A ten long noncoding RNA-based prognostic risk model construction and mechanism study in the basal-like immune-suppressed subtype of triple-negative breast cancer
Introduction
Triple-negative breast cancer (TNBC), an important subtype of breast cancer, lacks the expression of estrogen receptor (ER), progesterone receptor (PR), and human epidermal growth factor receptor 2 (HER2). Compared with non-TNBC, TNBC is more aggressive, and has a higher rate of recurrence and metastasis (1). Therefore, there remains an urgent need to identify new strategies to better predict patient clinical outcomes. In 2019, Jiang et al. from Fudan University Shanghai Cancer Center (FUSCC) compiled the largest multiomics atlas of TNBC in the world. The FUSCC system further divides TNBC into four subtypes: (I) luminal androgen receptor (LAR), (II) immunomodulatory (IM), (III) basal-like immune-suppressed (BLIS), and (IV) mesenchymal-like (2). Subsequently, Jiang et al. conducted the comprehensive FUTURE trial (ClinicalTrials.gov registry no. NCT03805399) on metastatic TNBC to evaluate the efficacy of the system (3). However, the therapeutic results of corresponding targeted therapies were unsatisfactory. For the BLIS subtype, the anti-vascular endothelial growth factor (anti-VEGFR) objective response rate (ORR) was 26%, and the disease control rate (DCR) was 39%, while for the poly ADP-ribose polymerase (PARP) inhibitor group, the ORR was 0%, and the DCR was 0% (3). The therapeutic effect of different drugs on TNBC subtype is very different. Therefore, our study aimed to investigate the BLIS subtype in order to identify new therapeutic targets.
The length of long noncoding RNA (lncRNA) is about 200 bp, and its main function is to regulate gene expression, histone modification, and chromatin remodeling (4). It has been found that lncRNA significantly impacts tumor proliferation, invasion, metastasis, and other processes (5), and thus potential molecular mechanisms and signaling pathways may be used as key points in the diagnosis, prognosis, and treatment of patients with cancer. Although lncRNAs show promising therapeutic effects, their use is still hindered by issues related to immunogenicity, targeting specificity, and drug delivery (6). At present, there are no drugs that target lncRNAs available on the market or that are being assessed in clinical trials. To elucidate the complex mechanisms of lncRNA, we reviewed the role of lncRNA in gene regulation (7), the role of lncRNA in cancer progression (5), and the therapeutic strategies targeting lncRNA (8) (Figure 1). For example, Li et al. found that overexpression of lncRNA MAPT-A S1 exacerbated cell proliferation and metastasis in breast cancer (9). Hu et al. found that oncogenic lncRNAs downregulated cancer cell antigen presentation and intrinsic tumor inhibition (10). Guo et al. screened out epithelial-to-mesenchymal transition (EMT)-related lncRNAs and established a prognosis risk model for TNBC (11). Zhang et al. reported that GATA3-AS1 could facilitate tumor progression and immune escape in TNBC through the destabilization of GATA3 and the stabilization of programmed cell death-ligand 1 (PD-L1) (12). However, these studies analyzed TNBC as a whole and did not include in-depth analysis of the specific subtypes. Due to the important role of lncRNA in tumorigenesis and cancer cell proliferation, studies on the BLIS subtype focusing on lncRNA would improve our understanding of the properties of this subtype.
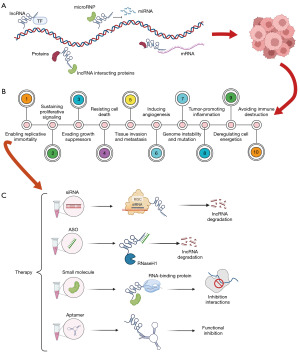
No specific targeted therapy for TNBC currently exists, and surgery and chemotherapy remain the only treatment options. In recent years, identifying new potential therapeutic targets has become a hotspot the research into TNBC treatment (13-15). In this study, we constructed a prognostic risk model based on lncRNAs and stratified BLIS patients with different prognoses. We then evaluated the risk model from a multiomics perspective, with signaling pathway, immune infiltration, variation spectrum, drug sensitivity, and clinical indicators being considered, and further explored the potential regulatory mechanism and therapeutic targets of the BLIS subtype. We present this article in accordance with the TRIPOD reporting checklist (available at https://tcr.amegroups.com/article/view/10.21037/tcr-23-147/rc).
Methods
Study design and data collection
The flow diagram of our study is displayed in Figure 2. The raw data of female Chinese patients with TNBC were obtained from FUSCC, which included 139 cases of the BLIS subtype. The data were stored in the National Omics Data Encyclopedia (NODE: OEP000155) (https://www.biosino.org/node/). Corresponding clinical information was also obtained from the literature (2). Log2[fragments per kilobase of transcript per million mapped reads (FPKM) + 1] expression data and the clinical data of 117 invasive breast carcinoma (BRCA) TNBC samples in The Cancer Genome Atlas (TCGA) were obtained from the UCSC Xena database (http://xena.ucsc.edu/).
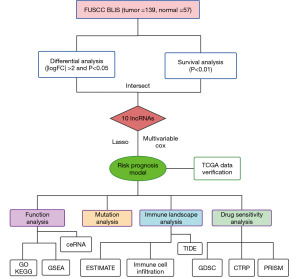
Raw reads were subjected to adaptor trimming and filtering of low-quality reads via FASTQ v. 0.21.0. We used HISAT2 v. 2.1.0 to align high-quality reads to the human reference genome (GRCh38) and generated sequence alignment map (SAM) files. The SAM files were converted to binary alignment map (BAM) files and sorted with SAMtools v. 1.11. To quantify the expression level of each gene in all samples, transcripts per million (TPM) were calculated with StringTie v. 2.1.2. Finally, the lncRNA matrix was extracted from the original data through the annotation file downloaded from the LNCipedia portal v 5.2 (https://lncipedia.org/), and the messenger RNA (mRNA) matrix was extracted from the original data through the annotation file on the UCSC portal (http://genome.ucsc.edu/). This study was conducted in accordance with the Declaration of Helsinki (as revised in 2013).
