A predictive model for frequent exacerbator phenotype of acute exacerbations of chronic obstructive pulmonary disease
Highlight box
Key findings
• A new predictive model can be used to predict frequent exacerbator phenotype of acute exacerbations of chronic obstructive pulmonary disease (AECOPD).
What is known and what is new?
• Several biomarkers have been shown to be effective in assessing AECOPD severity in recent years.
• Some demographic characteristics and blood parameters can predict the frequency of acute exacerbations in the management of chronic obstructive pulmonary disease.
What is the implication, and what should change now?
• If a patient is at high risk of developing to the frequent exacerbator phenotype of AECOPD in the future, early clinical intervention strategies should be carried out.
Introduction
Background
Chronic obstructive pulmonary disease (COPD) is a prevalent chronic respiratory condition characterized by progressive and irreversible airflow obstruction and persistent pulmonary inflammation (1-3). It is a global epidemic affecting approximately 10% of the population (1) and is responsible for three million deaths annually (4), making it the third leading cause of death worldwide (5). With the expected increase in the average lifespan, the prevalence of age-related chronic diseases like COPD is projected to rise by 2050. Hospitalizations, comorbidities, morbidity, and mortality associated with COPD are also expected to increase, imposing a significant burden on healthcare, society, and the economy (6,7).
Acute exacerbations of chronic obstructive pulmonary disease (AECOPD) is a critical event in the progression of COPD and a primary cause of mortality (8). AECOPD is characterized by a sudden worsening of symptoms, accelerated deterioration in respiratory function, reduced quality of life, and increased healthcare costs (9,10). A previous study has reported that 46% of COPD patients experienced at least one exacerbation in the previous year, and 19% required hospitalization (11).
Rationale and knowledge gap
COPD is a heterogeneous disease with various clinical phenotypes. The frequent exacerbator phenotype is defined as the occurrence of two or more AECOPD episodes in the preceding year and is associated with poorer clinical outcomes (12,13). Consequently, it is now recognized as a distinct clinical subgroup, and predicting and detecting this phenotype early is crucial for better prevention and management of acute exacerbations (14,15). However, the prediction of this phenotype based solely on a patient’s exacerbation history in the previous year has limited effectiveness (16). Therefore, establishing a comprehensive prediction model for the frequent exacerbator phenotype is essential.
Previous studies have shown that various peripheral blood cell parameters, such as eosinophil counts, lymphocyte counts, monocyte counts, red cell distribution width, and mean platelet volume (MPV), are significantly correlated with the occurrence, clinical features, hospital readmission, prognosis, and mortality of AECOPD (17-22). The neutrophil-to-lymphocyte ratio (NLR) and platelet-to-lymphocyte ratio (PLR) have been reported to be associated with mortality in AECOPD patients (23). Another study evaluated 287 COPD exacerbation patients and found that the eosinophil-to-neutrophil ratio (ENR), eosinophil-to-monocyte ratio (EMR), and eosinophil-to-lymphocyte ratio (ELR) could be used to assess eosinophilic airway inflammation in COPD exacerbations, and may serve as new biomarkers for exacerbations in COPD (24). Recent reports have also highlighted the association between AECOPD and blood indicators such as fibrinogen (25,26), D-dimer (27), C-reactive protein (CRP), procalcitonin (PCT) (27,28), bilirubin levels (29) and the C-reactive protein to serum albumin ratio (CAR) (30). However, these biomarkers have limitations in predicting AECOPD, and very few studies have developed a model to predict the frequent exacerbator phenotype of AECOPD.
Objective
Therefore, this study aimed to investigate whether blood indicators are associated with the frequent exacerbator phenotype of AECOPD, identify potential biomarkers for predicting this phenotype, and develop a new prediction model based on objective blood parameters. We present this article in accordance with the TRIPOD reporting checklist (available at https://jtd.amegroups.com/article/view/10.21037/jtd-23-931/rc).
Methods
Study participants
This retrospective, single-center, observational study was conducted at the Department of Respiratory and Critical Care Medicine of the Second Affiliated Hospital of Xi’an Jiaotong University. From January 2017 to December 2022, a total of 7,281 inpatients with COPD were recruited from the Big Data Platform for Respiratory Medicine, an integrated database of electronic medical record systems. The inclusion criteria for AECOPD patients were: (I) meeting the diagnostic criteria for COPD set by GOLD guidelines (4), and (II) having at least one AECOPD hospitalization between 2017 and 2022, the diagnosis of AECOPD from doctors mainly depends on the clinical course of acute onset, that is, the deterioration of the respiratory system exceeds the daily variation, and the main symptoms is the aggravation of dyspnea, often accompanied by wheezing, chest tightness, cough, and increased sputum volume, et al. (4). The exclusion criterion was incomplete medical records (n=5,045). Finally, 2,236 patients were eligible for this study. The primary outcome was the frequency of AECOPD, and the primary endpoint was measured based on the frequency of hospitalization for acute exacerbations in the past year. Patients were divided into the frequent exacerbation group if they experienced two or more exacerbations within one year, while the remaining patients were placed in the infrequent exacerbation group. The detailed selection criteria of the study are presented in Figure 1.
