Abstract
Polarimetry is a powerful tool for the analysis of the optical properties of materials and systems, such as human skin. However, in many polarimetric setups, the field of view is limited to a few square centimeters. In these cases, it is possible to resort to stitching techniques, which involve combining multiple Mueller matrix measurements obtained from different overlapping regions of the sample. In this paper, we propose a stitching technique for polarimetric data and discuss its advantages and limitations. We also describe the potential of image stitching for improving the accuracy and robustness of in vivo polarimetry in the presence of random patient movement. We conducted our research using a diverse set of samples which included porcine skin, human skin from arms and fingers, cold cuts of chicken and gelatine, alongside synthetically created sample data. Our results demonstrate the effectiveness of this technique for the application in dermatology. Each additional in vivo measurement enhances the field of view by approximately one third, thereby considerably augmenting the total observation area. We show that stitching enables for the polarimetric assessment of large skin patches which is useful for the diagnosis of inflammatory skin diseases.
Export citation and abstract BibTeX RIS

Original content from this work may be used under the terms of the Creative Commons Attribution 4.0 licence. Any further distribution of this work must maintain attribution to the author(s) and the title of the work, journal citation and DOI.
1. Introduction
In recent years, Mueller matrix polarimetry (MMP) has emerged as a powerful tool for characterizing the polarization properties of materials. It has a wide range of applications in fields such as optics, materials science, biology, and medicine including dermatology [1, 2].
In recent years, advancements have been made in contactless dermoscopy and other noninvasive optical modalities, and polarization imaging is also gaining importance [3–7].
In polarimetry for dermatology, stitching can be used to expand the variety of applications for polarization measurements. In skin imaging, it would be beneficial to compare the malignant skin area under study to the benign skin areas around it. Additionally, when it comes to the early detection of skin cancer, the size of the skin lesions, also denoted as nevi, can present a significant challenge. In MMP, capturing the entirety of a large nevus with sufficient spatial resolution in a single measurement can be difficult, leading to incomplete or fragmented data. However, even widefield polarimeters have relatively small fields of view (FoV) [8], e.g. the FoV of the polarimeter employed in this work is approximately 3 cm by 2 cm. For systems lacking a broad FoV, stitching may present a viable solution. In MMP, stitching involves combining multiple measurements taken at neighbouring skin spots to produce a more comprehensive characterization of the skin. In the context of skin imaging, stitching can be used to increase the FoV and enable the study of large patches of skin, such as in the case of inflammatory skin diseases.
Another reason why stitching may be used in polarimetry is the potential to reduce measurement errors. By stitching of multiple measurements, it is possible to average out some errors or uncertainties that may be present in individual measurements, resulting in a more accurate measurement [9].
Additionally, liquid crystal retarders (LCRs) are often employed in polarimeters because they permit the measurement of multiple polarization components by manipulating the induced retardance using an electric field. In general, however, the optical retardance induced by these devices is not uniform across the aperture. Here, stitching of measurements from the centre of the aperture could also reduce the error induced at the edges of the LCRs [10].
In this work, we explore the use of stitching in Mueller matrix polarimetry for skin imaging. We discuss the principles of stitching, its benefits and limitations. We employed various samples, both synthetic and biological, ranging from a printed symbol on paper, cold cut of chicken meat and gelatine, porcine skin, to human skin from arms and fingers. Our work paves the way for future investigations in Mueller matrix polarimetry and stitching techniques, with potential applications in diagnosing and characterizing inflammatory skin diseases. We will also highlight some of the key challenges and opportunities for future research in this area, including the potential for stitching to enable the study of large patches of skin and the characterization of inflammatory skin diseases.
2. Methods
2.1. Mueller matrix polarimetry
Mueller matrix polarimetry involves representing the polarization changing properties of a sample using a 4 × 4 matrix called the Mueller matrix [11].
