- 1Department of General Surgery, Renmin Hospital of Wuhan University, Wuhan, Hubei, China
- 2Hubei Key Laboratory of Digestive System Disease, Wuhan, Hubei, China
- 3Central Laboratory, Renmin Hospital of Wuhan University, Wuhan, Hubei, China
Objective: There is some evidence for an association between gut microbiota and nonalcoholic fatty liver disease (NAFLD), alcoholic liver disease (ALD), and viral hepatitis, but no studies have explored their causal relationship.
Methods: Instrumental variables of the gut microbiota (N = 13266) and gut microbiota-derived metabolites (N = 7824) were acquired, and a Mendelian randomization study was performed to explore their influence on NAFLD (1483 European cases and 17,781 European controls), ALD (2513 European cases and 332,951 European controls), and viral hepatitis risk (1971 European cases and 340,528 European controls). The main method for examining causality is inverse variance weighting (IVW).
Results: IVW results confirmed that Anaerotruncus (p = 0.0249), Intestinimonas (p = 0.0237), Lachnoclostridium (p = 0.0245), Lachnospiraceae NC2004 group (p = 0.0083), Olsenella (p = 0.0163), and Peptococcus (p = 0.0472) were protective factors for NAFLD, and Ruminococcus 1 (p = 0.0120) was detrimental for NAFLD. The higher abundance of three genera, Lachnospira (p = 0.0388), Desulfovibrio (p = 0.0252), and Ruminococcus torques group (p = 0.0364), was correlated with a lower risk of ALD, while Ruminococcaceae UCG 002 level was associated with a higher risk of ALD (p = 0.0371). The Alistipes (p = 0.0069) and Ruminococcaceae NK4A214 group (p = 0.0195) were related to a higher risk of viral hepatitis. Besides, alanine (p = 0.0076) and phenyllactate (p = 0.0100) were found to be negatively correlated with NAFLD, while stachydrine (Op = 0.0244) was found to be positively associated with NAFLD. The phenylacetate (p = 0.0353) and ursodeoxycholate (p = 0.0144) had a protective effect on ALD, while the threonate (p = 0.0370) exerted a detrimental influence on ALD. The IVW estimates of alanine (p = 0.0408) and cholate (p = 0.0293) showed their suggestive harmful effects against viral hepatitis, while threonate (p = 0.0401) displayed its suggestive protective effect against viral hepatitis.
Conclusion: In conclusion, our research supported causal links between the gut microbiome and its metabolites and NAFLD, ALD, and viral hepatitis.
1 Introduction
Nonalcoholic fatty liver disease (NAFLD) is a prevailing form of chronic liver disease that is marked by the accumulation of hepatic fat in patients who do not have a history of heavy alcohol intake (1). It comprises a wide range of gradually deteriorating pathological disorders, ranging from a straightforward case of nonalcoholic fatty liver to a more serious case of nonalcoholic steatohepatitis (NASH), the latter of which has a higher risk of developing cirrhosis, organ failure, and hepatocellular carcinoma (2–4). Alcoholic liver disease (ALD) is a disease due to chronic and excessive alcohol intake. The accumulation of fat in the liver cells is one of the early responses to excessive alcohol use. When alcohol abuse persists, steatosis may develop into steatohepatitis, fibrosis, cirrhosis, and ultimately hepatocellular cancer (5). As an inflammation of the liver, hepatitis can either go away on its own or develop into a serious condition that results in cirrhosis or hepatocellular cancer. Globally, the main cause of hepatitis is viral, with hepatitis B and C virus infections usually developing into chronic hepatitis (6). There is an urgent need to identify potential causal risk factors for NAFLD, ALD, and viral hepatitis since they pose a significant health burden globally.
The gut microbiota, as the “forgotten organ”, is a dynamic and intricate community of ecological bacteria (7). The liver is the first organ crossed by the portal vein of the intestine. The phrase “gut-liver axis” was coined to describe the close connection between the intestinal flora, the immune system, and the intestinal barrier that occurs in the gut and liver (8). Through the portal vein, the liver gets 75% of its blood from the gut. By secreting bile and other mediators, it also gives the intestines feedback (9). Thus, various gut factors, such as gut microbiota, bacterial composition, and gut microbiota-derived metabolites, are deeply involved in the homeostasis of the liver.
Recently, there has been growing evidence that intestinal flora is closely related to human health and is involved in the etiology of various complex diseases, including liver diseases (9, 10). However, there is controversy among these studies. For example, Zhu et al. revealed a higher relative abundance of Prevotella and no distinct alternation in Bacteroides in NAFLD patients than the control (11). However, Boursier et al. found that, compared to healthy controls, patients with NASH had higher levels of Bacteroides and lower levels of Prevotella (12). Besides, when compared to controls, several studies have demonstrated an increase in the Firmicutes to Bacteroidetes ratio in NAFLD and NASH (13, 14), while others have shown a decrease in this ratio (11, 15, 16). Confounding or reverse causation in observational studies could be to blame for the contradictory results in gut microbial dysbiosis in NAFLD.
As we know, confounding factors and reverse causation may both affect the findings of current observational epidemiological research, making causal inference difficult. The Mendelian randomization (MR) method using genetic variants as instrumental variables (IVs) in the epidemiological investigation has been generally accepted to estimate the causal influence of exposure on diseases (17). Based on the Mendelian inheritance rule, parental genetic alleles are randomly dispersed to their offspring during the meiotic process, which is regarded as a randomized controlled study (RCT). This method was chosen because it was practical, economical, and less likely to be confounded by covariables (18). Also, since genetic variants are already set at the time of conception, MR is less susceptible to the influence of reverse causation. Previous genetic research has shown that host genetic variants can affect the intestinal flora, allowing us to explore the relationship between gut microbiota and liver diseases using the MR approach.
Thus, in this study, the summary data from genome-wide association studies (GWASs) was used to explore the causal association of gut microbiota and metabolites with NAFLD, ALD, and viral hepatitis using the two-sample MR analysis.
2 Materials and methods
2.1 Study design
MR analysis is a genetic method that infers the causal effects of exposure on outcomes by using the random allocation of genetic variants at conception. The SNPs employed as IVs need to meet the following basic assumptions. First, there has to be a solid association between the SNPs and the exposure; second, the SNPs should not be related to the outcome via confounders; and third, the SNPs should not impact the outcome directly. Earlier research detailed further particulars of this method (19). The STROBE-MR guidelines were used to design this research (20). Figure 1 shows the flowchart of the MR study between gut microbiota and metabolites with liver diseases.
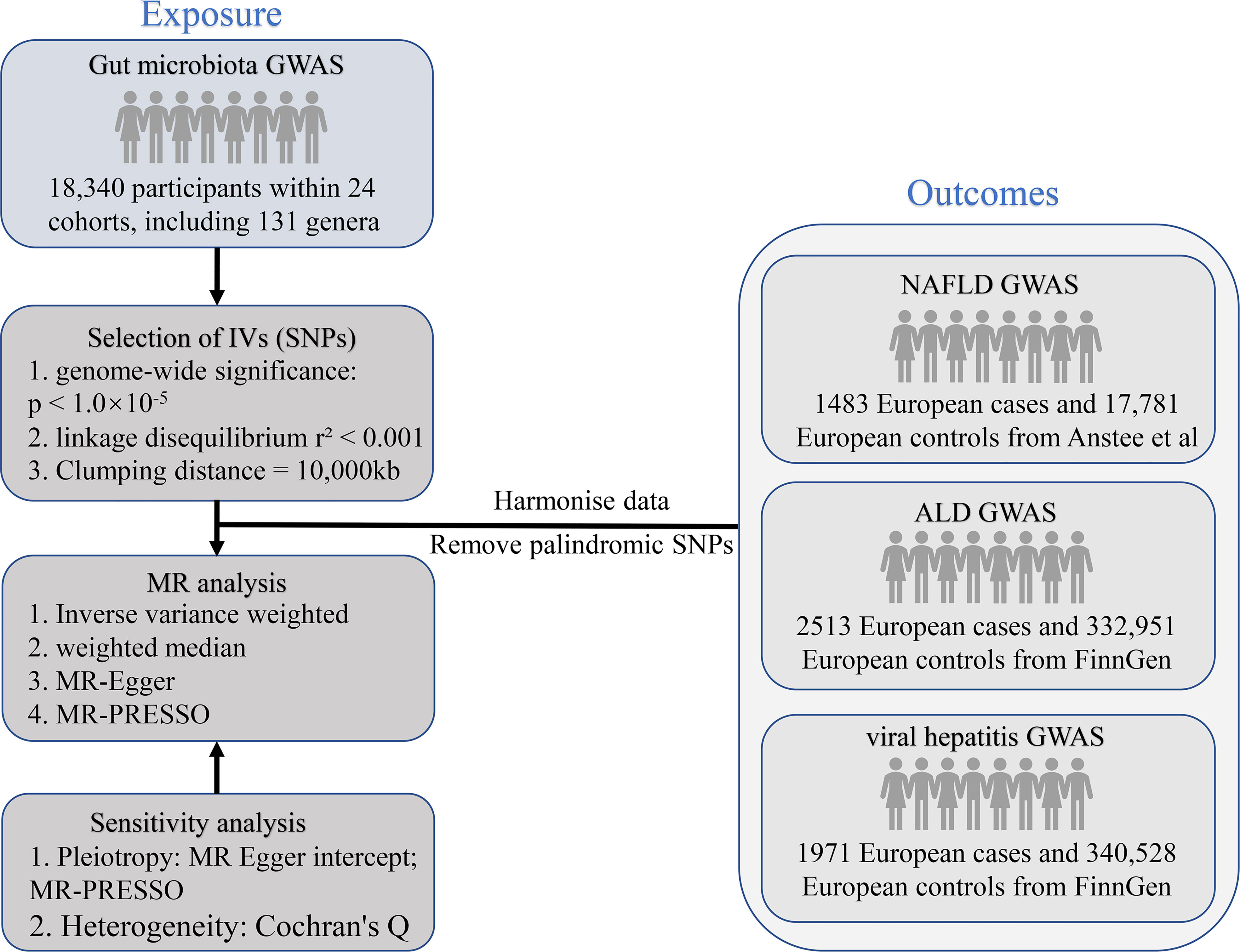
Figure 1 The study design of the present MR study of the associations of gut microbiota and metabolites and liver diseases. MR, Mendelian randomization; SNP, single nucleotide polymorphism; NAFLD, non-alcoholic fatty liver disease; ALD, alcoholic liver disease; GWAS, genome-wide association study; IV, instrumental variables.
