The prognostic significance of a novel ferroptosis-related gene model in breast cancer
Introduction
Breast cancer (BRCA) is the most common malignancy and the leading cause of cancer-related death among women worldwide (1). It is a highly heterogeneous disease with complex and multiple etiologies, including genetic factors, hormone levels, lifestyle factors, and so on (2). With the advancement of multidisciplinary treatment of BRCA, surgery, chemotherapy, radiotherapy, targeted therapy, and hormonotherapy have comprised the main treatment strategies (3). However, the overall prognosis of BRCA has remained unfavorable, especially for advanced-stage patients (4). Due to the high level of heterogeneity and complex causes, predicting individual prognosis and developing precision treatment plans has remained challenging. Moreover, exploring the establishment of novel prognostic models may provide new insights for the treatment of BRCA.
Ferroptosis is a newly defined form of programmed cell death, which is activated by iron oxidation and lipid peroxidation (5,6). Since the discovery that iron-dependent nonapoptotic cell death is induced by small compounds (e.g., erastin, RSL3) and certain clinical drugs (e.g., sulfasalazine, sorafenib, artesunate), the induction of ferroptosis has become a potential therapeutic approach for patients that do not respond well traditional treatment (7,8). Previous studies have reported that ferroptosis has some connections to BRCA. Siramesine and lapatinib induce ferroptosis in BRCA cell lines by catalyzing the production of iron-dependent labile iron pool reactive oxygen species (LIP-ROS) (9). It has also been reported that some triple-negative breast cancer (TNBC) cell lines are sensitive to ferroptosis pathways (10,11). Several genes have been identified as drivers or suppressors in the progress of BRCA, such as ACSL4, which regulates sensitivity to ferroptosis by changing the composition of cellular lipid (12), and PROM2, which plays a role in ferroptosis resistance of BRCA cells (13). Many clinical-pathological and genetic factors are related to the prognosis of BRCA, such as tumor size, grade, lymph node metastasis, hormone receptors status, BRCA gene mutation, etc. Nevertheless, the relationship between ferroptosis-related genes and the prognosis of BRCA patients is still largely unclear.
Nowadays, a single treatment did not meet the expected therapeutic effect, and a combination of chemotherapy, endocrine therapy and immunotherapy is be in development. Exploring the ferroptosis-related genes in BRCA and their correlation with the immune microenvironment may provide a new treatment trend. In this study, we aimed to develop a ferroptosis-related signature for BRCA by performing bioinformatics analysis. Moreover, we tried to explore the association between tumor immunity and ferroptosis by gene and functional enrichment analysis.
We present the following article in accordance with the Transparent Reporting of a multivariable prediction model for Individual Prognosis or Diagnosis (TRIPOD) reporting checklist (available at https://atm.amegroups.com/article/view/10.21037/atm-22-479/rc).
Methods
Data download
The RNA sequencing (RNA-seq) data of 1,222 human breast tissue samples, consisting of 1,109 BRCA samples and 113 adjacent normal samples, were derived from The Cancer Genome Atlas (TCGA; https://tcga-data.nci.nih.gov/tcga/). Survival data and clinicopathological information of 1,097 BRCA patients were also acquired from TCGA database for training data. Another cohort of 327 BRCA patients was downloaded from the Gene Expression Omnibus (GEO; https://www.ncbi.nlm.nih.gov/geo/) validation data. The gene expression and clinical data of these samples were collected from GSE20685 based on the Affymetrix Human Genome U133 Plus 2.0 Array platform (Affymetrix, Santa Clara, CA, USA). A total of 259 identified ferroptosis-related genes including 90 divers, 55 suppressors, 86 markers, and 28 multi-annotated genes were obtained from the FerrDb database (http://www.zhounan.org/ferrdb/index.html) (14). All data above is available to the public, so the ethical approval of this study was waived by the local Ethics Committee. The study was conducted in accordance with the Declaration of Helsinki (as revised in 2013).
Differentially expressed ferroptosis-related genes
To identify differentially expressed genes (DEGs) between BCRA tissue samples and adjacent normal samples, we used the ‘limma’ R package (https://www.r-project.org/), and defined genes with fold change (FC) >1.5 as upregulated genes and those with FC <0.67 as downregulated genes. The false discovery rate (FDR) <0.05 was set as the cut-off threshold. By taking the intersection between DEGs from TCGA and the ferroptosis-related gene list, ferroptosis-related DEGs (FeffDEGs) were acquired successfully.
