Toward a Universal Theoretical Framework to Understand Robustness and Resilience: From Cells to Systems
- 1Department of Biology, Radford University, Radford, VA, United States
- 2Department of Environmental Studies and Sciences, Siena College, Loudonville, NY, United States
- 3Department of Biology, University of Alabama at Birmingham, Birmingham, AL, United States
- 4Department of Natural Ecology Resource and Management, Oklahoma State University, Stillwater, OK, United States
Research across a range of biological subdisciplines and scales, ranging from molecular to ecosystemic, provides ample evidence that living systems generally exhibit both a degree of resistance to disruption and an ability to recover following disturbance. Not only do mechanisms of robustness and resilience exist across and between systems, but those mechanisms exhibit ubiquitous and scalable commonalities in pattern and function. Mechanisms such as redundancy, plasticity, interconnectivity, and coordination of subunits appear to be crucial internal players in the determination of stability. Similarly, factors external to the system such as the amplitude, frequency, and predictability of disruptors, or the prevalence of key limiting resources, may constrain pathways of response. In the face of a rapidly changing environment, there is a pressing need to develop a common framework for describing, assessing, and predicting robustness and resilience within and across living systems.
Introduction
Nearly all ecosystems are subject to periodic disturbances by natural stresses, such as flood, fire, drought, excessive heat or cold, predation, pathogen infection, and insect infestation, which exist within continua of intensity and duration and often interact with each other (Johnstone et al., 2016; Stevens-Rumann et al., 2018). Understanding how ecosystems respond to various disturbances and stresses and persist in a changing environment is a central scientific question (Kitano, 2007; Donohue et al., 2016; Urruty et al., 2016; Bradford et al., 2019; Kéfi et al., 2019; Yang et al., 2019).
The concepts of biological robustness and resilience are widely used in the scientific literature (Holling, 1973; Standish et al., 2014), although there is considerable confusion about their meanings. We listed definitions of the majority of technical terms in Table 1. We define robustness as the ability of a living system to survive disturbances largely intact. In contrast, the resilience of a living system is the ability of that system to withstand stress and be restored after disturbance without losing its integral structure and function (Desjardins et al., 2015). Understanding how systems persist over time in response to changing conditions is essential (Gunderson, 2000). This knowledge will provide novel insights into fundamental rules of organization and adaptability in biological systems. Principles of robustness and resilience operate at systems of different spatial and temporal scales, from the micro-scale of individual cells, their organelles and signaling networks to the macro-scale of whole organisms and their communities, culminating in mega-scale systems such as landscapes and the entire biosphere (Baho et al., 2017; Felton and Smith, 2017; Kristensen et al., 2018). The genetic code, the very basic signature of life as we know it, exhibits a degree of robustness that precisely specifies a mapping between DNA and proteins but has inbuilt redundancy and error correction mechanisms that provide resiliency and make genomes stable yet evolvable over millions of years (Koonin and Novozhilov, 2017). Similar pathways of redundancy and self-correction can be found in many homeostatic regulatory systems such as those involved in the regulation of blood pressure, which in vertebrates incorporates a variety of near-redundant neuroendocrine signals that create resilience through the incorporation of interlocking, self-regulating, and self-correcting features. Such commonalities across scale indicate that robustness and resilience may be powerfully selected for within many living systems. Additionally, components of biological systems interact not only with a given unit but also display strong connectivity and information flow across systems at multiple levels (Gao et al., 2016).
While the concept of scalability has been proposed in the context of biological robustness and resilience, a number of key questions still remain. What are the key connections that influence robustness and resilience within and across space, time, and scale? How can we advance our understanding of systems connections and interconnections across such complex and disparate interacting scales?
We present an example of how one type of disturbance—heat stress—affects biological systems across scales, from responses on the cellular and organismic, to population, community, ecosystem, and biosphere levels. We use this example to demonstrate that there is a commonality in the ways that biological and ecological systems respond to various stressors, and those reactions translate across levels of biological organization and scale. We identify barriers and challenges that currently limit our understanding of key mechanisms and pathways that influence cross-scalar interactions and processes. We argue that with the advancement of cross-scalar modeling approaches and network science, coupled with improved communication and collaboration among scientists from various biological subdisciplines, significant progress in understanding key underlying mechanisms and pathways that drive ecosystem robustness and resilience can be achieved.
Robustness and Resilience of Heat Stress Responses Across Scales
Few factors are more critical to the workings of evolution than stress, and the biological response to stress largely relies on the mechanisms of robustness and resilience (Côté et al., 2016). For example, the capacity to respond to heat stress is one of the most universal and evolutionarily conserved cellular stress signaling pathways (Richter et al., 2010; Gardner et al., 2013). The heat stress response has formed as a result of natural selection, improving the capacity of cells, organisms, and populations to withstand situations that require action. In eukaryotic cells, heat exposure triggers a multitude of responses that collectively upregulate a suite of molecular chaperones, such as Heat Shock Proteins (HSPs), to assist with protein folding and stress recovery, maintaining cell viability and ensuring robustness (Gardner et al., 2013; Afrin et al., 2020). While such adaptive responses may be triggered at moderately increased temperatures without leading to a change of cells’ fate, a true resilience-based response will allow the cell to cope with heat shock perturbation, recover, and develop tolerance to the next perturbation (Smirnova et al., 2015). At the molecular level, cellular signaling ensues that initiates stable changes in the genome (epigenetic memory) within the affected cell itself and/or adjacent cells/tissues; a phenomenon dubbed “epigenetic scarring” that is now considered to be the molecular basis for cellular resilience. For instance, the progeny of the heat-primed wheat plants exhibited elevated tolerance to high temperature stress as evidenced by the changes in the epigenetic marks (Wang et al., 2016).
Moreover, the “cellular thermometer” model has been proposed to connect the molecular and ecological dimensions of heat shock response, first outlined by Craig and Gross (1991). Heat Shock Proteins, especially HSP60 and HSP70, have been used as biomarkers in studies on acclimation, thermal tolerance and anticipatory resilience of a range of algae, invertebrates, fish, and higher vertebrates (Dietz and Somero, 1992; Roberts et al., 1997; Buckley et al., 2001; Tedeschi et al., 2015).
