracted metabolites (after FTC) during sonication. This altogether indicates the suitability of both the methods, FTC or SC, for investigating AMR-associated metabolites in S. aureus and Gram-positive pathogens; however, the metabolite of interest shall be key in choosing the method for metabolite extraction.
4.3. Analysis of metabolite extraction methods between Gram-positive pathogen, Staphylococcus aureus and Gram-negative pathogen, Klebsiella pneumoniae
In this investigation, the maximum number of metabolites from S. aureus were identified using SC (119 metabolites) and subsequently by FTC (116 metabolites) and FTC + SC (99 metabolites). Whereas for K. pneumoniae, the maximum number of metabolites were identified by the FTC (151) method followed by FTC + SC (132 metabolites) and SC (103 metabolites; Supplementary Table 2) (Kumar et al., 2022). Notably, the number of metabolites identified in K. pneumoniae was more when compared to S. aureus and the potential reason remains unknown. Among these, only a few metabolites were commonly identified between S. aureus and K. pneumoniae (26 by FTC, 45 by SC and 31 by FTC + SC). A similar pattern was observed in AMR-associated metabolites. This supports that the metabolomic architecture and AMR-associated metabolites among S. aureus (a Gram-positive pathogen) may be different than the K. pneumoniae (a Gram-negative pathogen). This also suggests that the optimal method for extracting metabolites from Gram-positive pathogens may be different than the method for Gram-negative pathogens.
5. Conclusion
The increasing emergence of AMR among Gram-positive pathogens such as S. aureus is becoming a global health concern and urgently demands strategies to control it. Understanding the metabolomic footprint of antibiotic-resistant/sensitive pathogens has been shown to improve our understanding of the emergence/spread of AMR superbugs. However, very few efforts have been made in this direction possible due to the lack of appropriate methods of metabolite extraction.
Therefore, in this investigation, we have compared the performance of the two most common methods, i.e., FTC and SC alone and in combination (FTC + SC), for extracting metabolites from S. aureus (gentamicin and methicillin-resistant) using a highly sensitive and advanced HPLC-coupled mass-spectrometer (ESI-LC–MS/MS). The SC and FTC methods were observed to identify a comparable number of total metabolites as well as AMR-associated metabolites and hence may be utilized for investigating the metabolome of S. aureus or other Gram-positive bacteria after further validation. FTC + SC gave a lower yield of metabolites possible due to the degradation of already extracted metabolites by FTC during sonication. The methods of metabolite extraction were also observed to have biasness toward specific metabolite(s) or class of metabolites (“Tryptamines” was unique to the SC method, “C20 isoprenoids and Jasmonic acids” were unique to FTC, and “Naphthalenes” were unique to FTC + SC method) and hence can potentially impact the overall finding of the metabolomics-based studies. Therefore, the method of metabolite extraction shall be primarily chosen based on the metabolites of interest in the investigation. Altogether, our data can help in designing/planning pathway specific/directed metabolomics studies which could improve understanding of the emergence/spread of AMR superbugs and ultimately contribute to improving the efficacy of existing antimicrobial therapies.
Data availability statement
The datasets presented in this study can be found in online repositories (MetaboLights study identifier-MTBLS7338). The names of the repository/repositories and accession number(s) can be found in the article/Supplementary material.
Author contributions
NK conceived, designed, and coordinated the study. SK and MK generated the study material. AK and YK performed most of the experiments. RS, LT, AK SK, YK, and NK performed the data analysis. LT, RS, and SR wrote the manuscript. NK and YK supervised the manuscript writing and revisions. All authors contributed to the article and approved the submitted version.
Funding
This work was supported by grants awarded to NK from the Indian Council of Medical Research Govt. of India (Grant No. AMR/Adhoc/233/2020-ECD-II) and Translational Health Science and Technology Institute Faridabad, India. RS received Senior Research Fellowship from the Council of Scientific and Industrial Research, Govt. of India, and SS and LT received Junior Research Fellowship from the Department of Biotechnology, Govt. of India.
Acknowledgments
We are grateful to Pramod Kumar Garg (Executive Director) and M. V. Santo (Head Administration) of Translational Health Science and Technology Institute Faridabad, India, for providing administrative support.