Development and validation of the prognostic model
Genes with a P value <0.05 were considered to be associated with the outcome of patients in the univariate Cox model, and prognostic ferroptosis-related ones were acquired from the overlapping area of survival-related genes and FeffDEGs. The protein-protein interaction (PPI) network of prognostic FeffDEGs was analyzed based on the Search Tool for the Retrieval of Interacting Genes/Proteins database (STRING; (https://string-db.org/). To better display the correlation coefficients intuitively between different candidate genes, the ‘igraph’ and ‘reshape’ R packages were also used to construct the network. Multivariate Cox analysis forward stepwise regression was used to further screen variables and obtain the best gene model. The regression coefficients (coef) and expression of each gene in a multivariate Cox model was used to calculate the risk score of each BRCA patient based on the following formula: risk score = Σ (expression of gene) × coef. Then, patients were evenly divided into 2 groups with the median risk score as the cut-off value. Principal component analysis (PCA) was performed by the ‘stats’ R package to evaluate gene expression distribution between different groups (15). As another dimensionality reduction tool, t-distributed stochastic neighbor embedding (t-SNE) was carried out by the ‘Rtsne’ R package (16). Kaplan-Meier survival analysis was carried out using the ‘survival’ and ‘survminer’ R packages. Receiver operating characteristic (ROC) curves were drawn using the ‘survivalROC’ R package to evaluate the accuracy of the prognostic model (17). The area under the curve (AUC) values range 0 to 1, with a value larger than 0.7 representing moderately accurate. The above validations were both performed in the TCGA and GEO datasets. Moreover, the protein expression levels of the selected genes in normal and tumor tissues were confirmed by the Human Protein Atlas database (HPA; https://www.proteinatlas.org/) to further verify the gene signature (18).
Construction of a nomogram model
Univariate and multivariate Cox regression analyses were performed to explore independent factors for the outcome of BRCA patients. The nomograms of the TCGA and GEO datasets were established based on the independent variables by the ‘rms’ R package. The calibration curves were plotted to show the models’ predictive performance concerning BRCA patients’ survival.
Functional enrichment analysis
Gene ontology (GO) annotation and Kyoto Encyclopedia of Genes and Genomes (KEGG) pathway enrichment analyses between 2 the groups of patients were performed with the ‘clusterProfiler’ R package (19). An FDR <0.05 and |log2FC| ≥1 were set as the cut-off values. Single sample gene set enrichment analysis (ssGSEA) was used to quantify the infiltrating level of immune cells and the activity of immune pathways for every gene set (20). To explore the relationship between immune status and risk score, ssGSEA analysis was utilized based on the ‘gsva’ R package.
Statistical analysis
We adjusted P values with Benjamini and Hochberg correction. The Mann-Whitney test method was carried out in ssGSEA analysis. The statistical software R version 4.0.2 (The R Foundation for Statistical Computing, Vienna, Austria) and SPSS version 26.0 (IBM Corp., Armonk, NY, USA) were utilized for all statistical analyses. A two-tailed P value <0.05 indicated statistical significance.
Results
Patients
A total of 1,424 BRCA patients, consisting of 1,097 cases from the TCGA database and 327 cases from the GEO database were included in this study. The detailed clinicopathological information is shown in Table 1. A simple flowchart of this study is shown in Figure 1.
Table 1
Characteristics | Training cohort, TCGA (n=1,097) | Validation cohort, GSE (n=327) |
---|---|---|
Age (median ± SD, years) | 58±13.22 | 46±1.69 |
Gender, n (%) | ||
Female | 1,085 (98.9) | NA |
Male | 12 (1.1) | NA |
T stage, n (%) | ||
T1 | 281 (25.6) | 101 (30.9) |
T2 | 635 (57.9) | 188 (57.5) |
T3 | 138 (12.6) | 26 (8.0) |
T4 | 40 (3.6) | 12 (3.7) |
Unknown | 3 (<0.1) | 0 (0.0) |
N stage, n (%) | ||
N0 | 516 (47.0) | 137 (41.9) |
N1 | 364 (33.2) | 87 (26.6) |
N2 | 120 (10.9) | 63 (19.3) |
N3 | 77 (7.0) | 40 (12.2) |
Unknown | 20 (1.8) | 0 (0.0) |
M stage, n (%) | ||
M0 | 912 (83.1) | 319 (97.6) |
M1 | 22 (0.2) | 8 (2.4) |
Unknown | 163 (14.9) | 0 (0.0) |
PR, n (%) | ||
Negative | 344 (31.4) | NA |
Positive | 699 (63.7) | NA |
Unknown | 54 (4.9) | NA |
ER, n (%) | ||
Negative | 238 (21.7) | NA |
Positive | 808 (73.7) | NA |
Unknown | 51 (4.6) | NA |
HER-2, n (%) | ||
Negative | 919 (83.8) | NA |
Positive | 79 (7.2) | NA |
Unknown | 99 (9.0) | NA |
BRCA, breast cancer; TCGA, The Cancer Genome Atlas; SD, standard deviation; T, tumor; N, node; M, metastasis; PR, progesterone receptor; ER, estrogen receptor; HER-2, human epidermal growth factor receptor-2; NA, not applicable.
