Metabolic abnormalities, rather than body mass index, associated with increased risk of recurrent stroke in hospitalized stroke patients: a cross-sectional study
Introduction
Obesity as a worldwide epidemic has emerged as a critical public health issue because of its considerable effect on several diseases, such as stroke, cardiovascular disease, diabetes, hypertension, and cancer (1). Abundant evidence suggests that obesity is also a risk factor for stroke. Being overweight or obese is associated with a significantly increased risk of stroke (2), and each unit increase in body mass index (BMI) has been associated with a significant 6% increase in relative risk (3). However, for secondary prevention, some prospective studies reported that obese patients had a lower risk of recurrent stroke (4), and overweight and mildly obese patients had better 10-year survival after stroke than normal-weight patients (5). The association between obesity and recurrent stroke is undetermined. An unfavorable metabolic status may be the cause of differences in recurrent stroke incidence between obese and non-obese patients (6).
Metabolic syndrome (MetS), an integrated state of metabolic disorders, including elevated plasma glycemia, central obesity, hypertension, and dyslipidemia, is an essential predictor of recurrent stroke, with patients having a 52% increased risk (7). However, among the components of MetS, obesity has a lower influence than other risk factors, whether on initial (8) or recurrent stroke (9,10). A meta-analysis of cohort studies showed that the hazard ratio of obesity for recurrent stroke was 1.12 (0.89–1.41), significantly lower than that of elevated glycemia and dyslipidemia (9). Furthermore, a multi-clinic study among mild-to-moderate stroke patients did not find a significant association between BMI and 5-year risk of recurrent stroke, whereas elevated blood pressure, elevated glycemia, and low high-density lipoprotein cholesterol (HDL-c) showed a significantly higher risk of recurrent stroke (11). Therefore, we can assume that recurrent stroke is probably influenced by obesity in conjunction with metabolic status.
Whether or not recurrent stroke is more likely related to poor metabolic health rather than obesity remains uncertain, while conflicting data have been similarly reported among new-onset stroke patients. A previous study concluded that obese individuals with an otherwise metabolically healthy profile, referred to as “metabolically healthy obesity (MHO),” did not have a higher risk of stroke than metabolically healthy non-obese patients (10). However, non-obese but metabolically unhealthy patients experienced a higher risk of stroke, and the risk increased as the number of MetS components increased (12). The discrepancies may be attributed to different BMI cut-off point for the obesity definition and elder participants than the prior study.
Thus, the present study aimed to compare metabolic phenotypes and BMI as indicators of recurrent stroke in Chinese hospitalized stroke patients and proposed the hypothesis that an unhealthy metabolic status is more closely associated with a high risk of recurrent stroke than obesity. We present the following article in accordance with the STROBE reporting checklist (available at https://atm.amegroups.com/article/view/10.21037/atm-22-4606/rc).
Methods
Study population
This cross-sectional study was conducted among hospitalized patients diagnosed with cerebrovascular diseases in the First People’s Hospital of Changzhou, Third Affiliated Hospital of Soochow University. From January 2017 to January 2018, a total of 870 participants were recruited with the following inclusion criteria: (I) patients agreed to participate in the present study and signed an informed consent form; and (II) the cerebrovascular diseases were diagnosed according to computed tomography (CT) or magnetic resonance imaging (MRI) scans. Each patient underwent a face-to-face interview for a standard questionnaire and provided blood samples for biochemical measurements. For the present analysis, the exclusion criteria were as follows: (I) patients with subarachnoid hemorrhaging or a transient ischemic attack without progression to stroke or lacunar infarction (n=7); (II) missing information on lipid profiles, blood pressure, and plasma glucose (n=4); and (III) an extreme BMI value (n=3). Eventually, 856 participants were enrolled in the present study.
This study was conducted in accordance with the Declaration of Helsinki (as revised in 2013). The study was approved by the Ethics Committee of the First People’s Hospital of Changzhou, Third Affiliated Hospital of Soochow University (No. 2020025) and informed consent was taken from all the patients.
Data collection
A face-to-face interview was performed with a standardized questionnaire to collect information on sociodemographic characteristics, lifestyle, and medical history. Current smokers and drinkers were defined as those who had smoked cigarettes and consumed alcohol in the past 6 months. Physical activity was divided into high physical activity or not, based on the International Physical Activity Questionnaire (IPAQ). Dietary habit was classified as predominantly omnivorous (a balanced consumption of vegetables and meat), vegetarian, or carnivorous. Data on the duration and frequency of consuming vegetables, fruits, and dairy products (≥5 or 3–4 or ≤2 days/week) were collected. A healthy diet was defined as a balanced consumption of vegetables and meat, and the frequency of vegetable and fruit consumption were both ≥5 days per week (13,14).