Conflict of interest
The authors declare that the research was conducted in the absence of any commercial or financial relationships that could be construed as a potential conflict of interest.
Publisher’s note
All claims expressed in this article are solely those of the authors and do not necessarily represent those of their affiliated organizations, or those of the publisher, the editors and the reviewers. Any product that may be evaluated in this article, or claim that may be made by its manufacturer, is not guaranteed or endorsed by the publisher.
Supplementary material
The Supplementary material for this article can be found online at: https://www.frontiersin.org/articles/10.3389/fmicb.2023.1152162/full#supplementary-material
Footnotes
References
Acharyya, S., Saha, S., Majumder, S., and Bhattacharya, M. (2021). Characterization of a mercury tolerant strain of staphylococcus arlettae from Darjeeling hills with an account of its antibiotic resistance pattern and metabolome. Arch. Microbiol. 203, 5745–5754. doi: 10.1007/s00203-021-02563-5
Alos, J. I. (2015). Antibiotic resistance: A global crisis. Enferm. Infecc. Microbiol. Clin. 33, 692–699. doi: 10.1016/j.eimc.2014.10.004
Ammons, M. C., Tripet, B. P., Carlson, R. P., Kirker, K. R., Gross, M. A., Stanisich, J. J., et al. (2014). Quantitative NMR metabolite profiling of methicillin-resistant and methicillin-susceptible Staphylococcus aureus discriminates between biofilm and planktonic phenotypes. J. Proteome Res. 13, 2973–2985. doi: 10.1021/pr500120c
Antimicrobial Resistance, C (2022). Global burden of bacterial antimicrobial resistance in 2019: a systematic analysis. Lancet 399, 629–655. doi: 10.1016/S0140-6736(21)02724-0
Antti, H., Fahlgren, A., Näsström, E., Kouremenos, K., Sundén-Cullberg, J., Guo, Y., et al. (2013). Metabolic profiling for detection of Staphylococcus aureus infection and antibiotic resistance. PLoS One 8:e56971. doi: 10.1371/journal.pone.0056971
Aros-Calt, S., Castelli, F. A., Lamourette, P., Gervasi, G., Junot, C., Muller, B. H., et al. (2019). Metabolomic investigation of Staphylococcus aureus antibiotic susceptibility by liquid chromatography coupled to high-resolution mass spectrometry. Methods Mol. Biol. 1871, 279–293. doi: 10.1007/978-1-4939-8814-3_18
Banerjee, D., Parmar, D., Bhattacharya, N., Ghanate, A. D., Panchagnula, V., and Raghunathan, A. (2017). A scalable metabolite supplementation strategy against antibiotic resistant pathogen Chromobacterium violaceum induced by NAD(+)/NADH(+) imbalance. BMC Syst. Biol. 11:51. doi: 10.1186/s12918-017-0427-z
Bassetti, M., Peghin, M., Vena, A., and Giacobbe, D. R. (2019). Treatment of infections due to MDR gram-negative bacteria. Front. Med. (Lausanne) 6:74. doi: 10.3389/fmed.2019.00074
Baysarowich, J., Koteva, K., Hughes, D. W., Ejim, L., Griffiths, E., Zhang, K., et al. (2008). Rifamycin antibiotic resistance by ADP-ribosylation: structure and diversity of Arr. Proc. Natl. Acad. Sci. U. S. A. 105, 4886–4891. doi: 10.1073/pnas.0711939105
Berg, M., García-Hernández, R., Cuypers, B., Vanaerschot, M., Manzano, J. I., Poveda, J. A., et al. (2015). Experimental resistance to drug combinations in Leishmania donovani: metabolic and phenotypic adaptations. Antimicrob. Agents Chemother. 59, 2242–2255. doi: 10.1128/AAC.04231-14
Breijyeh, Z., Jubeh, B., and Karaman, R. (2020). Resistance of gram-negative bacteria to current antibacterial agents and approaches to resolve it. Molecules 25:1340. doi: 10.3390/molecules25061340
Chambers, H. F., and Deleo, F. R. (2009). Waves of resistance: Staphylococcus aureus in the antibiotic era. Nat. Rev. Microbiol. 7, 629–641. doi: 10.1038/nrmicro2200