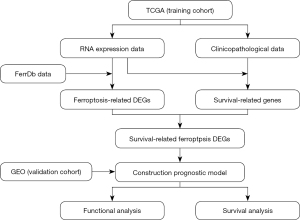
Identification of prognostic FeffDEGs
Through comparison of the expression of ferroptosis-related genes of tumor tissues and normal tissues, a total of 111 genes were identified as differentially expressed, including 49 down-regulated genes and 62 up-regulated genes. After univariate Cox regression analysis, there were 30 ferroptosis-related genes associated with OS. Therefore, we obtained 14 prognostic FeffDEGs based on the Venn diagram (Figure 2A), and a heatmap was created (Figure 2B). A PPI network was constructed based on the STRING database (Figure 2C). A correlation network of these genes quantifying the correlation coefficients through color depth is also shown in Figure 2D.
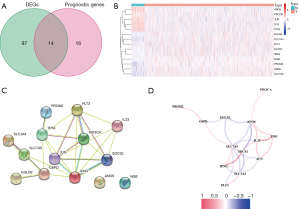
Construction and internal validation of a prognostic model
All of the 14 prognostic FeffDEGs were put into a multivariate Cox regression analysis, and eventually, 9 genes were identified as statistically significant (P<0.05) (Table 2). The staining intensity of G6PD, PROM2, NGB, IFNG and SLC1A4 in BRCA tissues were all higher than those in normal breast tissues, while the staining intensity of PIK3CA, ANO6 and TP63 were low in tumor tissues. Regrettably, the immunohistochemical analysis of FLT3 was unavailable (Figure 3). The risk score of each patient = 0.250256 × expression level of G6PD + 0.385493 × expression level of PIK3CA − 0.22191 × expression level of FLT3 − 0.62006 × expression level of IFNG + 0.457144 × expression level of ANO6 − 0.28589 × expression level of TP63 + 0.244391 × expression level of PROM2 − 0.28949 × SLC1A4 + 0.379179 × NGB. All patients were equally divided into a lower risk score group and a higher risk score group based on the median value of risk score. The risk score distribution and outcome status showed that more deaths happened in higher risk score groups (Figure 4A,4B). Also, PCA and t-SNE graphical analyses both displayed that this risk model divided patients into 2 opposite directions (Figure 4C,4D). The Kaplan-Meier curves illustrated that patients with lower risk scores had better survival than those with higher scores (Figure 4E). The AUC was 0.713 at 3 years, 0.713 at 5 years, and 0.684 at 10 years, which suggested the model had good predictive power (Figure 4F). Additionally, we explored the relationship between the ferroptosis risk score and the clinical characteristics of BCRA patients (Figure 5). The boxplot graphs showed the risk scores of patients with T4 tumor were significantly higher than those with T1, T2, or T3 tumors. Hormone receptor (HR)-positive BCRA patients had a lower risk score than HR-negative patents [progesterone receptor (PR): P<0.001, estrogen receptor (ER): P<0.001].
Table 2
Gene | Coef | HR (95% CI) | P value |
---|---|---|---|
G6PD | 0.250256 | 1.29 (1.05–1.56) | 0.012985 |
PIK3CA | 0.385493 | 1.47 (1.07–2.01) | 0.016308 |
FLT3 | −0.22191 | 0.80 (0.66–0.98) | 0.027936 |
IFNG | −0.62006 | 0.54 (0.34–0.84) | 0.006603 |
ANO6 | 0.457144 | 1.58 (1.14–2.18) | 0.005618 |
TP63 | −0.28589 | 0.75 (0.63–0.90) | 0.002007 |
PROM2 | 0.244391 | 1.27 (1.03–1.58) | 0.02628 |
SLC1A4 | −0.28949 | 0.75 (0.62–0.91) | 0.002991 |
NGB | 0.379179 | 1.46 (1.05–2.03) | 0.023989 |
HR, hazard ratio; CI, confidence interval.
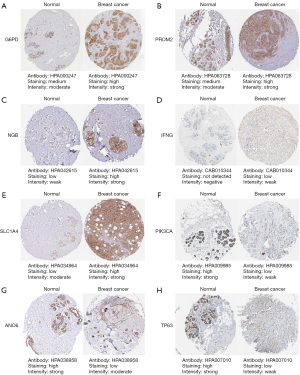
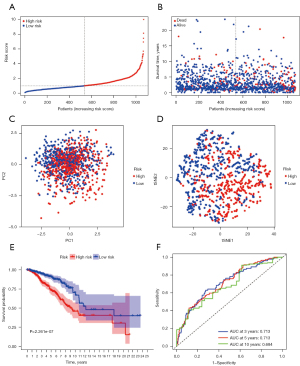
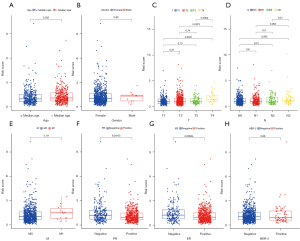
External validation and independent prognostic value of the model
We used BRCA patients from GSE20685 as an external validation cohort to further test the performance of the above model. Based on the same formula obtained in the training cohort, cases of the validation cohort were also categorized into a lower or higher risk score. The association between the outcome of patients and risk score groups is visualized in Figure 6A,6B. The PCA and t-SNE analyses showed that different distribution directions occurred between different risk score groups, which was similar to the above results (Figure 6C,6D). Patients from the GEO dataset with higher risk scores had a better outcome for OS (Figure 6E). The ROC analysis curve also certified the prognostic signature had a good predictive capability (Figure 6F). Additionally, to explore the clinical prognostic value of the 9-gene signature, the risk score had been looked at as a novel variable of each patient. Either in the TCGA cohort or the GEO cohort, the risk score was independently related to the OS of BCRA patients after univariate and multivariate Cox analyses (Table 3). Moreover, age and node (N) stage were also independently associated with outcome in TGCA cohort, and tumor (T) stage and N stage were identified as independent prognostic factors in the GEO cohort.
