Abstract
Road networks are some of the oldest and most permanent man-made structures in space, serving as valuable records of the conditions of the society through long periods of time. Quantitatively analyzing these networks will therefore reveal rich insights into the socio-political conditions of the society through history, and can provide awareness for effectively managing the growth and evolution in the future. Here, we extracted the state of the road network of Manila, Philippines at various points in history through georeferencing and digitization of hand-drawn historical maps. Visual and metrical analyses revealed key well-planned periods punctuating the otherwise self-organized growth, particularly the more recent densification at reclamation areas coincident with the rapid economic growth. The road network of Manila shows statistical regularities that are also observed for other global road network data sets, although the recent reclamation significantly increase the statistics of the very short and peripheral nodes. Finally, the clusters of nodes with the highest closeness centralities mimic the historical shape of the network, allowing for an automatic identification of the core historical sections of the city. Studies such as this one extract useful information from these permanent spatial records, which may then be useful for developing sound policy measures for handling further urbanization.
Export citation and abstract BibTeX RIS

Content from this work may be used under the terms of the Creative Commons Attribution 4.0 licence. Any further distribution of this work must maintain attribution to the author(s) and the title of the work, journal citation and DOI.
1. Introduction
Cities, as centers of socio-economic activity [1], have hosted more than half of the human population since the last decade, with the fraction expected to continue growing over the next few years [2]. The accelerated growth over these pockets of limited spaces brings about a host of problems and challenges both in the environment [3–5] and in the well-being of the population living in them [6–9]. Not surprisingly, the city and its interrelated components have been studied across various lenses of the natural, social, and engineering sciences [10–13]. Many such works benefit from the availability of huge, high-resolution data sets and faster, more intelligent computational techniques [9, 14, 15]. These studies give us a better picture of urbanization, which, in turn, help us develop effective policy measures for administering cities across various regions and socio-economic conditions.
One useful track that was made possible by the availability of data and computational capability to handle them is a quantitative historical analysis, wherein the current state of the city may be understood by studying the spatial patterns left by urbanization [16]. And among these spatial features, the road networks are the ones that outlast the other physical elements [17, 18], and, sometimes, even the very civilizations that built them [19]. Therefore, apart from studies that characterize the spatial [20–22] and topological properties [23–26] of the contemporary road network, a wealth of information about the state of the city and the forces of urbanization can be gained from studying the historical development of road networks.
In studying the history of road networks, two important and complementary aspects can be highlighted. On one hand, some studies describe the road network evolution, i.e. the changes that the network has undergone through the years. This aspect is the more straightforward of the two, and usually involves the physical layout of the network in space. For example, we now view road network evolution as an interplay between long-range exploration and densification [27], which result in a fractal growth in space [28, 29]. We can also associate the observed physical changes, especially the more drastic ones, with important events in the history of the city [30]. On the other hand, other studies also report the invariant properties, i.e. what are being preserved in the network architecture. For example, it is interesting to note that road networks show a remarkable statistical consistency in spite of the visual differences in their physical layouts in space. Strano et al [31] studied the road length distributions of the entire global road network, and realized that the distributions collapse into a universal curve upon rescaling with the characteristic road lengths. Klinkhamer et al [26] also observed a universal statistical behavior in the degree distributions of road networks under dual representation which is best represented as a power-law (Pareto) distribution with scaling exponent of −2.49. Masucci et al [32] observed a collapse of the cluster of road network elements vs spatial extent into a universal logistic growth, signifying a characteristic scale that leads to the giant connected cluster. Kirkley et al [33] even reported the invariance of the betweenness centrality distributions of road intersections, which is deemed to be a universal property of planar graphs.
In this work, we are guided by a simple research question: are there preserved properties of the road network that are embedded in its spatial architecture? In other words, is it possible to automatically identify an earlier physical state of the network given the contemporary map? These questions are inspired by the intuition that some sections of the road network, especially of cities that have a self-organized growth, must have been so important that their structure is simply embedded in the current physical state of the network. Identifying these regions, which are deemed to be critical sections of the network, have important implications, such as in directing vehicular traffic, which is expected to grow along with the continued urbanization.