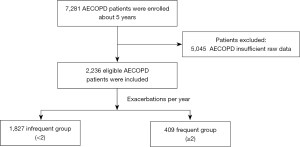
Ethical statement
The study was conducted in accordance with the Declaration of Helsinki (as revised in 2013). The study was approved by the institutional review board of the Second Affiliated Hospital of Xi’an Jiaotong University (No. 2023388) and individual consent for this retrospective analysis was waived.
Data collection
Baseline data of all enrolled patients, including demographic characteristics, comorbidities, examination data, and laboratory data, were obtained from the database of electronic medical record systems. Demographic information, such as gender, age, smoking status, hospital length of stay (LOS), and comorbidities such as diabetes, hypertension, heart failure, liver disease, and chronic renal disease, were collected. Complete blood counts, such as neutrophils, eosinophils, lymphocytes, monocytes, platelets, MPV, and other computable blood cell parameters, such as NLR, PLR, ENR, EMR, and ELR, were analyzed. Blood biochemistry, blood lipid, and coagulation function tests of AECOPD patients at hospital admission, including levels of bilirubin, γ-glutamyl transpeptidase, glucose, apoprotein B, fibrinogen, D-dimer, and others, were also collected.
Statistical analysis
All statistical analyses were performed using SAS (Statistical Analysis System, RRID:SCR_008567). The normal distribution of continuous variables was tested using the Shapiro-Wilk test. Data conforming to the normal distribution were expressed as mean ± standard deviation, while non-normally distributed data were expressed as median (interquartile range), and categorical variables were described by percentages (%). Univariate analysis was performed, and variables with P<0.05 in the univariate analysis were entered into multivariate logistic regression analysis. Based on the results of univariate analysis, multivariate logistic regression analysis was carried out to identify the various factors predicting the frequent exacerbator phenotype of AECOPD. The selected factors were used as independent variables, and the frequency of AECOPD within one year was used as the dependent variable to draw the receiver operator characteristic (ROC) curve. ROC curve analysis was used to evaluate the optimal cut-off value, area under the curve (AUC), sensitivity, and specificity of the factors for predicting the frequent exacerbator phenotype of AECOPD. Finally, we used R software (R Project for Statistical Computing, RRID:SCR_001905) to establish a nomogram risk prediction model. P values <0.05 were considered statistically significant in all tests.
Results
Baseline characteristics of the study participants
Table 1 shows that a total of 2,236 patients with AECOPD were included in the study. The electronic medical record systems of these patients were reviewed, and it was found that 1,546 (69.1%) were male, with a median age of 68 years. Of the total patients, 44.8% (n=1,001) had a history of smoking, while 53.9% (n=1,205) had never smoked. Additionally, the median LOS for all patients was 8 days.
Table 1
Characteristics | Values |
---|---|
Male | 1,546 (69.1) |
Age (years) | 68 [60–75] |
Smoking status | |
Never smoker | 1,205 (53.9) |
Former smoker | 510 (22.8) |
Current smoker | 491 (22.0) |
Unknown | 30 (1.3) |
Hypertension | 810 (36.2) |
Diabetes | 401 (17.9) |
Heart failure | 613 (27.4) |
Chronic renal disease | 43 (1.9) |
Liver disease | 642 (28.7) |
LOS (days) | 8 [7–11] |
Values are expressed as n (%) or median [interquartile range]. LOS, length of stay.
Univariate analysis assesses different predictors of frequent exacerbator phenotype of AECOPD
We observed that out of the 2,236 patients, 409 (18.3%) had developed the frequent exacerbator phenotype of AECOPD, while 1,827 (81.7%) patients did not. Significant differences were observed between the infrequent and frequent groups in terms of gender, age (≥78 years), smoking status, liver disease, and LOS (≥14 days) (P<0.001, P<0.001, P<0.001, P<0.001, P=0.01, respectively). However, other demographic variables, such as hypertension, diabetes, heart failure, and chronic renal disease, did not show significant differences between the two groups (Table 2).