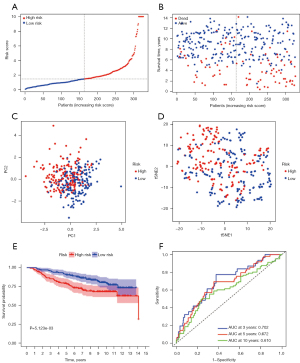
Table 3
Characteristics | Training cohort | Validation cohort | |||||||||
---|---|---|---|---|---|---|---|---|---|---|---|
Univariate | Multivariate | Univariate | Multivariate | ||||||||
HR (95% CI) | P value | HR (95% CI) | P value | HR (95% CI) | P value | HR (95% CI) | P value | ||||
Age (median) | 1.773 (1.158–2.713) |
0.008 | 1.914 (1.231–2.975) |
0.004 | 0.761 (0.492–1.177) |
0.219 | |||||
Gender (female/male) | 1.088 (1.151–7.841) |
0.933 | NA | ||||||||
T | |||||||||||
T1 | Ref | Ref | Ref | Ref | |||||||
T2 | 1.388 (0.806–2.392) |
0.237 | 1.233 (0.696–2.183) |
0.473 | 1.136 (0.664–1.943) |
0.643 | 0.763 (0.432–1.350) |
0.353 | |||
T3 | 1.663 (0.813–3.405) |
0.164 | 0.934 (0.423–2.066) |
0.867 | 4.777 (2.431–9.386) |
<0.001 | 2.352 (1.085–5.096) |
0.030 | |||
T4 | 4.862 (2.208–10.705) |
<0.001 | 2.351 (0.975–5.674) |
0.057 | 4.377 (1.923–9.960) |
<0.001 | 1.520 (0.485–4.762) |
0.472 | |||
N | |||||||||||
N0 | Ref | Ref | Ref | ||||||||
N1 | 1.350 (0.813–2.241) |
0.246 | 1.145 (0.662–1.982) |
0.627 | 2.400 (1.236–4.568) |
0.010 | 2.417 (1.229–4.753) |
0.011 | |||
N2 | 2.696 (1.471–4.944) |
0.001 | 2.931 (1.507–5.703) |
0.002 | 5.078 (2.728–9.450) |
<0.001 | 4.254 (2.173–8.330) |
<0.001 | |||
N3 | 4.925 (2.513–9.655) |
<0.001 | 3.026 (1.326–6.907) |
0.009 | 5.088 (2.538–10.201) |
<0.001 | 3.605 (1.662–7.819) |
0.001 | |||
M (M0/M1) | 4.428 (2.313–8.476) |
<0.001 | 1.665 (0.793–3.496) |
0.178 | 5.106 (2.345–11.121) |
<0.001 | 1.304 (0.425–4.003) |
0.643 | |||
PR (negative/positive) | 0.665 (0.435–1.017) |
0.060 | NA | ||||||||
ER (negative/positive) | 0.653 (0.416–1.026) |
0.064 | NA | ||||||||
HER-2 (negative/positive) | 1.903 (1.090–3.325) |
0.024 | 1.251 (0.686–2.281) |
0.465 | NA | ||||||
Risk (low/high) | 2.474 (1.589–3.853) |
<0.001 | 2.315 (1.468–3.648) |
<0.001 | 1.871 (1.197–2.926) |
0.006 | 1.629 (1.020–2.600) |
0.041 |
TCGA, The Cancer Genome Atlas; GEO, Gene Expression Omnibus; HR, hazard ratio; CI, confidence interval; T, tumor; N, node; M, metastasis; PR, progesterone receptor; ER, estrogen receptor; HER-2, human epidermal growth factor receptor-2; NA, not applicable.
Establishment and validation of the nomograms
To provide clinicians with more intuitive and easy-to-use prognostic models, nomograms were established based on independent factors. In the TCGA cohort, a nomogram with variables including age, N stage, and risk score, is shown in Figure 7A. As the calibration plots showed, the nomogram predicted 3-, 5-, and 10-year OS precisely, respectively (Figure 7B-7D). The nomogram using the independent variables in the GEO dataset, such as T stage, N stage, and risk score, showed excellent performance in 3-, 5-, and 10-year OS prediction (Figure 7E-7H).