To test this hypothesis, we apply the quantitative tools developed in complex systems and network studies to understand the development of roads in a region presented with geographical constraints. In particular, we describe the evolution of the road network of Manila, the capital city of the Philippines. Manila is an interesting case for study, as it is one of the oldest colonial cities in Southeast Asia whose development has been punctuated by periods of different Western influences under the Spanish (1571–1898) and American (1898–1946) colonial rule. The city had also gone from a complete destruction of war in 1945 to a recent rapid economic growth that is one of the fastest in the region over the last decade [34, 35]. This spurred the inward migration of people, which, in turn, resulted in an urban zone with one of the highest populations, population densities, and population growth rates in the world [36], and a road network that is the most congested in developing Asia [37]. Unlike other cities in the region, however, Manila and its conurbation is in a constrained geographical location in an isthmus [22], which raises the question on whether this development is sustainable given the limited physical space.
Through georeferencing and digitization of hand-drawn maps from various periods of its historical development, we translate analog map images into useful geographical information system (GIS) digital data sets with substantial accuracy. With this, the temporal state of the network can be studied and associated with the prevailing conditions at the time of the map creation. The road networks obtained for the different periods are then analyzed using simple geographical metrics and the tools of complex network theory, particularly the use of centrality values, which are the most common measures of node vitality in a graph [33, 38, 39].
In our results, we first quantified (i) what changed in the physical road networks and gave a picture of the degree of exploration and densification at various periods in history. For the case of Manila, we observe the effect of exploration in the early history of the city towards the end of the 19th century. More than a century later, however, we observe the sharp rates of densification, which we attribute to the finer and finer segmentation of the limited available space. We also gave an insight into the different types of development under the different periods of political influences. We also reported (ii) statistical regularities, particularly in the distributions of road lengths and betweenness and closeness centrality values. Finally, we observe (iii) spatial invariants; specifically, that the nodes with the highest closeness centrality values recover the oldest sections of the city. Through a comparison with traffic data from actual car counts and model projections from other works, we comment on the sustainability of these critical regions in the map, and offer possible directions for alleviating the traffic conditions.
2. Data
2.1. Historical and contemporary maps
Historical maps predate the digital maps obtained from satellite images, and are thus useful for extending the temporal scope of the study. The quality of the maps gives a glimpse as to the degree of scientific and artistic competency of the population during the period they are created [40]. Needless to say, hand-drawn historical maps, while correctly depicting the structure of the road network, lack the geographical accuracy required for quantitative analyses. As such, a historical map should first be subjected to proper georeferencing before digitization and data extraction.
In this work, we use historical maps of the city of Manila at various points in its historical development. The years and the data sources are presented in table 1. To provide a good representation of the different periods of development, we selected maps from the Spanish colonial period (1875 and 1894), the American colonial period (1908 and 1945), and the postwar republic (1991, and the contemporary 2019 map).
Table 1. Historical maps and their sources.
Map | Source |
---|---|
1875 | Filipinas Heritage Library |
1894 | National Archives of the Philippines |
1908 | Filipinas Heritage Library |
1945 | University of Wisconsin–Milwaukee |
1991 | University of the Philippines Main Library |
The raw historical map data are obtained by digitally scanning an actual physical copy of the map using the Niji scanner with a resolution of 600 dpi. Meanwhile, the contemporary map is extracted from OpenStreetMap (OSM) [41] imported through QGIS. The OSM data is accurate as of January 2019. For our purpose, this contemporary road network will serve as the baseline reference to evaluate the networks extracted from the maps.
2.2. Map georeferencing and road digitization
The historical maps, now being handled as digital images, are georeferenced with respect to the contemporary GIS map from OSM using the OpenLayer plug-in in QGIS. First, we create a convex hull outlining the borders of the city to limit the extent of the geographical matching. Afterwards, we establish ground control points (GCP) that are deemed to be corresponding locations in the historical and the OSM map. Whenever possible, road network features that are present in all historical maps are included in the GCP to ensure the consistency and accuracy. Using the WGS 84 Pseudo Mercator as the reference coordinate system, we select either a thin plate spline or polynomial 3 transformation and nearest-neighbour resampling method for each historical map to warp the planar image onto real space. The resulting digital image in geotiff format has every point corresponding to a real geographical location denoted by the longitude ϕ and latitude θ coordinate. An illustration of the process is presented in figure 1.