The anthropometric information of each participant, including blood pressure, height, and body weight, was measured by trained personnel according to a standard protocol. Blood pressure was measured using an automated electric device (OMRON Model HEM-752 FUZZY; Omron Company, Dalian, China) on the nondominant arm. Two blood pressure measurements were taken at 1-minute intervals. The mean of the two values was defined as the measured blood pressure in the current analysis. BMI was calculated as weight in kilograms divided by squared height in meters.
Biochemical measurements
After at least 10 hours of fasting, blood samples of each participant were obtained for the measurements of fasting plasma glucose (FPG) and lipid profiles [triglyceride (TG); low-density lipoprotein cholesterol (LDL-c); HDL-c; total cholesterol (TC)], which were measured by an autoanalyzer (AU-5800 Chemistry System; Beckman, USA).
Definitions
Obesity and metabolic phenotypes
Obesity phenotypes were defined according to the Asia-Pacific BMI criteria, which were determined by the World Health Organization Western Pacific Region. Obesity was defined as a BMI ≥25 kg/m2, and non-obesity as a BMI <25 kg/m2, both of which had been verified recently among the Chinese population (15,16).
According to the Adult Treatment Panel III (ATP-III), the metabolic phenotypes were determined as follows: (I) elevated blood pressure: systolic blood pressure (SBP) ≥130 mmHg and/or diastolic blood pressure (DBP) ≥85 mmHg and/or taking antihypertensive drugs; (II) elevated blood glucose: FPG ≥5.6 mmol/L and/or taking antidiabetic drugs; (III) high TG: TG ≥1.7 mmol/L and/or taking lipid-lowering medication; (IV) low HDL-c: HDL-c <1.04 mmol/L in men and <1.29 mmol/L in women. Individuals with fewer than two of the four items were considered metabolically healthy (17,18).
Combining the obese and metabolic phenotypes, we defined (I) metabolically healthy non-obesity (MHNO) as a BMI <25 kg/m2 and a metabolically healthy status, (II) MHO as a BMI ≥25 kg/m2 and a metabolically healthy status, (III) metabolically unhealthy non-obesity (MUNO) as a BMI <25 kg/m2 and a metabolically unhealthy status, and (IV) metabolically unhealthy obesity (MUO) as a BMI ≥25 kg/m2 and a metabolically unhealthy status.
Recurrent stroke
Patients recruited in the present study with a definite history of previous stroke diagnosed by neurologists based on clinical symptoms, physical signs, and CT/MRI imaging were considered recurrent stroke patients.
Statistical analyses
The current analyses were conducted based on the combined obesity and metabolic phenotypes. The mean ± standard deviation (SD) and median (interquartile range) were used to describe the normally distributed and skewed continuous variables, whereas numbers (proportions) were used to describe the categorical variables. We performed a one-way analysis of variance (ANOVA) on the continuous variables and the Chi-square test on the categorical variables for the comparisons among the four phenotypes. Linear regression was used to analyze the trend across the four phenotypes.
Dependent on the multivariate logistic regression analyses, odds ratios (ORs) and 95% confidence intervals (CIs) were presented as the risk of recurrent stroke across the various BMI groups, ATP-III components, and metabolic phenotypes, as well as the four phenotypes in the combined obesity and metabolic groups.
We calculated the C statistics, net reclassification index (NRI), and integrated discrimination improvement (IDI) to evaluate the incremental prognostic values of individual BMI/metabolic status or the combined risk factors beyond conventional risk factors (19).
We used SAS software (version 9.3, Cary, NC, USA) to perform the statistical analyses and considered two-tailed P values <0.05 statistically significant.
Results
Characteristics of the study population according to obesity and metabolic phenotypes
The sociodemographic and biochemical characteristics and medical history of the study population are presented in Table 1. Of the 856 participants, 44.9% (n=384) were obese, and 77.7% (n=665) were metabolically unhealthy. The prevalence of different phenotypes was 14.5% for MHNO, 7.8% for MHO, 40.7% for MUNO, and 37.0% for MUO. Additionally, MHO accounted for 17.4% of obese participants. Obese participants were younger and had higher blood pressure and serum lipid levels than non-obese individuals, whether in the metabolically healthy or unhealthy groups. Among the obesity/metabolic phenotypes, individuals in the MHO, MUNO, and MUO groups displayed higher levels of TG, TC, LDL-c, higher proportions of hypertension, diabetes, hypercholesterolemia, and higher usage of antihypertensive and antidiabetic drugs than MHNO individuals (all P values for trend <0.05).