Table 2
Characteristics | Infrequent exacerbation (n=1,827) |
Frequent exacerbation (n=409) |
P value |
---|---|---|---|
Gender | <0.001 | ||
Male | 1,225 (67.0) | 321 (78.5) | |
Female | 602 (33.0) | 88 (21.5) | |
Age (years) | <0.001 | ||
<78 | 1,508 (82.5) | 306 (74.8) | |
≥78 | 319 (17.5) | 103 (25.2) | |
Smoking status | <0.001 | ||
Never smoker | 1,018 (55.7) | 187 (45.7) | |
Former smoker | 385 (21.1) | 125 (30.6) | |
Current smoker | 398 (21.8) | 93 (22.7) | |
Unknown | 26 (1.4) | 4 (1.0) | |
Hypertension | 0.92 | ||
Yes | 661 (36.2) | 149 (36.4) | |
No | 1,166 (63.8) | 260 (63.6) | |
Diabetes | 0.07 | ||
Yes | 340 (18.6) | 61 (14.9) | |
No | 1,487 (81.4) | 348 (85.1) | |
Heart failure | 0.05 | ||
Yes | 485 (26.5) | 128 (31.3) | |
No | 1,342 (73.5) | 281 (68.7) | |
Chronic renal disease | 0.65 | ||
Yes | 34 (1.9) | 9 (2.2) | |
No | 1,793 (98.1) | 400 (97.8) | |
Liver disease | <0.001 | ||
Yes | 586 (32.1) | 56 (13.7) | |
No | 1,241 (67.9) | 353 (86.3) | |
LOS (days) | 0.01 | ||
<14 | 1,542 (84.4) | 324 (79.2) | |
≥14 | 285 (15.6) | 85 (20.8) |
Values are expressed as n (%). LOS, length of stay.
In terms of blood count parameters, significant differences were observed between the infrequent and frequent groups in neutrophils (>5.350×109/L), lymphocytes (>1.215×109/L), monocytes (>0.255×109/L), eosinophils (>0.275×109/L), and platelets (>155.500×109/L) (P=0.04, P=0.002, P=0.01, P=0.005, P=0.02, respectively). Additionally, significant differences were observed in PLR (>263.940) (P=0.04), but not in other computable blood cell parameters such as NLR, ELR, LMR, ENR, and EMR (Table 3).
Table 3
Variables | Infrequent exacerbation (n=1,827) |
Frequent exacerbation (n=409) |
P value |
---|---|---|---|
Neutrophils (×109/L) | 0.04 | ||
≤5.350 | 1,167 (63.9) | 283 (69.2) | |
>5.350 | 660 (36.1) | 126 (30.8) | |
Lymphocytes (×109/L) | 0.002 | ||
≤1.215 | 796 (43.6) | 212 (51.8) | |
>1.215 | 1,031 (56.4) | 197 (48.2) | |
Monocytes (×109/L) | 0.01 | ||
≤0.255 | 293 (16.0) | 87 (21.3) | |
>0.255 | 1,534 (84.0) | 322 (78.7) | |
Eosinophils (×109/L) | 0.005 | ||
≤0.275 | 1,604 (87.8) | 338 (82.6) | |
>0.275 | 223 (12.2) | 71 (17.4) | |
Platelets (×109/L) | 0.02 | ||
≤155.500 | 466 (25.5) | 126 (30.8) | |
>155.500 | 1,361 (74.5) | 283 (69.2) | |
MPV (fL) | 0.21 | ||
≤10.350 | 563 (30.8) | 139 (34.0) | |
>10.350 | 1,264 (69.2) | 270 (66.0) | |
Blood NLR | 0.09 | ||
≤2.415 | 596 (32.6) | 116 (28.4) | |
>2.415 | 1,231 (67.4) | 293 (71.6) | |
Blood PLR | 0.04 | ||
≤263.940 | 1,481 (81.1) | 314 (76.8) | |
>263.940 | 346 (18.9) | 95 (23.2) | |
Blood ELR | 0.08 | ||
≤0.185 | 1,571 (86.0) | 338 (82.6) | |
>0.185 | 256 (14.0) | 71 (17.4) | |
Blood LMR | 0.07 | ||
≤3.575 | 1,048 (57.4) | 254 (62.1) | |
>3.575 | 779 (42.6) | 155 (37.9) | |
Blood ENR | 0.12 | ||
≤0.055 | 1,456 (79.7) | 312 (76.3) | |
>0.055 | 371 (20.3) | 97 (23.7) | |
Blood EMR | 0.14 | ||
≤0.595 | 1,562 (85.5) | 338 (82.6) | |
>0.595 | 265 (14.5) | 71 (17.4) |
Values are expressed as n (%). MPV, mean platelet volume; NLR, neutrophil-to-lymphocyte ratio; PLR, platelet-to-lymphocyte ratio; ELR, eosinophil-to-lymphocyte ratio; LMR, lymphocyte-to-monocyte ratio; ENR, eosinophil-to-neutrophil ratio; EMR, eosinophil-to-monocyte ratio.