Identification of candidate lncRNA signatures
Differentially expressed lncRNAs were screened [|log fold change (FC)| >2 and P<0.01] with the R package “Limma” (The R Foundation for Statistical Computing) (16). A univariate Cox proportional risk regression model was used to screen for the lncRNAs that were significantly correlated with recurrence-free survival (RFS) time (P<0.05) via the “survival” “survminer” R package. The intersection of the two screening results was used to identify candidate lncRNA signatures.
Construction and validation of a prognostic model
Least absolute shrinkage and selection operator (LASSO) regression analysis was performed using the “glmnet” R package to further construct the prognostic model (17). After the expression value of each specific gene was included, the risk score formula for each patient was constructed and weighted by its estimated regression coefficient. The lncRNA risk model was formulated as follows: risk score =∑ (βf × Exp f), where βf represents the coefficient of f gene, and Exp f represents the expression value of F gene. According to the formula, BLIS patients in the training dataset were divided into a high-risk group and a low-risk group with the median risk score as the cutoff value. The survival difference between the two groups was assessed with the Kaplan-Meier survival curve. “SurvivalROC” R package was used to produce the receiver operating characteristic (ROC) curve for evaluating the prediction and differentiation ability of risk models over 1, 3, and 5 years (18). In addition, the TCGA-TNBC cohort was used to verify the performance of the model.
Functional analysis
The correlation between ten lncRNAs and mRNAs was calculated with the R package “Hmisc”, and the coexpressed lncRNA–mRNA pairs were predicted (positive correlation: r>0.5; negative correlation: r<−0.4; P<0.01). We used the “clusterProfiler” R package to execute Gene Ontology (GO) and Kyoto Encyclopedia of Genes and Genomes (KEGG) pathway assessment of the related mRNAs. A P value <0.05 was considered statistically significant (19). miRwalk (http://mirwalk.umm.uni-heidelberg.de) was used to predict the mRNA-miRNA interactions (20), and DIANA (https://diana.e-ce.uth.gr/lncbasev3) was used to predict the lncRNA-miRNA interactions (21). The predicted crossover results were constructed from the competing endogenous RNA (ceRNA) network and visualized using Cytoscape v. 3.9.0. In addition, we searched the literature on the PubMed database for the experimentally verified function of ten lncRNAs. Finally, we analyzed the differences between the high-risk group and the low-risk group using the R package “limma” to obtain the differential gene set and obtained the complete the Molecular Signatures Database (MSigDB) gene set using the “msigdbr” R package. The R package “clusterProfiler” was then used for gene set enrichment analysis (GSEA).
Immune landscape analysis
The “ESTIMATE” R package was applied to predict the stromal score and immune score among the different groups (22). “The xCell” R package was also used to evaluate the tumor microenvironment (TME) score in both groups (23). Tumor Immune Dysfunction and Exclusion (TIDE) (http://tide.dfci.harvard.edu/) was used to predict TIDE (24). Subsequently, the enrichment fractions of each immune cell type in the samples were calculated based on the single-sample gene set enrichment analysis (ssGSEA) to indicate the abundance of tumor-infiltrating immune cells. Finally, correlation analysis was conducted between the stromal score and the expression levels of immunotherapy markers.
Mutation analysis
Somatic mutation and copy number alteration in cancer were analyzed by VarScan2 software (25). Subsequently, we visualized somatic mutation data in mutation-annotated format (MAF) using the “maftools” R package, which provided a large number of analysis and visualization modules commonly used in cancer genome research (26). The OncodriveCLUST algorithm was used to detect the cancer driver genes in the protein-coding gene (27).
Drug sensitivity analysis
Using data from the Genomics of Drug Sensitivity in Cancer (GDSC) database (https://www.cancerrxgene.org/), we applied the “oncoPredict” R package to predict BLIS patients’ drug reaction (28). This R package uses ridge regression to build models and estimate drug responses from large amounts of cancer molecular data to identify relevant biomarkers. PubChem (https://pubchem.ncbi.nlm.nih.gov) was used to research the molecular structure of the drugs. The Cancer Therapeutics Response Portal (CTRP) database (https://portals.broadinstitute.org/ctrp.v2.1/) contains the sensitivity data for 481 compounds over 835 cancer cell lines (29), and the PRISM database (https://www.theprismlab.org/) contains the sensitivity data for 1,448 compounds over 482 cancer cell lines. We used the R package “pRRophetic” to construct ridge regression models and predict drug response based on the expression profile of BLIS subtypes to obtain area under the curve (AUC) estimates for each compound in each clinical sample. Connectivity Map (CMap) (https://clue.io/query) was used to search for drugs associated with gene expression patterns (differential analysis between BLIS subtypes and normal tissues), and compounds with CMap scores <−95, were considered to potentially exert a therapeutic effect in BLIS subtype patients. Details of the screened are is shown in Table S1.
Validation of RNA sequencing results through quantitative polymerase chain reaction (qPCR)
Mouse fibroblasts (3T3) were cultured in Dulbecco’s Modified Eagle Medium (DMEM) medium. Mouse breast cancer cells (4T1) were cultured in RPMI-1640 medium. The culture medium contained 10% fetal bovine serum (FBS), 100 U/mL of penicillin, and 100 µg/mL of streptomycin. The FBS used in RAW 264.7 cells was inactivated by incubation at 57 ℃ for 30 min. All cells were cultured in a cell incubator (37 ℃, 5% CO2). Total RNA samples were reverse transcribed to complement DNA (cDNA) using the RiboSCRIPT qRT-PCR Starter Kit (RiboBio, Guangzhou, China) according to the manufacturer’s instructions. qPCR was performed using the FastFire qPCR Premix (Tiangen Biotech, Beijing, China) on the CFX96 Real-Time PCR System (Bio-Rad Laboratories, Hercules, CA, USA). All the primers are presented in Table S2.