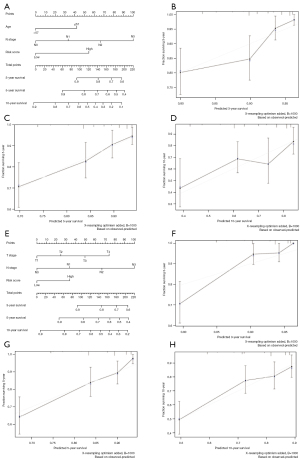
Functional enrichment analysis
To determine the association between the risk score and GO or biological pathways, gene functional enrichment analysis was used on DEGs between the different risk score groups of the TCGA cohort or GEO cohort. Cell component annotation analysis of the TCGA cohort showed that the DEGs were most significantly enriched in the mitochondrial matrix whose micro-structure would change when ferroptosis occurred. Immunoglobulin receptor binding, which is an immune-related molecular function was also significantly enriched (Figure 8A). Interestingly, 5 immune-related molecular functions were enriched in the GEO cohort, including immune receptor activity, antigen binding, cytokine receptor activity, major histocompatibility complex (MHC) protein compel binding, and MHC class II receptor activity. Besides, there were also many biological processes associated with immunity (Figure 8B). Some KEGG pathways, such as the chemokine signaling pathway, Th17 cell differentiation, T cell receptor signaling pathway, Th1 and Th2 cell differentiation, and programmed death-ligand 1 (PD-L1) expression and programmed cell death protein 1 (PD-1) checkpoint pathway in cancer, were both significantly enriched in both cohorts (Figure 8C,8D).
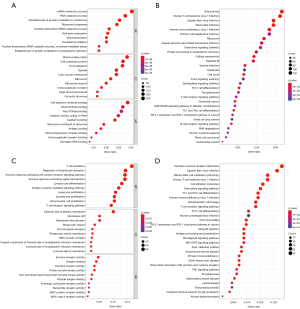
Differences of immune of status between patients with lower and higher risk scores
Through quantifying the infiltration levels of 16 immune cells, we found that either innate immune cells [dendritic cells (DC) and natural killer (NK) cells] or adaptive immune cells (B cells and kinds of T cells) infiltrated more in patients with lower risk score than those with higher risk score in both TCGA and GEO cohorts (Figure 9A,9B). Interestingly, the scores of some immune-related pathways, like antigen presentation process, chemokine receptor, checkpoint, T cell co-inhibition, and T cell co-stimulation were significantly different in lower and higher risk score patients, which were consistent with the results of KEGG pathway analyses (Figure 9C,9D).
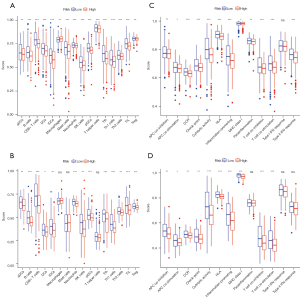
Data availability statement
All data of this study is openly available in The Cancer Genome Atlas (TCGA; https://tcga-data.nci.nih.gov/tcga/) database, Gene Expression Omnibus (GEO; https://www.ncbi.nlm.nih.gov/geo/) database, FerrDb database (http://www.zhounan.org/ferrdb/index.html), and the Human Protein Atlas (HPA; http://www.proteinatlas.org/) database.
Discussion
In this study, a ferroptosis-related signature that efficaciously stratified BRCA patients and predicted survival was successfully established based on the FerrDb and TCGA datasets. This predictive model exhibited excellent predictive ability in internal and external cohorts. Besides, we also confirmed that many immune cells and pathways were significantly different between different risk score patients classified by the model, and this might provide a new predictor for immunotherapy of BRCA.
Increasing numbers of genes have been demonstrated to be associated with the progress of ferroptosis in various carcinomas, and recently a well-curated database containing all ferroptosis-related genes identified so far was constructed (14). Some previous studies have suggested that PROM2 promotes ferroptosis resistance in BRCA cells through the PROM2-multiessiuclr bodies-exosome pathway and ACSL4 has a positive correlation to ferroptosis of human BRCA cell lines (12,13). However, the potential relationship between ferroptosis-related genes and the outcome of BRCA patients has remained unclear. Surprisingly, there were 30 ferroptosis-related genes identified as connected to the OS of BRCA patients in our study, among which ACSL4 was not included, and was thus not included in further analyses.