2.2 Exposure sources
Genetic instruments of intestinal microbiome were acquired from the largest genome-wide meta-analysis published by the MiBioGen consortium (21). The study contained 24 cohorts with 18,340 individuals, most of whom were of European ancestry (16 cohorts, N = 13,266). The study targeted variable regions V4, V3–V4, and V1–V2 of the 16S rRNA gene to profile the microbial composition and to conduct taxonomic classification using direct taxonomic binning. For each cohort, microbiota quantitative trait loci (mbQTL) mapping analysis included only the taxa presented in > 10% of the samples (21). The lowest taxonomic level in this study was genus, and 131 genera with a mean abundance > 1% were found, including 12 unknown genera (21). Thus, 119 genus-level taxa were obtained in our study for MR analysis. The included cohorts all made adjustments for sex and age as covariates in their calculations (21).
We also used summary-level data from the human metabolome GWAS performed among subjects of European descent (TwinsUK and KORA, N = 7824) in light of the significant roles gut metabolites play in microbiota-host interaction (22). Then we utilized HMDB (23) to acquire a list of 12 gut microbiota-derived metabolite traits from all the measured metabolites in the GWAS, such as betaine, carnitine, cholate, choline, alanine, phenylacetate, phenyllactate, stachydrine, threonate, and ursodeoxycholate.
2.3 Outcome sources
The genetic association with NAFLD was extracted from the newly published GWAS summary statistics by Anstee et al., consisting of 1483 European cases and 17,781 European controls (24). The top 5 genetic principal components and genotyping batch were corrected during the analysis (24). GWAS summary-level data for ALD (2513 European cases and 332,951 European controls) and viral hepatitis (1971 European cases and 340,528 European controls) were downloaded from FinnGen consortium R8 release data (25). During the analysis, age, sex, the first 10 principal components, and the genotyping batch were corrected (25).
2.4 Genetic instrument selection
To satisfy the above MR assumption, we selected IVs with linkage disequilibrium r² < 0.001 and distance > 10,000 kb and attaining genome-wide significance (p < 1.0×10-5) (26). The linkage disequilibrium reference panel was established utilizing the 1000 Genomes Project European sample (27). Each IV’s strength was determined utilizing the F statistics = beta2/se2 (28). For adequate strength to be determined, the F-statistics had to be >10.
2.5 Statistical analysis
The primary statistical analysis method was the inverse variance weighted (IVW) method under random effects. This method was supplemented with weighted median analysis (29), MR-Egger regression (30), and MR-PRESSO methods (31). IVW assumes that all genetic variation SNPs are valid IVs with an overall bias of zero. As for the weighted median analysis, this estimate is consistent even if up to half of the weights are from invalid instruments. Besides, MR-Egger analysis can identify horizontal pleiotropy through the intercept (p < 0.05 for the intercept indicates pleiotropy) (30). The MR-PRESSO method can detect possible outliers and generate causal estimates after the removal of outlying IVs (31). To measure the degree of heterogeneity, the Q-value from Cochrane was applied. The causal relationship is considered significant if: 1) the p-value of the IVW method is less than < 0.05; 2) the estimations obtained using the MR-Egger, weighted median, and IVW methods all have the same direction; and 3) neither the MR-Egger intercept test nor the MR-PRESSO global test has statistical significance (p > 0.05) (32). Furthermore, in addition to meeting the 3 conditions mentioned above, for the connection between gut microbiota or metabolites and liver diseases, a Bonferroni-adjusted IVW p (pFDR) value of 4.2 × 10-5 (p = 0.05/119) or 5 × 10-4 (P = 0.05/10) was employed as the cut-off for statistical significance. p < 0.05 but more than the Bonferroni corrected significance level was seen as suggestive of evidence for a potential association (33, 34). Each test was two-sided and conducted utilizing the TwoSampleMR and MR-PRESSO packages in the R software (version 4.2.1) (31, 35).
3 Results
3.1 Causal effect of gut microbiota on NAFLD
The results of IVW analyses demonstrated that Anaerotruncus (OR = 0.595, 95% CI: 0.378-0.937, p = 0.0249), Intestinimonas (OR = 0.726, 95% CI: 0.550-0.958, p = 0.0237), Lachnoclostridium (OR = 0.523, 95% CI: 0.297-0.920, p = 0.0245), Lachnospiraceae NC2004 group (OR = 0.676, 95% CI: 0.505-0.904, p = 0.0083), Olsenella (OR = 0.770, 95% CI: 0.623-0.953, p = 0.0163), and Peptococcus (OR = 0.817, 95% CI: 0.669-0.998, p = 0.0472) were negatively associated with NAFLD (Table 1 and Figure 2), indicating a protective impact of the above genera on NAFLD (Table 1). The results of IVW analyses revealed that Ruminococcus 1 (OR = 1.833, 95% CI: 1.142-2.940, p = 0.0120) was positively related to NAFLD, suggesting a detrimental effect on NAFLD (Table 1 and Figure 2). These associations were also supported by the MR-PRESSO method, as shown in Table 1. Besides, the MR estimates of the weighted median analysis showed similar results in Anaerotruncus (OR = 0.519, 95% CI: 0.273-0.985, p = 0.0449), Lachnoclostridium (OR = 0.429, 95% CI: 0.225-0.816, p = 0.0099), and Olsenella (OR = 0.743, 95% CI: 0.562-0.982, p = 0.0370) (Table 1 and Figure 2). Whereas, for Lachnoclostridium and Olsenella, MR-Egger analysis results were in the opposite direction to IVW and weighted median analysis results (Table 1 and Figure 2). Detailed statistics for the remaining genera are shown in Table S1. We do not find significant heterogeneity across these results using Cochrane Q statistics (Table 1). The estimation of the intercept that was generated from the MR-Egger regression was centered around 0 and did not offer definitive evidence of horizontal pleiotropy (Table 1). No outliers were found by MR-PRESSO. The average F-statistic was 21.386, ranging from 17.045 to 28.784, revealing that there was no weak IV bias (Table S2).
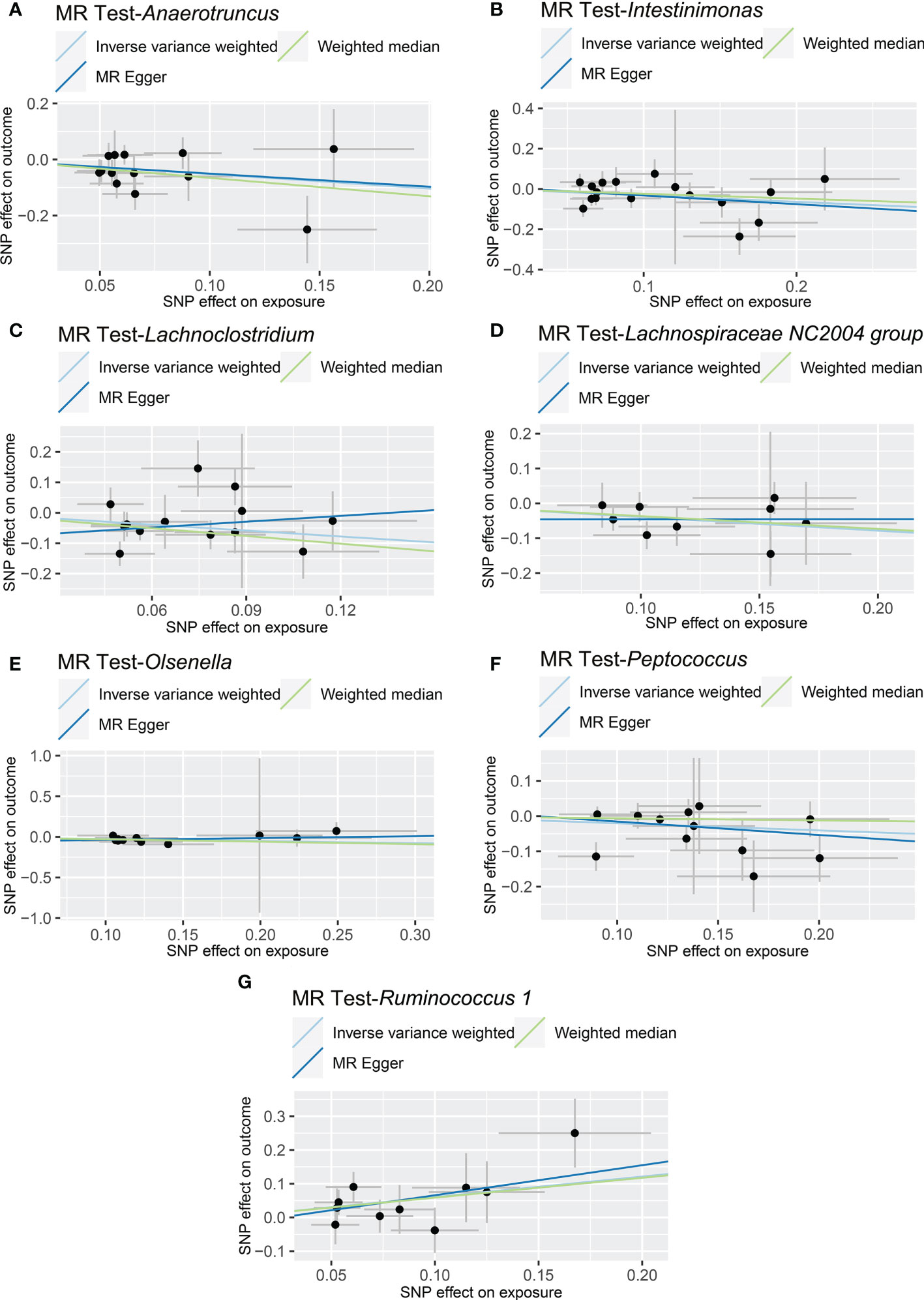
Figure 2 Causal relationship between gut microbiota and the risk of non-alcoholic fatty liver disease. Each point represents the SNP effects on Anaerotruncus (A), Intestinimonas (B), Lachnoclostridium (C), Lachnospiraceae NC2004 group (D), Olsenella (E), Peptococcus (F), Ruminococcus 1 (G) and non-alcoholic fatty liver disease. MR, Mendelian randomization; SNP, single nucleotide polymorphism.
3.2 Causal effect of gut microbiota on ALD
In the IVW method, we found that the genetically predicted higher relative abundance of three genera, Lachnospira (OR = 0.568, 95% CI: 0.332-0.971, p = 0.0388), Desulfovibrio (OR = 0.744, 95% CI: 0.574-0.964, p = 0.0252), and Ruminococcus torques group (OR = 0.621, 95% CI: 0.398-0.970, p = 0.0364), was correlated with a lower risk of ALD (Table 2 and Figure 3); while, the genetically predicted Ruminococcaceae UCG 002 level was associated with a higher risk of ALD (OR = 1.263, 95% CI: 1.014-1.572, p = 0.0371) (Table 2 and Figure 3). The results of the MR-PRESSO analysis were similar to those of the IVW method (Table 2). The IVW test, weighted median method, and MR-Egger test were all in the same direction, which strengthened the confidence in the true causal associations. Detailed statistics for the remaining genera are shown in Table S3. No significant heterogeneity was observed across these results (Table 2). MR-Egger regression confirmed that there was no horizontal pleiotropy between IVs and outcomes (Table 2). Moreover, neither outliers nor any indication of pleiotropy were observed in the MR-PRESSO analysis (Table 2). The F-statistics of IVs ranged between 18.53 and 31.28, indicating no evidence of weak instrument bias (Table S4).