Total bilirubin (TBil) (>15.285 µmol/L), direct bilirubin (DBil) (>3.415 µmol/L), gamma-glutamyl transferase (GGT) (>22.500 U/L), blood glucose (>6.625 mmol/L), and glucose-to-lymphocyte ratio (GLR) (>3.675) showed significant differences between the infrequent and frequent groups (P=0.03, P=0.006, P<0.001, P=0.04, P=0.01, respectively). However, no significant differences were observed between the two groups in other laboratory data such as indirect bilirubin (IBil), albumin, D-dimers, fibrinogen, and ApoB (Table 4).
Table 4
Variables | Infrequent exacerbation (n=1,827) |
Frequent exacerbation (n=409) |
P value |
---|---|---|---|
TBil (μmol/L) | 0.03 | ||
≤15.285 | 1,315 (72.0) | 315 (77.0) | |
>15.285 | 512 (28.0) | 94 (23.0) | |
IBil (μmol/L) | 0.49 | ||
≤5.485 | 385 (21.1) | 80 (19.6) | |
>5.485 | 1,442 (78.9) | 329 (80.4) | |
DBil (μmol/L) | 0.006 | ||
≤3.415 | 970 (53.1) | 248 (60.6) | |
>3.415 | 857 (46.9) | 161 (39.4) | |
GGT (U/L) | <0.001 | ||
≤22.500 | 783 (42.9) | 214 (52.3) | |
>22.500 | 1,044 (57.1) | 195 (47.7) | |
Blood glucose (mmol/L) | 0.04 | ||
≤6.625 | 1,420 (77.7) | 336 (82.2) | |
>6.625 | 407 (22.3) | 73 (17.8) | |
GLR | 0.01 | ||
≤3.675 | 848 (46.4) | 161 (39.4) | |
>3.675 | 979 (53.6) | 248 (60.6) | |
Albumin (g/L) | 0.23 | ||
≤32.360 | 378 (20.7) | 74 (18.1) | |
>32.360 | 1,449 (79.3) | 335 (81.9) | |
D-dimers (μg/L) | 0.27 | ||
≤265 | 280 (15.3) | 54 (13.2) | |
>265 | 1,547 (84.7) | 355 (86.8) | |
Fibrinogen (g/L) | 0.13 | ||
≤10.785 | 356 (19.5) | 93 (22.7) | |
>10.785 | 1,471 (80.5) | 316 (77.3) | |
ApoB (g/L) | 0.12 | ||
≤0.935 | 1,358 (74.3) | 319 (78.0) | |
>0.935 | 469 (25.7) | 90 (22.0) |
Values are expressed as n (%). TBil, total bilirubin; IBil, indirect bilirubin; DBil, direct bilirubin; GGT, gamma-glutamyl transferase; GLR, glucose-to-lymphocyte ratio.
Multivariate logistic regression analysis assesses different predictors of frequent exacerbator phenotype of AECOPD
The results revealed that gender (male), age (≥78 years), liver disease (no), LOS (≥14 days), neutrophils (≤5.350×109/L), monocytes (≤0.255×109/L), eosinophils (>0.275×109/L), DBil (≤3.415 µmol/L), GGT (≤22.500 U/L), and GLR (>3.675) (P<0.05) were independent risk factors for the frequent exacerbator phenotype of AECOPD (Table 5). Figure 2 shows the associations between these factors and the occurrence of frequent exacerbator phenotype of AECOPD. In the regression model, male gender, age, LOS, eosinophils, and GLR were significantly positively correlated with the occurrence of frequent exacerbator phenotype of AECOPD, whereas liver disease, neutrophils, monocytes, DBil, and GGT were significantly negatively correlated with the occurrence of frequent exacerbator phenotype of AECOPD.
Table 5
Variables | Multivariate logistic regression | |
---|---|---|
OR (95% CI) | P value | |
Gender (male) | ||
Yes | 1.78 (1.36–2.33) | <0.001 |
No | – | – |
Age (≥78 years) | ||
Yes | 1.49 (1.14–1.95) | 0.004 |
No | – | – |
Liver disease | ||
Yes | 0.39 (0.29–0.54) | <0.001 |
No | – | – |
LOS (≥14 days) | ||
Yes | 1.55 (1.17–2.06) | 0.003 |
No | – | – |
Neutrophils (>5.350×109/L) | ||
Yes | 0.69 (0.54–0.88) | 0.003 |
No | – | – |
Monocytes (>0.255×109/L) | ||
Yes | 0.70 (0.52–0.93) | 0.01 |
No | – | – |
Eosinophils (>0.275×109/L) | ||
Yes | 1.59 (1.16–2.18) | 0.004 |
No | – | – |
DBil (>3.415 μmol/L) | ||
Yes | 0.68 (0.54–0.86) | 0.001 |
No | – | – |
GGT (>22.500 U/L) | ||
Yes | 0.78 (0.62–0.98) | 0.03 |
No | – | – |
GLR (>3.675) | ||
Yes | 1.38 (1.08–1.76) | 0.01 |
No | – | – |
OR, odds ratio; CI, confidence interval; LOS, length of stay; DBil, direct bilirubin; GGT, gamma-glutamyl transferase; GLR, glucose-to-lymphocyte ratio.