The proposed prognostic model comprises 9 genes, including PROM2, ANO6, FLT3, G6PD, IFNG, NGB, PIK3CA, SLC1A4, and TP63. As a pentaspanin protein implicated in regulating lipid dynamics, PROM2 facilitates resistance against ferroptosis in mammary epithelial and BRCA cells (13). The activation of ANO6 is an important component of ferroptotic cell death induced by RSL3/erastin (21). As a Ca2+-activated Cl− channel, ANO6 has an impact on cancer cell migration and a relationship with poor prognosis according to recent research (22). In this study, ANO6 was related to poor outcome in BRCA patients, but it was down-regulated in tumor tissues, and the underlying mechanisms require further analysis. As a class III receptor tyrosine kinase, FLT3 acts as an inhibitor in the blockade of ferroptotic cell death by reducing oxidative glutamate toxicity (23). In our research, FLT3 was related to poor survival while down-regulated in BRCA samples, which is consistent with the results in a previous study (24). As an integral part of the PI3K pathway, PIK3CA is one of the most recurrently mutated genes in BRCA (25). Both FLT3 and PIK3CA inhibitors have been shown to be potent protectors against glutamate toxicity that involves a combination of ferroptosis and apoptosis-inducing factor-dependent apoptosis through preventing ROS generation and lipid peroxidation (23). Glucose-6-phosphate dehydrogenase (G6PD), has been reported as overexpressed in BRCA and to promote migration through the G6PD/HIF-1α/Notch1 axis (26,27). Knocking down G6PD in non-small cell lung cancer cells can prevent cells from erastin-induced ferroptosis (5). The IFNG gene is a potent activator of macrophages that promotes tumor ferroptosis by downregulating the expression of subunits of the glutamate-cystine antiporter system Xc- (28). In this study, the high expression of IFNG was related to a better outcome of BRCA patients, which is similar to the finding of Yeong et al.’s research (29). As an oxygen-binding protein that can provide protection under hypoxic/ischemic conditions, NGB has endogenous neuroprotective effects to ferroptosis, with the evidence of 0.68 fold less cell death in erastin-stressed human neuroglobin-EGFP cells detected (30). In terms of TP63, it inhibits ferroptosis in human squamous cell lung cancer, in which it is frequently amplified (31). Solute carrier family 1 member 4 (SLC1A4) was significantly upregulated in cells resistant to ferroptosis and could act as a marker of ferroptosis (32). The specific underlying mechanisms of SLC1A4 in the process of ferroptosis remain poorly understood. In total, 5 of the genes (ANO6, FLT3, G6PD, IFNG, PIK3CA) are drivers in the progress of ferroptosis, while 2 of them (PROM2, TP63) are suppressors, and the other 2 genes (NGB, SLC1A4) act as markers. It is worth noting that only PROM2 was certified to promote resistance to ferroptosis of BRCA cancer cells among these genes, while whether the other genes promote or inhibit tumorigenesis and progression in BRCA through playing a role in ferroptosis of tumor cells still needs exploration.
Since Professor Dixon proposed that ferroptosis is a novel programmed cell death (33), the mechanisms of ferroptosis in various kinds of tumors has emerged as one of the currently most popular research directions. However, the potential link between ferroptosis and tumor immunity has remained poorly understood. In this study, we surprisingly found a lot of immune-related cells and biological pathways were significantly enriched by performing ssGSEA analyses for DEGs between the lower and higher risk score groups. Interestingly, higher-risk patients with worse survival had lower scores of immune cell infiltration, which was probably caused by weakening antitumor immunity in BRCA patients with higher risk scores. As the innate immune cells, DC and NK cells play an important role in cancer immunosurveillance (34), and infiltrated less in higher-risk patients. Meanwhile, T cells, which infiltrated more in lower risk samples, were abundantly enriched in breast tumor issues (35), and CD8+ T cells were certified to inhibit metastasis of BRCA and have a positive impact on survival in recent studies (36,37). Besides, the levels of antigen presentation process co-inhibition and co-stimulation were different between the 2 risk score groups, and the reason might be that ferroptosis would lead to the release of lipid mediators and the aggregation of antigen presentation cells (38). Additionally, lower risk scores were related to a high level of check point pathway, and this may be due to the high level of T cells in lower-risk patients. Therefore, it is reasonable to speculate that there is a close relationship between tumor immunity and ferroptosis, but the detailed connection requires further exploration.
Some limitations existed in this study. First, both the construction and validation of the prognostic model were based on retrospective public data, so more prospective studies are required to verify its accuracy and utility. Second, as an inevitable disadvantage of using a single marker to establish a prognostic signature, some remarkable prognostic genes for BRCA may have been missed. Meanwhile, some environmental and genetic factors closely related to the occurrence of BRCA are inevitably missed. Finally, some functional experimental analyses regarding the links between immunity and ferroptosis-related risk score should be further performed.
Conclusions
To sum up, a novel ferroptosis-related prognostic model for BRCA was defined in this study. The model exhibited excellent predictive ability in both internal and external validations, and the risk score calculated using the model was verified as an independent factor for the OS of BRCA patients. Immune status and related pathways were different between the 2 risk groups, which might provide a new research angle for ferroptosis-related cancer therapy, but potential underlying mechanisms between tumor immunity and ferroptosis in BRCA require further exploration.