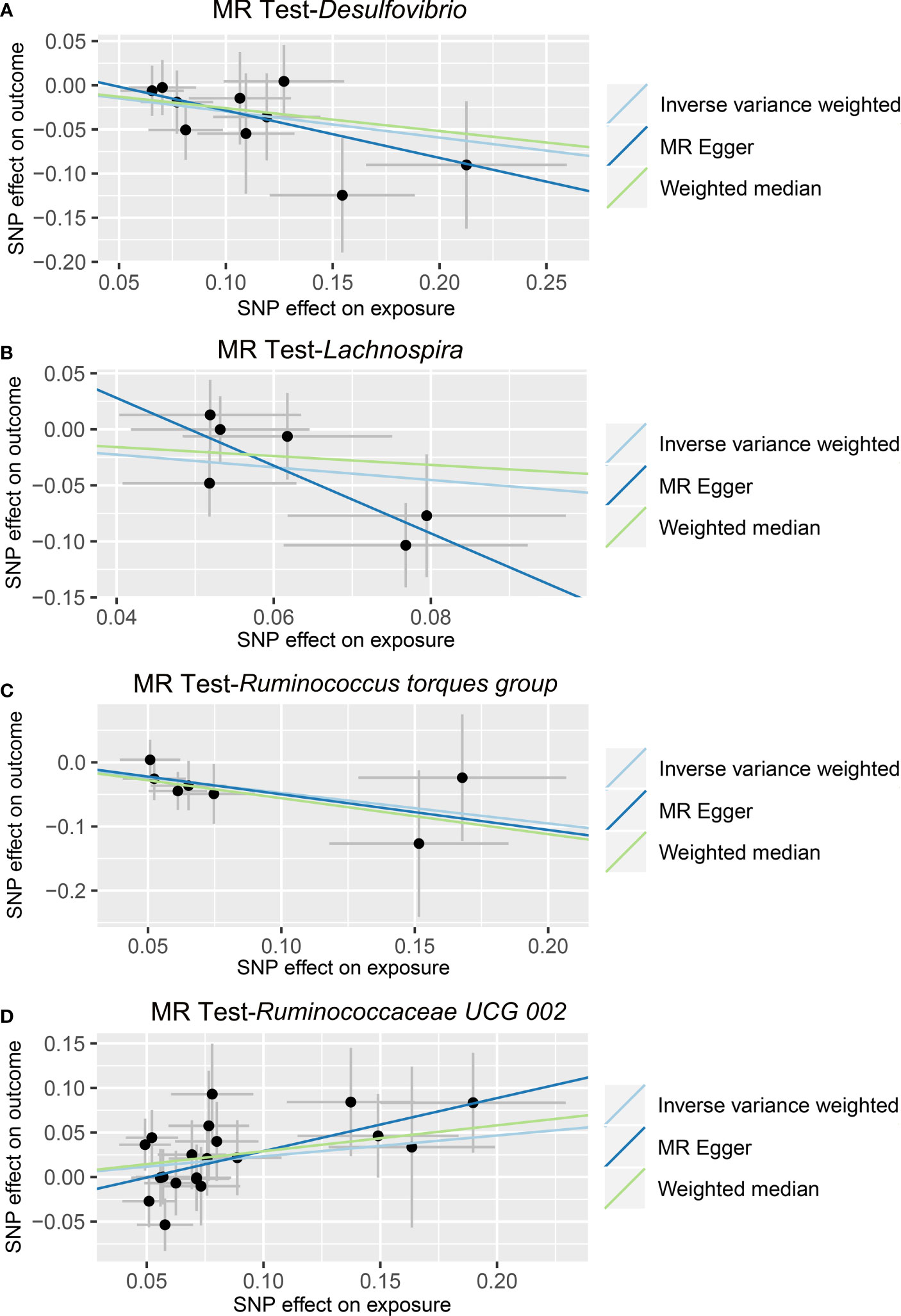
Figure 3 Causal relationship between gut microbiota and the risk of alcoholic liver disease. Each point represents the SNP effects on Desulfovibrio (A), Lachnospira (B), Ruminococcus torques group (C), Ruminococcaceae UCG 002 (D), and alcoholic liver disease. MR, Mendelian randomization; SNP, single nucleotide polymorphism.
3.3 Causal effect of gut microbiota on viral hepatitis
As shown in Figure 4 and Table 3, we observed that Alistipes (OR = 1.720, 95% CI: 1.160-2.550, p = 0.0069) and Ruminococcaceae NK4A214 group (OR = 1.460, 95% CI: 1.063-2.006, p = 0.0195) were related to a higher risk of viral hepatitis. The results of the MR-PRESSO analysis supported the above findings. Detailed statistics for the remaining genera are shown in Table S5. None of the MR-Egger regression intercepts deviated from null, and no outliers were detected with the MR-PRESSO test, suggesting no evidence of horizontal pleiotropy (Table 3). Besides, the F statistic was larger than 10, and the Cochrane Q statistic results revealed no significant heterogeneity (Tables 3, S6).
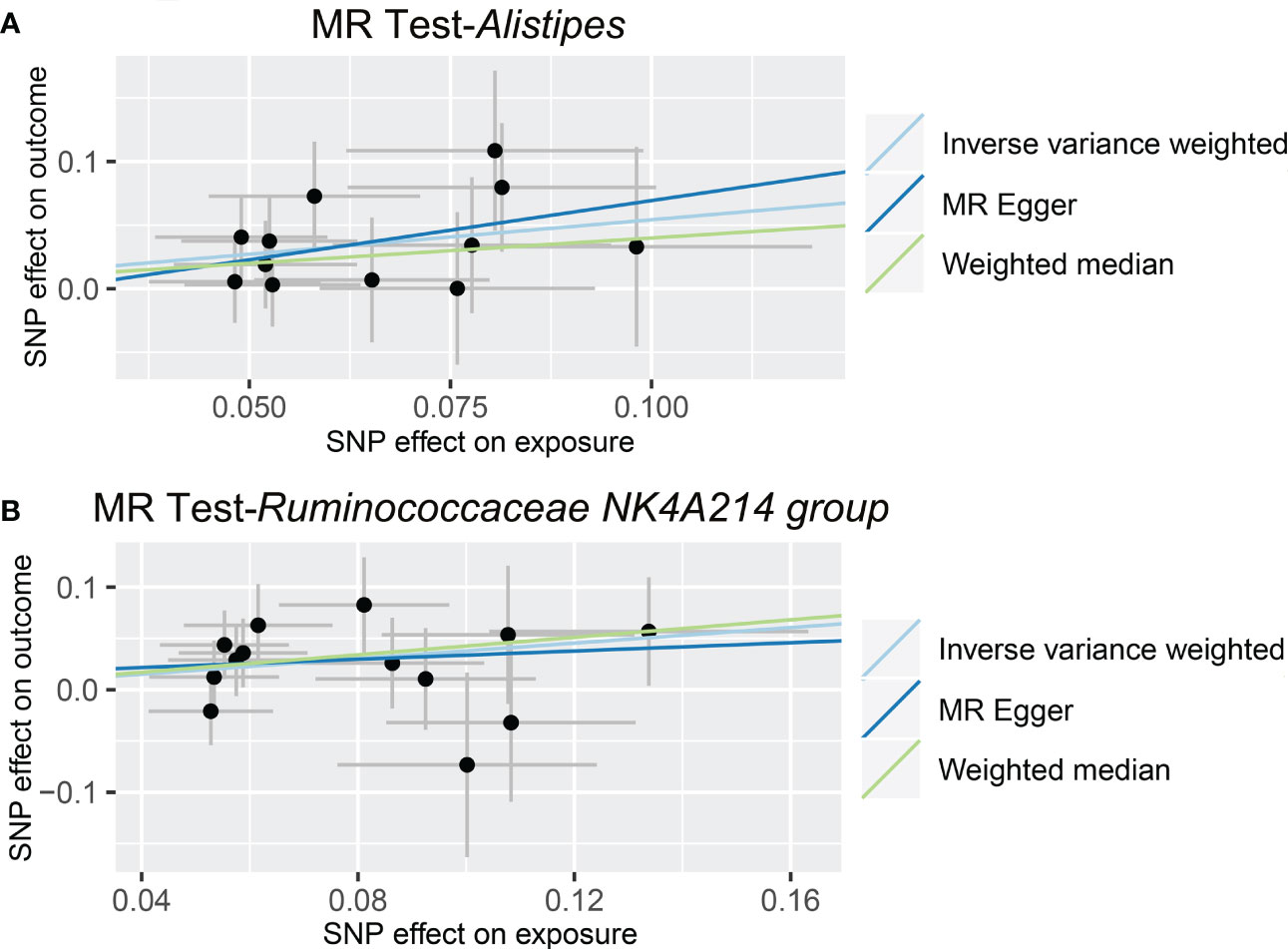
Figure 4 Causal relationship between gut microbiota and the risk of viral hepatitis. Each point represents the SNP effects on Alistipes (A), Ruminococcaceae NK4A214 group (B), and viral hepatitis. MR, Mendelian randomization; SNP, single nucleotide polymorphism.
3.4 Causal effect of gut microbiota-derived metabolites on liver diseases
As shown in Tables 4, S7, and Figure 5, alanine (OR = 19.586, 95% CI: 2.206-173.934, p = 0.0076) and phenyllactate (OR = 0.212, 95% CI: 0.065-0.689, p = 0.0100) were found to be negatively correlated with NAFLD, while stachydrine (OR = 2.228, 95% CI: 1.109-4.474, p = 0.0244) was found to be positively associated with NAFLD in the IVW and MR-PRESSO methods. The IVW estimate indicated that phenylacetate (OR = 0.496, 95% CI: 0.258-0.953, p = 0.0353) and ursodeoxycholate (OR = 0.662, 95% CI: 0.476-0.921, p = 0.0144) had a protective effect on ALD; while threonate (OR = 1.570, 95% CI: 1.028-2.397, p = 0.0370) exerts a detrimental influence on ALD (Tables 5, S8, and Figure 6). Besides, the IVW estimate of alanine (OR = 3.348, 95% CI: 1.052-10.655, p = 0.0408) and cholate (OR = 1.560, 95% CI: 1.046-2.327, p = 0.0293) showed its suggestive harmful effect against viral hepatitis; and threonate (OR = 0.621, 95% CI: 0.385-0.971, p = 0.0401) displayed its suggestive protective effect against viral hepatitis (Tables 6, S9, and Figure 7). No heterogeneity and horizontal pleiotropy were observed in these analyses (Tables 4–6). The F-statistics of IVs ranged between 17.64 and 88.97 (Tables S10–12).