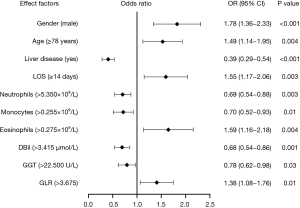
Diagnostic performance of combining predictors in prediction of frequent exacerbator phenotype of AECOPD
Subsequently, we performed an ROC curve analysis to evaluate the value of the combination of the above predictors in predicting the frequent exacerbator phenotype of AECOPD (Figure 3). The sensitivity and specificity were 63.6% [95% confidence interval (CI): 58.9–68.2%] and 65.0% (95% CI: 60.3–69.6%), respectively. The diagnostic accuracy, as measured by the AUC, was 0.681 (95% CI: 0.653–0.708).
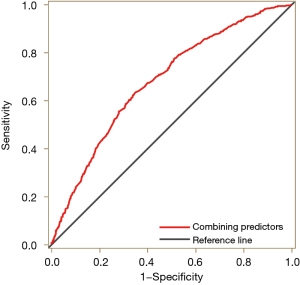
Establishing the corresponding nomogram risk prediction model of frequent exacerbator phenotype of AECOPD based on the above ten indicators
Finally, we constructed a nomogram model using the logistic regression algorithm to predict the frequent exacerbator phenotype of AECOPD patients (Figure 4). The predictors included gender, age, liver disease, neutrophils, monocytes, eosinophils, LOS, DBil, GGT, and GLR, which met the criteria of P<0.05 for risk assessment. Our nomogram provides a simple and accurate method for predicting the prognosis of AECOPD patients.
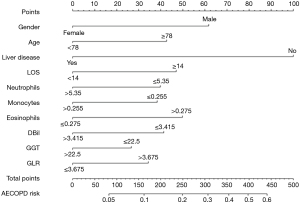
Discussion
Key findings
AECOPD is a significant event that reflects worsening of chronic airway inflammation and can lead to hospitalization and mortality (31,32). Clinical scoring systems such as Modified Medical Research Council (mMRC) and COPD Assessment Test (CAT) questionnaires are currently used to assess AECOPD, but objective blood indicators are needed to improve accuracy. This study aimed to identify reliable and simple parameters and biomarkers to predict frequent exacerbator phenotype of AECOPD. The results of multivariate logistic regression analysis indicated that gender (male), age (≥78 years), liver disease (no), LOS (≥14 days), neutrophils (≤5.350×109/L), monocytes (≤0.255×109/L), eosinophils (>0.275×109/L), DBil (≤3.415 µmol/L), GGT (≤22.500 U/L) and GLR (>3.675) were independent predictors for frequent exacerbator phenotype of AECOPD. By combining these predictors, ROC analysis showed improved predictive accuracy with better sensitivity and specificity. These objective blood indicators could be used to complement clinical scoring systems for accurate risk assessment of frequent exacerbator phenotype of AECOPD in clinical practice.
Strengths and limitations
Compared with other previous publication regarding biomarkers associated with AECOPD, our study has a strength in that it establishes a novel prediction model using rapid, inexpensive, and commonly available blood indicators and a large sample size. Furthermore, we discussed the frequent exacerbator phenotype of AECOPD, which is a significant phenotype of COPD characterized by experiencing at least two exacerbations per year and associated with the number of exacerbations. Therefore, we can draw up the treatment plan of stable period of COPD according to the evaluation results.
In spite of all efforts, our study is subject to some limitations, including the fact that blood indicators were measured cross-sectionally, these blood indicators results were the first measurements when they were admitted into the hospital, as the baseline characteristics. In our study, we divided AECOPD patients into the infrequent exacerbation group if they experienced less than two exacerbations within one year, while this group may be admitted to another hospital when experience another acute exacerbation. Furthermore, the difficulties in the construction this platform lie in how to integrate multisource heterogeneous data. Take pulmonary function as an example, data were stored in a third-party supplier and were failed to be plugged into this platform due to extremely high cost at this moment. The fact that some essential parameters of AECOPD patients were not included represents a significant limitation of this study, such as pulmonary function, clinical signs and drug therapy. This could impact the AUC of the prediction model to some extent. It is a single-center study without patient follow-up data, and that the patients included were heterogeneous in terms of AECOPD clinical severity, more clinical studies with higher sample size numbers are warranted to investigate. Additionally, patients were grouped based on hospitalization at a single tertiary hospital, which may not be representative of all COPD patients in the community, and it remains to be determined whether the predictive performance we were able to detect in our cohort can likewise be observed in other groups, so we will validate our predictive model in future through data source from other medical centers.