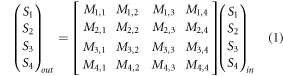
Here, and
denote the Stokes vectors of outgoing and incoming light, respectively. A Stokes vector, composed of four parameters characterizes the polarization state of a light wave. The Mueller matrix can be used to describe the effects of more complex polarization-modifying samples, such as those that introduce changes in phase or intensity of the light [12]. It can be used to describe a wide range of polarization phenomena, including depolarization power, retardance, diattenuation and polarizance [13]. Depolarization power refers to a mediums's ability to randomize the polarization state of light. Retardance is the phase difference created by a material between two orthogoanl polarization components of light. Diattenuation describes the polarization-dependet absoprtion of light. Finally, polarizance is the differential transmission of orthogonal polarization states through an optical element.
Figure 1 shows a schematic of an exemplary measurement with the employed polarimeter in diffuse reflection mode.
Figure 1. Schematic on the Mueller matrix polarimetry setup (top). It consists of a polarization state generator (PSG) and a polarization state analyzer (PSA) which are both build from a linear polarizer and two liquid crystal retarders, respectively (bottom). The generated polarization states are linear vertical (V), linear horizontal (H), linear at +45° (P), linear at −45° (M), right circular (R) and left circular (L).
Download figure:
Standard image High-resolution imageFor our experiments involving the 532 nm laser, we utilized a power of 1 mW. For those involving the 633 nm laser, the power was 10 mW. The dual-wavelength configuration facilitates the investigation of wavelength-dependent polarization characteristics of skin tissues, attributed to their differential penetration depths and scattering properties. For the current study, data was predominantly acquired using the 532 nm laser wavelength, chosen due to its efficacy in balancing polarimetric contrast in skin samples.
To perform a MMP measurement, a laser light source is used to illuminate the sample under study, and the polarization state of the transmitted or reflected light is measured [14]. Figure 2 illustrates the data acquisition process.
Figure 2. Exemplary data acquisition needed to determine the entry of the MM. For the calculation of
four measurements XY (X is the state of the PSG; Y is the state of the PSA.) are required. The sample is illuminated with light of one particular polarization state. The reflected light is analyzed by a polarization sensitive filter.
Download figure:
Standard image High-resolution imageOnce the detector intensities for the individual polarization settings of the light have been measured, the Mueller matrix can be calculated using mathematical algorithms, which can be found in the literature [15] and figure 6. Equation (2) shows the exemplary calculation of from the data acquistion illustrated in figure 2.

The elements of the Mueller matrix can then be analyzed to extract information about the polarization properties of the sample, such as its degree of linear or circular polarization, its diattenuation and retardance, and its degree of anisotropy [16]. The entry is utilized for the normalization of all MM entries.
Our system's precision in polarization imaging is ensured by calibration using the Compain method [17] and regular tests with known optical samples. We have implemented various measures to address signal-to-noise improvement, including a controlled environment that minimizes ambient light and consistent calibration checks. Any deviations observed in the experimentally acquired MMs of samples with known polarization properties are within acceptable limits for our applications.
2.2. Stitching of polarimetric data
Image stitching is the process of combining multiple images into a single, larger image. It is a technique that is commonly used in photography and computer vision to create panoramic images or to extend the field of view [18].
Figure 3 displays the effect of the random patient movement (due to shaking, breathing, etc) on the data acquisition for the case of MMP.
Figure 3. Due to patient motion, every pair of stitched images contains a unique region of skin overlap. Only in the intersection of area 1 and area 2, the Mueller matrix is completely defined. In the remaining regions, only some entries of the Mueller matrix can be fully described (see figure 6). The overlap is necessary for the stitching since a sufficient number of matching key points are required. One measurement constitutes the acquisition of the 36 raw images, which are illustrated by the blue squares.
Download figure:
Standard image High-resolution imagePatient motion moves the FoV for every acquisition and results in a small region of skin overlap. The complete specification of the Mueller matrix is only possible within the intersection of area 1 and area 2, shown in figure 3. In the residual regions, the description of the Mueller matrix remains partially incomplete, with only certain entries being fully defined. Figure 6 also shows the raw data requiring overlap for the computation of the MM entry. Strong patient motion could potentially result in insufficient overlap between these raw data images, impeding the calculation of the MM entry consequently.