The quantitative reverse transcription PCR (RT-qPCR) amplification program was as follows: predenaturation at 95 ℃ for 30 s, followed by 40 cycles of 95 ℃ for 5 s and 60 ℃ for 30 s. The 2−ΔΔCt method was used to analyze the relative expression levels of lncRNAs and mRNAs.
Statistical analysis
The Wilcoxon test was applied to assess the expression differences of BLIS between tumor and normal tissues. RFS was evaluated using the Kaplan-Meier method and log-rank tests. The association of BLIS expression and stromal score was assessed with the Spearman rank correlation test. P<0.05 was considered to indicate a statistically significant difference. All statistical analyses were performed using RStudio (version 3.6.1).
Results
Identification of candidate lncRNA signatures
In the FUSCC dataset, 69 upregulated lncRNAs and 367 downregulated lncRNAs were found between the BLIS tumor and normal tissue samples. Univariate Cox regression analysis was conducted for each gene, and 191 lncRNAs were found to be significantly correlated with patient survival. Finally, ten overlapping lncRNA signatures were identified (Figure 3A-3C). Increased expression of C5ORF66-AS2, DIO3OS, FZD10-DT, LINC00393, LNC-ERI1-32, LNC-FOXO1-2, and LNC-SPARCL1-1 was associated with poor prognosis and decreased survival, while reduced expression of HCG23, LNC-MMD-4, and LNC-TMEM106C-6 was associated with poor prognosis and decreased survival (Figure 3D). We further found that the 10 lncRNAs showed consistent differential expression in the FUSCC cohort and TCGA cohort (Figure 3E,3F).
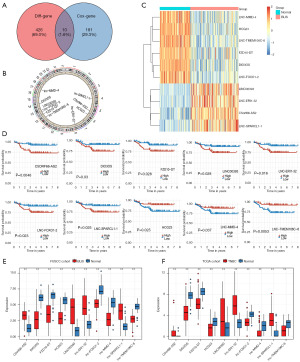
Construction and validation of a prognostic model
We further identified 10 lncRNAs closely associated with prognosis via LASSO regression, and multivariate Cox regression was used to construct a risk prognostic model (Figure 4). The forest plot showed that LNC-SPARCL1-1, LNC-FOXO1-2, LINC00393, DIO3OS, and C5ORF66-AS2 were significant protective factors, while LNC-MMD-4 was a significant risk factor (Figure 4A). The median risk score was used as the cutoff point to divide BLIS patients into high- and low-risk groups. The survival curve showed that patients in the low-risk group had a better RFS rate compared to the high-risk group (P=0.00091; Figure 4B). The risk curve and scatter plot showed that the risk coefficient and mortality in the low-risk group were lower than those in the high-risk group. A heatmap was used to visualize the expression level of ten lncRNAs between the two groups (Figure 4D). We further constructed a nomogram model to predict the 1-, 3-, and 5-year survival of BLIS patients using independent prognostic factor risk score and clinical information, including risk score, age, tumor stage, TMN stage, Ki-67 score, tumor size, stromal tumor-infiltrating lymphocytes (sTILs), intratumoral infiltrating lymphocytes (iTILs), and ERBB2 score. The results showed that the prognostic model could predict the survival rate of BLIS patients well (Figure 4H). The ROC curves showed that the AUC values of 1-, 3-, and 5-year survival prediction were 0.72, 0.84, and 0.91, respectively, indicating the good predictive ability of the prognostic model for BLIS patients (Figure 4C). TCGA cohort was used to validate the prognostic model. The results showed that there was also a significant difference in survival between the high- and low-risk groups, and the overall survival (OS) of the low-risk group was significantly higher than that of the high-risk group (P=0.013; Figure 4E,4F). The AUC values of 1-, 3-, and 5-year survival prediction were 0.78, 0.63, and 0.58 (Figure 4G). In general, these ten lncRNAs can be used as biomarkers for RFS prediction in BLIS patients, and the risk prognosis model constructed based on ten lncRNAs had good prognostic value in BLIS patients. The patient characteristics of the FUSCC training cohort (n=139) and TCGA external validation cohort (n=117) are shown in Table 1.
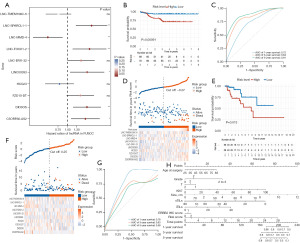
Table 1
Characteristic | FUSCC cohort (n=139) | TCGA cohort (n=117) | |||||||
---|---|---|---|---|---|---|---|---|---|
Total | High risk | Low risk | P | Total | High risk | Low risk | P | ||
Age | 0.152 | 0.685 | |||||||
<45 years | 42 | 18 | 24 | 27 | 14 | 13 | |||
≥45 years | 97 | 51 | 46 | 90 | 44 | 46 | |||
T stage | 0.329 | 0.004 | |||||||
T1 | 47 | 26 | 21 | 26 | 13 | 13 | |||
T2 | 89 | 42 | 47 | 76 | 33 | 43 | |||
T3 | 3 | 1 | 2 | 11 | 8 | 3 | |||
N stage | 0.02 | 0.08 | |||||||
N0 | 100 | 48 | 52 | 75 | 35 | 40 | |||
N1 | 23 | 12 | 11 | 27 | 13 | 14 | |||
N2 | 11 | 5 | 6 | 8 | 5 | 3 | |||
N3 | 5 | 4 | 1 | 7 | 5 | 2 | |||
Survival | <0.001 | <0.001 | |||||||
Alive | 117 | 51 | 66 | 98 | 44 | 54 | |||
Dead | 22 | 18 | 4 | 19 | 14 | 5 |
T stage clinical data were not available for four patients and were not included. FUSCC, Fudan University Shanghai Cancer Center; TCGA, The Cancer Genome Atlas.