Figure 1. Schematic illustration of the digitized road network shapefile extraction. Corresponding GCP from the historical and contemporary maps are matched by the algorithm, after which the road network coordinates are extracted.
Download figure:
Standard image High-resolution imageUsing the georeferenced historical maps, shapefiles of the Manila road network are drawn in QGIS. The roads are manually traced by following the centerlines of the hand-drawn roads from the map. While a combination of several techniques from image processing and machine learning can be used to make this process automated, we opted to manually trace the roads in QGIS for two reasons: (1) to be able to follow the tracks of roads by their names; and (2) to ensure substantial accuracy, especially in regions where the roads are no longer extant. Needless to say, the process also has drawbacks, chief of which is the fact that the egregious systematic errors of the cartographer can never be corrected.
The road network shapefiles are then extracted for the nodes in QGIS. Majority of the nodes mark intersections of two or more distinct roads, or bends for winding roads. Additionally, some nodes can be found along a stroke of road. This happens when long isolated portions of the road have been segmented to denote important points, such as locations of national monuments or local markers, or in places where the road widens or constricts. There are also cases when a stroke of road consists of two distinctly named streets; in such a case, the node marks the boundary between the two.
3. Parameters
3.1. Measures of road network evolution
The road network formation is a complex self-organizing process [27, 30], one that forms fractals [10, 29, 42] and other morpohological patterns [20–22] in space. In quantifying the evolution of the road network, one can view it as a collection of individual roads r, as named by the city. In this case, each road can be measured based on its geographical length Lr. The entire network for each map representing the different periods can be characterized by: (i) the total road length Lsum = ∑rLr; (ii) the average road length ⟨L⟩ = L/N, where N is the number of roads obtained in the map over that period; (iii) and the road length area density, ρL = Lsum/A, where A is the area of the convex hull bounded by the existing roads over the given map period.
Because of its structure, the road networks can also be understood using the techniques developed in complex network theory [43]. Mathematically, graphs are constructs made up of nodes whose interactions are modeled by a connection or edge drawn between them. For this work, we create the primal representation of the road network wherein an individual road is a path in the network, the geographical intersections or turning points are the nodes i, and the physical portions of the road between intersections i and j are the edges eij. With this, we can think of the network at any given map period as a collection of nodes and edges, and we can track: (i) the number of nodes ni and edges ne; and (ii) the node and edge densities over A, pi = ni/A and Pe = ne/A, respectively.
3.2. Centrality measures
Apart from viewing it as a simple collection of elements, we represent the road network as a weighted graph wherein each edge has a weight value wij corresponding to the geographical length of eij. This introduces real-world traffic implications and node-to-node relationships. In traversing the network, the path of minimum distance required to reach j from i is called the shortest path length lij, which is reported in terms of the number of node-to-node jumps.
Additionally, representing the road network using graphs allows us to utilize the tools of complex network theory for characterizing the state of the network over time. Traditionally, the importance of each node in the network [39] are quantified via different measures of centrality, with each having different interpretations based on the specific aspects of the network connectivity being measured. For our purpose, we track the closeness and betweenness centralities, two measures that are related to the physical layout of the nodes in space. The closeness centrality ψ is given by
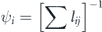
for all j ≠ i; this metric gives the structural center of the network, as the node with highest closeness centrality has the minimum shortest paths, , to all the other nodes. On the other hand, the betweenness centrality is given by

for all h ≠ j ≠ i. It measures how many times the node i has appeared, σhj(i), in the collection of all shortest paths in the network, σhj. The high-δ nodes, therefore, may be thought of as bridges where efficient connections between peripheral nodes are passing through. In the subsequent analyses, the structural differences between the historical and contemporary maps can therefore be quantified using these metrics.
4. Results
4.1. Geographical road network evolution
Figure 2 shows the digitized maps from various years (colored roads) laid over each other and on top of the contemporary road network structure in the region from OSM (gray roads). A closer inspection of the maps reveal road patterns that are not directly coinciding. In very limited cases, we find instances of road translations by 75 m from contemporary locations. As mentioned previously, the georeferencing procedure is subject to possible errors due to manual cartography, resulting in minor deviations in road network patterns. Additionally, the postwar reconstruction resulted in actual geographical changes in some roads, which explains some differences between pre-war and post-war layouts. We find that these changes have minimal effect on the characterization, especially in computing for the topological properties.