Acknowledgments
Funding: This study was funded by grants from Suzhou Municipal Science and Technology Bureau (SLJ202011) and Jiangsu Commission of Health (M2020043).
Footnote
Reporting Checklist: The authors have completed the TRIPOD reporting checklist. Available at https://atm.amegroups.com/article/view/10.21037/atm-22-479/rc
Conflicts of Interest: All authors have completed the ICMJE uniform disclosure form (available at https://atm.amegroups.com/article/view/10.21037/atm-22-479/coif). The authors have no conflicts of interest to declare.
Ethical Statement: The authors are accountable for all aspects of the work in ensuring that questions related to the accuracy or integrity of any part of the work are appropriately investigated and resolved. All information from TCGA, GEO, FerrDb, and HPA databases is freely available to the public, so the approval of the medical ethics committee board was not necessary. The study was conducted in accordance with the Declaration of Helsinki (as revised in 2013).
Open Access Statement: This is an Open Access article distributed in accordance with the Creative Commons Attribution-NonCommercial-NoDerivs 4.0 International License (CC BY-NC-ND 4.0), which permits the non-commercial replication and distribution of the article with the strict proviso that no changes or edits are made and the original work is properly cited (including links to both the formal publication through the relevant DOI and the license). See: https://creativecommons.org/licenses/by-nc-nd/4.0/.
References
- Bray F, Ferlay J, Soerjomataram I, et al. Global cancer statistics 2018: GLOBOCAN estimates of incidence and mortality worldwide for 36 cancers in 185 countries. CA Cancer J Clin 2018;68:394-424. [Crossref] [PubMed]
- Song JL, Chen C, Yuan JP, et al. Progress in the clinical detection of heterogeneity in breast cancer. Cancer Med 2016;5:3475-88. [Crossref] [PubMed]
- Huang P, Li F, Li L, et al. lncRNA profile study reveals the mRNAs and lncRNAs associated with docetaxel resistance in breast cancer cells. Sci Rep 2018;8:17970. [Crossref] [PubMed]
- Tao Z, Shi A, Lu C, et al. Breast Cancer: Epidemiology and Etiology. Cell Biochem Biophys 2015;72:333-8. [Crossref] [PubMed]
- Dixon SJ, Lemberg KM, Lamprecht MR, et al. Ferroptosis: an iron-dependent form of nonapoptotic cell death. Cell 2012;149:1060-72. [Crossref] [PubMed]
- Gaschler MM, Andia AA, Liu H, et al. FINO2 initiates ferroptosis through GPX4 inactivation and iron oxidation. Nat Chem Biol 2018;14:507-15. [Crossref] [PubMed]
- Hassannia B, Vandenabeele P, Vanden Berghe T. Targeting Ferroptosis to Iron Out Cancer. Cancer Cell 2019;35:830-49. [Crossref] [PubMed]
- Zhou B, Liu J, Kang R, et al. Ferroptosis is a type of autophagy-dependent cell death. Semin Cancer Biol 2020;66:89-100. [Crossref] [PubMed]
- Ma S, Henson ES, Chen Y, et al. Ferroptosis is induced following siramesine and lapatinib treatment of breast cancer cells. Cell Death Dis 2016;7:e2307. [Crossref] [PubMed]
- Chen MS, Wang SF, Hsu CY, et al. CHAC1 degradation of glutathione enhances cystine-starvation-induced necroptosis and ferroptosis in human triple negative breast cancer cells via the GCN2-eIF2α-ATF4 pathway. Oncotarget 2017;8:114588-602. [Crossref] [PubMed]
- Yu M, Gai C, Li Z, et al. Targeted exosome-encapsulated erastin induced ferroptosis in triple negative breast cancer cells. Cancer Sci 2019;110:3173-82. [Crossref] [PubMed]
- Doll S, Proneth B, Tyurina YY, et al. ACSL4 dictates ferroptosis sensitivity by shaping cellular lipid composition. Nat Chem Biol 2017;13:91-8. [Crossref] [PubMed]
- Brown CW, Amante JJ, Chhoy P, et al. Prominin2 Drives Ferroptosis Resistance by Stimulating Iron Export. Dev Cell 2019;51:575-586.e4. [Crossref] [PubMed]
- Zhou N, Bao J. FerrDb: a manually curated resource for regulators and markers of ferroptosis and ferroptosis-disease associations. Database (Oxford) 2020;2020:baaa021.