Table 1
Characteristics | Metabolically healthy | Metabolically unhealthy | P for trend | |||
---|---|---|---|---|---|---|
Non-obese (MHNO) (n=124) | Obese (MHO) (n=67) | Non-obese (MUNO) (n=348) | Obese (MUO) (n=317) | |||
Male, n (%) | 98 (79.0) | 48 (71.6) | 210 (60.3) | 222 (70.0) | 0.093 | |
Age (years) | 69.3±11.6 | 64.2±12.9 | 68.8±11.9 | 64.3±12.4 | 0.0007 | |
BMI (kg/m2) | 21.2±1.7 | 25.8±1.7 | 21.7±1.7 | 26.8±2.6 | <0.0001 | |
High school education or above, n (%) | 31 (25.0) | 24 (35.8) | 73 (21.0) | 96 (30.3) | 0.47 | |
Annual income ≥10,000 RMB, n (%) | 103 (83.1) | 64 (95.5) | 281 (80.8) | 260 (82.0) | 0.30 | |
Lifestyle factors, n (%) | ||||||
Current smokers | 65 (52.4) | 32 (47.8) | 131 (37.6) | 139 (43.9) | 0.10 | |
Current drinkers | 44 (35.5) | 23 (34.3) | 84 (24.1) | 99 (31.2) | 0.35 | |
High physical activity | 43 (34.7) | 24 (35.8) | 128 (36.8) | 102 (32.2) | 0.54 | |
Healthy diet | 20 (16.1) | 6 (9.0) | 56 (16.1) | 52 (16.4) | 0.60 | |
Metabolism-related measurements | ||||||
SBP (mmHg) | 149±24 | 147±22 | 151±19 | 152±18 | 0.093 | |
DBP (mmHg) | 83±13 | 86±11 | 83±12 | 87±13 | 0.023 | |
TG (mmol/L) | 1.07 (0.83–1.28) | 1.16 (0.90–1.46) | 1.56 (1.16–2.07) | 1.89 (1.36–2.66) | <0.0001 | |
TC (mmol/L) | 4.36±0.89 | 4.54±0.82 | 4.40±1.10 | 4.67±1.05 | 0.0061 | |
HDL-c (mmol/L) | 1.43±0.51 | 1.31±0.23 | 1.06±0.30 | 1.04±0.40 | <0.0001 | |
LDL-c (mmol/L) | 2.26±0.57 | 2.39±0.66 | 2.36±0.78 | 2.58±0.88 | <0.0001 | |
FPG (mmol/L) | 4.94 (4.52–5.43) | 4.90 (4.44–5.40) | 5.90 (4.92–7.59) | 6.10 (5.10–7.66) | <0.0001 | |
Diseases, n (%) | ||||||
Hypertension | 90 (72.6) | 49 (73.1) | 305 (87.6) | 287 (90.5) | <0.0001 | |
Diabetes | 1 (0.8) | 1 (1.5) | 153 (44.0) | 140 (44.2) | <0.0001 | |
Hypercholesterolemia | 1 (0.8) | 2 (3.0) | 13 (3.7) | 23 (7.3) | 0.0022 | |
Drug use, n (%) | ||||||
Antihypertensive drug use | 48 (38.7) | 31 (46.3) | 221 (63.5) | 210 (66.3) | <0.0001 | |
Antidiabetic drug use | 0 (0.0) | 0 (0.0) | 87 (25.0) | 77 (24.3) | <0.0001 | |
Lipid-lowering drug use | 1 (0.8) | 0 (0.0) | 8 (2.3) | 8 (2.5) | 0.15 |
Data are mean ± SD for continuous variables and number (percentage) for categorical variables. P values for trends across the four phenotypes were calculated from linear regression. MHNO, metabolically healthy non-obesity; MHO, metabolically healthy obesity; MUNO, metabolically unhealthy non-obesity; MUO, metabolically unhealthy obesity; BMI, body mass index; SBP, systolic blood pressure; DBP, diastolic blood pressure; TC, total cholesterol; TG, triglycerides; HDL-c, high-density lipoprotein cholesterol; LDL-c, low-density lipoprotein cholesterol; FPG, fasting plasma glucose; SD, standard deviation.