The overlapping region is critical in our work with Mueller matrix polarimetry, particularly for aligning images of diverse samples like porcine skin, human skin from arms and fingers, and synthetically created sample data. Without a sufficient overlap, it becomes challenging to precisely correlate the same areas of the skin across the 36 images capturing similar, but not identical, skin regions. The overlap enables the algorithm to identify a substantial number of corresponding key points, which greatly aid in the accurate alignment of the images. In the absence of an overlap region, the process of manually finding matching pairs becomes a significant hurdle. This not only proves time-consuming but may also be practically impossible to achieve with high accuracy. Consequently, the overlap plays a key role in enhancing the precision and efficiency of image stitching in our study and facilitates the robust analysis of large skin patches. Figure 4 illustrates the two settings that can be utilized for data acquisition, particularly when trying to measure a larger field of view.
Figure 4. Patient- (left) and system-based (right) movement. System movement offers potential stability by fixing the skin region and allows integration into body scanning systems. In contrast, patient movement is cost-effective but reproducibility is lower.
Download figure:
Standard image High-resolution imageFor the data acquisition, either the patient or the system has to be moved. System movement might lead to a reduction in random patient movement as the skin region under study can be fixed slightly. Additionally, it could be integrated into total body scanning systems. While patient movement is the more cost-effective and simpler to execute option, it introduces complexity in terms of ensuring reproducibility and control.
There are several techniques that can be used for image stitching. Feature-based stitching involves detecting distinctive features in each image and using these traits to align and stitch the images [19]. Homography-based stitching methods use a mathematical model known as homography to transform and align the images, taking into consideration any perspective shifts that may have happened [20]. Direct techniques compute the best transformation directly from the images, without intermediate processes such as feature extraction or homography estimation [19]. The choice of the stitching approach will depend on the specific requirements and constraints of the application, such as the type and quality of the images, the desired level of accuracy, and the computational processing resources available [21].
In this study, we propose a robust feature-based image stitching technique for polarimetric data for in vivo MMP. Additionally, the method provides an alignment functionality. Further, the method is capable of automatic and manual polarimetric data stitching. Figure 5 illustrates the developed stitching process.
Figure 5. Flowchart of the proposed image stitching algorithm for polarimetric data. Starting with raw data acquisition from multiple measurements, the KAZE technique identifies and matches distinctive image features, either automatically or manually. Following stitching, images are aligned using a chosen reference image. To meet the MM algorithm criteria, final images are cropped or padded to ensure uniformity in size.
Download figure:
Standard image High-resolution imageThe algorithm consists of the three main steps: stitching, aligning and cropping. Firstly, the raw data of at least two measurements is acquired. The algorithm will detect and extract the image features before matching them. With a sufficient number of matching feature pairs, the two images will be stitched. Otherwise, the operator can manually choose at least two matching pairs of features. This is continued for all 36 polarimetric input images recorded. A high number of matching feature pairs ensures high stitching quality. We integrate automatic feature-based registration with manual control point mapping. The feature extraction and description in this system are performed using KAZE [22], which has already been succesfully employed for the registration of in vivo polarimetric data [23]. Using this technique, feature detection identifies the regions of an image that have distinctive information, such as blobs [24]. The feature detection locates potential key points that are not always related to physical structures. It is important to identify features that are locally invariant so they can be recognized even in the presence of rotation or scaling [25].
The stitched images are aligned to one another in the second step. To align all stitched images, we employed a similar approach like the stitching method, using feature-based and control point mapping tools. The technique provides the option to select a stitched image as a reference, before aligning all other stitched images to that reference image.
The MM algorithm requires all stitched images to have the same size in order to calculate the MM after stitching and aligning the stitched images. To solve this problem, the algorithm can crop the stitched and aligned images to the area of interest chosen by the user, the maximum reference overlap zone of all images, or add zeros in the missing areas, which is the standard configuration.
Each full measurement cycle comprising the acquisition of 36 raw data images typically takes about 20 seconds. This duration can be subject to slight variations depending on the reflective properties of the particular skin sample being examined. On the other hand, patient motion, especially in a clinical setting for in vivo measurements, tends to occur on a much shorter timescale. Thus, despite the relatively brief duration of each measurement, the potential for patient movement during this period underscores the importance of stabilizing measures and careful timing to maintain the integrity of the data collection process. Figure 6 visualizes the data loss resulting from random patient motion.