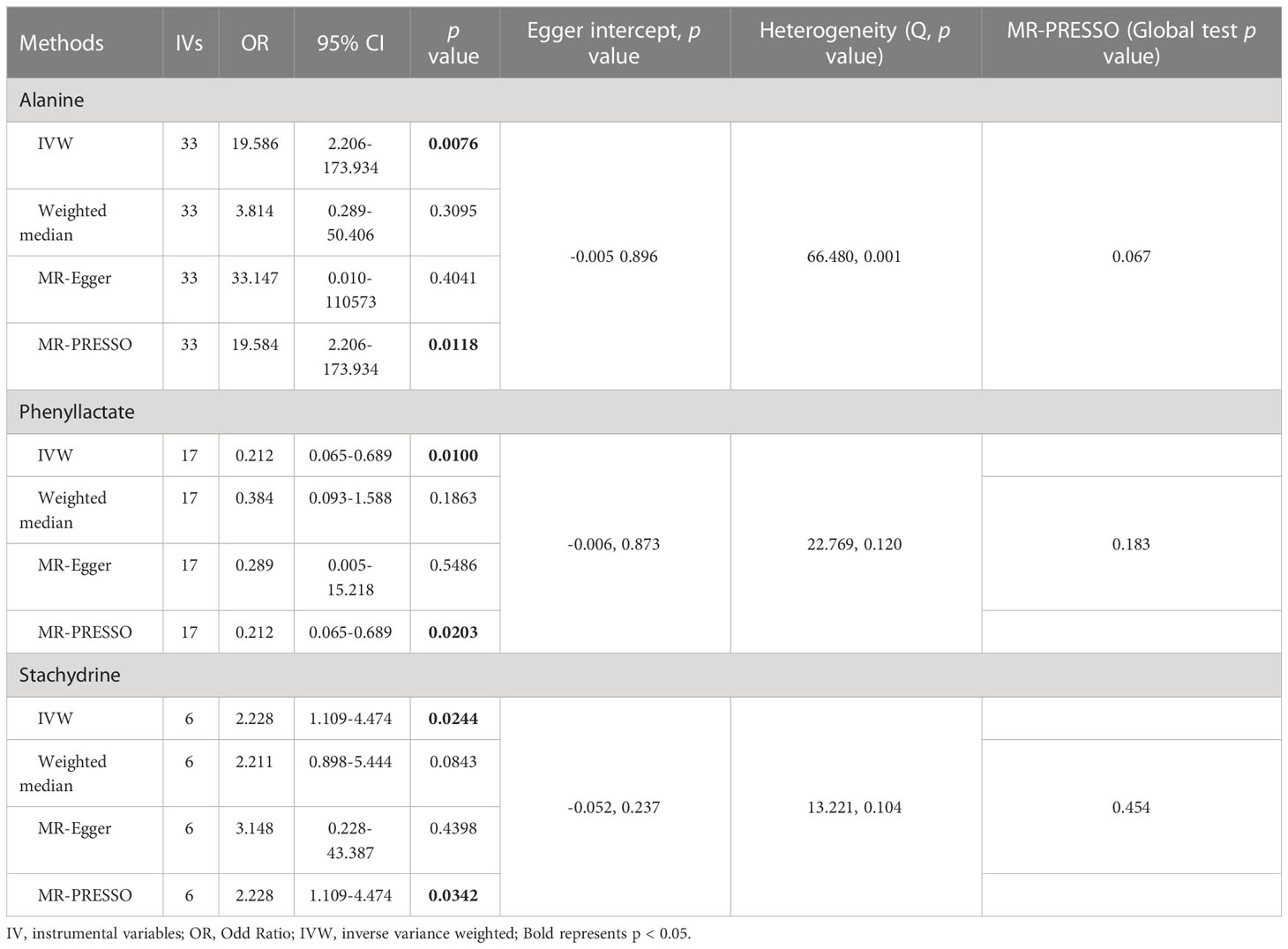
Table 4 Association of genetically predicted gut microbiota derived metabolites with non-alcoholic fatty liver disease.
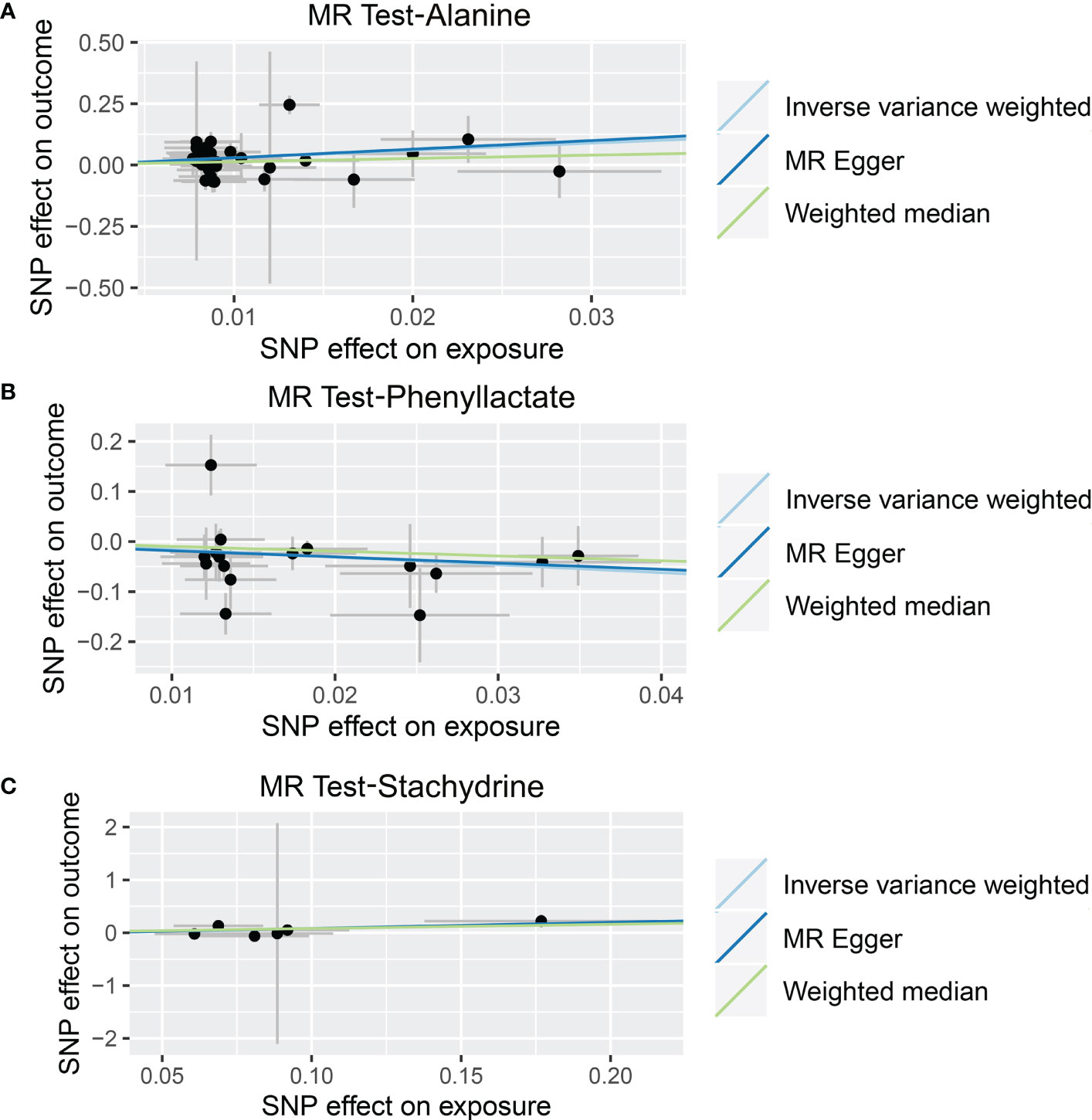
Figure 5 Causal relationship between gut microbiota-derived metabolites and the risk of non-alcoholic fatty liver disease. Each point represents the SNP effects on Alanine (A), Phenyllactate (B), Stachydrine (C), and non-alcoholic fatty liver disease. MR, Mendelian randomization; SNP, single nucleotide polymorphism.
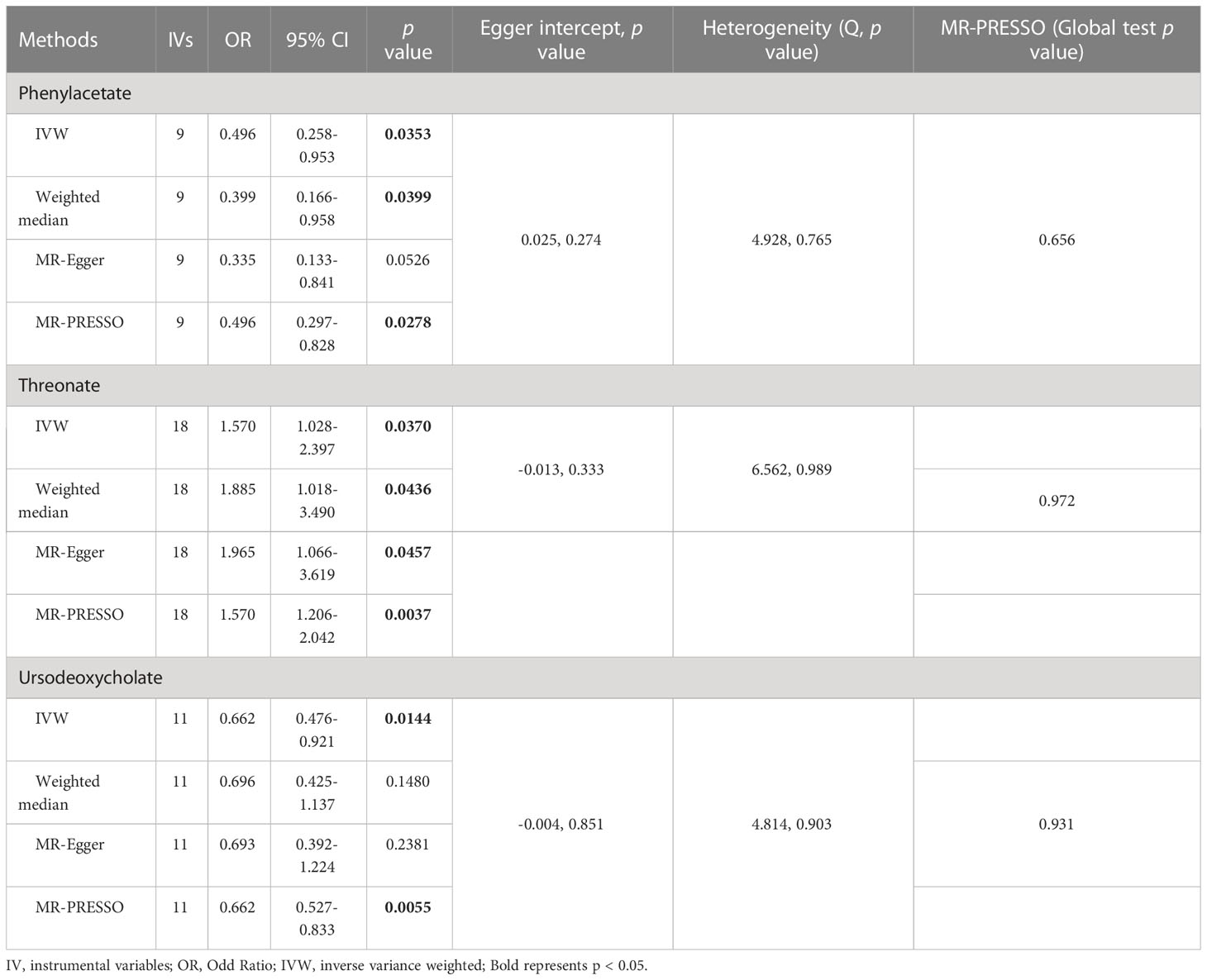
Table 5 Association of genetically predicted gut microbiota derived metabolites with alcoholic liver disease.