Obesity/metabolic phenotypes and risk of recurrent stroke
Table 2 shows the obesity/metabolic phenotypes and risk of recurrent stroke using multiple logistic regression. After dividing individuals into two groups based on the BMI, the risk of recurrent stroke increased by 50% (OR =1.50, 95% CI: 1.06–2.13) in obese compared with non-obese individuals after adjusting for age, sex, lifestyle, education, income, serum lipids, blood pressure, glucose, medication, and family history. However, a significantly increased risk of recurrent stroke was not found with an increase in BMI. Among the ATP-III components in the multivariate-adjusted model, elevated blood pressure and glucose, and low HDL-c but not high TG, were associated with an increased risk of recurrent stroke (OR =3.25, 95% CI: 1.33–7.93 for elevated blood pressure; OR =1.62, 95% CI: 1.14–2.28 for elevated blood glucose; and OR =1.46, 95% CI: 1.01–2.11 for low HDL-c). Regarding metabolic status, metabolically unhealthy individuals had a 72% (OR =1.72, 95% CI: 1.10–2.68) increased risk of recurrent stroke.
Table 2
Variables | Model 1, OR (95% CI) | Model 2, OR (95% CI) |
---|---|---|
Obesity† | ||
Non-obesity, BMI <25 kg/m2 | 1.00 (Ref) | 1.00 (Ref) |
Obesity, BMI ≥25 kg/m2 | 1.50 (1.07–2.08) | 1.50 (1.06–2.13) |
BMI, 1-SD increased (3.26 kg/m2) | 1.03 (0.88–1.21) | 1.09 (0.92–1.30) |
ATP-III component‡ | ||
BP ≥130/85 mmHg or on medication | 3.08 (1.31–7.23) | 3.25 (1.33–7.93) |
FPG ≥5.6 mmol/L or on medication | 1.77 (1.28–2.44) | 1.62 (1.14–2.28) |
TG ≥1.7 mmol/L or on medication | 0.83 (0.59–1.15) | 0.75 (0.52–1.10) |
HDL-c <1.04 mmol/L (<1.29 mmol/L in women) | 1.59 (1.13–2.23) | 1.46 (1.01–2.11) |
Metabolic status§ | ||
Metabolically healthy | 1.00 (Ref) | 1.00 (Ref) |
Metabolically unhealthy | 1.81 (1.18–2.80) | 1.72 (1.10–2.68) |
Model 1, adjusted for age and sex; Model 2, based on Model 1: †, further adjusted for current smoker (yes/no), current drinker (yes/no), physical activity, dietary habits, education, income, use of antihypertensive/antidiabetic/antilipemic drugs, and family history of hypertension/diabetes/hyperlipemia, TG, HDL-c, SBP, and FPG; ‡, further adjusted for current smoker (yes/no), current drinker (yes/no), physical activity, dietary habits, education, income, use of antihypertensive/antidiabetic/antilipemic drugs, and family history of hypertension/diabetes/hyperlipemia, BMI, TG, HDL-c, SBP, FPG (except for the analyzed ATP-III component); §, further adjusted for current smoker (yes/no), current drinker (yes/no), physical activity, dietary habits, education, income, family history of hypertension/diabetes/hyperlipemia, and BMI. ATP-III, Adult Treatment Panel III; BMI, body mass index; BP, blood pressure; CI, confidence interval; FPG, fasting plasma glucose; HDL-c, high-density lipoprotein cholesterol; OR, odds ratio; SBP, systolic blood pressure; SD, standard deviation; TG, triglycerides.
Combining the obesity and metabolic phenotypes, we reclassified the participants into four groups: MHNO, MHO, MUNO, and MUO. The prevalence of recurrent stroke was 16.1% (20/124) in MHNO, 13.4% (9/67) in MHO, 21.8% (76/348) in MUNO, and 27.4% (87/317) in MUO, and 22.4% in total participants. The multivariate-adjusted logistic regression (Figure 1) did not find a significant association between MHO individuals and recurrent stroke compared to their non-obese counterparts. Likewise, individuals with MUNO did not have a higher risk of recurrent stroke than those with MHNO. However, when combining the obesity and metabolic status, a 2.24-fold increased risk of recurrent stroke was found in MUO compared to MHNO individuals.
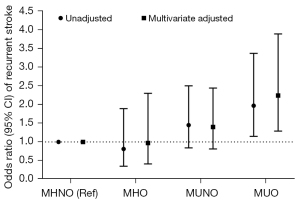
Incremental prognostic values of BMI/metabolic status
Table 3 shows whether individual BMI or metabolic status could add the prognostic value of recurrent stroke to conventional risk factors. The C statistic (95% CI) of the predictive models of conventional risk factors was 0.607 (0.574–0.640), and the addition of BMI or metabolic status did not increase the C statistic. Nevertheless, we found metabolic status, but not BMI, could significantly improve the reclassification risk of recurrent stroke when combined with conventional risk factors. Adding the combined BMI and metabolic status to conventional risk factors resulted in greater incremental prognostic values (all P values <0.05).