Figure 2. The road network within the Manila political boundary (colored), overlaying the contemporary road network data from OSM. The immediately successive maps are isolated in the bottom, where the colored regions denote the regions of growth.
Download figure:
Standard image High-resolution imageDue to the geographical constraint (the Manila Bay to the left), the spatial growth had generally extended eastward across the observation period. Interestingly, however, we find the development of new features that coincide with key events in the history of the city. At the bottom panels of figure 2, we present the successive maps, with the older map in black and the later map retaining its color, to be able to observe the changes that transpired. The 1875 map clearly shows the road network plan for Manila developed by the Spanish since the late 16th century: the core city, near the opening of the river to the bay, is grid-like and walled. This is called the Intramuros ('within the walls') district, and is still extant and preserved.
The period between 1875 and 1894 correspond to the waning periods of Spanish colonization of the Philippines, before the Philippine revolution of 1896. As such, we find very minimal growth between this period, with just a few pockets of grid-like developments sprouting in 1894 from an already stable road network layout. The period between 1894 and 1908, in contrast, can be characterized to be very dynamic, as reflected in the changes in the physical network layout. Interestingly, this is associated with the corresponding socio-political conditions at the time: Spain ceded control of the Philippines to the United States in 1898, thereby beginning the American colonization and, with it, massive construction in the capital. In 1904, in an attempt to resolve the status of the friars, the United States bought the major portions of the friar's holdings of 1,660 km2, half of which was in the vicinity of Manila [44]. This largely increased the territorial coverage of the city available for infrastructure growth. These major efforts in building new and longer roads expanded the road network span but lessened the density of roads built. Merely ten years after the Americans took over, the road network structure grew significantly. In the figure, we find the development of what would now be the South Port of Manila to the west of Intramuros, and other grid-like developments southward in the 1908 map. Incidentally, this map also showed exploratory mechanisms towards the east, as part of the efforts to connect the capital to the nearby provinces.
Before the end of World War II in 1945, we observe a heavily homogenized space in the Manila map. The grid-like portions in the northeast coincided with the construction of the planned Quezon City that started in 1939, which was designed to be the new capital. The boundary between these two cities, containing residential communities of 'New Manila', are heavily densified to recover uniformly sized blocks, inspired by the utilitarian network layouts typical of other American urban areas such as Manhattan in New York and Chicago [45]. Between the 1945 and 1991 maps, not much changed in the network layout, save for the road network development at the North Port of Manila to the northwest of Intramuros. However, between the 1991 and 2019 maps, we find major road network growth in the northwestern part of the city due to reclamation efforts [46]. Incidentally, the development of these regions correspond to the period of rapid economic development of the Philippines, which resulted in the rise of infrastructure spending by the government and private entities. These areas now host the bigger port operations and large business and mixed-used establishments.
4.2. Measures of road growth
Apart from the qualitative description provided by the spatial mapping, the metrical and topological properties for all the years are plotted in figure 3. The top panels denote the raw values of the metrical properties (a) N and (b) Lsum, and the topological properties (c) ni and (d) ne. As expected, the road network grows in spatial extent and topological (network) complexity in time. Comparing the spatial and network growth rates of Manila with other cities in literature such as Groane, Italy [27] and Paris, France [30] reveals a faster growth rate for Manila over similar periods, most likely due to the constrained geographical limits. In particular, the heavy spikes observed between 1991 and the contemporary data for the number of roads (figure 3(a)), number of nodes (figure 3(c)), and number of edges (figure 3(d)) signify the extent and rates of the reclamation and the road-building during this relatively short period. However, the total length of roads (figure 3(b)) is expected to show a tapering off, as the space cannot be entirely converted into roads. These results suggest the type of development over the last few decades: new city areas that are added by reclamation are developed to have multiple short roads that divide the space into regular blocks. These punctuations of highly-planned development over the predominantly self-organized growth are also easily captured by the visual map comparisons from figure 2.