Functional analysis
LncRNAs typically exert their function by regulating mRNA expression (30,31). In order to study the function of the ten lncRNAs in the model, we screened a total of 155 co-expressed lncRNA-mRNA genes through correlation analysis (Tables S3-S6). Of these genes, 37 were screened as upregulated genes (LINC00393, LNC-ERI1-32, C5ORF66-AS2 and LNC-SPARCL1-1; positive correlation: 16 pairs; negative correlation: 21 pairs). We performed KEGG analysis on these mRNAs. We found that the upregulated lncRNAs may participate in cell stromal-related pathways such as ECM-receptor interaction and focal adhesion by regulating the upregulation of related mRNAs (Figure 5A). Four lncRNAs were also found to regulate the downregulation of related mRNAs and participate in natural killer (NK) cell-mediated cytotoxicity, PD-L1 expression and programmed cell death 1 (PD-1) checkpoint pathway in cancer, and other immune-related pathways (Figure 5B). Additionally, 118 gene pairs were screened as downregulation genes (LNC-MMD-4, HCG23, LNC-TMEM106C-6, FZD10-DT, DIO3OS; positive correlation: 47 pairs; negative correlation: 71 pairs). Serotonergic synapse, chemokine signaling pathway, and other signaling pathways were found to be involved in regulating the upregulation and downregulation of associated mRNAs (Figure 5C). These mRNAs were also found to take participate in neurodegeneration diseases related to Parkinson disease and Alzheimer disease (Figure 5D). GO enrichment analysis showed that these gene-mediated biological processes were related to extracellular matrix and immune cells (Figure S1). LncRNA can change the expression level of target mRNA by competitive binding to microRNA (miRNA), thus affecting some of the biological characteristics of cancer, such as invasiveness, metastasis, and drug resistance (32). We constructed an ceRNA regulatory network based on 10 lncRNAs to clarify the potential regulatory relationship (Figure 5E). Furthermore, according to the GSEA results, the high-risk group was found to be associated with stromal-related and EMT pathway (Figure 5F). In order to enrich the functions of the model, PubMed was searched for literature related to the experimental verification of the six lncRNAs (LNC-FOXO1-2, LNC-ERI1-32, DIO3OS, HCG23, LINC00393, LNC-SPARCL1-1) (Table 2). We found that DIO3OS promoted the EMT of benign prostatic hyperplasia epithelial cells and the proliferation of prostatic stromal cells by regulating the target CTGF and ZEB1 (Table 2). Therefore, we inferred that the prognostic model based on the ten lncRNAs was associated with stromal pathways. DIO3OS has been shown to have an important role in the development of several cancers, including liver cancer. However, its role in TNBC remains unclear. We therefore aimed to characterize the role of DIO3OS in TNBC via qRT-PCR assay and lncRNA-mRNA coexpression analysis. qRT-PCR results showed that DIO3OS had a low expression in TNBC; was positively correlated with NOVA1, MFAP4, ABCA9, ACKR1, and AOX1 gene expression; and negatively correlated with TRIB3, PSMB5, and UQCRH gene expression (Figure 5G). Therefore, we hypothesized that DIO3OS could affect the progression of tumors by regulating the expression of related mRNAs.
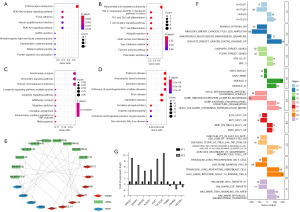
Table 2
LncRNA | mRNA-target | Disease | Experiment | Function | Reference |
---|---|---|---|---|---|
LNC-FOXO1-2 | CCND2 | – | Knockdown | Cell cycle regulation | (33) |
LNC-ERI1-32 | – | Kidney | Knockdown | Protein transport | (34) |
DIO3OS | CTGF, ZEB1 | BPH | Pull-down | EMT promotion and proliferation of stromal cells | (35) |
HCG23 | – | Pemphigus | RT-PCR | Cell adhesion Immunological signaling | (36) |
LINC00393 | – | BC | RT-PCR, WB | Th-cell differentiation T-cell migration | (37) |
LNC-SPARCL1-1 | HSPD1, MMP14, ITGB1 | NASH | RT-PCR | Aiding discrimination between NASH cases and control | (38) |
LncRNAs, long non-coding RNAs; BPH, benign prostatic hyperplasia; EMT, epithelial-to-mesenchymal transition; BC, breast cancer; RT-PCR, reverse transcription polymerase chain reaction; NASH, nonalcoholic steatohepatitis; WB, Western blot.
Immune landscape analysis
As KEGG analysis revealed that the BLIS subtype is BLIS and enriched in stromal- and immune-related pathways, we investigated whether this model is related to the TME (Figure 6). The “ESTIMATE” R package provides researchers with scores for the level of stromal cells present and the infiltration level of immune cells in tumor tissues based on expression profile. We found that there was no significant difference in immune score and TIDE score between the high-risk group and the low-risk group (Figure 6B-6D) because the immune regulatory pathway of BLIS subtype was inhibited and there was no significant internal difference (39). The stromal score in the high-risk group was significantly higher than that of the low-risk group (Figure 6A). Previous studies have shown that the key components of stromal in TME are conducive to the growth and metastasis of tumor cells, which may be one of the reasons for the poor prognosis of high-risk patients (40,41). To verify this conclusion, we also used the “xCell” R package to calculate the stromal score and immune score of the high- and low-risk groups and reached the same conclusion (Figure 6E,6F). Subsequently, the enrichment fractions of 23 immune cell types were calculated based on the ssGSEA algorithm. We found that B-cell infiltration abundance was higher in the low-risk group, while NK cell and neutrophil infiltration abundance was higher in the high-risk group (Figure 6G). Moreover, there was a slight correlation found between the expression of the 10 lncRNAs and immune cell infiltration abundance (Figure 6H), and the risk score was only positively correlated with stromal score, but not with other scores (Figure 6I). The expression of the immunotherapy biomarkers (CX3CL1, TNFSF4, VEGFA) were higher in the high-risk group than low-risk group, but the expression of immune checkpoints (PD-L1, CTLA4, PD-1) were not significantly different between the high-risk and low-risk groups (Figure 6J). Finally, we conducted correlation analysis on the stromal score and the expression levels of the immunotherapy biomarker and found that there was a significant positive correlation, which enriched the positive regulation of various immune-related biological processes (Figure 6K).