Conlon, B. P., Rowe, S. E., Gandt, A. B., Nuxoll, A. S., Donegan, N. P., Zalis, E. A., et al. (2016). Persister formation in Staphylococcus aureus is associated with ATP depletion. Nat. Microbiol. 1, 1–7. doi: 10.1038/nmicrobiol.2016.51
Deng, L., Guo, F., Cheng, K. K., Zhu, J., Gu, H., Raftery, D., et al. (2020). Identifying significant metabolic pathways using multi-block partial least-squares analysis. J. Proteome Res. 19, 1965–1974. doi: 10.1021/acs.jproteome.9b00793
Dersch, P., Khan, M. A., Mühlen, S., and Görke, B. (2017). Roles of regulatory RNAs for antibiotic resistance in bacteria and their potential value as novel drug targets. Front Microbiol. 8:803. doi: 10.3389/fmicb.2017.00803
Dhingra, S., Rahman, N. A. A., Peile, E., Rahman, M., Sartelli, M., Hassali, M. A., et al. (2020). Microbial resistance movements: an overview of global public health threats posed by antimicrobial resistance, and how best to counter. Front. Public Health 8:535668. doi: 10.3389/fpubh.2020.535668
Eichler, K., Buchet, A., Lemke, R., Kleber, H. P., and Mandrand-Berthelot, M. A. (1996). Identification and characterization of the caiF gene encoding a potential transcriptional activator of carnitine metabolism in Escherichia coli. J. Bacteriol. 178, 1248–1257. doi: 10.1128/jb.178.5.1248-1257.1996
Fan, L., Pan, Z., Liao, X., Zhong, Y., Guo, J., Pang, R., et al. (2023). Uracil restores susceptibility of methicillin-resistant Staphylococcus aureus to aminoglycosides through metabolic reprogramming. Front. Pharmacol. 14. doi: 10.3389/fphar.2023.1133685
Feehily, C., and Karatzas, K. A. (2013). Role of glutamate metabolism in bacterial responses towards acid and other stresses. J. Appl. Microbiol. 114, 11–24. doi: 10.1111/j.1365-2672.2012.05434.x
Fernández-García, M., Rojo, D., Rey-Stolle, F., García, A., and Barbas, C. (2018). Metabolomic-based methods in diagnosis and monitoring infection progression. Exp. Suppl. 109, 283–315. doi: 10.1007/978-3-319-74932-7_7
Galeano Garcia, P., Zimmermann, B. H., and Carazzone, C. (2019). Hydrophilic interaction liquid chromatography coupled to mass spectrometry and multivariate analysis of the De novo pyrimidine pathway metabolites. Biomolecules 9:328. doi: 10.3390/biom9080328
Gauttam, R., Desiderato, C. K., Radoš, D., Link, H., Seibold, G. M., and Eikmanns, B. J. (2021). Metabolic engineering of Corynebacterium glutamicum for production of UDP-N-Acetylglucosamine. Front. Bioeng. Biotechnol. 9:748510. doi: 10.3389/fbioe.2021.748510
Gil-Gil, T., Ochoa-Sánchez, L. E., and Martínez, J. L. (2022). The antibiotic fosfomycin mimics the effects of the intermediate metabolites phosphoenolpyruvate and glyceraldehyde-3-phosphate on the stenotrophomonasmaltophilia transcriptome. Int. J. Mol. Sci. 23:159. doi: 10.3390/ijms23010159
Harper, L., Balasubramanian, D., Ohneck, E. A., Sause, W. E., Chapman, J., Mejia-Sosa, B., et al. (2018). Staphylococcus aureus responds to the central metabolite pyruvate to regulate virulence. MBio 9, e02272–e02217. doi: 10.1128/mBio.02272-17
Harrieder, E. M., Kretschmer, F., Böcker, S., and Witting, M. (2022). Current state-of-the-art of separation methods used in LC-MS based metabolomics and lipidomics. J. Chromatogr. B Anal. Technol. Biomed. Life Sci. 1188:123069. doi: 10.1016/j.jchromb.2021.123069
Harvey, R. J., and Collins, E. B. (1963). Roles of citrate and Acetoin in the metabolism of streptococcus Diacetilactis. J. Bacteriol. 86, 1301–1307. doi: 10.1128/jb.86.6.1301-1307.1963
Holt, G. S., Lodge, J. K., McCarthy, A. J., Graham, A. K., Young, G., Bridge, S. H., et al. (2017). Shigatoxin encoding bacteriophage varphi24(B) modulates bacterial metabolism to raise antimicrobial tolerance. Sci. Rep. 7:40424. doi: 10.1038/srep40424
Hudson, A. W. (2022). Role of FUCOSE utilization on Klebsiella Pneumoniae gut colonization and pathogenesis. [Doctoral dissertation]. Wake Forest University.