At the organismic level robustness and resilience are tightly connected to the concepts of stress, homeostasis, and allostasis (McEwen et al., 2015). We may see robustness in an organism that, when faced with increased environmental temperatures, maintains a relatively constant internal temperature through means of alterations in its physiology, such as sweating or vasodilation, or behavior, such as shade-seeking or burrowing (Bernabucci et al., 2010). The same organism may show resilience after facing heat stress by means of recovery through regrowth (Kojima et al., 2007), reallocation of internal resources to compensate for damaged tissues (Klepsatel et al., 2016) or shifts in behavior to acquire new resources and avoid additional energy expenditures (Yusishen et al., 2020).
How an individual responds to heat stress can alter the dynamics at the population, community, and ecosystem levels simultaneously. For instance, some corals are robust to warming temperatures owing to unusual algal symbionts, which enable them to survive thermal stress, while other corals that lack those unusual algal symbionts die (Baker et al., 2004). Similarly, the presence of a diverse suite of algal symbionts within a coral reef may contribute to greater resilience of the ecosystem to a variable array of thermal stresses (Berkelmans and Van Oppen, 2006). The ability of some corals to survive thermal perturbation may allow the entire community and ecosystem to remain robust. In contrast, for a coral population that lacks any of the thermally-resistant algal symbionts, thermal stress may result in a major disruption in the community structure and ecosystem function. More generally, how environmental stressors influence population dynamics could be predicted via understanding individual responses.
These examples of heat stress response at various scales illustrate that commonalities and connections exist in systems within and across scales. Some of the paradigms that have been proposed to be particularly useful for conceptually unifying the trans-scalar properties of systems are: (1) division of labor and spatiotemporal organization of production and exchange, such as the presence of cellular compartments, limb diversification in arthropods, or different tasks performed by different ant workers within the nest (Rueffler et al., 2012); (2) systemic homeostasis, achieved by balancing the economic efficiency and adaptability; while the former requires adequate and stable organization, the latter is best supported by flexibility and change (Kurakin, 2009); (3) “small-world” network (SWN) propensity, where high local clustering of nodes is accompanied by short average path length between any two nodes. The emergence of SWN patterns has been described to occur within biochemical pathways in the cell, the brain neural network or ecological food webs (Telesford et al., 2011; Jarman et al., 2017); and (4) trade-off effects on growth and fitness at the cost of a heightened stress response. For example, an activated heat stress response or pathogen infection reduces overall growth due to the high metabolic cost of stress signaling and halted protein translation. Similarly, behavioral responses in avoiding excessive heat by mammals leads to the selection of cooler habitats and decreasing locomotion for foraging activities, even if that causes sub-par foraging opportunities resulting in lower food uptake (Pajerowska-Mukhtar et al., 2012; Francesca et al., 2019). Yet, to date, research addressing the robustness and resilience of systems to disturbance or other stressors has largely been conducted at one or a limited number of disconnected scales (Kéfi et al., 2019). As such, the ability to reveal the prevalence and extent of commonalities in response to stressors, and to identify key connections across scales has been hindered.
Current Barriers and Challenges
Given the potential breadth of cross-scalar connections of systems, from molecular to biosphere levels, expertise from various subdisciplines of biological sciences is necessary to advance our understanding of robustness and resilience across scales (Reyer et al., 2015). Yet, as the various subdisciplines of biological sciences have progressed in recent decades, there has been a concomitant increase in the specialized training and analytical methodologies required in those subdisciplines. Communication and collaboration between individuals working in different specialties is further impaired both by a lack of common terminology (Calabrese et al., 2007), lack of compatibility in the format of generated data (Sansone et al., 2012), and a restriction of focus to familiar scales and models. This isolation has been forcing the biological community to continuously “reinvent the wheel”; to develop parallel conceptual frameworks but fail to grasp the similarity, parallelism, and interaction between those frameworks. The specialization in methodology, training, and terminology has led to considerable fragmentation of the subdisciplines. These obstacles to communication and a lack of substantive interactions outside specialized fields have been a major stumbling block to developing a cross-system framework for conceptualizing robustness and resilience.
A second major obstacle has been on the analytical front. The use of analytical techniques has often been specific to the subdiscipline. Some analytical frameworks or techniques tend to become popular in subdisciplines, yet other techniques are used less or are overlooked. Furthermore, as analytical techniques become advanced, it becomes increasingly challenging to master all of the techniques available. A lack of understanding of the variety of analytical tools and frameworks, coupled with the specialization and lack of communication between subdisciplines, have been at the root of limiting our ability to develop an analytical framework and approach to meet the challenges of better understanding robustness and resilience across scales (Gomez-Cabrero et al., 2014).
Solutions
To overcome these barriers we must develop a shared conceptual language and a common set of processes and protocols for assaying robustness and resilience across levels and systems. This will in turn help us to develop new ways to analyze the data and integrate expertise across different biological subdisciplines.
Interdisciplinarity and multi-model integration are essential, in part because the problem itself is so complex. It is anticipated that both robustness and resilience come at a cost of imposing severe constraints on the underlying architecture of a system, which substantially impacts the principles of the general design motifs found in many networks across scales of biological complexity (Beller et al., 2019). In addition, any accurate model must incorporate multi-scale robustness and resilience across multiple scales of size and time and include the dynamic nature of changes and stasis in the face of challenge.
Integrating conceptual paradigms from multiple scales and various sub-disciplines of biology will allow us to map and build a common core of principles. Combining and bridging models in this way will make it possible to address cross-scalar issues and expand our conceptualization of resilience at each constituent level. Systems concepts benefit from exploring how mechanisms that provide resilience at the molecular level interact with similar mechanisms at the organismic level and how both interface with and produce robustness at the population and ecosystem levels. The rapidly growing research on mathematical modeling and its application in life sciences has presented a plethora of new approaches to complex systems modeling and integrative analysis that can be harnessed for a cross-scalar understanding of biological robustness and resilience.
Cross-Scalar Integrative Models
As proposed by Kurakin (2009), common patterns and universal principles can emerge within biological systems at all levels of complexity once they are re-interpreted within alternative paradigms that are based on the concepts of non-equilibrium thermodynamics and economics. For example, as the behaviors of people in an economy are open-ended and never fully predictable, many structural and functional aspects of proteins, cells, organisms, and populations are not predetermined by design but only statistically more likely to be engaged in certain patterns of actions and interactions over other ones. Therefore, all scales of life alike remain flexible and malleable to adaptation, reorganization, and evolution. Data yielded through social-ecological network analyses indicate that different types of perturbations tend to be stabilized by cross-scalar mechanisms (Naylor et al., 2019). Some ecosystems are more robust than others when facing perturbation, but such stability is often predicated on plasticity/at other scales. For example, a hypothetical disease might kill the majority of a population and destabilize an ecosystem. However, if mechanisms exist that produce a variable range of molecular or systemic responses to the disease, some individuals may be more resistant, and such individuals would be most likely to repopulate via the founder effect. As a result of that small-scale variation, the whole population, and the ecosystem beyond it may exhibit robust persistence.