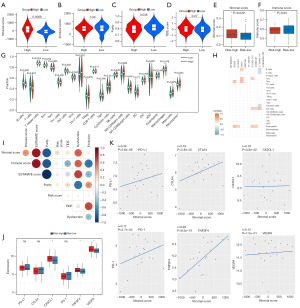
Mutation analysis
In the process of cell growth, the genome is constantly regulated by endogenous and exogenous DNA damage, and a mutation signal leads to a mutation that causes DNA damage. To gain a deeper understanding of the underlying genomic abnormalities driving the heterogeneity in the high- and low-risk groups, BLIS patients’ mutation profiles were obtained from raw data, and mutation analysis were performed using the “maftools” R package to reveal the environmental and endogenous sources of mutations in the high- and low-risk patients (Figure 7). The main variant classification of BLIS subtype was found to be missense mutation, while the main variant type of BLIS subtype was found to be single-nucleotide polymorphism (SNP) (Figure 7A). The mutation frequency of TP53, TTN, COL15A1, PKHD1L1, OBSCN, and MUC16 was higher in the high-risk group, while the mutation frequency of ANKRD11, FLG, USH2A, RB1, and RYR2 was higher in the low-risk group (Figure 7B). The OncodriveCLUST algorithm was used to identify the cancer driver genes, and it was found that the cancer driver genes in the high-risk group were COL15A1, TP53, and PKHD1L1, while the cancer driver gene in the low-risk group was TP53 (Figure 7C,7D). TP53 is a well-known tumor-suppressor gene that regulates cell growth, proliferation, and injury repair (42). COL15A1 is the member of the fibril-associated collagens with interrupted triple helices (FACITs) collagen family and may function by adhering basement membranes to underlying connective tissue stroma (43). PKHDL1 encodes a receptor with inducible T-lymphocyte expression (44). This suggests that patients with a higher stromal score are at a higher risk of mutations in COL15A1, which could be a potential therapeutic target in the high-risk group. A lollipop plot was created to visualize the mutation site in the protein structure of COL15A1, TP53, and PKHD1L1. In the high-risk group, the TP53 tetramer was more likely to be a mutation hotspot, with higher mutation frequency, while there was no mutation in the TP53 tetramer in the low-risk group (Figure 7E-7G). Patients with TP53 and COL15A1 mutations had a lower RFS, which may explain the poorer prognosis in the high-risk group (Figure 7J-7L). Finally, we also conducted enrichment analysis of carcinogenic pathways in the high- and low-risk groups and found that NFE2L2 pathway was enriched in the high-risk group and transforming growth factor-β (TGF-β) pathway was enriched in the low-risk group (Figure 7H,7I).
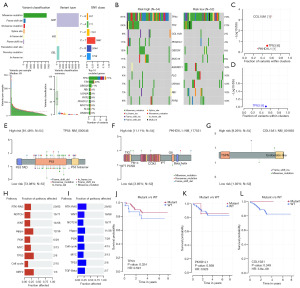
Drug sensitivity analysis
The FUTURE study indicated that BLIS patients may be insensitive to both PARP inhibitor and anti-VEGFR, so we aimed to clarify the relationship between risk groups and chemotherapy resistance by constructing a prognostic risk score model (Figure 8). The high-risk group was more sensitive to cyclophosphamide, zoledronate, fulvestrant, and JAK1, while the low-risk group was more sensitive to sinularin. Furthermore, we found that the high-risk group was more sensitive to stromal- and immune-related drugs [BMS-345541 (45), XAV939 (46), leflunomide (47), entinostat (48)], which correlated with the stromal properties of the high-risk group. The results showed that the model could be a potential predictor of chemical drug sensitivity (Figure 8A-8I). To identify additional therapeutic agents for patients in the high- and low-risk groups, we used the CTRP and PRISM databases to calculate drug sensitivity. The 10 candidate compounds identified showed a higher drug sensitivity in the high-risk score patients. We subsequently used CMap analysis to support the conclusion that these compounds had a therapeutic effect in the BLIS subtype. The results showed that 3 compounds, including BMS-754807, cytochalasin b, and linifanib, had CMap scores <−95, indicating that these compounds might exert a potential therapeutic effect in BLIS subtype patients (Figure 8J,8K).
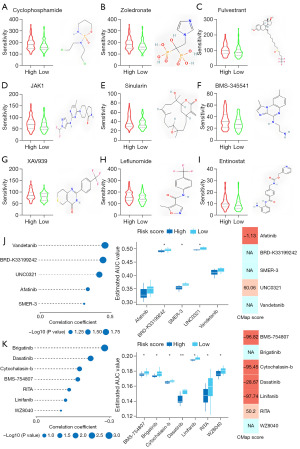
Discussion
In recent years, great efforts have been made to explore the pathogenesis of TNBC and improve the clinical outcomes of patients with this disease. Accurate prognosis has been shown to benefit healthcare decision-making and individualized treatment strategies (49,50). As a result, an increasing number of prognostic biomarkers have been identified, which can help in stratifying patients by predicting potential outcomes for tumor-related progression, recurrence, and survival (51,52). Previous researchers have proposed that lncRNAs are closely associated with the prognosis of TNBC (15). Therefore, the search for reliable lncRNA-related prognostic indicators may promote early risk stratification and improve treatment decisions for BLIS patients.