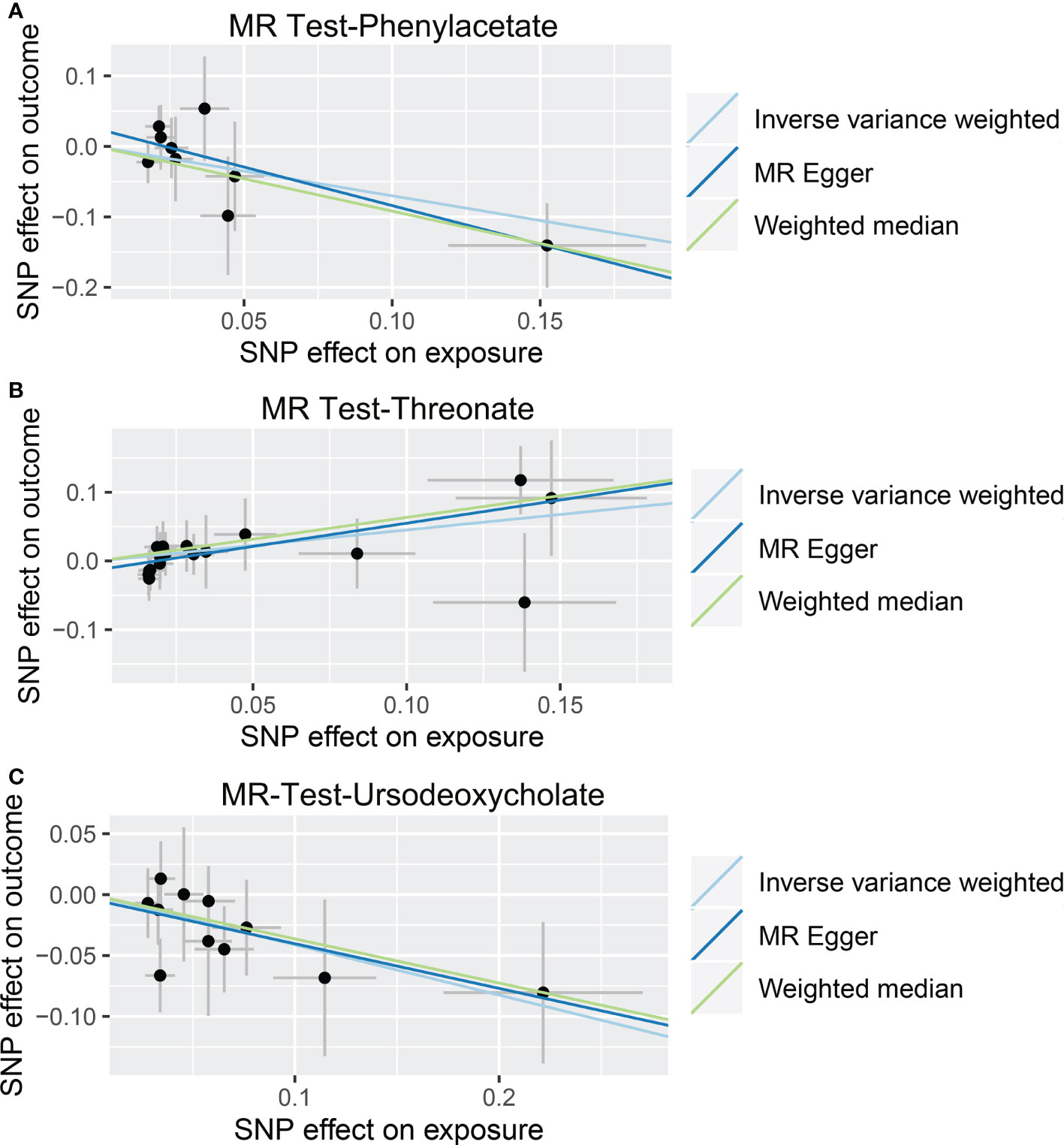
Figure 6 Causal relationship between gut microbiota-derived metabolites and the risk of alcoholic liver disease. Each point represents the SNP effects on Phenylacetate (A), Threonate (B), Ursodeoxycholate (C), and alcoholic liver disease. MR, Mendelian randomization; SNP, single nucleotide polymorphism.
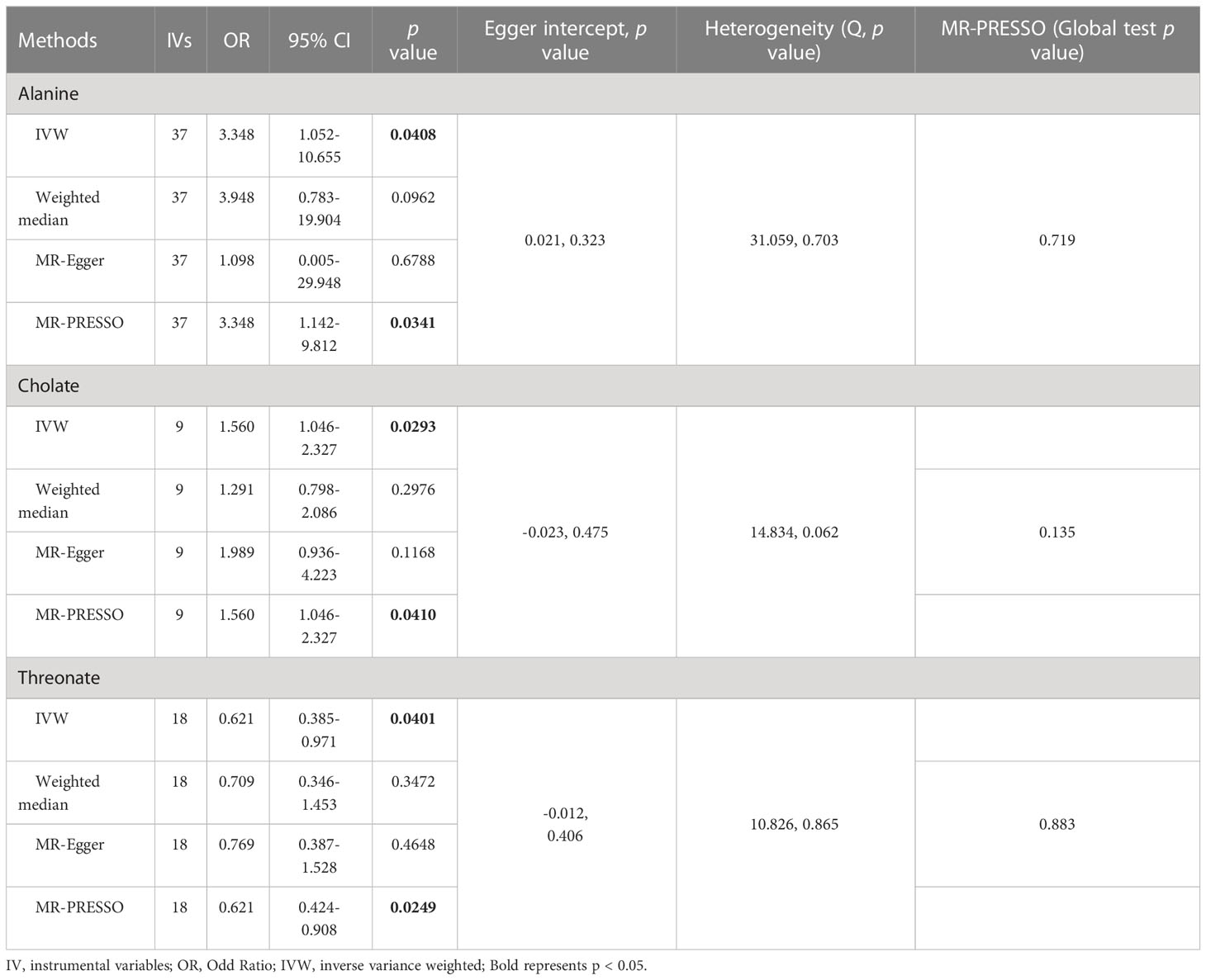
Table 6 Association of genetically predicted gut microbiota derived metabolites with viral hepatitis.