Ito, Y., Ito, T., Yamashiro, K., Mineshiba, F., Hirai, K., Omori, K., et al. (2020). Antimicrobial and antibiofilm effects of abietic acid on cariogenic Streptococcus mutans. Odontology 108, 57–65. doi: 10.1007/s10266-019-00456-0
Jiang, M., Yang, L., Chen, Z. G., Lai, S. S., Zheng, J., and Peng, B. (2020). Exogenous maltose enhances Zebrafish immunity to levofloxacin-resistant vibrio alginolyticus. Microb. Biotechnol. 13, 1213–1227. doi: 10.1111/1751-7915.13582
Jubeh, B., Breijyeh, Z., and Karaman, R. (2020). Resistance of gram-positive bacteria to current antibacterial agents and overcoming approaches. Molecules 25:2888. doi: 10.3390/molecules25122888
Konopka, J. B. (2012). N-acetylglucosamine (GlcNAc) functions in cell signaling. Scientifica (Cairo) 2012, 1–15. doi: 10.6064/2012/489208
Kulakova, A. N., Kulakov, L. A., Akulenko, N. V., Ksenzenko, V. N., Hamilton, J. T. G., and Quinn, J. P. (2001). Structural and functional analysis of the phosphonoacetate hydrolase (phnA) gene region in Pseudomonas fluorescens 23F. J. Bacteriol. 183, 3268–3275. doi: 10.1128/JB.183.11.3268-3275.2001
Kumar, A., Singh, S., Gupta, S. K., Kumar, S., Kumar, S., Singh, R., et al. (2022). Identification of metabolite extraction method for targeted exploration of antimicrobial resistance associated metabolites of Klebsiella pneumoniae. Sci. Rep. 12:8939. doi: 10.1038/s41598-022-12153-0
Kumar, V., Sun, P., Vamathevan, J., Li, Y., Ingraham, K., Palmer, L., et al. (2011). Comparative genomics of Klebsiella pneumoniae strains with different antibiotic resistance profiles. Antimicrob. Agents Chemother. 55, 4267–4276. doi: 10.1128/AAC.00052-11
Kumari, N., Pandey, S., and Menghani, E. J. I. J. O. G.-M. S. (2022). Evaluation of actinomycetes isolated antimicrobial metabolites as potent inhibitor of multidrug resistant organisms. Indian J Mar Sci. 50, 29–36. doi: 10.56042/ijms.v50i01.66080
Leadbetter, J. R., and Greenberg, E. P. (2000). Metabolism of acyl-homoserine lactone quorum-sensing signals by Variovorax paradoxus. J. Bacteriol. 182, 6921–6926. doi: 10.1128/JB.182.24.6921-6926.2000
Lee, A. S., de Lencastre, H., Garau, J., Kluytmans, J., Malhotra-Kumar, S., Peschel, A., et al. (2018). Methicillin-resistant Staphylococcus aureus. Nat. Rev. Dis. Primers. 4:18033. doi: 10.1038/nrdp.2018.33
Lee, Y., Sathesh-Prabu, C., Kwak, G. H., Bang, I., Jung, H. W., Kim, D., et al. (2022). Enhanced production of nonanedioic acid from nonanoic acid by engineered Escherichia coli. Biotechnol. J. 17:e2000416. doi: 10.1002/biot.202000416
Li, Y., Liang, W., and Li, C. J. M. R. (2023) Exogenous adenosine and/or guanosine enhances tetracycline sensitivity of Vibrio splendidus persister cells. Microbiol. Res. 270:127321. doi: 10.1016/j.micres.2023.127321
Li, T., Mei, Y., He, B., Sun, X., and Li, J. (2018). Reducing quorum sensing-mediated virulence factor expression and biofilm formation in Hafnia alvei by using the potential quorum sensing inhibitor L-Carvone. Front. Microbiol. 9:3324. doi: 10.3389/fmicb.2018.03324