Previous studies constructed different models for TNBC based on TCGA public database. For example, Zhang et al. constructed and validated a risk prognostic model for BRC, and identified a robust immune-related signature including 10 genes (IL-10, C14orf79, C1orf168, C1orf226, CELSR2, FABP7, FGFBP1, KLRB1, PLEKHO1, and RAC2) (53). Yan et al. constructed and validated a risk prognostic model for TNBC based on CDKN1A, CTSD, CTSL, EIF4EBP1, TMEM74, and VAMP3 (54). However, TNBC is not a uniform disease and rather encompasses multiple entities with pronounced histopathological, transcriptomic, and genomic heterogeneity (55). It is thus necessary that TNBC be classified and its subtypes further characterized to deepen our understanding of the heterogeneity of TNBC and develop target precision medicine for patients. In this study, ten prognostic lncRNAs were identified via multivariate Cox and LASSO analysis, and a risk prognosis model of the BLIS subtype was constructed accordingly. The results suggested that these lncRNAs are critical for the stromal characterization of BLIS. Interestingly, DIO3OS, LINC00393, and HCG23 were highly correlated with the immune microenvironment. Low DIO3OS expression has been positively correlated with tumor immune infiltration (56). Epigenetically dysregulated lncRNA (LINC01983) can control T helper 17 (Th17)-cell differentiation, and T-cell migration (57), while HCG23 is involved in cell adhesion immunological signaling (36). The risk model divided BLIS patients into two risk groups, with poorer prognosis in the high-risk group and better prognosis in the low-risk group. Subsequently, we further verified the validity of our model using TCGA cohort. Our findings suggest that the ten lncRNAs play an important role in the progression of the BLIS subtype. In order to further clarify the biological function of this model in the BLIS subtype, we screened mRNAs with high correlation with the ten lncRNAs and performed GSEA and KEGG analysis. The results suggested that ten lncRNAs are critical to the stromal characterization of BLIS.
The FUTURE study also indicated that BLIS patients may be insensitive to both PARP inhibitor and anti-VEGFR chemotherapy, which represents a considerable challenge to the treatment of BLIS patients.
We screened potential therapeutic drugs for BLIS subtype patients via drug sensitivity analysis, but the relationship between the ten lncRNAs and these drugs remains unclear and warrants further study.
We found that the high-risk group showed strong stromal characteristics. Therefore, we speculated that higher levels of stromal cells may partly explain the poor prognosis in the high-risk BLIS patients. Stromal cells are important parts of the TME and are critically involved in tumorigenesis, and metastasis. Most anticancer drugs specifically target cancer cells, but stromal cells promote resistance to these therapies (57,58). Therefore, novel therapeutic strategies should combine anticancer drugs and anti-stromal drugs. Recently, immunotherapy has been intensely researched in relation to the treatment of TNBC, but there is a considerable degree of crosstalk between stromal cells and immune cells (59). In cancer, infiltrating immune cells establish a chemokine and cytokine environment that shapes the recruitment and activation of stromal cells within the tumor (60); therefore, the influence of stromal on immunotherapy cannot be ignored for patients with TNBC.
Conclusions
In this study, prognostic risk models were established based on ten independent prognostic lncRNAs. These four lncRNA-based prognostic risk models were used to divide patients with TNBC into high-risk and low-risk groups. The high-risk group had a poorer prognosis and more pronounced stromal characteristics than did the low-risk group. Thus, stromal characteristics may be linked to poor prognosis and represent a potential therapeutic direction. The ten lncRNAs can be used as a potential therapeutic target in patients with the BLIS subtype and aid in implementing precision medicine in patients with TNBC.
Acknowledgments
We would like to thank Dr. Jianming Zeng (University of Macau), all the members of his bioinformatics team, and Bio-trainee for generously sharing their experience and codes. We are grateful for being permitted to use the bio-RStudio high performance computing cluster (https://biorstudio.cloud) at Bio-trainee.
Funding: None.
Footnote
Reporting Checklist: The authors have completed the TRIPOD reporting checklist. Available at https://tcr.amegroups.com/article/view/10.21037/tcr-23-147/rc
Peer Review File: Available at https://tcr.amegroups.com/article/view/10.21037/tcr-23-147/prf
Conflicts of Interest: All authors have completed the ICMJE uniform disclosure form (available at https://tcr.amegroups.com/article/view/10.21037/tcr-23-147/coif). The authors have no conflicts of interest to declare.
Ethical Statement: The authors are accountable for all aspects of the work in ensuring that questions related to the accuracy or integrity of any part of the work are appropriately investigated and resolved. The study was conducted in accordance with the Declaration of Helsinki (as revised in 2013).
Open Access Statement: This is an Open Access article distributed in accordance with the Creative Commons Attribution-NonCommercial-NoDerivs 4.0 International License (CC BY-NC-ND 4.0), which permits the non-commercial replication and distribution of the article with the strict proviso that no changes or edits are made and the original work is properly cited (including links to both the formal publication through the relevant DOI and the license). See: https://creativecommons.org/licenses/by-nc-nd/4.0/.