Figure 3. The evolution of the (a) N, (b) Lsum, (c) ni, and (d) ne show the rapid growth over the last few decades. When divided by the effective area of the road network extent, both the (e) road count density N/A and (f) ρL show dips at 1908 signifying the dominance of exploration. The (g) ρi and (h) ρe, in contrast, follow mostly the trends of the actual counts in (c) and (d) respectively, signifying that the road network complexity is independent of the actual area covered.
Download figure:
Standard image High-resolution imageTo further investigate the growth, we obtain the effective spatial area A spanned by the roads within the convex hull of the Manila map for the particular period in consideration. The bottom panels of figure 3 are measures of spatial density (i.e. raw values divided by the effective area being covered) of the metrical and topological properties over the years being considered. If the expansion is uniform, we expect to see flat regions in the spatial density plots. In figures 3(e)–(h), however, we find generally increasing trends, especially at the later years, signifying a densified development.
Despite the generally increasing trends in the density values, interestingly, both the road count density N/A (figure 3(e)) and road length density ρL (figure 3(f)) show dips in 1908. These signify exploration; the road network increased in longer and curved roads following the terrain instead of short, densified roads forming blocks. The decrease in N/A in figure 3(e) is associated with the fact that the Americans extended some pre-existing eastward roads, increasing the extent of the convex hull without significantly increasing the number of roads. On the other hand, the decrease in ρL for 1908 suggests that the exploratory mechanisms dominate over the densification, resulting in large swaths of areas without roads in the east, as can be observed in figure 2 between the 1894 and 1908 maps. Incidentally, a similar decrease in ρL is observed for the contemporary map (figure 3(f)). This is attributed to the fact that the city has grown in area due to reclamation, and that the newly formed roads are well-planned, resulting in an increase in the convex hull area that is not commensurate with the increase in total road length.
Finally, the ρi (figure 3(g)) and ρe (figure 3(h)) count densities are found to follow mostly the trends of the actual counts in (c) and (d) respectively, signifying that the road network complexity is independent of the actual area spanned by the roads in space. This, again, is illustrative of the densification process; in many instances, the increase in the intersections (nodes) and the corresponding street segments (edges) happens in the interstitial spaces between the larger blocks that are already present in the network and less likely to happen for peripheral areas not yet reached by previous roads.
4.3. Invariant statistical properties
Despite the various events that have happened throughout its historical past, and even in the absence of a centralized planning body in modern periods, we observed statistical regularities in the Manila road network over time. Figure 4(a) shows that the road lengths follow unimodal fat-tailed distributions P(L) throughout the years. The distributions are highly skewed and non-normal, implying that the creation of roads is not simply a random process. Similar trends have been observed for other data sets such as London, United Kingdom [28], Amiens, France [21], and even for the global road network (GRN) [31], which the researchers opted to model with different fitting functions. For London [28], the trend is fitted by a log-normal behavior. On the other hand, the length distribution for Amiens [21] was fitted with a combination of an exponential and a powerlaw. The choice of fitting function indicates that there is no pre-existing typical road length scale; road creation in cities is a multiplicative process of cutting through bigger blocks until a considerable homogenized state [27]. Interestingly, the GRN [31] is found to collapse into a universal form upon rescaling by the characteristic quantities of the road network, regardless of the type and function of road. These studies strengthen the case for identifying the road network as a complex system that produce emergent statistical features.
Figure 4. (a) The road length distributions P(L) of Manila over the years show a unimodal trend suggesting similar mechanisms of road creation. (b) The tails of the distributions collapse to a power-law with exponent of 2.90 after rescaling with their corresponding mean. (c) Obtaining the universal scaling function by plotting Lγ ⋅ P(L) by L/⟨L⟩α, where γ = 1/(2 − α) (here, α = 1) highlights the deviations of the contemporary network at the shortest scales, possibly indicative of the planned nature of development over the last few decades. (d) The modern map (blue) with the convex hull defining the 1875 road network span (red). (e) Conditional P(L) distributions for regions inside and outside the 1875 convex hull, showing the densification of the modern road network (i.e. short-road preponderance) within the 1875 expanse.