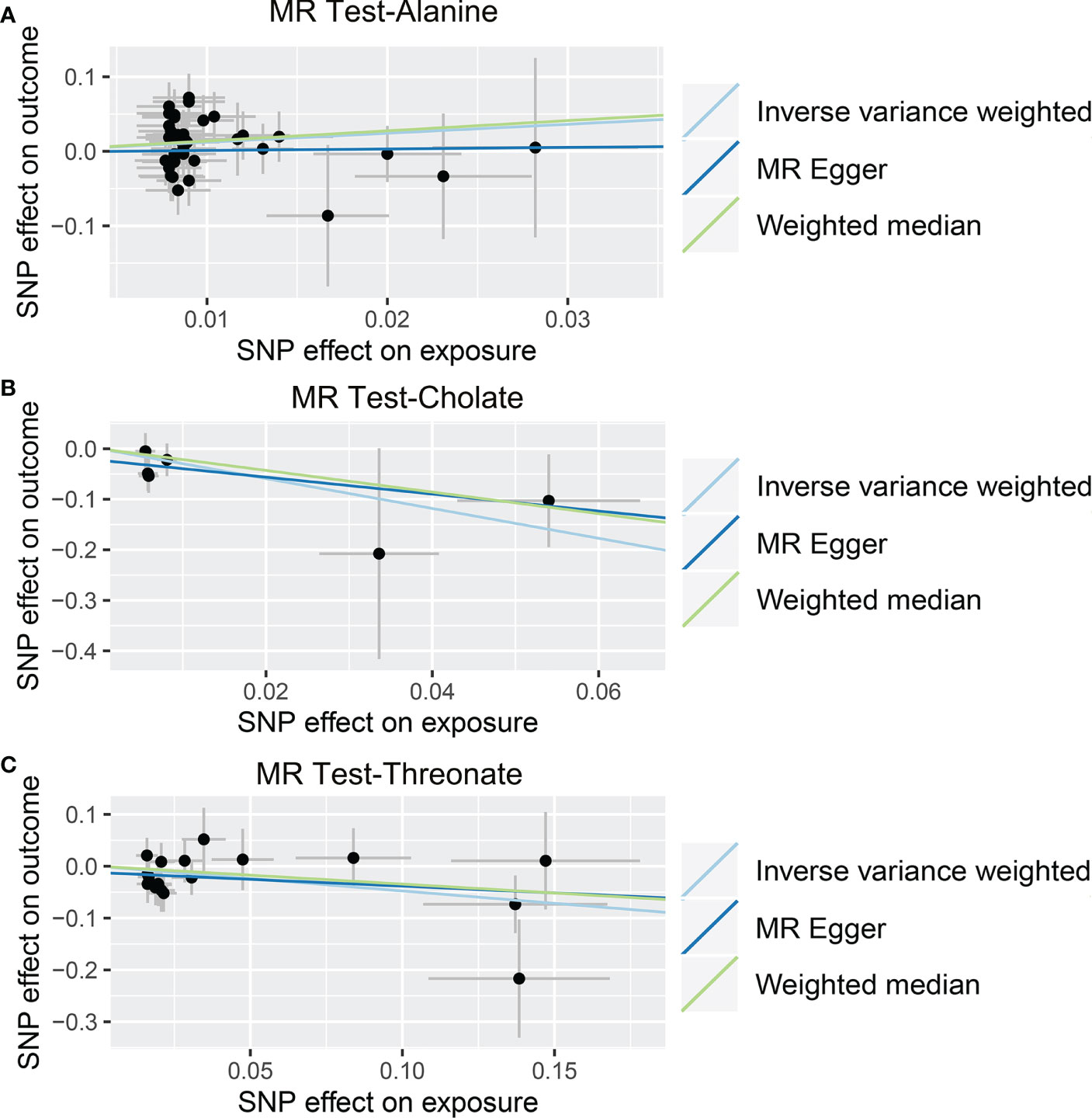
Figure 7 Causal relationship between gut microbiota-derived metabolites and the risk of viral hepatitis. Each point represents the SNP effects on Alanine (A), Cholate (B), Threonate (C), and viral hepatitis. MR, Mendelian randomization; SNP, single nucleotide polymorphism.
4 Discussion
According to our knowledge, this study is the first to estimate the causal relationships between gut microbiota, gut microbiota-derived metabolites, and liver diseases using MR analysis. Our results revealed that multiple gut microbiota and metabolites play significant roles in the development of liver diseases, 5 suggestive microbial taxa (Anaerotruncus, Intestinimonas, Lachnospiraceae NC2004 group, Peptococcus, and Ruminococcus 1) and 3 suggestive metabolites (Alanine, Phenyllactate, and Stachydrine) in NAFLD, 4 suggestive microbial taxa (Ruminococcaceae UCG 002, Lachnospira, Desulfovibrio, and Ruminococcus torques group) and 3 suggestive metabolites (Phenylacetate, Threonate, and Ursodeoxycholate) in ALD, 2 suggestive microbial taxa (Alistipes and Ruminococcaceae NK4A214 group) and 3 suggestive metabolites (Alanine, Cholate, and Threonate) in viral hepatitis. Notably, the MR test p values for both gut microbiota and metabolites and liver diseases were greater than pFDR.
Anaerotruncus and Intestinimonas were revealed to be butyrate-producing bacterium in the intestine (36–39). Intestinimonas is generally recognized as beneficial bacteria with anti-inflammatory and anti-obesity properties (40). Rodriguez-Diaz et al. (41) found a significant decrease in the abundance of Intestinimonas in patients with NAFLD compared to the healthy population. Supplementation with Adzuki beans has been shown to significantly reduce high-fat diet-induced obesity and lipid accumulation, as well as lipopolysaccharide levels, and alleviate liver function impairment and hepatic steatosis (42). Besides, it significantly reversed the imbalance of gut microbiota caused by high-fat diets and significantly increased the abundance of Lachnoclostridium (42). As for Olsenella, Zhong et al. showed that probiotic-fermented blueberry juice significantly reduced low-density lipoprotein cholesterol levels and fat accumulation, ameliorated insulin resistance, and improved the abundance and diversity of intestinal microbial communities in high-fat diet mice (43). The blueberry juice-treated mouse showed a relatively high abundance of lean bacteria (Olsenella and Bifidobacterium) and a lower abundance of obesity-associated bacteria (Oscillibacter and Alistipes) compared to the high-fat diet-fed mouse (43). Interestingly, Li et al. revealed that the gut formation of propionic acid and acetic acid is related to an increase in Olsenella in pectin-fed mice (44). Recently, Pan et al. diagnosed 21 chronic hepatitis B and 42 NAFLD patients with the classic damp-heat (DH) syndrome group and identified 29 chronic hepatitis B and 28 NAFLD patients as the non-DH syndrome group. They found a decreased relative abundance of the Lachnospiraceae NC2004 group in patients with the DH syndrome compared to the non-DH syndrome (45). Taken together, these studies were in agreement with our MR analysis that this aforementioned genus plays a protective role in NAFLD. In contrast, Pung et al. demonstrated that Ulva prolifera polysaccharide greatly slowed high-fat diet-induced weight gain, ameliorated metabolic disturbances in high-fat diet-fed mice, and improved intestinal flora disorders, as evidenced by the growth in Bifidobacterium abundance and downregulation of Ruminococcus 1 abundance (46). This implies that Ruminococcus 1 may play a negative role in NAFLD.
Alistipes is mainly found in the intestines of healthy humans (47, 48). However, Alistipes has also been isolated from the bloodstream, appendiceal, and abdominal, highlighting its possible opportunistic pathogenic involvement in human disorders (48). Feng et al. found that Alistipes could promote the development of colorectal cancer via the interleukin-6/signal transducer and activator of transcription 3 pathway (49). As for Ruminococcaceae UCG 002, there was significant enrichment of Ruminococcaceae UCG 002 abundance in prostate cancer patients compared to the healthy population, suggesting a pathogenic role (50). These studies support our conclusions. We found that Ruminococcaceae UCG 002 and Alistipes play a pathogenic role in ALD and viral hepatitis, respectively.
Lachnospira was significantly lower in all disease cohorts (multiple sclerosis, inflammatory bowel disease, and rheumatoid arthritis) relative to healthy controls (51). Due to its reduced abundance, studies suggest that Lachnospira may have a protective effect under inflammatory conditions (51, 52). Desulfovibrio was negatively related to the host body mass index, waist, triglyceride, and uric acid, which are signs of obesity or metabolic disorders (53–56). The abundance of Desulfovibrio was positively related to the diversity of flora, favoring microbiome stability and host health (57, 58). Besides, Desulfovibrio was positively correlated with the beneficial bacteria Oscillospira, Phascolarctobacterium, Prevotella, Coprococcus, Dialister, Ruminococcus, Akkermansia, Roseburia, Faecalibacterium, and Bacteroides and negatively correlated with the harmful bacteria Streptococcus, Clostridium, Escherichia, Klebsiella, and Ralstonia (59–68). Previous studies have shown a positive correlation between the Ruminococcus torques group and short-chain fatty acid levels by studying some people who ingested less starch in order to lose weight (69). Recently, Wan et al. found that improvement in colitis was associated with a higher Ruminococcus torques group, suggesting that the Ruminococcus torques group may have another application as a potential probiotic in the anti-inflammatory response (70). The above studies revealed their beneficial role in human diseases and supported our findings.
This work also has some limitations. First, because only people of European heritage were included in the GWAS, the conclusions of this study might not apply to people of other racial or ethnic backgrounds. Second, the sequencing of the 16S rRNA genes only permitted resolution from the genus to the phylum level, not at a more specific level, and the results were skewed when certain specific species affected the risk of liver diseases. Third, our results are not significant after the Bonferroni adjustment. However, multiple statistical corrections may overlook GM taxa with a potential causal connection to liver diseases because they are excessively tight and cautious. Furthermore, although the Mendelian randomization analysis was comparable to the level of evidence from the RCT study, further animal experimental confirmation is necessary.
5 Conclusion
In conclusion, our research supported causal links between the gut microbiome and its metabolites and NAFLD, ALD, and viral hepatitis. It is necessary to conduct further population-based research on the potential mechanisms of gut microbiota and liver disease development.
Data availability statement
The original contributions presented in the study are included in the article/Supplementary Material. Further inquiries can be directed to the corresponding authors.
Ethics statement
Ethical review and approval was not required for the study on human participants in accordance with the local legislation and institutional requirements. Written informed consent for participation was not required for this study in accordance with the national legislation and the institutional requirements.
Author contributions
LilZ, LiuZ, TK, PW, RL, and WW conceived and designed the study. LilZ, LiuZ, TK, ZQ, KW, ZW, and LL were responsible for the collection and assembly of data, data analysis, interpretation, and writing the manuscript. RL, PW, and WW revised the manuscript. All the work was performed under RL, PW, and WW instructions. All authors contributed to the article and approved the submitted version.
Funding
This work was supported by grants from the National Natural Science Foundation of China (No. 82172855, 81870442), and the Natural Science Foundation of Hubei Province, China (No. 2021CFB365).
Acknowledgments
We want to acknowledge the participants and investigators of the FinnGen study.
Conflict of interest
The authors declare that the research was conducted in the absence of any commercial or financial relationships that could be construed as a potential conflict of interest.
Publisher’s note
All claims expressed in this article are solely those of the authors and do not necessarily represent those of their affiliated organizations, or those of the publisher, the editors and the reviewers. Any product that may be evaluated in this article, or claim that may be made by its manufacturer, is not guaranteed or endorsed by the publisher.