Lin, Y., Li, W., Sun, L., Lin, Z., Jiang, Y., Ling, Y., et al. (2019). Comparative metabolomics shows the metabolic profiles fluctuate in multi-drug resistant Escherichia coli strains. J. Proteome 207:103468. doi: 10.1016/j.jprot.2019.103468
Liu, Q., Cai, J., Nichols, R. G., Tian, Y., Zhang, J., Smith, P. B., et al. (2019). A quantitative HILIC-MS/MS assay of the metabolic response of Huh-7 cells exposed to 2,3,7,8-Tetrachlorodibenzo-p-dioxin. Meta 9:118. doi: 10.3390/metabo9060118
Liu, S. R., Peng, X. X., and Li, H. (2019). Metabolic mechanism of ceftazidime resistance in vibrio alginolyticus. Infect. Drug Resist. 12, 417–429. doi: 10.2147/IDR.S179639
Low, Y. M., et al. (2018). Elucidating the survival and response of carbapenem resistant Klebsiella pneumoniae after exposure to imipenem at sub-lethal concentrations. Pathog Glob Health. 112, 378–386. doi: 10.1080/20477724.2018.1538281
Masimen, M. A. A., Harun, N. A., Maulidiani, M., and Ismail, W. I. W. (2022). Overcoming methicillin-resistance Staphylococcus aureus (MRSA) using antimicrobial peptides-silver nanoparticles. Antibiotics (Basel) 11:951. doi: 10.3390/antibiotics11070951
Mehta, Y., Hegde, A., Pande, R., Zirpe, K. G., Gupta, V., Ahdal, J., et al. (2020). Methicillin-resistant Staphylococcus aureus in intensive care unit setting of India: a review of clinical burden, patterns of prevalence, preventive measures, and future strategies. Indian J. Crit. Care Med. 24, 55–62. doi: 10.5005/jp-journals-10071-23337
Morris, R. P., Nguyen, L., Gatfield, J., Visconti, K., Nguyen, K., Schnappinger, D., et al. (2005). Ancestral antibiotic resistance in mycobacterium tuberculosis. Proc. Natl. Acad. Sci. U. S. A. 102, 12200–12205. doi: 10.1073/pnas.0505446102
Mulani, M. S., Kamble, E. E., Kumkar, S. N., Tawre, M. S., and Pardesi, K. R. (2019). Emerging strategies to combat ESKAPE pathogens in the era of antimicrobial resistance: a review. Front. Microbiol. 10:539. doi: 10.3389/fmicb.2019.00539
Niu, G., and Tan, H. (2015). Nucleoside antibiotics: biosynthesis, regulation, and biotechnology. Trends Microbiol. 23, 110–119. doi: 10.1016/j.tim.2014.10.007
Oxford, U.o . (2019). An estimated 1.2 million people died in 2019 from antibiotic-resistant bacterial infections. 7 10 22. Available from: https://www.ox.ac.uk/news/2022-01-20-estimated-12-million-people-died-2019-antibiotic-resistant-bacterial-infections#:~:text=One%20pathogen%2Ddrug%20combination%20%E2%80%93%20methicillin,between%2050%2C00020and20100%2C000%20deaths.
Pahalagedara, A., Flint, S., Palmer, J., Subbaraj, A., Brightwell, G., and Gupta, T. B. (2020). Antimicrobial activity of soil clostridium enriched conditioned media against bacillus mycoides, Bacillus cereus, and Pseudomonas aeruginosa. Front. Microbiol. 11:608998. doi: 10.3389/fmicb.2020.608998
Pang, R., Su, Y. B., Zhou, H., and Chen, X. (2020). Metabolites alleviate staphylococcal bloodstream infection in a NO-dependent manner via arginase inhibition. bioRxiv.