Download figure:
Standard image High-resolution imageIn the case of Manila, we observe generally unimodal distributions, as shown by figure 4(a). The unimodality of the distributions suggests a similar mechanism for the road creation even in the absence of a strong, centralized planning and strict zoning rules. In the case of Amiens, a bimodality is observed due to local modifications [21]. However, one feature of the distributions shown in figure 4(a) that is deemed to be more important are the fat tails that appear to follow similar trends. The persistence of the fat-tailed behavior for all the years considered implies that very long roads were deliberately created during the exploration stages that served as main trunks from which shorter local roads emerge during the later densification. And, throughout the evolution of the network, these longest roads are rarely, if ever, being cut into shorter roads, thus preserving the statistics at the tails.
Because of their particular significance, we first focused on an approximate description of the tails of P(L). In figure 4(b), we rescaled the distributions by their correponding mean to remove the variability for all the years. Interestingly, the tails of the distributions collapsed under a single curve, while the short road regimes did not. The tails of the distribution all scale as P(L) ≈ L−ζ, where ζ = 2.90. This is consistent with previous findings for the 2016 network of Manila [22]. This observed invariance is a result of the consistency of the hierarchy of roads in the network [20]. Note that the Manila road network grew organically over the years; it is impossible to have had a continuous, coherent plan that spanned the entire period being observed here, due to the various historical conditions of the city. The collapse into a universal scaling with exponent ζ is an emergent property that is manifested in the temporal domain of the road network evolution. Incidentally, previous works have shown that ζ is also a universal scaling exponent of the P(L) tails for all the cities in the Manila conurbation [22].
The shorter-than-mean regimes in P(L) give us an insight into the degree of the densification process. As shown in figures 4(a) and (b), the fraction of the very short roads in the 2019 P(L) significantly increased compared to the other years, even upon rescaling with the mean. This suggests that the Manila city government has fairly recently embarked on a major road-building process with very densified (i.e. short roads) geometries, consistent with the sudden spikes in figures 3(a) and (b) and observed in figure 2.
To further highlight the extent of the creation of very short roads, we use the rescaling procedure utilized by Strano et al [31] for the GRN. The universal scaling function F(⋅) may be obtained by plotting Lγ ⋅ P(L) with L/⟨L⟩α, where γ = 1/(2 − α) ensures normalization. In figure 4(c), the case of α = 1 (and, thus, γ = 1) is presented, i.e. rescaling by the simple mean road length ⟨L⟩ of the data. As expected, all the distributions collapse into the universal scaling function upon the proper rescaling. Noticeably, the contemporary road network shows higher regimes at the very short road length scales, that deviates significantly from the collapsed plots for the other maps. This appears to indicate the nature (i.e. very short roads) and extent (i.e. significantly higher preponderance) of the recent densification of Manila, which, by comparing with figure 2, is highly planned.
The densification is not confined to the reclaimed areas, however. To probe the road growth within the preexisting road layout, we plot in figure 4(d) the contemporary road network, and mark the convex hull of the 1875 network, the oldest historical map that we have. We establish an arbitrary boundary between the regions within and outside the 1875 convex hull and present the conditional road length distributions in figure 4(d). A quick comparison between the original road length distribution of 1875 and that of the modern network within the 1875 bounds reveals an order-of-magnitude shift in the mode towards the shorter L values, and an increase in the actual probability density P(L) at this new modal value. This shift quantifies the within-city densification, which, along with the planned development at the reclaimed areas, contributed to the short-L regimes in the P(L) distribution of the 2019 network.
We also looked at the distributions of the closeness ψ and betweenness centralities δ of the networks from the different periods and present them in figure 5. As stated previously, the ψ and δ values are the most associated with physical space among all centrality measures. Previous works have indicated that their distributions (particularly, that of the δ) show statistical consistency across various city data sets [33].
Figure 5. Closeness centrality (top panels) and betweenness centrality (bottom panels) distributions, presented as (left to right): (a) and (d) raw probability densities; (b) and (e) distributions rescaled by the mean; and (c) and (f) rescaled relative frequency distributions.