Supplementary material
The Supplementary Material for this article can be found online at: https://www.frontiersin.org/articles/10.3389/fendo.2023.1159148/full#supplementary-material
References
1. Younossi ZM, Koenig AB, Abdelatif D, Fazel Y, Henry L, Wymer M. Global epidemiology of nonalcoholic fatty liver disease-meta-analytic assessment of prevalence, incidence, and outcomes. Hepatology (2016) 64(1):73–84. doi: 10.1002/hep.28431
2. Hardy T, Oakley F, Anstee QM, Day CP. Nonalcoholic fatty liver disease: Pathogenesis and disease spectrum. Annu Rev Pathol (2016) 11:451–96. doi: 10.1146/annurev-pathol-012615-044224
3. Marjot T, Moolla A, Cobbold JF, Hodson L, Tomlinson JW. Nonalcoholic fatty liver disease in adults: Current concepts in etiology, outcomes, and management. Endocr Rev (2020) 41(1):bnz009. doi: 10.1210/endrev/bnz009
4. Yuan Q, Deng D, Pan C, Ren J, Wei T, Wu Z, et al. Integration of transcriptomics, proteomics, and metabolomics data to reveal HER2-associated metabolic heterogeneity in gastric cancer with response to immunotherapy and neoadjuvant chemotherapy. Front Immunol (2022) 13:951137. doi: 10.3389/fimmu.2022.951137
5. Osna NA, Donohue TM Jr., Kharbanda KK. Alcoholic liver disease: Pathogenesis and current management. Alcohol Res (2017) 38(2):147–61.
6. Ikeda K, Saitoh S, Suzuki Y, Kobayashi M, Tsubota A, Koida I, et al. Disease progression and hepatocellular carcinogenesis in patients with chronic viral hepatitis: a prospective observation of 2215 patients. J Hepatol (1998) 28(6):930–8. doi: 10.1016/s0168-8278(98)80339-5
7. O'Hara AM, Shanahan F. The gut flora as a forgotten organ. EMBO Rep (2006) 7(7):688–93. doi: 10.1038/sj.embor.7400731
8. Bruneau A, Hundertmark J, Guillot A, Tacke F. Molecular and cellular mediators of the gut-liver axis in the progression of liver diseases. Front Med (Lausanne) (2021) 8:725390. doi: 10.3389/fmed.2021.725390
9. Manzoor R, Ahmed W, Afify N, Memon M, Yasin M, Memon H, et al. Trust your gut: The association of gut microbiota and liver disease. Microorganisms (2022) 10(5):1045. doi: 10.3390/microorganisms10051045
10. Xu M, Luo K, Li J, Li Y, Zhang Y, Yuan Z, et al. Role of intestinal microbes in chronic liver diseases. Int J Mol Sci (2022) 23(20):12661. doi: 10.3390/ijms232012661
11. Zhu L, Baker SS, Gill C, Liu W, Alkhouri R, Baker RD, et al. Characterization of gut microbiomes in nonalcoholic steatohepatitis (NASH) patients: a connection between endogenous alcohol and NASH. Hepatology (2013) 57(2):601–9. doi: 10.1002/hep.26093
12. Boursier J, Mueller O, Barret M, Machado M, Fizanne L, Araujo-Perez F, et al. The severity of nonalcoholic fatty liver disease is associated with gut dysbiosis and shift in the metabolic function of the gut microbiota. Hepatology (2016) 63(3):764–75. doi: 10.1002/hep.28356
13. Del Chierico F, Nobili V, Vernocchi P, Russo A, De Stefanis C, Gnani D, et al. Gut microbiota profiling of pediatric nonalcoholic fatty liver disease and obese patients unveiled by an integrated meta-omics-based approach. Hepatology (2017) 65(2):451–64. doi: 10.1002/hep.28572
14. Monga Kravetz A, Testerman T, Galuppo B, Graf J, Pierpont B, Siebel S, et al. Effect of gut microbiota and PNPLA3 rs738409 variant on nonalcoholic fatty liver disease (NAFLD) in obese youth. J Clin Endocrinol Metab (2020) 105(10):e3575–85. doi: 10.1210/clinem/dgaa382
15. Jasirwan COM, Lesmana CRA, Hasan I, Sulaiman AS, Gani RA. The role of gut microbiota in non-alcoholic fatty liver disease: pathways of mechanisms. Biosci Microbiota Food Health (2019) 38(3):81–8. doi: 10.12938/bmfh.18-032
16. Wong VW, Tse CH, Lam TT, Wong GL, Chim AM, Chu WC, et al. Molecular characterization of the fecal microbiota in patients with nonalcoholic steatohepatitis–a longitudinal study. PloS One (2013) 8(4):e62885. doi: 10.1371/journal.pone.0062885
17. Emdin CA, Khera AV, Kathiresan S. Mendelian randomization. JAMA (2017) 318(19):1925–6. doi: 10.1001/jama.2017.17219
18. Paternoster L, Tilling K, Davey Smith G. Genetic epidemiology and mendelian randomization for informing disease therapeutics: Conceptual and methodological challenges. PloS Genet (2017) 13(10):e1006944. doi: 10.1371/journal.pgen.1006944
19. Dimou NL, Tsilidis KK. A primer in mendelian randomization methodology with a focus on utilizing published summary association data. Methods Mol Biol (2018) 1793:211–30. doi: 10.1007/978-1-4939-7868-7_13
20. Skrivankova VW, Richmond RC, Woolf BAR, Davies NM, Swanson SA, VanderWeele TJ, et al. Strengthening the reporting of observational studies in epidemiology using mendelian randomisation (STROBE-MR): explanation and elaboration. BMJ (2021) 375:n2233. doi: 10.1136/bmj.n2233
21. Kurilshikov A, Medina-Gomez C, Bacigalupe R, Radjabzadeh D, Wang J, Demirkan A, et al. Large-scale association analyses identify host factors influencing human gut microbiome composition. Nat Genet (2021) 53(2):156–65. doi: 10.1038/s41588-020-00763-1
22. Ning J, Huang SY, Chen SD, Zhang YR, Huang YY, Yu JT. Investigating casual associations among gut microbiota, metabolites, and neurodegenerative diseases: A mendelian randomization study. J Alzheimers Dis (2022) 87(1):211–22. doi: 10.3233/JAD-215411
23. Wishart DS, Feunang YD, Marcu A, Guo AC, Liang K, Vazquez-Fresno R, et al. HMDB 4.0: the human metabolome database for 2018. Nucleic Acids Res (2018) 46(D1):D608–D17. doi: 10.1093/nar/gkx1089
24. Anstee QM, Darlay R, Cockell S, Meroni M, Govaere O, Tiniakos D, et al. Genome-wide association study of non-alcoholic fatty liver and steatohepatitis in a histologically characterised cohort. J Hepatol (2020) 73(3):505–15. doi: 10.1016/j.jhep.2020.04.003
25. Kurki MI, Karjalainen J, Palta P, Sipilä TP, Kristiansson K, Donner K, et al. FinnGen: Unique genetic insights from combining isolated population and national health register data. medRxiv (2022) 2022.03.03.22271360. doi: 10.1101/2022.03.03.22271360
26. Sanna S, van Zuydam NR, Mahajan A, Kurilshikov A, Vich Vila A, Vosa U, et al. Causal relationships among the gut microbiome, short-chain fatty acids and metabolic diseases. Nat Genet (2019) 51(4):600–5. doi: 10.1038/s41588-019-0350-x
27. Clarke L, Zheng-Bradley X, Smith R, Kulesha E, Xiao C, Toneva I, et al. The 1000 genomes project: data management and community access. Nat Methods (2012) 9(5):459–62. doi: 10.1038/nmeth.1974
28. Xie J, Huang H, Liu Z, Li Y, Yu C, Xu L, et al. The associations between modifiable risk factors and nonalcoholic fatty liver disease: A comprehensive mendelian randomization study. Hepatology (2023) 77(3):949–64. doi: 10.1002/hep.32728
29. Bowden J, Davey Smith G, Haycock PC, Burgess S. Consistent estimation in mendelian randomization with some invalid instruments using a weighted median estimator. Genet Epidemiol (2016) 40(4):304–14. doi: 10.1002/gepi.21965
30. Bowden J, Davey Smith G, Burgess S. Mendelian randomization with invalid instruments: effect estimation and bias detection through egger regression. Int J Epidemiol (2015) 44(2):512–25. doi: 10.1093/ije/dyv080
31. Verbanck M, Chen CY, Neale B, Do R. Detection of widespread horizontal pleiotropy in causal relationships inferred from mendelian randomization between complex traits and diseases. Nat Genet (2018) 50(5):693–8. doi: 10.1038/s41588-018-0099-7
32. Zhang Y, Liu Z, Choudhury T, Cornelis MC, Liu W. Habitual coffee intake and risk for nonalcoholic fatty liver disease: a two-sample mendelian randomization study. Eur J Nutr (2021) 60(4):1761–7. doi: 10.1007/s00394-020-02369-z
33. Yu XH, Yang YQ, Cao RR, Bo L, Lei SF. The causal role of gut microbiota in development of osteoarthritis. Osteoarthritis Cartilage (2021) 29(12):1741–50. doi: 10.1016/j.joca.2021.08.003
34. Zhuang Z, Li N, Wang J, Yang R, Wang W, Liu Z, et al. GWAS-associated bacteria and their metabolites appear to be causally related to the development of inflammatory bowel disease. Eur J Clin Nutr (2022) 76(7):1024–30. doi: 10.1038/s41430-022-01074-w
35. Hemani G, Zheng J, Elsworth B, Wade KH, Haberland V, Baird D, et al. The MR-base platform supports systematic causal inference across the human phenome. Elife (2018) 7:e34408. doi: 10.7554/eLife.34408
36. Bailen M, Bressa C, Martinez-Lopez S, Gonzalez-Soltero R, Montalvo Lominchar MG, San Juan C, et al. Microbiota features associated with a high-Fat/Low-Fiber diet in healthy adults. Front Nutr (2020) 7:583608. doi: 10.3389/fnut.2020.583608
37. Wang J, Ji H, Wang S, Liu H, Zhang W, Zhang D, et al. Probiotic lactobacillus plantarum promotes intestinal barrier function by strengthening the epithelium and modulating gut microbiota. Front Microbiol (2018) 9:1953. doi: 10.3389/fmicb.2018.01953
38. Luo L, Hu M, Li Y, Chen Y, Zhang S, Chen J, et al. Association between metabolic profile and microbiomic changes in rats with functional dyspepsia. RSC Adv (2018) 8(36):20166–81. doi: 10.1039/c8ra01432a
39. Bui TP, Ritari J, Boeren S, de Waard P, Plugge CM, de Vos WM. Production of butyrate from lysine and the amadori product fructoselysine by a human gut commensal. Nat Commun (2015) 6:10062. doi: 10.1038/ncomms10062