The granularity of the data needs to be continuously optimized upon research needs. We will include the image data in the near future, either by extract the key words of the image report by nature language processing or the image itself using deep learning image recognition. After gathering all the relevant data, we believe the precision of the prediction model will be highly improved.
Comparison with similar researches
In recent years, several studies have reported a strong association between neutrophils, monocytes, and eosinophils and AECOPD, which is consistent with our study findings. In the pathogenesis of COPD, inflammation and oxidative stress are crucial factors (33). Bilirubin, a potent antioxidant, has been associated with better lung function, slower lung function decline, and lower risk of incident of COPD and its progression (34-36). Dai et al. (29) found that bilirubin levels on admission may be related to the number of all-cause COPD-related readmissions, which can be regarded as a biomarker to predict readmission rates in the course of the COPD. In another research, Brown et al. found that bilirubin may be a biomarker of AECOPD risk and may be a novel therapeutic target to reduce AECOPD risk (37). A study from Sun et al. found that serum GGT level is inversely associated with pulmonary function, and it may serve as a biomarker during the progression of COPD to evaluate COPD and its exacerbation risk (38). A previous study was designed to assess the prognostic value of GLR in AECOPD patients admitted to intensive care unit (ICU), they demonstrated that GLR can independently predict the in-hospital mortality in AECOPD patients admitted to ICU (39).
Explanations of findings
The distribution of gender and age in our study was significantly associated with the frequency of AECOPD, indicating the influence of traditional risk factors on AECOPD. Our study identified a novel finding, that is the predictive accuracy of LOS in AECOPD patients, which means LOS and AECOPD are highly correlated. Our study found that lower levels of direct bilirubin (DBil ≤3.415 µmol/L) were independent risk factors for frequent exacerbator phenotype of AECOPD. Additionally, our results indicate that serum GGT levels and GLR can predict frequent exacerbator phenotype of AECOPD. Serum GGT levels have been suggested as a novel biomarker for oxidative stress and may serve as a biomarker to evaluate COPD and its exacerbation risk. Our study demonstrates that GLR >3.675 is an independent risk factor for frequent exacerbator phenotype of AECOPD. In conclusion, our study identifies DBil, GGT, and GLR as useful biomarkers for the early identification of further AECOPD exacerbations within one year.
Implications and actions needed
The frequent exacerbator phenotype of AECOPD is associated with significant negative outcomes, including decreased lung function, reduced quality of life, and increased mortality rates. Accurate and timely prediction of patients at risk for this phenotype is essential for effective management and treatment of COPD.
Conclusions
Our study presents a novel and reliable joint prediction model for identifying patients at risk of frequent exacerbator phenotype of AECOPD. According to our model, we can identify patients who are at the higher risk for COPD acute exacerbations occurring two or more times within 1 year. Thus, this model could provide an efficient tool for clinicians to identify high-risk COPD patients and implement preventive measures accordingly.
Acknowledgments
We would like to gratefully thank the staff of the Chongqing Nanpeng Artificial Intelligence Technology Research Institute Co., Ltd. for their assistance in the data extraction and data analysis of this study.
Funding: This work was supported by
Footnote
Reporting Checklist: The authors have completed the TRIPOD reporting checklist. Available at https://jtd.amegroups.com/article/view/10.21037/jtd-23-931/rc
Data Sharing Statement: Available at https://jtd.amegroups.com/article/view/10.21037/jtd-23-931/dss
Peer Review File: Available at https://jtd.amegroups.com/article/view/10.21037/jtd-23-931/prf
Conflicts of Interest: All authors have completed the ICMJE uniform disclosure form (available at https://jtd.amegroups.com/article/view/10.21037/jtd-23-931/coif). L.Z. and X.S. are employees of Chongqing Nanpeng Artificial Intelligence Technology Research Institute Co., Ltd. The other authors have no conflicts of interest to declare.
Ethical Statement: The authors are accountable for all aspects of the work in ensuring that questions related to the accuracy or integrity of any part of the work are appropriately investigated and resolved. The study was conducted in accordance with the Declaration of Helsinki (as revised in 2013). The study was approved by the institutional review board of the Second Affiliated Hospital of Xi’an Jiaotong University (No. 2023388) and individual consent for this retrospective analysis was waived.
Open Access Statement: This is an Open Access article distributed in accordance with the Creative Commons Attribution-NonCommercial-NoDerivs 4.0 International License (CC BY-NC-ND 4.0), which permits the non-commercial replication and distribution of the article with the strict proviso that no changes or edits are made and the original work is properly cited (including links to both the formal publication through the relevant DOI and the license). See: https://creativecommons.org/licenses/by-nc-nd/4.0/.