Figure 6. Top: Random patient movement reduces the skin area with defined MM. Each color patch rectangle represents an image of the raw data. Different MM entries can be determined depending on the overlap of the raw data. Bottom: Calculation of the MM from either 16 or 36 images.
Download figure:
Standard image High-resolution imageThe colored patches represent a single image, e.g., the violet patch shows the HH image. For visualization purposes, we only show six of the 36 acquired images. The dashed line rectangle marks the skin area in which the MM entries
and
are defined, as the images HH, HV, VH and VV have a common overlap. The solid line rectangle shows the skin area in which the additional entries
and
are defined, i.e. where the images HH, HV, VH, VV, HP and VP have a common overlap. The MM can be calculated either from 16 or 36 images, where the latter improves the overall measurement accuracy. Stitching can counteract the reduction of the field of view which is reduced by random patient motion.
2.3. Blending
Blending is a commonly used technique in image stitching where the pixels of two or more images are combined to create a seamless view. We employ three specific feature-based stitching techniques and consider their accuracy, their efficiency, and their wide application in related fields [26, 27]. Firstly, compared to other techniques such as intensity-based methods, feature-based techniques tend to yield higher accuracy in feature matching, a crucial factor in our work. In fact, preliminary attempts using intensity-based methods produced less satisfactory results in terms of alignment precision [23]. Feature-based methods are also particularly fast and straightforward to implement [28]. This efficiency becomes an important consideration when dealing with numerous images that need to be processed in a reasonable timeframe.
The blending process can be accomplished in various ways, one being alpha blending [29]. In this research, three blending approaches are compared in the context of polarimetry.
A fundamental method for stitching images is called overwrite blending, which involves swapping out a specific area of one image for a comparable area of another image. According to the following description, the mathematical model for overwrite blending.
Suppose that, A and B stand for two images of the size M × N. In order to accomplish overwrite blending, we can build a binary matrix W of size M × N, where W(i,j) = 1 if pixel (i,j) in image A should be replaced by the matching pixel in image B, and W(i,j) = 0 otherwise. The final blended image, C, can be understood as by:

Depending on the value of W at that position, equation (3) calculates the blended pixel value at each location (i, j) as a weighted sum of the corresponding pixel values from A and B. The pixel value from B is applied if W(i, j) = 1; otherwise, if W(i, j) = 0, the pixel value from A is used.
A method of overlaying and blending, which is used to stitch images, involves layering one image on top of the other and modifying the opacity of the top image to achieve a transition that is smooth. The mathematical model for overlaying blending can now be described as follows:
Suppose again that, A and B are two images with dimensions M × N. The blending function F(x,y), which mixes the pixel value x from image A with the equivalent pixel value y from image B to create the final pixel value F, can be used to realize overlaying blending. A definition of the blending function is:

where the blending coefficients a and b regulate the opacity of the two images. Typically, a + b = 1, so that the total opacity of the blended image is 1.
The process of mixing two or more images in a way that creates a smooth and seamless transition between them is known as seamless blending.
This variant of blending involves the application of a weight function. We can create a blending function, G(x,y), that takes the pixel value x from image A and interacts it with the equivalent pixel value y from image B to create the final pixel value G. This allows us to execute flawless blending. One definition of the blending function is:

where w(x) is a blending weight function that establishes exactly how the two images are blended. This method typically computes the blending weights by examining the gradients, colors, or frequencies of the overlapping portions of the images.
In figure 7, the working principles of the stitching methods are visualized.
Figure 7. Visualization of the three image-stitching methods employed. The overwrite blending method directly takes pixel values from either image 1 or 2, resulting in a direct replacement without transition in the fused image. In contrast, both the overlaying blending and seamless blending methods modify pixel values in the overlapped regions to ensure a smooth transition between the images. Overlaying blending superimposes one image over the other, while seamless blending also modifies pixels outside the overlapped regions.
Download figure:
Standard image High-resolution imageWith the help of pixel visualization, figure 7 depicts the three different stitching techniques. It shows that overwrite blending uses either the pixel value from image A or image B for the fused image.