Park, J. Y., Kang, B. R., Ryu, C. M., Anderson, A. J., and Kim, Y. C. (2018). Polyamine is a critical determinant of pseudomonas chlororaphis O6 for GacS-dependent bacterial cell growth and biocontrol capacity 19, 1257–1266. doi: 10.1111/mpp.12610
Patel, Y., Soni, V., Rhee, K. Y., and Helmann, J. D. (2023). Mutations in rpoB that confer rifampicin resistance can alter levels of peptidoglycan precursors and affect β-lactam susceptibility. MBio, e03168–e03122. doi: 10.1128/mbio.03168-22
Pinu, F. R., Goldansaz, S. A., and Jaine, J. (2019). Translational metabolomics: current challenges and future opportunities. Meta 9:108. doi: 10.3390/metabo9060108
Pontes, M. H., and Groisman, E. A. (2019). Slow growth determines nonheritable antibiotic resistance in salmonella enterica. Sci. Signal. 12:eaax3938. doi: 10.1126/scisignal.aax3938
Pyke, S. (2022). Effects of small molecules produced by commensal bacteria on antibiotic resistant Pseudomonas aeruginosa. [Master’s thesis, Cumming School of Medicine]. University of Calgary.
Rahmanian, M., Waller, G. R., and Smith, W. G. (1971). Biosynthesis of D-aspartic acid by Streptococcus faecalis. J. Biol. Chem. 246, 823–830. doi: 10.1016/S0021-9258(18)62483-4
Ranjit, D. K., Moye, Z. D., Rocha, F. G., Ottenberg, G., Nichols, F. C., Kim, H. M., et al. (2022). Characterization of a bacterial kinase that phosphorylates Dihydrosphingosine to form dhS1P. Microbiol. Spectr. 10, e00002–e00022. doi: 10.1128/spectrum.00002-22
Reith, J., Berking, A., and Mayer, C. (2011). Characterization of an N-acetylmuramic acid/N-acetylglucosamine kinase of clostridium acetobutylicum. J. Bacteriol. 193, 5386–5392. doi: 10.1128/JB.05514-11
Robinson, J. I., Weir, W. H., Crowley, J. R., Hink, T., Reske, K. A., Kwon, J. H., et al. (2019). Metabolomic networks connect host-microbiome processes to human Clostridioides difficile infections. J. Clin. Invest. 129, 3792–3806. doi: 10.1172/JCI126905
Rosenberg, C. R., Fang, X., and Allison, K. R. (2020). Potentiating aminoglycoside antibiotics to reduce their toxic side effects. PLoS One 15:e0237948. doi: 10.1371/journal.pone.0237948
Salton, M. R., and Schmitt, M. D. (1967). Effects of diphenylamine on carotenoids and menaquinones in bacterial membranes. Biochim. Biophys. Acta 135, 196–207. doi: 10.1016/0005-2736(67)90114-9
Schelli, K., Zhong, F., and Zhu, J. (2017). Comparative metabolomics revealing Staphylococcus aureus metabolic response to different antibiotics. Microb. Biotechnol. 10, 1764–1774. doi: 10.1111/1751-7915.12839
Serganov, A., Huang, L., and Patel, D. J. J. N. (2008). Structural insights into amino acid binding and gene control by a lysine riboswitch. Nature. 455, 1263–1267. doi: 10.1038/nature07326
Somerville, J. E., Cassiano, L., Bainbridge, B., Cunningham, M. D., and Darveau, R. P. (1996). A novel Escherichia coli lipid a mutant that produces an antiinflammatory lipopolysaccharide. J. Clin. Investig. 97, 359–365. doi: 10.1172/JCI118423”10.1172/JCI118423
Stahl, R. S., Bisha, B., Mahapatra, S., and Chandler, J. C. (2020). A model for the prediction of antimicrobial resistance in Escherichia coli based on a comparative evaluation of fatty acid profiles. Diagn. Microbiol. Infect. Dis. 96:114966. doi: 10.1016/j.diagmicrobio.2019.114966
Su, H. C., Ramkissoon, K., Doolittle, J., Clark, M., Khatun, J., Secrest, A., et al. (2010). The development of ciprofloxacin resistance in Pseudomonas aeruginosa involves multiple response stages and multiple proteins. Antimicrob. Agents Chemother. 54, 4626–4635. doi: 10.1128/AAC.00762-10