40. Cai W, Xu J, Li G, Liu T, Guo X, Wang H, et al. Ethanol extract of propolis prevents high-fat diet-induced insulin resistance and obesity in association with modulation of gut microbiota in mice. Food Res Int (2020) 130:108939. doi: 10.1016/j.foodres.2019.108939
41. Rodriguez-Diaz C, Taminiau B, Garcia-Garcia A, Cueto A, Robles-Diaz M, Ortega-Alonso A, et al. Microbiota diversity in nonalcoholic fatty liver disease and in drug-induced liver injury. Pharmacol Res (2022) 182:106348. doi: 10.1016/j.phrs.2022.106348
42. Zhao Q, Hou D, Fu Y, Xue Y, Guan X, Shen Q. Adzuki bean alleviates obesity and insulin resistance induced by a high-fat diet and modulates gut microbiota in mice. Nutrients (2021) 13(9):3240. doi: 10.3390/nu13093240
43. Zhong H, Abdullah, Deng L, Zhao M, Tang J, Liu T, et al. Probiotic-fermented blueberry juice prevents obesity and hyperglycemia in high fat diet-fed mice in association with modulating the gut microbiota. Food Funct (2020) 11(10):9192–207. doi: 10.1039/d0fo00334d
44. Li W, Zhang K, Yang H. Pectin alleviates high fat (Lard) diet-induced nonalcoholic fatty liver disease in mice: Possible role of short-chain fatty acids and gut microbiota regulated by pectin. J Agric Food Chem (2018) 66(30):8015–25. doi: 10.1021/acs.jafc.8b02979
45. Pan Y, Guo J, Hu N, Xun Y, Zhang B, Feng Q, et al. Distinct common signatures of gut microbiota associated with damp-heat syndrome in patients with different chronic liver diseases. Front Pharmacol (2022) 13:1027628. doi: 10.3389/fphar.2022.1027628
46. Pung HC, Lin WS, Lo YC, Hsu CC, Ho CT, Pan MH. Ulva prolifera polysaccharide exerts anti-obesity effects via upregulation of adiponectin expression and gut microbiota modulation in high-fat diet-fed C57BL/6 mice. J Food Drug Anal (2022) 30(1):46–61. doi: 10.38212/2224-6614.3395
47. Parker BJ, Wearsch PA, Veloo ACM, Rodriguez-Palacios A. The genus alistipes: Gut bacteria with emerging implications to inflammation, cancer, and mental health. Front Immunol (2020) 11:906. doi: 10.3389/fimmu.2020.00906
48. Shkoporov AN, Chaplin AV, Khokhlova EV, Shcherbakova VA, Motuzova OV, Bozhenko VK, et al. Alistipes inops sp. nov. and coprobacter secundus sp. nov., isolated from human faeces. Int J Syst Evol Microbiol (2015) 65(12):4580–8. doi: 10.1099/ijsem.0.000617
49. Feng Q, Liang S, Jia H, Stadlmayr A, Tang L, Lan Z, et al. Gut microbiome development along the colorectal adenoma-carcinoma sequence. Nat Commun (2015) 6:6528. doi: 10.1038/ncomms7528
50. Tsai KY, Wu DC, Wu WJ, Wang JW, Juan YS, Li CC, et al. Exploring the association between gut and urine microbiota and prostatic disease including benign prostatic hyperplasia and prostate cancer using 16S rRNA sequencing. Biomedicines (2022) 10(11):2676. doi: 10.3390/biomedicines10112676
51. Forbes JD, Chen CY, Knox NC, Marrie RA, El-Gabalawy H, de Kievit T, et al. A comparative study of the gut microbiota in immune-mediated inflammatory diseases-does a common dysbiosis exist? Microbiome (2018) 6(1):221. doi: 10.1186/s40168-018-0603-4
52. Wright EK, Kamm MA, Wagner J, Teo SM, Cruz P, Hamilton AL, et al. Microbial factors associated with postoperative crohn's disease recurrence. J Crohns Colitis (2017) 11(2):191–203. doi: 10.1093/ecco-jcc/jjw136
53. Osborne G, Wu F, Yang L, Kelly D, Hu J, Li H, et al. The association between gut microbiome and anthropometric measurements in bangladesh. Gut Microbes (2020) 11(1):63–76. doi: 10.1080/19490976.2019.1614394
54. He Y, Wu W, Wu S, Zheng HM, Li P, Sheng HF, et al. Linking gut microbiota, metabolic syndrome and economic status based on a population-level analysis. Microbiome (2018) 6(1):172. doi: 10.1186/s40168-018-0557-6
55. Zeng Q, Li D, He Y, Li Y, Yang Z, Zhao X, et al. Discrepant gut microbiota markers for the classification of obesity-related metabolic abnormalities. Sci Rep (2019) 9(1):13424. doi: 10.1038/s41598-019-49462-w
56. Tito RY, Chaffron S, Caenepeel C, Lima-Mendez G, Wang J, Vieira-Silva S, et al. Population-level analysis of blastocystis subtype prevalence and variation in the human gut microbiota. Gut (2019) 68(7):1180–9. doi: 10.1136/gutjnl-2018-316106
57. Le Chatelier E, Nielsen T, Qin J, Prifti E, Hildebrand F, Falony G, et al. Richness of human gut microbiome correlates with metabolic markers. Nature (2013) 500(7464):541–6. doi: 10.1038/nature12506
58. Vieira-Silva S, Falony G, Darzi Y, Lima-Mendez G, Garcia Yunta R, Okuda S, et al. Species-function relationships shape ecological properties of the human gut microbiome. Nat Microbiol (2016) 1(8):16088. doi: 10.1038/nmicrobiol.2016.88
59. Konikoff T, Gophna U. Oscillospira: a central, enigmatic component of the human gut microbiota. Trends Microbiol (2016) 24(7):523–4. doi: 10.1016/j.tim.2016.02.015
60. Zhang J, Guo Z, Xue Z, Sun Z, Zhang M, Wang L, et al. A phylo-functional core of gut microbiota in healthy young chinese cohorts across lifestyles, geography and ethnicities. ISME J (2015) 9(9):1979–90. doi: 10.1038/ismej.2015.11
61. Valles-Colomer M, Falony G, Darzi Y, Tigchelaar EF, Wang J, Tito RY, et al. The neuroactive potential of the human gut microbiota in quality of life and depression. Nat Microbiol (2019) 4(4):623–32. doi: 10.1038/s41564-018-0337-x
62. Precup G, Vodnar DC. Gut prevotella as a possible biomarker of diet and its eubiotic versus dysbiotic roles: a comprehensive literature review. Br J Nutr (2019) 122(2):131–40. doi: 10.1017/S0007114519000680
63. Duncan SH, Barcenilla A, Stewart CS, Pryde SE, Flint HJ. Acetate utilization and butyryl coenzyme a (CoA):acetate-CoA transferase in butyrate-producing bacteria from the human large intestine. Appl Environ Microbiol (2002) 68(10):5186–90. doi: 10.1128/AEM.68.10.5186-5190.2002
64. Hiippala K, Jouhten H, Ronkainen A, Hartikainen A, Kainulainen V, Jalanka J, et al. The potential of gut commensals in reinforcing intestinal barrier function and alleviating inflammation. Nutrients (2018) 10(8):988. doi: 10.3390/nu10080988
65. Li W, Zhu Y, Li Y, Shu M, Wen Y, Gao X, et al. The gut microbiota of hand, foot and mouth disease patients demonstrates down-regulated butyrate-producing bacteria and up-regulated inflammation-inducing bacteria. Acta Paediatr (2019) 108(6):1133–9. doi: 10.1111/apa.14644
66. Plovier H, Everard A, Druart C, Depommier C, Van Hul M, Geurts L, et al. A purified membrane protein from akkermansia muciniphila or the pasteurized bacterium improves metabolism in obese and diabetic mice. Nat Med (2017) 23(1):107–13. doi: 10.1038/nm.4236
67. Hou Q, Zhao F, Liu W, Lv R, Khine WWT, Han J, et al. Probiotic-directed modulation of gut microbiota is basal microbiome dependent. Gut Microbes (2020) 12(1):1736974. doi: 10.1080/19490976.2020.1736974
68. Chen YR, Jing QL, Chen FL, Zheng H, Chen LD, Yang ZC. Desulfovibrio is not always associated with adverse health effects in the guangdong gut microbiome project. PeerJ (2021) 9:e12033. doi: 10.7717/peerj.12033
69. Zhang L, Ouyang Y, Li H, Shen L, Ni Y, Fang Q, et al. Metabolic phenotypes and the gut microbiota in response to dietary resistant starch type 2 in normal-weight subjects: a randomized crossover trial. Sci Rep (2019) 9(1):4736. doi: 10.1038/s41598-018-38216-9
70. Wan F, Wang M, Zhong R, Chen L, Han H, Liu L, et al. Supplementation with chinese medicinal plant extracts from lonicera hypoglauca and scutellaria baicalensis mitigates colonic inflammation by regulating oxidative stress and gut microbiota in a colitis mouse model. Front Cell Infect Microbiol (2021) 11:798052. doi: 10.3389/fcimb.2021.798052
Keywords: alcoholic liver disease, nonalcoholic fatty liver disease, viral hepatitis, gut microbiota, gut microbiota-derived metabolites, mendelian randomization analysis
Citation: Zhang L, Zi L, Kuang T, Wang K, Qiu Z, Wu Z, Liu L, Liu R, Wang P and Wang W (2023) Investigating causal associations among gut microbiota, metabolites, and liver diseases: a Mendelian randomization study. Front. Endocrinol. 14:1159148. doi: 10.3389/fendo.2023.1159148
Received: 05 February 2023; Accepted: 13 April 2023;
Published: 05 July 2023.
Edited by:
Peiyuan Yin, Dalian Medical University, ChinaReviewed by:
Valentina Caputi, University College Cork, IrelandCan Liu, National University of Singapore, Singapore
Copyright © 2023 Zhang, Zi, Kuang, Wang, Qiu, Wu, Liu, Liu, Wang and Wang. This is an open-access article distributed under the terms of the Creative Commons Attribution License (CC BY). The use, distribution or reproduction in other forums is permitted, provided the original author(s) and the copyright owner(s) are credited and that the original publication in this journal is cited, in accordance with accepted academic practice. No use, distribution or reproduction is permitted which does not comply with these terms.
*Correspondence: Weixing Wang, wangwx@whu.edu.cn; Peng Wang, wangpeng01222022@163.com; Rongqiang Liu, freemanliurq@163.com
†These authors have contributed equally to this work