The pixel values in the overlapped regions are changed in overlaying blending and seamless blending, respectively, to produce a seamless transition between the images. This can be seen in figure 8 along with a comparison of stitching methods using raw data from pork skin.
Figure 8. Comparison of stitching results on the raw data. The sample is pork skin. The images with the red and green edges are the input imags which are being stitched. The top-right images in all three examples show where the input images complement each other. The bottom-right images show the stitching result for the different techniques.
Download figure:
Standard image High-resolution imageFigure 8 also shows that the stitching technique can have a strong influence on the relative distribution of the pixel intensities in the fused image.
3. Results
Results were generated in both controlled and in vivo conditions, the latter reflecting practical conditions seen in dermatological applications. Additionally, synthetically generated data was studied. In the initial step of the algorithm, the image processing module performs feature extraction on the input images using the KAZE features. The algorithm then matches corresponding feature pairs between the images. The identification of true matches plays a crucial role to produce an accurate and reliable output.
3.1. Synthetic data analysis
Figure 9 presents the MMs derived from synthetically generated data. The synthetic dataset, created with MATLAB, consists of 36 distinct images. Each image features a polygon randomly positioned within a 500 × 500 pixel grid. Notably, the greyscale intensities of the foreground (the polygon) and the background exhibit random variations within a predetermined range.
Figure 9. Depiction of synthetic data illustrating the dependency of the resultant Field of View (FoV) on specific entries within the Mueller Matrix (MM).
Download figure:
Standard image High-resolution imageThe findings from the stitching process applied to the synthetic raw Mueller matrix data, particularly under conditions involving motion, reveal an intriguing characteristic: the resultant field of view (FoV) differs across each Mueller matrix entry post-stitching and computation. This suggests that the areas determining each Mueller matrix entry are not universally applicable or consistent throughout. In contrast, upon examining the original images from which specific Mueller matrix images are computed, it becomes clear that the defining areas for each Mueller matrix entry are distinctly unique. This discrepancy underscores the complexity and variability inherent in the process of stitching polarimetric raw data.
Since overwrite blending does not modify the pixel values, it ensures consistency in the MM calculation across all images and avoids any potential inconsistencies that may arise from using different blending techniques. On the other hand, overlaying and seamless blending change the pixel values which affects the MM calculation and introduces an error in the analysis. By its inherent nature, overwriting does not have the potential to mitigate the error generated by noise. To demonstrate this, we create synthetic polarimetric raw data, which allows us to illustrate how various stitching techniques are capable of reducing the error stemming from random or speckle noise. The use of synthetic data thus provides an effective platform to investigate and compare the noise reduction capabilities of different stitching methodologies. Figure 10 illustrates the outcomes of the stitching process, specifically highlighting noise reduction in the context of synthetic raw data.
Figure 10. Depiction of synthetic data demonstrating the potential for noise reduction in MMs through the appropriate application of stitching techniques. The yellow marked upper half of each entry is showing the results for Gaussian Noise data. The lower half of each entry shows the results for the data as described above the respective matrix.
Download figure:
Standard image High-resolution imageIn figure 10, we present the resulting entries of the Mueller matrices after the application of various stitching techniques. A visual examination of the data reveals a notable reduction in noise between the yellow marked Gaussian Noise data and the stitched data, contingent upon the employment of suitable stitching methodologies. This observation underlines the effectiveness of strategic stitching in attenuating noise interference, thus enhancing the reliability of the Mueller matrix outputs.
Figure 11 shows the standard deviation for all entries within the stitched Mueller matrices in the horizontal direction. This is demonstrated across different stitching techniques.
Figure 11. Depiction of synthetic data demonstrating the potential for noise reduction and precision enhancement in MMs by showing the horizontal standard deviation through the appropriate application of stitching techniques. The standard deviation is reduced by a half for most entries.