Download figure:
Standard image High-resolution imageThe P(ψ) distributions (figure 5(a)) are expected to shift to smaller values simply because of its definition as an inverse of the spatial extent; the average shortest distance increases with continued spatial growth. Upon a simple rescaling with the mean (figure 5(b)) or by the scaling function (figure 5(c)), the characteristic modes appear to align near the value of the mean, or at ψ/⟨ψ⟩ ≈ 1. One noteworthy feature of the contemporary map that is observed in figure 5(b) but is not readily apparent from figure 5(c) is the large proportion of ψ → 0 relative to the other periods. This adds a detail into the picture of the densification process described earlier. The sudden surge in the number of nodes with very small ψ suggests that the densification phase—already visualized in figure 2, and found to be due to very short roads in figure 4(c)—happened at the periphery, significantly far away from the other pre-existing nodes. This, of course, is expected of the reclamation areas.
Figure 5(d), on the other hand, shows the P(δ) across the different periods, with a general shape that resembles the one observed previously for other road networks and for random planar graphs [33]. A simple rescaling by the mean in figure 5(e) significantly aligned the tails of the distributions, while it can be shown that a rescaling by the number of nodes ni results in the matching of the secondary peaks [33]. When the rescaling is done to reveal the relative frequency distributions (figure 5(f)), the contemporary network reveals a significant proportion of very small δ nodes, which may be attributed to the fact that the planned development at the reclamation areas suddenly increased the number of nodes with low importance in terms of bridging the others efficiently.
These results paint a picture of continuing development of the road network despite the geophysical constraints faced by Manila. As observed previously, the densification of Manila, as measured by the significant increase in the P(L) of very short roads (figures 4(a)–(c)) happened from (i) within the existing road boundaries (figure 4(e)); and (ii) at the periphery (figure 5(b)). The fact that the P(δ) also shows an excess of very small values (figure 5(f)) suggests that the peripheral densification dominated the statistics: if the development were from within, the P(δ) would only rearrange the most important bridging nodes and not produce regimes having very small importance.
4.4. Spatial distribution of central nodes
After discussing the evolution of the road network through the historical map, we focus our attention to the contemporary map. Since road networks are the oldest extant elements in the urban landscape, we expect that the historical development of the city must be embedded in their architecture, and can be extracted automatically from the current network.
In figure 6, we present the locations of the nodes with centralities above the percentile Q (black points, middle and bottom columns) in the 2019 map. To provide context, the historical road networks (black lines, top column) are also presented, along with their convex hull areas (colored overlays). We observed stark differences in the spatial arrangements of the highest ψ and highest δ nodes.
Figure 6. Evolution of the network, presented with the contemporary map where the nodes with highest ψ and δ values are highlighted. The development of the road network extent is embedded in the spatial distribution of highest ψ nodes.
Download figure:
Standard image High-resolution imageThe spatial configuration of the highest ψ nodes (middle panels) is found to mimic the layout of the historical road networks. This indicates that the development of the road network preserves the historical core; new pathways in the network aim to connect new locations to these central locations. For the case of Manila, this historical core is found to be around the Intramuros area, the oldest district in the city. Additionally, the spatial arrangement of the highest ψ nodes can also indicate sudden, well-planned developments within the city. When the cluster of nodes with the highest closeness centralities deviates from the area defined by the core historical portions of the network, the directionality of the shift should point to the regions of sudden, densified construction that did not undergo the self-organized process of expansion and gradual block homogenization. For example, in the Manila road network, the high-ψ clusters show a slight bias to the northeast, indicated by the grid-like regions that are outside of the convex hull of the historical maps. This, of course, is introduced by the planned construction of Quezon city, as discussed previously.
The automatic identification of the historical core regions from just the high-closeness-centrality node clusters in the contemporary map has practical significance, as it gives an easy window for highlighting the risk-prone areas in terms of urban development. The oldest quarters in the city contain heritage structures that are oftentimes preserved, making them inflexible to modern urban development. More importantly, these districts are oftentimes created without regard for the eventual rapid pace of urbanization, making them prone to traffic congestion or urban decay. For example, the ψ > Q90 clusters in Manila are found to correspond to the Binondo district, one of the oldest Chinatowns in the world and one that organically grew to accommodate foot and horse traffic.
In contrast, the spatial profile of the highest δ nodes of the contemporary map (bottom panels) reveal no such comparable patterns as the historical structure of the road network. The spatial arrangement of connectivity is expected to vary radically as new developments are being added into the network. However, it has been suggested that these high-δ nodes indicate possible locations of traffic congestions [33]. In figure 7, we obtained traffic information in the form of annual average daily traffic (AADT) from the data of actual car counts by the Metri Manila Development Authority (MMDA), Philippines from 2012–2018 (figure 7(a)) [47] and a model projection of volume vs capacity for 2030 by the Japan International Cooperation Agency (JICA) [48]. Superposing the traffic data with the δ > Q90 nodes from figure 6 reveals that the traffic chokepoints indeed lie along the paths of the highest-δ nodes.