To truly appreciate the mechanisms underlying resilience we need to examine how responses interact across levels. Cross-scalar interactions have been incorporated into social-ecological system modeling (e.g., Robustness Framework) to consider that the capacity and intent of humans can strongly influence the resilience and trajectory of the system (Anderies et al., 2004; Walker et al., 2004; Naylor et al., 2019); however, the number of scales considered in these models is limited. Developing a truly cross-scalar framework will allow us to produce tools to predict system stability, to select essential variables to study through model sensitivity analyses, to generate novel hypotheses, and to modulate that stability. The ability to predict which systems are vulnerable to collapse would allow us to anticipate and interfere with pathways of disease and dysfunction, to better identify resource models that are non-sustainable, and ultimately, to predict and prevent the collapse of threatened species, populations, and environments.
Recent developments have produced a broad range of disruption models across multiple scales and fields; however, models remain largely isolated and lack interconnectivity. Our ability to collect and process very large data sets is at a point where large-scale pattern recognition is feasible in a way that was previously inaccessible. As such, a strong integrative framework for resilience and robustness is both possible and timely.
Spatial Consumer-Resource Models
Recent experimental work has highlighted the improvement made by using a spatial consumer-resource model to predict metapopulation (assemblages of local populations) dynamics in heterogeneous environments (Zhang et al., 2017). Consumer-resource models use a mechanistic, bottom-up approach with explicit resources to explicitly consider the resource inputs and time scales of feedback between organisms and their resource (Zhang et al., 2017). Comparing to the classical logistic equation model, is that it well capture the impact of environmental heterogeneity across multiple levels by scaling up individual behaviors (e.g., individual responses to the environmental change) and interaction between organisms and environments (e.g., resource dynamics). For instance, recent work revealed that with the same dose of stressor, a homogeneous distribution leads to much lower metapopulation abundance than a heterogeneous distribution, when the stressor increases individual death rate and/or simultaneously decreases growth rate and reproduction (Zhang et al., 2020a). As illustrated in Figure 1, communities with a larger number of resilient populations of various species (triangles, diamonds, circles) are more resilient (diversity, functionality) than communities with fewer resistant populations of the same species. Hence, investigating system response and tolerance to external stressors at a higher scale requires a solid mechanistic understanding of the diversity of responses to environmental change among species that contribute to the same ecosystem function (Elmqvist et al., 2003; Walker et al., 2004) and the consumer-resource model can be an appropriate tool to scale up individual resilience to the population, community levels. More importantly, most species in nature disperse across the landscape, which is a critical process of response diversity (Elmqvist et al., 2003) that scales up population dynamics to the metapopulation level (Wang et al., 2015; Zhang et al., 2020b). This consumer-resource model is also an appropriate model system to project the role of dispersal, especially in a heterogeneous environment. Together, this model framework could be a suitable option to understand systems connections and interconnections across complex and disparate interacting scales.
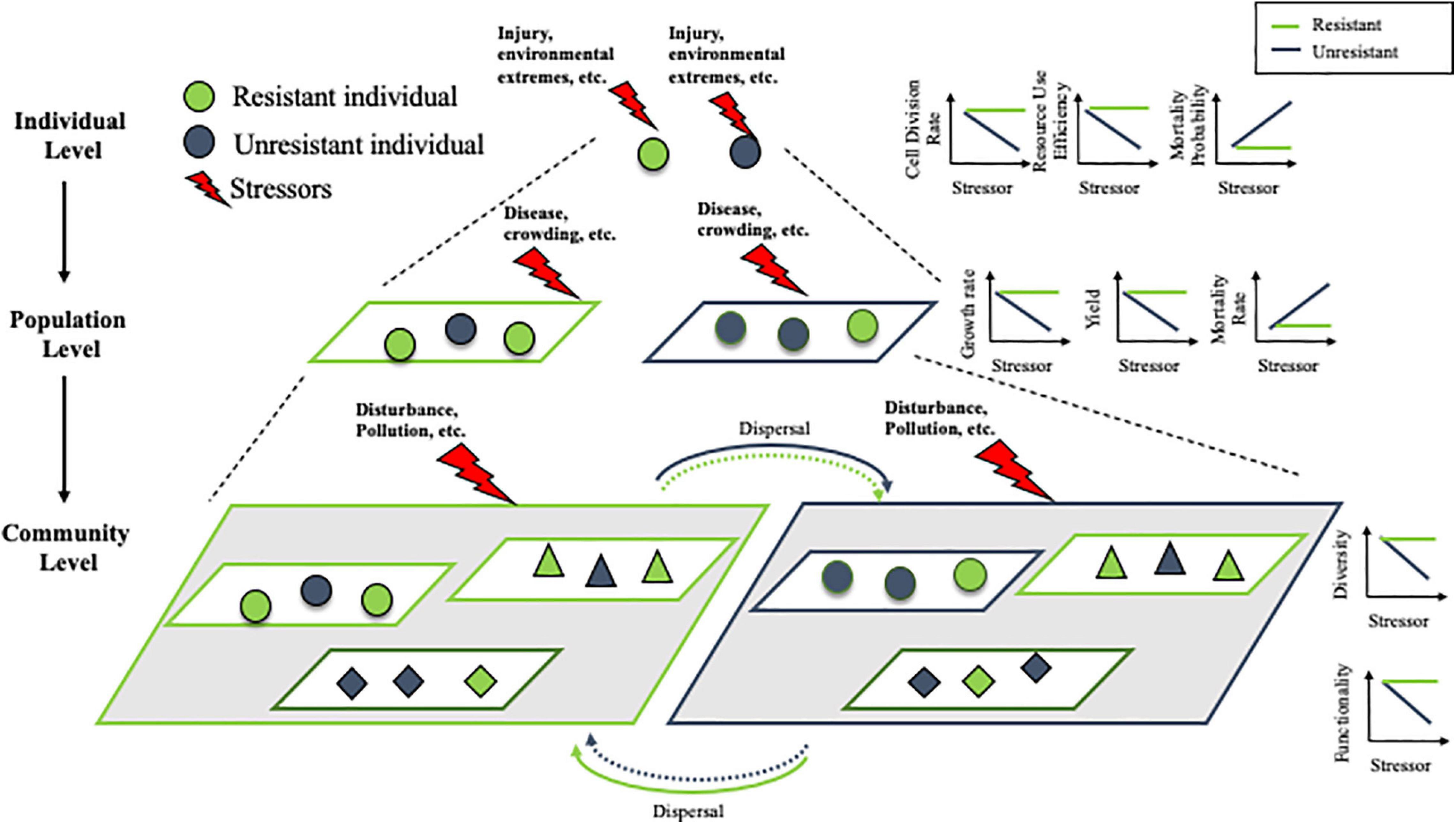
Figure 1. Scaling the effect of stressors from individuals to communities. We hypothesize the resistant individuals (green) are less sensitive to stressor impact at the individual level (cell division rate, resource use efficiency and mortality probability). Populations composed primarily of resistant individuals are similarly more resistant (growth rate, yield, and mortality rate) than populations with a majority of non-resistant (charcoal gray) individuals. This trend continues to scale to the community level, where communities with a larger number of resistant populations of various species (triangles, diamonds, circles) are more resistant (diversity, functionality) than communities with fewer resistant populations of the same species. When there is dispersal between communities, resistant individuals are more likely to disperse into preferable, resistant communities, while non-resistant individuals may be more likely to disperse into suboptimal, non-resistant communities.