References
- Shen M, Pan H, Chen Y, et al. A review of current progress in triple-negative breast cancer therapy. Open Med (Wars) 2020;15:1143-9. [Crossref] [PubMed]
- Jiang YZ, Ma D, Suo C, et al. Genomic and Transcriptomic Landscape of Triple-Negative Breast Cancers: Subtypes and Treatment Strategies. Cancer Cell 2019;35:428-440.e5. [Crossref] [PubMed]
- Jiang YZ, Liu Y, Xiao Y, et al. Molecular subtyping and genomic profiling expand precision medicine in refractory metastatic triple-negative breast cancer: the FUTURE trial. Cell Res 2021;31:178-86. [Crossref] [PubMed]
- Song Z, Lin J, Li Z, et al. The nuclear functions of long noncoding RNAs come into focus. Noncoding RNA Res 2021;6:70-9. [Crossref] [PubMed]
- Bach DH, Lee SK. Long noncoding RNAs in cancer cells. Cancer Lett 2018;419:152-66. [Crossref] [PubMed]
- Winkle M, El-Daly SM, Fabbri M, et al. Noncoding RNA therapeutics - challenges and potential solutions. Nat Rev Drug Discov 2021;20:629-51. [Crossref] [PubMed]
- Liu SJ, Dang HX, Lim DA, et al. Long noncoding RNAs in cancer metastasis. Nat Rev Cancer 2021;21:446-60. [Crossref] [PubMed]
- Chen D, Lu T, Tan J, et al. Long Non-coding RNAs as Communicators and Mediators Between the Tumor Microenvironment and Cancer Cells. Front Oncol 2019;9:739. [Crossref] [PubMed]
- Li B, Liu D, Xu J, et al. Overexpression of lncRNA MAPT-AS1 exacerbates cell proliferation and metastasis in breast cancer. Transl Cancer Res 2022;11:835-47. [Crossref] [PubMed]
- Hu Q, Ye Y, Chan LC, et al. Oncogenic lncRNA downregulates cancer cell antigen presentation and intrinsic tumor suppression. Nat Immunol 2019;20:835-51. [Crossref] [PubMed]
- Guo J, Yi X, Ji Z, et al. Development of a Prognostic Model Based on the Identification of EMT-Related lncRNAs in Triple-Negative Breast Cancer. J Oncol 2021;2021:9219961. [Crossref] [PubMed]
- Zhang M, Wang N, Song P, et al. LncRNA GATA3-AS1 facilitates tumour progression and immune escape in triple-negative breast cancer through destabilization of GATA3 but stabilization of PD-L1. Cell Prolif 2020;53:e12855. [Crossref] [PubMed]
- Fachal L, Aschard H, Beesley J, et al. Fine-mapping of 150 breast cancer risk regions identifies 191 likely target genes. Nat Genet 2020;52:56-73. [Crossref] [PubMed]
- Vagia E, Mahalingam D, Cristofanilli M. The Landscape of Targeted Therapies in TNBC. Cancers (Basel) 2020;12:916. [Crossref] [PubMed]
- Li YX, Wang SM, Li CQ. Four-lncRNA immune prognostic signature for triple-negative breast cancer Running title: Immune lncRNAs predict prognosis of TNBC. Math Biosci Eng 2021;18:3939-56. [Crossref] [PubMed]
- Ritchie ME, Phipson B, Wu D, et al. limma powers differential expression analyses for RNA-sequencing and microarray studies. Nucleic Acids Res 2015;43:e47. [Crossref] [PubMed]
- Friedman J, Hastie T, Tibshirani R. Regularization Paths for Generalized Linear Models via Coordinate Descent. J Stat Softw 2010;33:1-22. [Crossref] [PubMed]
- Heagerty PJ, Lumley T, Pepe MS. Time-dependent ROC curves for censored survival data and a diagnostic marker. Biometrics 2000;56:337-44. [Crossref] [PubMed]
- Wu T, Hu E, Xu S, et al. clusterProfiler 4.0: A universal enrichment tool for interpreting omics data. Innovation (Camb) 2021;2:100141. [Crossref] [PubMed]
- Sticht C, De La Torre C, Parveen A, et al. miRWalk: An online resource for prediction of microRNA binding sites. PLoS One 2018;13:e0206239. [Crossref] [PubMed]
- Karagkouni D, Paraskevopoulou MD, Tastsoglou S, et al. DIANA-LncBase v3: indexing experimentally supported miRNA targets on non-coding transcripts. Nucleic Acids Res 2020;48:D101-10. [PubMed]
- Yoshihara K, Shahmoradgoli M, Martínez E, et al. Inferring tumour purity and stromal and immune cell admixture from expression data. Nat Commun 2013;4:2612. [Crossref] [PubMed]
- Aran D, Hu Z, Butte AJ. xCell: digitally portraying the tissue cellular heterogeneity landscape. Genome Biol 2017;18:220. [Crossref] [PubMed]
- Jiang P, Gu S, Pan D, et al. Signatures of T cell dysfunction and exclusion predict cancer immunotherapy response. Nat Med 2018;24:1550-8. [Crossref] [PubMed]
- Koboldt DC, Zhang Q, Larson DE, et al. VarScan 2: somatic mutation and copy number alteration discovery in cancer by exome sequencing. Genome Res 2012;22:568-76. [Crossref] [PubMed]
- Mayakonda A, Lin DC, Assenov Y, et al. Maftools: efficient and comprehensive analysis of somatic variants in cancer. Genome Res 2018;28:1747-56. [Crossref] [PubMed]
- Tamborero D, Gonzalez-Perez A, Lopez-Bigas N. OncodriveCLUST: exploiting the positional clustering of somatic mutations to identify cancer genes. Bioinformatics 2013;29:2238-44. [Crossref] [PubMed]
- Maeser D, Gruener RF, Huang RS. oncoPredict: an R package for predicting in vivo or cancer patient drug response and biomarkers from cell line screening data. Brief Bioinform 2021;22:bbab260. [Crossref] [PubMed]
- Rees MG, Seashore-Ludlow B, Cheah JH, et al. Correlating chemical sensitivity and basal gene expression reveals mechanism of action. Nat Chem Biol 2016;12:109-16. [Crossref] [PubMed]
- Chen Y, Li Z, Chen X, et al. Long non-coding RNAs: From disease code to drug role. Acta Pharm Sin B 2021;11:340-54. [Crossref] [PubMed]
- Liu H, Wang S, Zhou S, et al. Drug Resistance-Related Competing Interactions of lncRNA and mRNA across 19 Cancer Types. Mol Ther Nucleic Acids 2019;16:442-51. [Crossref] [PubMed]
- Yang R, Xing L, Wang M, et al. Comprehensive Analysis of Differentially Expressed Profiles of lncRNAs/mRNAs and miRNAs with Associated ceRNA Networks in Triple-Negative Breast Cancer. Cell Physiol Biochem 2018;50:473-88. [Crossref] [PubMed]