References
- Barnes PJ. Oxidative stress-based therapeutics in COPD. Redox Biol 2020;33:101544. [Crossref] [PubMed]
- Linden D, Guo-Parke H, Coyle PV, et al. Respiratory viral infection: a potential "missing link" in the pathogenesis of COPD. Eur Respir Rev 2019;28:180063. [Crossref] [PubMed]
- Guo-Parke H, Linden D, Weldon S, et al. Deciphering Respiratory-Virus-Associated Interferon Signaling in COPD Airway Epithelium. Medicina (Kaunas) 2022;58:121. [Crossref] [PubMed]
- Singh D, Agusti A, Anzueto A, et al. Global Strategy for the Diagnosis, Management, and Prevention of Chronic Obstructive Lung Disease: the GOLD science committee report 2019. Eur Respir J 2019;53:1900164. [Crossref] [PubMed]
- Guo-Parke H, Linden D, Weldon S, et al. Mechanisms of Virus-Induced Airway Immunity Dysfunction in the Pathogenesis of COPD Disease, Progression, and Exacerbation. Front Immunol 2020;11:1205. [Crossref] [PubMed]
- Guilleminault L, Rolland Y, Didier A. Characteristics of non-pharmacological interventions in the elderly with COPD. Smoking cessation, pulmonary rehabilitation, nutritional management and patient education. Rev Mal Respir 2018;35:626-41. [Crossref] [PubMed]
- Dall TM, Gallo PD, Chakrabarti R, et al. An aging population and growing disease burden will require a large and specialized health care workforce by 2025. Health Aff (Millwood) 2013;32:2013-20. [Crossref] [PubMed]
- Pépin JL, Degano B, Tamisier R, et al. Remote Monitoring for Prediction and Management of Acute Exacerbations in Chronic Obstructive Pulmonary Disease (AECOPD). Life (Basel) 2022;12:499. [Crossref] [PubMed]
- Hurst JR, Skolnik N, Hansen GJ, et al. Understanding the impact of chronic obstructive pulmonary disease exacerbations on patient health and quality of life. Eur J Intern Med 2020;73:1-6. [Crossref] [PubMed]
- Celli BR, Fabbri LM, Aaron SD, et al. An Updated Definition and Severity Classification of Chronic Obstructive Pulmonary Disease Exacerbations: The Rome Proposal. Am J Respir Crit Care Med 2021;204:1251-8. [Crossref] [PubMed]
- Lim S, Lam DC, Muttalif AR, et al. Impact of chronic obstructive pulmonary disease (COPD) in the Asia-Pacific region: the EPIC Asia population-based survey. Asia Pac Fam Med 2015;14:4. [Crossref] [PubMed]
- Wu YK, Su WL, Yang MC, et al. Characterization Associated with the Frequent Severe Exacerbator Phenotype in COPD Patients. Int J Chron Obstruct Pulmon Dis 2021;16:2475-85. [Crossref] [PubMed]
- Le Rouzic O, Roche N, Cortot AB, et al. Defining the "Frequent Exacerbator" Phenotype in COPD: A Hypothesis-Free Approach. Chest 2018;153:1106-15. [Crossref] [PubMed]
- Wilkinson TMA, Aris E, Bourne SC, et al. Drivers of year-to-year variation in exacerbation frequency of COPD: analysis of the AERIS cohort. ERJ Open Res 2019;5:00248-2018. [Crossref] [PubMed]
- Mirza S, Clay RD, Koslow MA, et al. COPD Guidelines: A Review of the 2018 GOLD Report. Mayo Clin Proc 2018;93:1488-502. [Crossref] [PubMed]
- Dang X, Kang Y, Wang X, et al. Frequent exacerbators of chronic obstructive pulmonary disease have distinguishable sputum microbiome signatures during clinical stability. Front Microbiol 2022;13:1037037. [Crossref] [PubMed]
- Hegewald MJ, Horne BD, Trudo F, et al. Blood Eosinophil Count and Hospital Readmission in Patients with Acute Exacerbation of Chronic Obstructive Pulmonary Disease. Int J Chron Obstruct Pulmon Dis 2020;15:2629-41. [Crossref] [PubMed]
- Ruiying W. Zhaoyun, Jianying X. Clinical features and three-year prognosis of AECOPD patients with different levels of blood eosinophils. Heart Lung 2022;56:29-39. [Crossref] [PubMed]
- Hu Y, Long H, Cao Y, et al. Prognostic value of lymphocyte count for in-hospital mortality in patients with severe AECOPD. BMC Pulm Med 2022;22:376. [Crossref] [PubMed]