Table 3
Variables | C statistic | NRI (continuous) | IDI | |||||
---|---|---|---|---|---|---|---|---|
Estimate (95% CI) | P value‡ | Estimate (95% CI) | P value‡ | Estimate (95% CI) | P value‡ | |||
Conventional risk factors† | 0.607 (0.574–0.640) | – | Reference | – | Reference | – | ||
Adding BMI/metabolic status to conventional risk factors | ||||||||
Adding BMI | 0.608 (0.574–0.641) | 0.89 | 0.139 (−0.021 to 0.299) | 0.089 | 0.0029 (−0.0005 to 0.0064) | 0.095 | ||
Adding metabolic status | 0.624 (0.590–0.656) | 0.19 | 0.176 (0.054–0.297) | 0.0047 | 0.007 (0.0014–0.0127) | 0.014 | ||
Adding BMI and metabolic status | 0.624 (0.591–0.657) | 0.17 | 0.195 (0.058–0.332) | 0.0052 | 0.009 (0.0025–0.0148) | 0.0059 |
†, conventional risk factors, including age, sex, current smoker (yes/no), current drinker (yes/no), physical activity, dietary habits, education, and income; ‡, P values were compared to the conventional risk factors. BMI, body mass index; NRI, net reclassification index; IDI, integrated discrimination improvement; CI, confidence interval.
Discussion
In the present study, we found that among Chinese hospitalized stroke patients, metabolically unhealthy status and ATP-III components (elevated blood pressure and glucose and low HDL-c) significantly increased the risk of recurrent stroke, regardless of BMI. In contrast, no association existed between BMI and risk of recurrent stroke. Furthermore, we found that metabolic status, not BMI, significantly improved the prognostic value for recurrent stroke when combined with conventional risk factors.
Numerous previous studies have demonstrated that MetS is associated with an increased risk of recurrent stroke (11,20,21). The ACROSS-China study showed a hazard ratio of 1.94 (1.39–2.73) in patients with MetS versus no MetS (21), and another longitudinal study calculated a higher risk of recurrent stroke, with a hazard ratio of 1.43 (1.01–2.01) (11). Further controlling for the components of MetS, the significant associations disappeared. Consistent with previous studies, our study found that metabolically unhealthy individuals had a 72% higher risk of recurrent stroke than metabolically healthy ones, and individual metabolic risk factors, such as elevated blood pressure, fasting glucose, and low HDL-c levels, were also associated with an increased risk of recurrent stroke. These results underline the crucial value of metabolic status on stroke prognosis.
Although obesity is a considerable risk factor for stroke (whether defined on waist circumference or BMI), certain studies interestingly reported that obesity might be a “protective” factor for stroke recurrence (4,22,23). The obesity paradox is partly attributed to selection bias (obese patients are younger, more likely to be female, and smoke less) and the limitation of the obesity definition (23). Inconsistent with these previous findings, our study found that obese patients (defined as BMI ≥25 kg/m2) had a marginally higher risk of recurrent stroke when metabolic confounding factors were controlled. However, BMI as a continuous variable was not found to be associated with recurrent stroke. The discrepancies might be attributed to further adjustments with metabolic-related confounders and the indiscriminate gender proportion (P=0.42, data not shown) and lifestyle (P=0.60 for current smoker and P=0.07 for current drinker, data not shown) between obese and non-obese individuals.
The current study is the first to investigate and compare the effects of metabolic status and BMI on recurrent stroke. To confirm the effect of metabolic status and BMI, we stratified the population based on metabolic phenotypes and different BMI groups (BMI ≥25 kg/m2 and BMI <25 kg/m2) into four groups: MHNO, MHO, MUNO, and MUO. Previous studies investigating obesity/metabolic phenotypes and stroke have focused on the primary prevention field and have drawn inconsistent conclusions (24-27). For example, a nationwide population-based study in Korea reported that MHO individuals were not at an increased risk of stroke (24). In contrast, another longitudinal study of 7,122 participants found that MHO individuals were at an increased risk of cardiovascular disease, including stroke, and stroke separately (25). Studies investigating the effect of obesity/metabolic phenotypes on stroke prognosis are sparse. The present study showed that MUO individuals had a statistically higher risk of recurrent stroke than MHNO individuals, and the OR of MUNO individuals was higher than that of MHO individuals (although the OR P values did not reach statistical significance). A metabolically unhealthy status and its components were significantly associated with a higher risk of recurrent stroke. However, no association was detected between BMI and recurrent stroke. Furthermore, as evidenced by NRI and IDI, adding metabolic status to conventional risk factors substantially improved the risk stratification for recurrent stroke, whereas adding BMI did not. These results suggest that metabolic abnormalities, not BMI, increase the risk of recurrent stroke.