Tan, R., Jin, M., Shao, Y., Yin, J., Li, H., Chen, T., et al. (2022). High-sugar, high-fat, and high-protein diets promote antibiotic resistance gene spreading in the mouse intestinal microbiota. Gut Microbes 14:2022442. doi: 10.1080/19490976.2021.2022442
Tang, J. (2011). Microbial metabolomics. Curr. Genomics 12, 391–403. doi: 10.2174/138920211797248619
Teufel, R., Mascaraque, V., Ismail, W., Voss, M., Perera, J., Eisenreich, W., et al. (2010). Bacterial phenylalanine and phenylacetate catabolic pathway revealed. Proc. Natl. Acad. Sci. U. S. A. 107, 14390–14395. doi: 10.1073/pnas.1005399107
Thomas, N., Thorn, C., Richter, K., Thierry, B., and Prestidge, C. (2016). Efficacy of poly-lactic-co-glycolic acid micro- and nanoparticles of ciprofloxacin against bacterial biofilms. J. Pharm. Sci. 105, 3115–3122. doi: 10.1016/j.xphs.2016.06.022
Usuda, Y., and Kurahashi, O. (2005). Effects of deregulation of methionine biosynthesis on methionine excretion in Escherichia coli. Appl. Environ. Microbiol. 71, 3228–3234. doi: 10.1128/AEM.71.6.3228-3234.2005
Vanaporn, M., and Titball, R. W. (2020). Trehalose and bacterial virulence. Virulence 11, 1192–1202. doi: 10.1080/21505594.2020.1809326
Vazquez-Guillamet, C., and Kollef, M. H. (2014). Treatment of gram-positive infections in critically ill patients. BMC Infect. Dis. 14:92. doi: 10.1186/1471-2334-14-92
Vetter, A. M., Helmecke, J., Schomburg, D., and Neumann-Schaal, M. (2019). The impact of pyroglutamate: Sulfolobus acidocaldarius has a growth advantage over Saccharolobus solfataricus in glutamate-containing media. Archaea. 3208051. doi: 10.1155/2019/3208051
Viola, R. E., Zhao, M., Blumenthal, R. M., Wijayasinghe, Y. S., and Bhansali, P. (2015). A surprising range of modified-methionyl S-adenosylmethionine analogues support bacterial growth. Microbiology (Reading) 161, 674–682. doi: 10.1099/mic.0.000034
Vollmer, W., Blanot, D., and de Pedro, M. A. (2008). Peptidoglycan structure and architecture. FEMS Microbiol. Rev. 32, 149–167. doi: 10.1111/j.1574-6976.2007.00094.x
Wang, Q., Lin, M., Shen, P., and Guan, Y. (2021). Elevation of fatty acid biosynthesis metabolism contributes to Zhongshengmycin resistance in Xanthomonas oryzae. Antibiotics (Basel) 10:1166. doi: 10.3390/antibiotics10101166
Wang, Q., Wang, H., Lv, M., Wang, X., and Chen, L. (2023). Sulfamethoxazole degradation by Aeromonas caviae and co-metabolism by the mixed bacteria. Chemosphere 317:137882. doi: 10.1016/j.chemosphere.2023.137882
Wang, Y., Ren, B., Zhou, X., Liu, S., Zhou, Y., Li, B., et al. (2017). Growth and adherence of Staphylococcus aureus were enhanced through the PGE2 produced by the activated COX-2/PGE2 pathway of infected oral epithelial cells 12:e0177166. doi: 10.1371/journal.pone.0177166
Whiteley, A. T., Garelis, N. E., Peterson, B. N., Choi, P. H., Tong, L., Woodward, J. J., et al. (2017). C-di-AMP modulates L isteria monocytogenes central metabolism to regulate growth, antibiotic resistance and osmoregulation 104, 212–233. doi: 10.1111/mmi.13622
Whitmore, S. E., and Lamont, R. J. (2012). Tyrosine phosphorylation and bacterial virulence. Int. J. Oral Sci. 4, 1–6. doi: 10.1038/ijos.2012.6
WHO (2020). Lack of new antibiotics threatens global efforts to contain drug-resistant infections. [cited 2020 10 9 22]. Available from: https://www.who.int/news/item/17-01-2020-lack-of-new-antibiotics-threatens-global-efforts-to-contain-drug-resistant-infections.