Figure 7. (a) Annual average daily traffic (AADT) on important Manila roads from 2012–2018 as reported by the MMDA (b) simulated traffic situation on Manila roads without intervention by 2030 conducted by the JICA. For both panels, nodes with the highest betweenness values (90th percentile) are plotted in black. Noticeably, these black circles (high betweenness) trace the roads with high traffic load (orange and red lines).
Download figure:
Standard image High-resolution image5. Conclusions
In this work, we have expanded the temporal extent of previous studies focused on the road network of Manila via georeferencing and map digitization. Historical studies such as this one naturally report two aspects of the development: (i) which properties have changed through time; and (ii) which ones have been preserved. Here, through geographical and topological characterizations, we have presented a quantitative description of the historical development of the city, one that is tied to the socio-political conditions during the development of the network and the city in general. Several implications to the current situation and sustainability are also provided.
The visual changes in the geographical layout of the roads revealed the imprints of development during key historical instances, and these are also supported by the observed trends, particularly in the area densities of the physical and topological metrics. For example, the changes that transpired between the 1894 and 1908 maps have been captured by the dips in the road count and road length density plots, suggesting a sudden exploratory phase in road network development during the early American colonization period. Two periods of highly-planned development punctuating the otherwise self-organized growth have been captured: one during the tailend of the American colonization, when Quezon city was built, that was captured by the 1945 map; and another during the recent decades, when the socio-economic conditions allowed for massive reclamation projects that utilized well-defined blocks of very short roads. We firmly believe that when these analyses are done for the road networks of other urban conurbations, a lot of important information can similarly be extracted, because road networks, as discussed in the introduction, contain indelible records of socio-political conditions of the civilizations that built them.
The road networks preserve some statistical properties in time. For example, the road length distributions show tails that scale as power-laws with exponent −2.90, due to the fact that longer roads that result from the early exploration phase of the evolution are usually preserved in space, i.e. they do not get 'sliced' into smaller pieces. The road length distribution also collapsed to the universal scaling function reported from the global road network analysis [31], although the recent well-planned development in Manila resulted in the preponderance of very short roads. The distribution of closeness centralities and betweenness centralities also show an alignment of modes upon rescaling; for Manila, however, the higher frequencies at the very small values of these centralities further highlight the peripheral planned growth through land reclamation. The statistical regularities found in the evolution of the road network indicate the dominance of a self-organized, emergent growth.
Apart from the preservation of the statistical distributions, we also recovered spatially preserved features in the form of clusters of high-closeness centrality nodes. For the case of Manila, these clusters roughly correspond to the oldest city sections. Additionally, they also point to regions of sudden, well-planned developments within the city bounds. Lastly, we observe that the high betweenness centrality nodes are sensitive to the network growth and are thus not preserved spatially throughout the network evolution; instead the segments of nodes of highest betweenness correspond to the traffic hotspots in the network.
The work presents a relatively straightforward means of uncovering the wealth of historical information that are stored in road networks. Despite the differences in their socio-economic conditions, different urban conurbations in the world can be subjected to the spatio-temporal analyses presented here to reveal the historical patterns of evolution. Not only will these works reveal the commonalities emergent in road network growth; additionally, they can reveal properties that may be unique to the geographical region being considered. Knowledge of these properties can guide future strategies for managing urbanization.
Acknowledgments
The authors acknowledge the Filipinas Heritage Library and the National Archives of the Philippines for the historical maps. The authors thank Prof. Ari Ide-Ektessabi of the Advanced Imaging Technology Laboratory (AITL) of the Kyoto University for the digital scanning of the maps. M T C would like to acknowledge the financial support from the Advanced Science and Technology Human Resources Development Program (ASTHRDP) of the Science Education Institute (SEI) of the Department of Science and Technology (DOST), Philippines.
Data availability
The data that support the findings of this study are available from the corresponding author upon reasonable request.