Several mechanisms could be responsible for metabolic status being more important than BMI in the risk of recurrent stroke. First, chronic inflammation has been postulated to initiate recurrent stroke in metabolically unhealthy stroke patients, regardless of BMI. It is well known that inflammation plays a critical role in the development of atherosclerosis, plaque instability, and the triggering of plaque rupture in the pathogenesis of cerebrovascular diseases (28). Several population-based cohort studies have similarly demonstrated that the most extensively used blood marker of inflammation, C-reactive protein (CRP), significantly contributed to recurrent stroke (29-31). Recently, other studies have reported that metabolically unhealthy obese and non-obese individuals had an unfavorable inflammation status compared to their metabolically healthy counterparts (32-34), with higher levels of CRP, interleukin 6 (IL-6), tumor necrosis factor α (TNF-α), and adipocyte fatty acid binding protein (A-FABP). Thus, it is likely that the adverse inflammation status of metabolically unhealthy individuals mediated the risk of recurrent stroke. In addition, visceral adipose tissue may play a substantial role. Inflammation of visceral adipose tissue has been found to mediate a metabolically unhealthy status regardless of generalized obesity (35). Camhi et al. also found that metabolically unhealthy individuals, whether obese or non-obese, had higher amounts of visceral adiposity and liver fat than metabolically healthy participants (36). Therefore, there is sufficient evidence to suggest that visceral adiposity is a risk factor for recurrent stroke (7,37). A retrospective study in Japan found that recurrent ischemic stroke patients had significantly higher visceral fat levels than those without recurrence, and visceral fat level was associated with a higher risk of recurrent stroke (OR =2.44, 95% CI: 1.08–5.49) (37).
The present study had some limitations that require mention. First, medications and treatment that may have affected the results were not fully adjusted for in our analysis. However, our multivariate logistic regression did adjust for antihypertensive/antidiabetic drugs and lipid-lowering drugs, which may have a substantial influence on the development of atherosclerosis and plaque instability. Additionally, the treatment for stroke was a critical confounding factor and may affect the results. More information on the treatment for stroke would be collected in follow-up visits. Second, as this was a cross-sectional study, data concerning the incident stroke were unavailable. We calculated ORs instead of hazard ratios for evaluating the risk of recurrent stroke. Accordingly, the seriousness of the incident stroke, which significantly affects prognosis, was unknown. Additionally, in the cross-sectional study, the mechanisms underlying the significant relationship between metabolic abnormalities and stroke could not be thoroughly elaborated, i.e., there is a possibility that elevated BMI results in metabolic abnormalities, which further results in stroke. Therefore, a prospective study is warranted to further define the associations between metabolic phenotypes and BMI in recurrent stroke. Third, our study was conducted with Chinese hospital patients, so caution is required in generalizing our findings to other ethnicities.
Conclusions
In conclusion, the present study found a significant association between increased recurrent stroke risk and metabolic abnormalities, whereas an association between BMI and recurrent stroke was not detected. In addition, when combined with conventional risk factors, metabolic status statistically improved the prognostic value for recurrent stroke, whereas BMI did not. Therefore, we suggest that recurrent stroke is, to a large extent, related to poor metabolic status rather than BMI. Controlling metabolic abnormalities could be a more efficacious method to prevent recurrent stroke than controlling weight in stroke patients.
Acknowledgments
We thank all the participants for their participation and clinical colleagues for their valuable assistance.
Funding: This study was supported by the National Natural Science Foundation of China (grant No. 81900768), the Young Talent Development Plan of Changzhou Health Commission (grant No. CZQM2020007), and the Applied and Basic Research Program of Changzhou Scientific and Technological Project (grant No. CJ20190103).
Footnote
Reporting Checklist: The authors have completed the STROBE reporting checklist. Available at https://atm.amegroups.com/article/view/10.21037/atm-22-4606/rc
Data Sharing Statement: Available at https://atm.amegroups.com/article/view/10.21037/atm-22-4606/dss
Conflicts of Interest: All authors have completed the ICMJE uniform disclosure form (available at https://atm.amegroups.com/article/view/10.21037/atm-22-4606/coif). The authors have no conflicts of interest to declare.
Ethical Statement: The authors are accountable for all aspects of the work in ensuring that questions related to the accuracy or integrity of any part of the work are appropriately investigated and resolved. This study was conducted in accordance with the Declaration of Helsinki (as revised in 2013). The study was approved by the Ethics Committee of the First People’s Hospital of Changzhou, Third Affiliated Hospital of Soochow University (No. 2020025) and informed consent was taken from all the patients.