Network Science Approaches
The future of modeling biological systems robustness and resilience will also involve multi- and higher-dimensional mathematical models employing theories of network science. A network is composed of a system’s components, which can correspond to genes and gene products or species in cellular and ecological networks, respectively. The relationships (interactions) between the system’s components are termed edges. Network topological features, such as degree, shortest path, and betweenness, provide a valuable source of information for inferring functional patterns of individual nodes (Table 1; Garbutt et al., 2014; Gao et al., 2016).
Those parameters characterizing networks may act as indicators of the “most influential nodes” within a network and prioritize important components in diverse biological systems for building testable hypotheses. As shown in Figure 2, a number of network properties, such as the nodes or edge connectivity, can influence the robustness and resilience of various types of regulatory and protein-protein interaction networks, ranging from the microscale of a cell to the macroscale of an ecosystem (Gardner et al., 2013; Gomez-Cabrero et al., 2014; Ahmed et al., 2018). In addition to connectivity, properties such as diversity, redundancy of nodes, network dynamics, and topology also contribute to the robustness of various networks, from an ecological cascade to cellular signaling pathways.
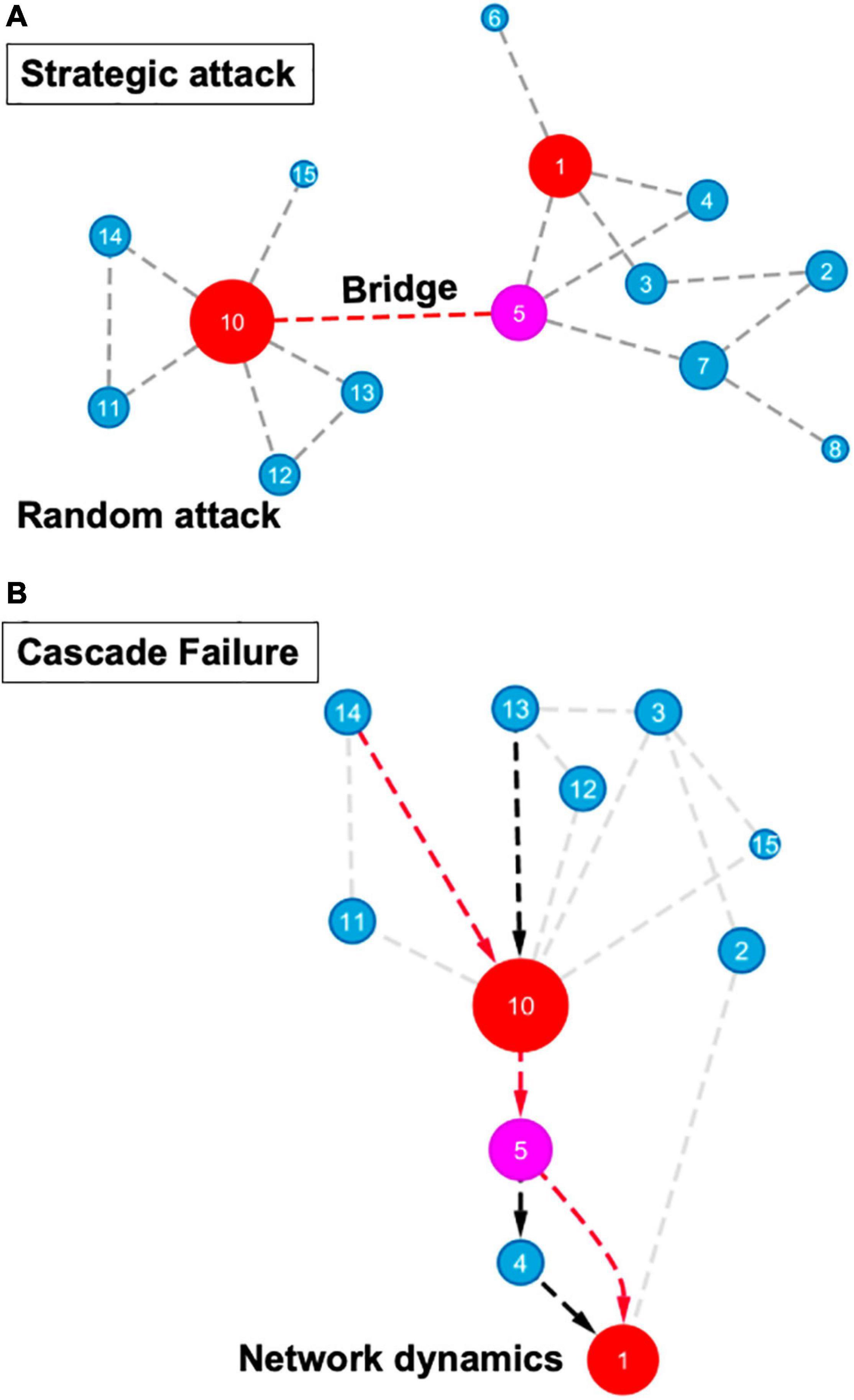
Figure 2. Topological features in network robustness and resilience. (A) Node and edge percolations in strategic or random attacks are displayed. Highly connected nodes (hubs) are represented as red circles, high betweenness node (a bottleneck) is shown in magenta, blue circles represent remaining network nodes. Circle size correlates with node connectivity. A strategic attack involves the disruption of a bridge connecting two high centrality nodes, such as the one located between nodes #5 and #10. The degree of distribution and other connectivity measures are not important for random attacks (such as disruption of an interaction between two blue nodes). (B) Functional or structural redundancy as well as network dynamics to reduce cascade failure are shown. Red arrows indicate the mainstream information flow pathway while the black arrows depict an alternate pathway that allows rewiring information flow in a robust network. Circle colors and sizes correspond to features described in (A).