WHO (2021). Antimicrobial resistance. [cited 2022 13 9 22]. Available from: https://www.who.int/news-room/fact-sheets/detail/antimicrobial-resistance.
Wolucka, B. A. J. T. F. J. (2008). Biosynthesis of D-arabinose in mycobacteria–a novel bacterial pathway with implications for antimycobacterial therapy 275, 2691–2711. doi: 10.1111/j.1742-4658.2008.06395.x,
Xiao, J. F., Zhou, B., and Ressom, H. W. (2012). Metabolite identification and quantitation in LC-MS/MS-based metabolomics. Trends Anal. Chem. 32, 1–14. doi: 10.1016/j.trac.2011.08.009
Yao, Z., Li, W., Lin, Y., Wu, Q., Yu, F., and Lin, W. (2016). Proteomic analysis reveals that metabolic flows affect the susceptibility of Aeromonas hydrophila to antibiotics. Sci. Rep. 6:39413. doi: 10.1038/srep39413
Ye, J. Z., Lin, X. M., Cheng, Z. X., Su, Y. B., Li, W. X., Ali, F. M., et al. (2018). Identification and efficacy of glycine, serine and threonine metabolism in potentiating kanamycin-mediated killing of Edwardsiella piscicida. J. Proteome 183, 34–44. doi: 10.1016/j.jprot.2018.05.006
Yong, Y., Zhou, Y., Liu, K., Liu, G., Wu, L., and Fang, B. (2021). Exogenous Citrulline and glutamine contribute to reverse the resistance of salmonella to Apramycin. Front. Microbiol. 12:759170. doi: 10.3389/fmicb.2021.759170
Zampieri, M., Enke, T., Chubukov, V., Ricci, V., Piddock, L., and Sauer, U. (2017). Metabolic constraints on the evolution of antibiotic resistance. Mol. Syst. Biol. 13:917. doi: 10.15252/msb.20167028
Zhang, P., Lu, G., Sun, Y., Yan, Z., Dang, T., and Liu, J. (2022). Metagenomic analysis explores the interaction of aged microplastics and roxithromycin on gut microbiota and antibiotic resistance genes of Carassius auratus. J. Hazard. Mater. 425:127773. doi: 10.1016/j.jhazmat.2021.127773
Zhang, S., and Zhu, J. (2022). Untargeted metabolomics sensitively differentiates gut bacterial species in single culture and co-culture systems. ACS Omega 7, 14643–14652. doi: 10.1021/acsomega.1c07114
Zhang, X. X., Ritchie, S. R., and Rainey, P. B. (2014). Urocanate as a potential signaling molecule for bacterial recognition of eukaryotic hosts. Cell. Mol. Life Sci. 71, 541–547. doi: 10.1007/s00018-013-1527-6
Keywords: antimicrobial resistance, Staphylococcus aureus, Gram-positive, metabolites extraction method, freeze-thaw cycle, sonication cycle
Citation: Singh R, Thakur L, Kumar A, Singh S, Kumar S, Kumar M, Kumar Y and Kumar N (2023) Comparison of freeze-thaw and sonication cycle-based methods for extracting AMR-associated metabolites from Staphylococcus aureus. Front. Microbiol. 14:1152162. doi: 10.3389/fmicb.2023.1152162
Edited by:
Vijay Soni, Weill Cornell Medical Center, United StatesReviewed by:
Arka Banerjee, Weill Cornell Medical Center, United StatesBrendon Lee, Cornell University, United States
Bhupendra Singh Rawat, Rutgers University, Newark, United States
Saurabh Mishra, Cornell University, United States
Copyright © 2023 Singh, Thakur, Kumar, Singh, Kumar, Kumar, Kumar and Kumar. This is an open-access article distributed under the terms of the Creative Commons Attribution License (CC BY). The use, distribution or reproduction in other forums is permitted, provided the original author(s) and the copyright owner(s) are credited and that the original publication in this journal is cited, in accordance with accepted academic practice. No use, distribution or reproduction is permitted which does not comply with these terms.
*Correspondence: Yashwant Kumar, y.kumar@thsti.res.in; Niraj Kumar, nkumar@thsti.res.in
†These authors have contributed equally to this work