Open Access Statement: This is an Open Access article distributed in accordance with the Creative Commons Attribution-NonCommercial-NoDerivs 4.0 International License (CC BY-NC-ND 4.0), which permits the non-commercial replication and distribution of the article with the strict proviso that no changes or edits are made and the original work is properly cited (including links to both the formal publication through the relevant DOI and the license). See: https://creativecommons.org/licenses/by-nc-nd/4.0/.
References
- Poirier P, Giles TD, Bray GA, et al. Obesity and cardiovascular disease: pathophysiology, evaluation, and effect of weight loss: an update of the 1997 American Heart Association Scientific Statement on Obesity and Heart Disease from the Obesity Committee of the Council on Nutrition, Physical Activity, and Metabolism. Circulation 2006;113:898-918. [Crossref] [PubMed]
- Strazzullo P, D'Elia L, Cairella G, et al. Excess body weight and incidence of stroke: meta-analysis of prospective studies with 2 million participants. Stroke 2010;41:e418-26. [Crossref] [PubMed]
- Kurth T, Gaziano JM, Berger K, et al. Body mass index and the risk of stroke in men. Arch Intern Med 2002;162:2557-62. [Crossref] [PubMed]
- Doehner W, Schenkel J, Anker SD, et al. Overweight and obesity are associated with improved survival, functional outcome, and stroke recurrence after acute stroke or transient ischaemic attack: observations from the TEMPiS trial. Eur Heart J 2013;34:268-77. [Crossref] [PubMed]
- Aparicio HJ, Himali JJ, Beiser AS, et al. Overweight, Obesity, and Survival After Stroke in the Framingham Heart Study. J Am Heart Assoc 2017;6:004721. [Crossref] [PubMed]
- Oesch L, Tatlisumak T, Arnold M, et al. Obesity paradox in stroke - Myth or reality? A systematic review. PLoS One 2017;12:e0171334. [Crossref] [PubMed]
- Chen W, Pan Y, Jing J, et al. Recurrent Stroke in Minor Ischemic Stroke or Transient Ischemic Attack With Metabolic Syndrome and/or Diabetes Mellitus. J Am Heart Assoc 2017;6:e005446. [Crossref] [PubMed]
- Byun AR, Kwon S, Lee SW, et al. Metabolic health is more closely associated with prevalence of cardiovascular diseases or stroke than obesity: A cross-sectional study in Korean populations. Medicine (Baltimore) 2016;95:e3902. [Crossref] [PubMed]
- Li X, Li X, Fang F, et al. Is Metabolic Syndrome Associated with the Risk of Recurrent Stroke: A Meta-Analysis of Cohort Studies. J Stroke Cerebrovasc Dis 2017;26:2700-5. [Crossref] [PubMed]
- Li Z, Guo X, Liu Y, et al. Metabolism rather than obesity is associated with ischemic stroke: a cross-sectional study in rural Northeastern China. Springerplus 2016;5:1419. [Crossref] [PubMed]
- Fang X, Liu H, Zhang X, et al. Metabolic Syndrome, Its Components, and Diabetes on 5-Year Risk of Recurrent Stroke among Mild-to-Moderate Ischemic Stroke Survivors: A Multiclinic Registry Study. J Stroke Cerebrovasc Dis 2016;25:626-34. [Crossref] [PubMed]
- Seo YG, Choi HC, Cho B. The Relationship between Metabolically Obese Non-Obese Weight and Stroke: The Korea National Health and Nutrition Examination Survey. PLoS One 2016;11:e0160846. [Crossref] [PubMed]
- Wang C, Huang XL, Xiang SK, et al. The effect of an overall healthy lifestyle on early-onset stroke: a cross-sectional study. Ann Palliat Med 2020;9:2623-30. [Crossref] [PubMed]
- Lv J, Yu C, Guo Y, et al. Adherence to Healthy Lifestyle and Cardiovascular Diseases in the Chinese Population. J Am Coll Cardiol 2017;69:1116-25. [Crossref] [PubMed]
- Lin L, Zhang J, Jiang L, et al. Transition of metabolic phenotypes and risk of subclinical atherosclerosis according to BMI: a prospective study. Diabetologia 2020;63:1312-23. [Crossref] [PubMed]
- Lin L, Peng K, Du R, et al. Metabolically healthy obesity and incident chronic kidney disease: The role of systemic inflammation in a prospective study. Obesity (Silver Spring) 2017;25:634-41. [Crossref] [PubMed]
- Hinnouho GM, Czernichow S, Dugravot A, et al. Metabolically healthy obesity and risk of mortality: does the definition of metabolic health matter? Diabetes Care 2013;36:2294-300. [Crossref] [PubMed]