Perhaps the most powerful way of modeling biological systems’ robustness and resilience involves perturbation of nodes and/or edges. From a network biology standpoint, a complex abiotic or biotic stressor can be viewed as a set of strategic perturbations, which cause a drastic disruption and frequently lead to a failure of a biological system. Modeling of connectivity, edge ranking, and percolation of a specific node and/or an edge can determine the likelihood of system failure, indicating the relative robustness to external disruptions and internal cues in a complex network. Moreover, network science-fueled approaches can help predict the outcome of a random or strategic attack on a network, expose the most vulnerable components and help design systems with enhanced robustness and resilience (Lordan and Albareda-Sambola, 2019; Figure 2). For instance, a strategic attack on hubs or bottlenecks will substantially reduce the number of connections and increase the average path length within a system (Figure 2). The robustness or resilience of a network does not only depend upon the integrity of these modular modes or edges. In addition to connectivity, the diversity of the systems’ components plays an equally important role in the stability of a network. Systems with increased node heterogeneity and incomplete connectivity adjust gradually to change, whereas highly connected networks and homogeneity of the units may remain unchanged in response to various stresses until a “tipping point” is reached (Scheffer et al., 2012). With the perturbation of a node or an edge, a cascade effect may be triggered that can sequentially and systemically spread throughout the entire system causing a catastrophic network failure. Functional and structural redundancies as well as network dynamicity within a system may avoid network failure propagation (Figure 2). In addition, different types of cascading effects, including domino effects and hidden feedbacks, can contribute to network failures and result in network rewiring, regime shifts, and critical transitions, which adds another layer of complexity to the network-science aided modeling of robustness and resilience (Scheffer et al., 2012; Rocha et al., 2018). While cascade failure has been primarily described for social-ecological systems, it’s important to note that cellular systems also follow similar network architecture.
In a true systems-biology fashion, network science approaches will provide the toolbox for integrating data and cross-scalar models across domains and levels of granularity. Such systems-level approaches will help improve the detection of common patterns, as well as predict and simulate complex systems’ behavior to help engineer improved resilience and robustness in biological systems at all scales (Gao et al., 2016; Lordan and Albareda-Sambola, 2019).
Future Directions and Implications
We posit that mechanisms to achieve robustness and resilience are ubiquitous and fundamental aspects of life. Understanding how ecosystems respond and persist in a changing environment is a central scientific question with massive real-world applications. Change is a prerequisite for adaptation, but living systems are also selected to maintain their integrity as unstable systems fail to cohere (Padilla and Tsukimura, 2014). We occupy a point in time where biological systems are faced with an almost unanticipated degree of disruptive challenge (Carnicer et al., 2011; Scheffers et al., 2016; Cohen et al., 2018). As such, a strong integrative framework for resilience and robustness is both timely and urgently needed.
It should be noted that research into pathways and promoters of systemic robusticity is already well established in engineering (Woods, 2015; Nielsen and Keasling, 2016). In several cases, such studies have turned to biological systems for inspiration and insight (Doyle and Csetem, 2005). This work has been, and continues to be, highly productive. However, much of the ongoing work on systemic robusticity and resilience from an engineering perspective has been focused at particular scales of function (Chandra et al., 2011; Wu et al., 2016) with relatively less attention given to trans-scalar integration and interlinked, emergent robusticity. Future inquiries into resilience will benefit greatly not only from explorational modeling of feedback regulation, network pathways and hierarchical organization within systems but also by incorporating the impact of interlocking features across systems operating at various scales of organization. In order to achieve such a deeply integrative perspective, multiple disciplinary perspectives must be actively pursued and encouraged to interact.
Ultimately, the development of an integrative, truly cross-scalar framework mapping robustness and resilience will make it possible to predict system stability, select the most essential variables to study through model sensitivity analyses, generate testable hypotheses, create unification across scales and ultimately, allow us to design more stable, efficient, and resilient systems. Such a model framework would greatly improve both the ability to assess and predict system stability in the face of disruption and provide new tools for turning systems toward resilience equilibrium or move the system away from an undesired or unstable outcome. Novel approaches rooted in system dynamic and hybrid models, which can investigate interactions and feedbacks between the individual involved models, demonstrate a high potential for modeling complex applications to allow prediction of biological adaptation strategies (Kirk et al., 2012; Martin and Schlüter, 2015; Pope and Gimblett, 2015; Wang et al., 2016). This effort has direct implications for moderating the impact of anthropogenic disturbance and climate change at both local and global levels. A cross-scalar framework could be used to predict ecosystemic responses to drought, changes in salinity, temperature disruptions, invasive species, and loss of key species. Similarly, such a framework could be used to model changes in microbiomes both in soil and water and within other living organisms, providing useful applications for both agriculture and epidemiology.
Conclusion
Our comprehension of projecting system robustness and resilience has largely advanced in the last few years; yet, understanding the rules of synthesizing system robustness and resilience across different spatial and temporal scales remains to be a challenge. This study highlighted major barriers and roadblocks and suggested some model systems that can help overcome those barriers, ultimately advancing our current understanding of cross-scalar robustness and resilience. Furthermore, this appreciation for common mechanistic underpinnings of robustness and resilience will provide a deeper understanding of how life persists and evolves. We posit that the development of common tools and terminology will be necessary to allow for seamless integration of the vast datasets obtained from sources across scales of biological organization and to help inform future research directions. To address questions of this significance and transdisciplinary we must make a variety of changes not only to funding institutions but to our academic culture. Additionally, we must work to develop a well-balanced and distributed yet collaborative network of integrated specialists in which experts from various fields are encouraged, aided in, and rewarded for communicating with one another, and wherein multiple types of expertise and perspective are valued for their inherent diversity. Hence, we believe that the time is right for a new framework of training and collaboration of life science experts representing multiple disciplines that will promote a cultural shift to stimulate diversity and inclusion.