- Yano K, Morinaka Y, Wang F, et al. GWAS with principal component analysis identifies a gene comprehensively controlling rice architecture. Proc Natl Acad Sci U S A 2019;116:21262-7. [Crossref] [PubMed]
- Cieslak MC, Castelfranco AM, Roncalli V, et al. t-Distributed Stochastic Neighbor Embedding (t-SNE): A tool for eco-physiological transcriptomic analysis. Mar Genomics 2020;51:100723. [Crossref] [PubMed]
- Heagerty PJ, Lumley T, Pepe MS. Time-dependent ROC curves for censored survival data and a diagnostic marker. Biometrics 2000;56:337-44. [Crossref] [PubMed]
- Asplund A, Edqvist PH, Schwenk JM, et al. Antibodies for profiling the human proteome-The Human Protein Atlas as a resource for cancer research. Proteomics 2012;12:2067-77. [Crossref] [PubMed]
- Yu G, Wang LG, Han Y, et al. clusterProfiler: an R package for comparing biological themes among gene clusters. OMICS 2012;16:284-7. [Crossref] [PubMed]
- Rooney MS, Shukla SA, Wu CJ, et al. Molecular and genetic properties of tumors associated with local immune cytolytic activity. Cell 2015;160:48-61. [Crossref] [PubMed]
- Ousingsawat J, Schreiber R, Kunzelmann K. TMEM16F/Anoctamin 6 in Ferroptotic Cell Death. Cancers (Basel) 2019;11:625. [Crossref] [PubMed]
- Jacobsen KS, Zeeberg K, Sauter DR, et al. The role of TMEM16A (ANO1) and TMEM16F (ANO6) in cell migration. Pflugers Arch 2013;465:1753-62. [Crossref] [PubMed]
- Kang Y, Tiziani S, Park G, Kaul M, Paternostro G. Cellular protection using Flt3 and PI3Kα inhibitors demonstrates multiple mechanisms of oxidative glutamate toxicity. Nat Commun 2014;5:3672. [Crossref] [PubMed]
- Srour MK, Gao B, Dadmanesh F, et al. Gene expression comparison between primary triple-negative breast cancer and paired axillary and sentinel lymph node metastasis. Breast J 2020;26:904-10. [Crossref] [PubMed]
- Yeang CH, McCormick F, Levine A. Combinatorial patterns of somatic gene mutations in cancer. FASEB J 2008;22:2605-22. [Crossref] [PubMed]
- Zhang HS, Zhang ZG, Du GY, et al. Nrf2 promotes breast cancer cell migration via up-regulation of G6PD/HIF-1α/Notch1 axis. J Cell Mol Med 2019;23:3451-63. [Crossref] [PubMed]
- Stanton RC. Glucose-6-phosphate dehydrogenase, NADPH, and cell survival. IUBMB Life 2012;64:362-9. [Crossref] [PubMed]
- Wang W, Green M, Choi JE, et al. CD8+ T cells regulate tumour ferroptosis during cancer immunotherapy. Nature 2019;569:270-4. [Crossref] [PubMed]
- Yeong J, Lim JCT, Lee B, et al. Prognostic value of CD8 + PD-1+ immune infiltrates and PDCD1 gene expression in triple negative breast cancer. J Immunother Cancer 2019;7:34. [Crossref] [PubMed]
- Van Acker ZP, Van Raemdonck GA, Logie E, et al. Connecting the Dots in the Neuroglobin-Protein Interaction Network of an Unstressed and Ferroptotic Cell Death Neuroblastoma Model. Cells 2019;8:873. [Crossref] [PubMed]
- Wang GX, Tu HC, Dong Y, et al. ΔNp63 Inhibits Oxidative Stress-Induced Cell Death, Including Ferroptosis, and Cooperates with the BCL-2 Family to Promote Clonogenic Survival. Cell Rep 2017;21:2926-39. [Crossref] [PubMed]
- Dixon SJ, Patel DN, Welsch M, et al. Pharmacological inhibition of cystine-glutamate exchange induces endoplasmic reticulum stress and ferroptosis. Elife 2014;3:e02523. [Crossref] [PubMed]
- Dixon SJ. Ferroptosis: bug or feature? Immunol Rev 2017;277:150-7. [Crossref] [PubMed]
- Burr ML, Sparbier CE, Chan KL, et al. An Evolutionarily Conserved Function of Polycomb Silences the MHC Class I Antigen Presentation Pathway and Enables Immune Evasion in Cancer. Cancer Cell 2019;36:385-401.e8. [Crossref] [PubMed]
- Zhao Y, Pu C, Liu Z. Exploration the Significance of a Novel Immune-Related Gene Signature in Prognosis and Immune Microenvironment of Breast Cancer. Front Oncol 2020;10:1211. [Crossref] [PubMed]
- Mahmoud SM, Paish EC, Powe DG, et al. Tumor-infiltrating CD8+ lymphocytes predict clinical outcome in breast cancer. J Clin Oncol 2011;29:1949-55. [Crossref] [PubMed]
- Matsumoto H, Thike AA, Li H, et al. Increased CD4 and CD8-positive T cell infiltrate signifies good prognosis in a subset of triple-negative breast cancer. Breast Cancer Res Treat 2016;156:237-47. [Crossref] [PubMed]
- Friedmann Angeli JP, Krysko DV, Conrad M. Ferroptosis at the crossroads of cancer-acquired drug resistance and immune evasion. Nat Rev Cancer 2019;19:405-14. [Crossref] [PubMed]
(English Language Editor: J. Jones)