- Expert Panel on Detection, Evaluation, and Treatment of High Blood Cholesterol in Adults. Executive Summary of The Third Report of The National Cholesterol Education Program (NCEP) Expert Panel on Detection, Evaluation, And Treatment of High Blood Cholesterol In Adults (Adult Treatment Panel III). JAMA 2001;285:2486-97. [Crossref] [PubMed]
- Pencina MJ, D'Agostino RB, Pencina KM, et al. Interpreting incremental value of markers added to risk prediction models. Am J Epidemiol 2012;176:473-81. [Crossref] [PubMed]
- Liu L, Zhan L, Wang Y, et al. Metabolic syndrome and the short-term prognosis of acute ischemic stroke: a hospital-based retrospective study. Lipids Health Dis 2015;14:76. [Crossref] [PubMed]
- Mi D, Jia Q, Zheng H, et al. Metabolic syndrome and stroke recurrence in Chinese ischemic stroke patients--the ACROSS-China study. PLoS One 2012;7:e51406. [Crossref] [PubMed]
- Zhao L, Du W, Zhao X, et al. Favorable functional recovery in overweight ischemic stroke survivors: findings from the China National Stroke Registry. J Stroke Cerebrovasc Dis 2014;23:e201-6. [Crossref] [PubMed]
- Ovbiagele B, Bath PM, Cotton D, et al. Obesity and recurrent vascular risk after a recent ischemic stroke. Stroke 2011;42:3397-402. [Crossref] [PubMed]
- Lee HJ, Choi EK, Lee SH, et al. Risk of ischemic stroke in metabolically healthy obesity: A nationwide population-based study. PLoS One 2018;13:e0195210. [Crossref] [PubMed]
- Hinnouho GM, Czernichow S, Dugravot A, et al. Metabolically healthy obesity and the risk of cardiovascular disease and type 2 diabetes: the Whitehall II cohort study. Eur Heart J 2015;36:551-9. [Crossref] [PubMed]
- Aung K, Lorenzo C, Hinojosa MA, et al. Risk of developing diabetes and cardiovascular disease in metabolically unhealthy normal-weight and metabolically healthy obese individuals. J Clin Endocrinol Metab 2014;99:462-8. [Crossref] [PubMed]
- Sánchez-Iñigo L, Navarro-González D, Fernández-Montero A, et al. Risk of incident ischemic stroke according to the metabolic health and obesity states in the Vascular-Metabolic CUN cohort. Int J Stroke 2017;12:187-91. [Crossref] [PubMed]
- Di Napoli M, Elkind MS, Godoy DA, et al. Role of C-reactive protein in cerebrovascular disease: a critical review. Expert Rev Cardiovasc Ther 2011;9:1565-84. [Crossref] [PubMed]
- Kitagawa K, Hosomi N, Nagai Y, et al. Cumulative Effects of LDL Cholesterol and CRP Levels on Recurrent Stroke and TIA. J Atheroscler Thromb 2019;26:432-41. [Crossref] [PubMed]
- Matsuo R, Ago T, Hata J, et al. Plasma C-Reactive Protein and Clinical Outcomes after Acute Ischemic Stroke: A Prospective Observational Study. PLoS One 2016;11:e0156790. [Crossref] [PubMed]
- Liu H, Qian S, Zhong C, et al. Predictive Value of Cystatin C for Stroke Recurrence in Patients With Acute Ischemic Stroke. Circ J 2021;85:213-9. [Crossref] [PubMed]
- Graf C, Ferrari N. Metabolic Health-The Role of Adipo-Myokines. Int J Mol Sci 2019;20:6159. [Crossref] [PubMed]
- Lee TH, Jeon WS, Han KJ, et al. Comparison of Serum Adipocytokine Levels according to Metabolic Health and Obesity Status. Endocrinol Metab (Seoul) 2015;30:185-94. [Crossref] [PubMed]
- Phillips CM, Perry IJ. Does inflammation determine metabolic health status in obese and nonobese adults? J Clin Endocrinol Metab 2013;98:E1610-9. [Crossref] [PubMed]
- Blüher M. The distinction of metabolically 'healthy' from 'unhealthy' obese individuals. Curr Opin Lipidol 2010;21:38-43. [Crossref] [PubMed]
- Camhi SM, Katzmarzyk PT. Differences in body composition between metabolically healthy obese and metabolically abnormal obese adults. Int J Obes (Lond) 2014;38:1142-5. [Crossref] [PubMed]
- Ushio M, Kanaoka M, Kinoshita Y, et al. Moderate-to-vigorous physical activity and the risk of stroke recurrence in patients with a history of minor ischemic stroke in Japan: a retrospective analysis. Top Stroke Rehabil 2018;25:591-8. [Crossref] [PubMed]
(English Language Editor: C. Fitzgerald)