Data Availability Statement
The original contributions presented in the study are included in the article/supplementary material, further inquiries can be directed to the corresponding author/s.
Author Contributions
All authors actively participated in developing the concepts and writing the manuscript.
Funding
A grant from the National Science Foundation (EF-1940791) supported a “Reintegrating Biology Jumpstart” workshop that fostered this collaborative work. KP-M was funded by the National Science Foundation CAREER award (IOS-1350244). BZ was supported by McIntire-Stennis funds, Oklahoma State University.
Conflict of Interest
The authors declare that the research was conducted in the absence of any commercial or financial relationships that could be construed as a potential conflict of interest.
Acknowledgments
We thank Dr. Shahid Mukhtar for helpful discussions on network biology and Dr. Sarah Foltz for advice relating to anthropogenic disturbance and scaling flow, and appreciate the valuable feedback from the editor and reviewers that strengthened the manuscript. We also thank the National Science Foundation (NSF) funded project (Reintegrating Biology) for organizing the collaborative workshop.
References
Afrin, T., Diwan, D., Sahawneh, K., and Pajerowska-Mukhtar, K. (2020). Multilevel regulation of endoplasmic reticulum stress responses in plants: where old roads and new paths meet. J. Exp. Bot. 71, 1659–1667. doi: 10.1093/jxb/erz487
Ahmed, H., Howton, T., Sun, Y., Weinberger, N., Belkhadir, Y., and Mukhtar, M. S. (2018). Network biology discovers pathogen contact points in host protein-protein interactomes. Nat. Commun. 9:2312.
Anderies, J. M., Janssen, M. A., and Ostrom, E. (2004). A framework to analyze the robustness of social-ecological systems from an institutional perspective. Ecol. Soc. 9:18.
Baho, D. L., Allen, C. R., Garmestani, A. S., Fried-Petersen, H. B., Renes, S. E., Gunderson, L. H., et al. (2017). A quantitative framework for assessing ecological resilience. Ecol. Soc. 22:17.
Baker, A. C., Starger, C. J., McClanahan, T. R., and Glynn, P. W. (2004). Coral reefs: corals’ adaptive response to climate change. Nature 430, 741–741. doi: 10.1038/430741a
Beller, E. E., Spotswood, E. N., Robinson, A. H., Anderson, M. G., Higgs, E. S., Hobbs, R. J., et al. (2019). Building ecological resilience in highly modified landscapes. Bioscience 69, 80–92. doi: 10.1093/biosci/biy117
Berkelmans, R., and Van Oppen, M. J. (2006). The role of zooxanthellae in the thermal tolerance of corals: a ‘nugget of hope’ for coral reefs in an era of climate change. Proc. R. Soc. B Biol. Sci. 273, 2305–2312. doi: 10.1098/rspb.2006.3567
Bernabucci, U., Lacetera, N., Baumgard, L. H., Rhoads, R. P., Ronchi, B., and Nardone, A. (2010). Metabolic and hormonal acclimation to heat stress in domesticated ruminants. Animal 4, 1167–1183. doi: 10.1017/s175173111000090x
Bradford, J., Shlaepfer, D. R., Lauenroth, W. K., Palmquist, K. A., Chambers, J. C., and Maestas, J. D. (2019). Climate-driven shifts in soil temperature and moisture regimes suggest opportunities to enhance assessments of dryland resilience and resistance. Front. Ecol. Evol. 7:358. doi: 10.3389/fevo.2019.00358
Buckley, B. A., Owen, M. E., and Hofmann, G. E. (2001). Adjusting the thermostat: the threshold induction temperature for the heat-shock response in intertidal mussels (genus Mytilus) changes as a function of thermal history. J. Exp. Biol. 204, 3571–3579.
Calabrese, E. J., Bachmann, K. A., Bailer, A. J., Bolger, P. M., Borak, J., Cai, L., et al. (2007). Biological stress response terminology: integrating the concepts of adaptive response and preconditioning stress within a hormetic dose–response framework. Toxicol. Appl. Pharmacol. 222, 122–128.
Carnicer, J., Coll, M., Ninyerola, M., Pons, X., Sánchez, G., and Peñuelas, J. (2011). Widespread crown condition decline, food web disruption, and amplified tree mortality with increased climate change-type drought. Proc. Natl. Acad. Sci. U.S.A. 108, 1474–1478. doi: 10.1073/pnas.1010070108
Chandra, F. A., Buzi, G., and Doyle, J. C. (2011). Glycolytic oscillations and limits on robust efficiency. Science 333, 187–192. doi: 10.1126/science.1200705
Cohen, J. M., Lajeunesse, M. J., and Rohr, J. R. (2018). A global synthesis of animal phenological responses to climate change. Nat. Clim. Change 8, 224–228. doi: 10.1038/s41558-018-0067-3
Côté, I. M., Darling, E. S., and Brown, C. J. (2016). Interactions among ecosystem stressors and their importance in conservation. Proc. R. Soc. B Biol. Sci. 283:20152592. doi: 10.1098/rspb.2015.2592
Craig, E. A., and Gross, C. A. (1991). Is hsp70 the cellular thermometer? Trends Biochem. Sci. 16, 135–140. doi: 10.1016/0968-0004(91)90055-z
Desjardins, E., Barker, G., Lindo, Z., Dieleman, C., and Dussault, A. C. (2015). Promoting resilience. Q. Rev. Biol. 90, 147–165.
Dietz, T. J., and Somero, G. N. (1992). The threshold induction temperature of the 90-kDa heat shock protein is subject to acclimatization in eurythermal goby fishes (genus Gillichthys). Proc. Natl. Acad. Sci. U.S.A. 89, 3389–3393. doi: 10.1073/pnas.89.8.3389
Donohue, I., Hillebrand, H., Montoya, J. M., Petchey, O. L., Pimm, S. L., Fowler, M. S., et al. (2016). Navigating the complexity of ecological stability. Ecol. Lett. 19, 1172–1185.
Doyle, J., and Csetem, M. (2005). Motifs, control, and stability. PLoS Biol. 3:e392. doi: 10.1371/journal.pbio.0030392
Elmqvist, T., Folke, C., Nyström, M., Peterson, G., Bengtsson, J., and Walker, B. (2003). Response diversity, ecosystem change, and resilience. Front. Ecol. Environ. 1, 488–494. doi: 10.1890/1540-9295(2003)001[0488:rdecar]2.0.co;2
Felton, A. J., and Smith, M. D. (2017). Integrating plant ecological responses to climate extremes from individual to ecosystem levels. Philos. Trans. R. Soc. B Biol. Sci. 372:20160142. doi: 10.1098/rstb.2016.0142
Francesca, B., Milena, Z., Stefano, G., Apollonio, M., and Simone, C. (2019). Forecasting the response to global warming in a heat-sensitive species. Sci. Rep. 9:3048.