- Jeong OS, Chae YC, Jung H, et al. Long noncoding RNA linc00598 regulates CCND2 transcription and modulates the G1 checkpoint. Sci Rep 2016;6:32172. [Crossref] [PubMed]
- Weirick T, Militello G, Ponomareva Y, et al. Logic programming to infer complex RNA expression patterns from RNA-seq data. Brief Bioinform 2018;19:199-209. [PubMed]
- Chen Y, Xu H, Liu C, et al. LncRNA DIO3OS regulated by TGF-β1 and resveratrol enhances epithelial mesenchymal transition of benign prostatic hyperplasia epithelial cells and proliferation of prostate stromal cells. Transl Androl Urol 2021;10:643-53. [Crossref] [PubMed]
- Salviano-Silva A, Becker M, Augusto DG, et al. Genetic association and differential expression of HLAComplexGroup lncRNAs in pemphigus. J Autoimmun 2021;123:102705. [Crossref] [PubMed]
- Zhao H, Liu X, Yu L, et al. Comprehensive landscape of epigenetic-dysregulated lncRNAs reveals a profound role of enhancers in carcinogenesis in BC subtypes. Mol Ther Nucleic Acids 2021;23:667-81. [Crossref] [PubMed]
- Albadawy R, Agwa SHA, Khairy E, et al. Clinical Significance of HSPD1/MMP14/ITGB1/miR-6881-5P/Lnc-SPARCL1-1:2 RNA Panel in NAFLD/NASH Diagnosis: Egyptian Pilot Study. Biomedicines 2021;9:1248. [Crossref] [PubMed]
- Liu YR, Jiang YZ, Xu XE, et al. Comprehensive transcriptome analysis identifies novel molecular subtypes and subtype-specific RNAs of triple-negative breast cancer. Breast Cancer Res 2016;18:33. [Crossref] [PubMed]
- Shen M, Jiang YZ, Wei Y, et al. Tinagl1 Suppresses Triple-Negative Breast Cancer Progression and Metastasis by Simultaneously Inhibiting Integrin/FAK and EGFR Signaling. Cancer Cell 2019;35:64-80.e7. [Crossref] [PubMed]
- Ham SL, Thakuri PS, Plaster M, et al. Three-dimensional tumor model mimics stromal - breast cancer cells signaling. Oncotarget 2017;9:249-67. [Crossref] [PubMed]
- Pollock NC, Ramroop JR, Hampel H, et al. Differences in somatic TP53 mutation type in breast tumors by race and receptor status. Breast Cancer Res Treat 2022;192:639-48. [Crossref] [PubMed]
- Hurskainen M, Ruggiero F, Hägg P, et al. Recombinant human collagen XV regulates cell adhesion and migration. J Biol Chem 2010;285:5258-65. [Crossref] [PubMed]
- Hogan MC, Griffin MD, Rossetti S, et al. PKHDL1, a homolog of the autosomal recessive polycystic kidney disease gene, encodes a receptor with inducible T lymphocyte expression. Hum Mol Genet 2003;12:685-98. [Crossref] [PubMed]
- Zhu X, Li Q, Hu G, et al. BMS-345541 inhibits airway inflammation and epithelial-mesenchymal transition in airway remodeling of asthmatic mice. Int J Mol Med 2018;42:1998-2008. [Crossref] [PubMed]
- Li C, Zheng X, Han Y, et al. XAV939 inhibits the proliferation and migration of lung adenocarcinoma A549 cells through the WNT pathway. Oncol Lett 2018;15:8973-82. [Crossref] [PubMed]
- Zhang C, Chu M. Leflunomide: A promising drug with good antitumor potential. Biochem Biophys Res Commun 2018;496:726-30. [Crossref] [PubMed]
- Truong AS, Zhou M, Krishnan B, et al. Entinostat induces antitumor immune responses through immune editing of tumor neoantigens. J Clin Invest 2021;131:e138560. [Crossref] [PubMed]
- Yang X, Weng X, Yang Y, et al. A combined hypoxia and immune gene signature for predicting survival and risk stratification in triple-negative breast cancer. Aging (Albany NY) 2021;13:19486-509. [Crossref] [PubMed]
- Yi J, Shuang Z, Zhong W, et al. Identification of Immune-Related Risk Characteristics and Prognostic Value of Immunophenotyping in TNBC. Front Genet 2021;12:730442. [Crossref] [PubMed]
- Domagala P, Jakubowska A, Jaworska-Bieniek K, et al. Prevalence of Germline Mutations in Genes Engaged in DNA Damage Repair by Homologous Recombination in Patients with Triple-Negative and Hereditary Non-Triple-Negative Breast Cancers. PLoS One 2015;10:e0130393. [Crossref] [PubMed]
- Sukumar J, Gast K, Quiroga D, et al. Triple-negative breast cancer: promising prognostic biomarkers currently in development. Expert Rev Anticancer Ther 2021;21:135-48. [Crossref] [PubMed]
- Zhang Y, Di X, Chen G, et al. An immune-related signature that to improve prognosis prediction of breast cancer. Am J Cancer Res 2021;11:1267-85. [PubMed]
- Yan C, Liu Q, Jia R. Construction and Validation of a Prognostic Risk Model for Triple-Negative Breast Cancer Based on Autophagy-Related Genes. Front Oncol 2022;12:829045. [Crossref] [PubMed]
- Marra A, Trapani D, Viale G, et al. Practical classification of triple-negative breast cancer: intratumoral heterogeneity, mechanisms of drug resistance, and novel therapies. NPJ Breast Cancer 2020;6:54. [Crossref] [PubMed]
- Wang Y, Wang J, Wang C, et al. DIO3OS as a potential biomarker of papillary thyroid cancer. Pathol Res Pract 2022;229:153695. [Crossref] [PubMed]
- Drain AP, Zahir N, Northey JJ, et al. Matrix compliance permits NF-κB activation to drive therapy resistance in breast cancer. J Exp Med 2021;218:e20191360. [Crossref] [PubMed]
- Fertal SA, Poterala JE, Ponik SM, et al. Stromal Characteristics and Impact on New Therapies for Metastatic Triple-Negative Breast Cancer. Cancers (Basel) 2022;14:1238. [Crossref] [PubMed]
- Garcia-Gomez A, Rodríguez-Ubreva J, Ballestar E. Epigenetic interplay between immune, stromal and cancer cells in the tumor microenvironment. Clin Immunol 2018;196:64-71. [Crossref] [PubMed]
- Turley SJ, Cremasco V, Astarita JL. Immunological hallmarks of stromal cells in the tumour microenvironment. Nat Rev Immunol 2015;15:669-82. [Crossref] [PubMed]