- Zinellu A, Mangoni AA. The Emerging Clinical Significance of the Red Cell Distribution Width as a Biomarker in Chronic Obstructive Pulmonary Disease: A Systematic Review. J Clin Med 2022;11:5642. [Crossref] [PubMed]
- Ma Y, Zong D, Zhan Z, et al. Feasibility of mean platelet volume as a biomarker for chronic obstructive pulmonary disease: A systematic review and meta-analysis. J Int Med Res 2019;47:5937-49. [Crossref] [PubMed]
- Lin CH, Li YR, Lin PR, et al. Blood monocyte levels predict the risk of acute exacerbations of chronic obstructive pulmonary disease: a retrospective case-control study. Sci Rep 2022;12:21057. [Crossref] [PubMed]
- Luo Z, Zhang W, Chen L, et al. Prognostic Value of Neutrophil:Lymphocyte and Platelet:Lymphocyte Ratios for 28-Day Mortality of Patients with AECOPD. Int J Gen Med 2021;14:2839-48. [Crossref] [PubMed]
- Gao J, Chen B, Wu S, et al. Blood cell for the differentiation of airway inflammatory phenotypes in COPD exacerbations. BMC Pulm Med 2020;20:50. [Crossref] [PubMed]
- Sun W, Cao Z, Ma Y, et al. Fibrinogen, a Promising Marker to Evaluate Severity and Prognosis of Acute Exacerbation of Chronic Obstructive Pulmonary Disease: A Retrospective Observational Study. Int J Chron Obstruct Pulmon Dis 2022;17:1299-310. [Crossref] [PubMed]
- Mohan M, Parthasarathi A. Fibrinogen: A Feasible Biomarker in Identifying the Severity and Acute Exacerbation of Chronic Obstructive Pulmonary Disease. Cureus 2021;13:e16864. [Crossref] [PubMed]
- Bai JS, Liu JX, Zhang Q, et al. A Predictive Model Based on Blood Indicators for Admission to the ICU with AECOPD. Clin Lab 2022;68: [Crossref] [PubMed]
- Mou S, Zhang W, Deng Y, et al. Comparison of CRP, Procalcitonin, Neutrophil Counts, Eosinophil Counts, sTREM-1, and OPN between Pneumonic and Nonpneumonic Exacerbations in COPD Patients. Can Respir J 2022;2022:7609083. [Crossref] [PubMed]
- Dai L, Liang BM, Ou XM. Predictive value of neutrophil-to-lymphocyte ratio and bilirubin levels in the readmission of acute exacerbation of chronic obstructive pulmonary disease. Am J Med Sci 2023;365:169-75. [Crossref] [PubMed]
- Li H, Ma Y, Xue J, et al. C-Reactive Protein to Serum Albumin Ratio as a Novel Biomarker to Predict Prognosis in Patients with Chronic Obstructive Pulmonary Disease. Clin Lab 2021;67: [Crossref] [PubMed]
- Papi A, Luppi F, Franco F, et al. Pathophysiology of exacerbations of chronic obstructive pulmonary disease. Proc Am Thorac Soc 2006;3:245-51. [Crossref] [PubMed]
- Lima FV, Yen TY, Patel JK. Trends in In-Hospital Outcomes Among Adults Hospitalized With Exacerbation of Chronic Obstructive Pulmonary Disease. COPD 2015;12:636-42. [Crossref] [PubMed]
- Dhakal N, Lamsal M, Baral N, et al. Oxidative stress and nutritional status in chronic obstructive pulmonary disease. J Clin Diagn Res 2015;9:BC01-4. [Crossref] [PubMed]
- Curjuric I, Imboden M, Adam M, et al. Serum bilirubin is associated with lung function in a Swiss general population sample. Eur Respir J 2014;43:1278-88. [Crossref] [PubMed]
- Apperley S, Park HY, Holmes DT, et al. Serum Bilirubin and Disease Progression in Mild COPD. Chest 2015;148:169-75. [Crossref] [PubMed]
- Horsfall LJ, Rait G, Walters K, et al. Serum bilirubin and risk of respiratory disease and death. JAMA 2011;305:691-7. [Crossref] [PubMed]
- Brown KE, Sin DD, Voelker H, et al. Serum bilirubin and the risk of chronic obstructive pulmonary disease exacerbations. Respir Res 2017;18:179. [Crossref] [PubMed]
- Sun D, Liu H, Ouyang Y, et al. Serum Levels of Gamma-Glutamyltransferase During Stable and Acute Exacerbations of Chronic Obstructive Pulmonary Disease. Med Sci Monit 2020;26:e927771. [Crossref] [PubMed]
- Hu T, Liu X, Liu Y. Usefulness of Glucose to Lymphocyte Ratio to Predict in-Hospital Mortality in Patients with AECOPD Admitted to the Intensive Care Unit. COPD 2022;19:158-65. [Crossref] [PubMed]