Gao, J., Barzel, B., and Barabási, A. L. (2016). Universal resilience patterns in complex networks. Nature 530, 307–312. doi: 10.1038/nature16948
Garbutt, C. C., Bangalore, P. V., Kannar, P., and Mukhtar, M. (2014). Getting to the edge: protein dynamical networks as a new frontier in plant–microbe interactions. Front. Plant Sci. 5:312. doi: 10.3389/fpls.2014.00312
Gardner, B. M., Pincus, D., Gotthardt, K., Gallagher, C. M., and Walter, P. (2013). Endoplasmic reticulum stress sensing in the unfolded protein response. Cold Spring Harb. Perspect. Biol. 5:a013169.
Gomez-Cabrero, D., Abugessaisa, I., Maier, D., Teschendorff, A., Merkenschlager, M., Gisel, A., et al. (2014). Data Integration in the Era of Omics: Current and Future Challenges. London: BioMed Central.
Gunderson, L. H. (2000). Ecological resilience—in theory and application. Annu. Rev. Ecol. Syst. 31, 425–439. doi: 10.1146/annurev.ecolsys.31.1.425
Holling, C. S. (1973). Resilience and stability of ecological systems. Annu. Rev. Ecol. Syst. 4, 1–23. doi: 10.1007/978-3-030-54560-4_1
Jarman, N., Steur, E., Trengove, C., Tyukin, I. Y., and van Leeuwen, C. (2017). Self-organisation of small-world networks by adaptive rewiring in response to graph diffusion. Sci. Rep. 7:13158.
Johnstone, J. F., Allen, C. D., Franklin, J. F., Frelich, L. E., Harvey, B. J., Higuera, P. E., et al. (2016). Changing disturbance regimes, ecological memory, and forest resilience. Front. Ecol. Environ. 14, 369–378. doi: 10.1002/fee.1311
Kéfi, S., Domínguez-García, V., Donohue, I., Fontaine, C., Thébault, E., and Dakos, V. (2019). Advancing our understanding of ecological stability. Ecol. Lett. 22, 1349–1356. doi: 10.1111/ele.13340
Kirk, P., Griffin, J. E., Savage, R. S., Ghahramani, Z., and Wild, D. L. (2012). Bayesian correlated clustering to integrate multiple datasets. Bioinformatics 28, 3290–3297. doi: 10.1093/bioinformatics/bts595
Kitano, H. (2007). Towards a theory of biological robustness. Mol. Syst. Biol. 3:137. doi: 10.1038/msb4100179
Klepsatel, P., Gáliková, M., Xu, Y., and Kühnlein, R. P. (2016). Thermal stress depletes energy reserves in Drosophila. Sci. Rep. 6:33667.
Kojima, A., Goto, K., Morioka, S., Naito, T., Akema, T., Fujiya, H., et al. (2007). Heat stress facilitates the regeneration of injured skeletal muscle in rats. J. Orthop. Sci. 12, 74–82. doi: 10.1007/s00776-006-1083-0
Koonin, E. V., and Novozhilov, A. S. (2017). Origin and evolution of the universal genetic code. Annu. Rev. Genet. 51, 45–62.
Kristensen, T. N., Ketola, T., and Kronholm, I. (2018). Adaptation to environmental stress at different timescales. Ann. N. Y. Acad. Sci. 1476, 5–22. doi: 10.1111/nyas.13974
Kurakin, A. (2009). Scale-free flow of life: on the biology, economics, and physics of the cell. Theor. Biol. Med. Model. 6:6.
Lordan, O., and Albareda-Sambola, M. (2019). Exact calculation of network robustness. Reliabil. Eng. Syst. Saf. 183, 276–280. doi: 10.1016/j.ress.2018.11.020
Martin, R., and Schlüter, M. (2015). Combining system dynamics and agent-based modeling to analyze social-ecological interactions-an example from modeling restoration of a shallow lake. Front. Environ. Sci. 3:66. doi: 10.3389/fenvs.2015.00066
McEwen, B. S., Gray, J. D., and Nasca, C. (2015). Recognizing resilience: learning from the effects of stress on the brain. Neurobiol. Stress 1, 1–11. doi: 10.1016/j.ynstr.2014.09.001
Naylor, L. A., Brady, U., Quinn, T., Brown, K., and Anderies, J. M. (2019). A multiscale analysis of social-ecological system robustness and vulnerability in Cornwall, UK. Reg. Environ. Change 19, 1835–1848. doi: 10.1007/s10113-019-01530-7
Nielsen, J., and Keasling, J. D. (2016). Engineering cellular metabolism. Cell 164, 1185–1197. doi: 10.1016/j.cell.2016.02.004
Padilla, D. K., and Tsukimura, B. (2014). A new organismal systems biology: how animals walk the tight rope between stability and change. Am. Zool. 54, 218–222. doi: 10.1093/icb/icu038
Pajerowska-Mukhtar, K. M., Wang, W., Tada, Y., Oka, N., Tucker, C. L., Fonseca, J. P., et al. (2012). The HSF-like transcription factor TBF1 is a major molecular switch for plant growth-to-defense transition. Curr. Biol. 22, 103–112. doi: 10.1016/j.cub.2011.12.015
Pope, A. J., and Gimblett, R. (2015). Linking Bayesian and agent-based models to simulate complex social-ecological systems in semi-arid regions. Front. Environ. Sci. 3:55. doi: 10.3389/fenvs.2015.00055
Reyer, C. P., Brouwers, N., Rammig, A., Brook, B. W., Epila, J., Grant, R. F., et al. (2015). Forest resilience and tipping points at different spatio-temporal scales: approaches and challenges. J. Ecol. 103, 5–15. doi: 10.1111/1365-2745.12337
Richter, K., Haslbeck, M., and Buchner, J. (2010). The heat shock response: life on the verge of death. Mol. Cell 40, 253–266. doi: 10.1016/j.molcel.2010.10.006
Roberts, D. A., Hofmann, G. E., and Somero, G. N. (1997). Heat-shock protein expression in Mytilus californianus: acclimatization (seasonal and tidal-height comparisons) and acclimation effects. Biol. Bull. 192, 309–320. doi: 10.2307/1542724
Rocha, J. C., Peterson, G., Bodin, Ö., and Levin, S. (2018). Cascading regime shifts within and across scales. Science 362, 1379–1383. doi: 10.1126/science.aat7850
Rueffler, C., Hermisson, J., and Wagner, G. P. (2012). Evolution of functional specialization and division of labor. Proc. Natl. Acad. Sci. U.S.A. 109, E326–E335.
Sansone, S. A., Rocca-Serra, P., Field, D., Maguire, E., Taylor, C., Hofmann, O., et al. (2012). Toward interoperable bioscience data. Nat. Genet. 44, 121–126.
Scheffer, M., Carpenter, S. R., Lenton, T. M., Bascompte, J., Brock, W., and Dakos, V. (2012). Anticipating critical transitions. Science 338, 344–348.
Scheffers, B. R., De Meester, L., Bridge, T. C., Hoffmann, A. A., Pandolfi, J. M., Corlett, R. T., et al. (2016). The broad footprint of climate change from genes to biomes to people. Science 354:aaf7671. doi: 10.1126/science.aaf7671
Smirnova, L., Harris, G., Leist, M., and Hartung, T. (2015). Cellular resilience. ALTEX 32, 247–260. doi: 10.14573/altex.1509271
Standish, R. J., Hobbs, R. J., Mayfield, M. M., Bestelmeyer, B. T., Suding, K. N., Battaglia, L. L., et al. (2014). Resilience in ecology: abstraction, distraction, or where the action is? Biol. Conserv. 177, 43–51. doi: 10.1016/j.biocon.2014.06.008
Stevens-Rumann, C. S., Kemp, K. B., Higuera, P. E., Harvey, B. J., Rother, M. T., Donato, D. C., et al. (2018). Evidence for declining forest resilience to wildfires under climate change. Ecol. Lett. 21, 243–252. doi: 10.1111/ele.12889
Tedeschi, J. N., Kennington, W., Berry, O., Whiting, S., Meekan, M., and Mitchell, N. (2015). Increased expression of Hsp70 and Hsp90 mRNA as biomarkers of thermal stress in loggerhead turtle embryos (Caretta caretta). J. Therm. Biol. 47, 42–50. doi: 10.1016/j.jtherbio.2014.11.006
Telesford, Q. K., Joyce, K. E., Hayasaka, S., Burdette, J. H., and Laurienti, P. J. (2011). The ubiquity of small-world networks. Brain Connect. 1, 367–375. doi: 10.1089/brain.2011.0038
Urruty, N., Tailliez-Lefebvre, D., and Huyghe, C. (2016). Stability, robustness, vulnerability and resilience of agricultural systems. A review. Agron. Sustain. Dev. 36:15.
Walker, B., Holling, C. S., Carpenter, S. R., and Kinzig, A. (2004). Resilience, adaptability and transformability in social–ecological systems. Ecol. Soc. 9:5.
Wang, S., Haegeman, B., and Loreau, M. (2015). Dispersal and metapopulation stability. PeerJ 3:e1295. doi: 10.7717/peerj.1295
Wang, X., Xin, C., Cai, J., Zhou, Q., Dai, T., Cao, W., et al. (2016). Heat priming induces Trans-generational tolerance to high temperature stress in wheat. Front. Plant Sci. 7:501. doi: 10.3389/fpls.2016.00501
Woods, D. D. (2015). Four concepts for resilience and the implications for the future of resilience engineering. Reliabil. Eng. Syst. Saf. 141, 5–9. doi: 10.1016/j.ress.2015.03.018
Wu, G., Yan, Q., Jones, J. A., Tang, Y. J., Fong, S. S., and Koffas, M. A. (2016). Metabolic burden: cornerstones in synthetic biology and metabolic engineering applications. Trends Biotechnol. 34, 652–664. doi: 10.1016/j.tibtech.2016.02.010
Yang, Q., Fowler, M. S., Jackson, A. L., and Donohue, I. (2019). The predictability of ecological stability in a noisy world. Nat. Ecol. Evol. 3, 251–259. doi: 10.1038/s41559-018-0794-x
Yusishen, M. E., Yoon, G. R., Bugg, W., Jeffries, K. M., Currie, S., and Anderson, W. G. (2020). Love thy neighbor: social buffering following exposure to an acute thermal stressor in a gregarious fish, the lake sturgeon (Acipenser fulvescens). Comp. Biochem. Physiol. A Mol. Integr. Physiol. 243:110686. doi: 10.1016/j.cbpa.2020.110686
Zhang, B., DeAngelis, D., Ni, W. M., Wang, Y., Zhai, L., Kula, A., et al. (2020a). Effect of stressors on the carrying capacity of spatially distributed metapopulations. Am. Nat. 196, E46–E60.
Zhang, B., DeAngelis, D. L., and Ni, W. M. (2020b). Carrying capacity of spatially distributed metapopulations. Trends Ecol. Evol. doi: 10.1016/j.tree.2020.10.007 [Epub ahead of print].
Keywords: ecosystem, resilience, robustness, scaling, scale up
Citation: Davis JE, Kolozsvary MB, Pajerowska-Mukhtar KM and Zhang B (2021) Toward a Universal Theoretical Framework to Understand Robustness and Resilience: From Cells to Systems. Front. Ecol. Evol. 8:579098. doi: 10.3389/fevo.2020.579098
Received: 01 July 2020; Accepted: 11 December 2020;
Published: 08 January 2021.
Edited by:
Cang Hui, Stellenbosch University, South AfricaReviewed by:
John M. Anderies, Arizona State University, United StatesHenintsoa Onivola Minoarivelo, University of Cape Town, South Africa
Copyright © 2021 Davis, Kolozsvary, Pajerowska-Mukhtar and Zhang. This is an open-access article distributed under the terms of the Creative Commons Attribution License (CC BY). The use, distribution or reproduction in other forums is permitted, provided the original author(s) and the copyright owner(s) are credited and that the original publication in this journal is cited, in accordance with accepted academic practice. No use, distribution or reproduction is permitted which does not comply with these terms.
*Correspondence: Mary Beth Kolozsvary, mkolozsvary@siena.edu; Karolina M. Pajerowska-Mukhtar, kmukhtar@uab.edu; Bo Zhang, bozhangophelia@gmail.com
†These authors have contributed equally to this work