Download figure:
Standard image High-resolution imageFigure 11 presents the computed standard deviation for each entry in the horizontally-oriented stitched Mueller matrices across different stitching techniques. The black arrows show that the standard deviation is reduced by a half for all stitching methods indicating a noise reduction. The absense of significant variation in standard deviation values - as marked with the black ellipses - showcases the potential impact of the stitching process on the accuracy of the resultant matrices. However, no specific stitching technique seems to be particularly more effective than the others. This may be due to the following: In the process of image alignment, specifically during the geometric transformation and warping stages, the images may undergo subtle modifications. However, these alterations are not inherently aimed at blurring or denoising of the images. The perceived blurring or denoising might be a by-product of the transformation and warping process. Notably, during the warping phase, the images undergo interpolation, which, in many cases, might lead to a semblance of blurring. This effect appears to be more pronounced than the noise reduction associated with the stitching technique. The premise for this observation is that the overlapped region of the stitched images consistently retained its noise levels, irrespective of the stitching technique employed.
These observations suggest that the transformation and warping procedures inherent to image alignment might inadvertently induce some blurring effects, thereby reducing the noise levels in the stitched images. The blurring effect introduced by these procedures supersedes the potential noise reduction that could be achieved through the various stitching techniques, as indicated by the invariant noise present within the overlapped regions of the stitched images.
In figure 12, we present the raw data of HH images corresponding to both the synthetic and stitched data sets. The figure also includes the corresponding histograms for these two data sets. These histograms provide a detailed illustration of the pixel intensity distributions, further accentuating the contrasts and similarities between the synthetic and stitched data. The data representations facilitate the analysis of image transformation effects on the pixel distribution, thereby aiding in understanding the fundamental changes occurring due to the stitching process.
Figure 12. Top: Visual representation of the transformation-induced blurring effect on Mueller Matrix raw data with the HH polarization component of the raw data. Bottom: Histograms of the HH polarization images for the synthetic and the stitched data. The Y-axis shows the normalized count and the X-axis gives the pixel values.
Download figure:
Standard image High-resolution imageThe top-left panel shows the original synthetic data with notable noise levels. In contrast, the other panels illustrate the resultant image post-stitching, where the noise appears to be noticeably reduced, indicating the potential blurring effect introduced by the transformation process. The comparative analysis of these images visibly demonstrates the blurring that is inherent to the image transformation during the stitching procedure.
The potential for noise reduction in Mueller matrix polarimetry via image stitching could depend upon the noise level and noise type; further exploration in this realm could be beneficial in future studies. However, in the context of the current setup, this differentiation is not significant. Nevertheless, caution must be taken when implementing stitching techniques. The transformation processes involved in image stitching can inadvertently manipulate the Mueller matrix data, potentially introducing artefacts or biases. Therefore, the careful management and interpretation of these data transformations are crucial in the generation of accurate and meaningful polarimetric analyses.
3.2. Ex vivo sample analysis
In the controlled experimental setup, we used a printed symbol on paper as a phantom. The generation of phantom data involved the motion of the phantom during the acquisition of raw data. Subsequent image processing divided the image into two sections, labeled as the left and right portions. These portions were designed to share a significant overlap of 50%, meaning half of the content from each image could be located in its counterpart. This technique provides a reliable means of showing the alignment and image quality.
Figure 13 shows the exemplary raw data of the phantom (HH, HM, HP, HR and HV) and the corresponding stitching result. Additionally, the resulting MMs for the different stitching techniques are displayed.
Figure 13. Top: Stitching results of exemplary raw data of the desgined phantom. Bottom: Resulting MMs for stitching of the phantom data for the three stitching techniques considered in this work. The white circles exemplary mark the polarimetrix artefacts.
Download figure:
Standard image High-resolution imageThe stitching technique overwrite demonstrated excellent performance in recreating the accurate overall polarimetric picture. Nevertheless, the application of both alpha blending techniques—fused and seamless—can result in the generation of unwanted polarimetric artefacts. These could potentially distract dermatologists, particularly if Mueller Matrix Polarimetry (MMP) were to be implemented as an imaging technique in clinical practice, i.e. for in vivo measurements. The presence of these artefacts might inadvertently complicate the interpretation of images, thus potentially hindering the efficiency and accuracy of diagnostic procedures.
Firstly, we show that stitching is possible for a biological sample in a controlled setting without motion by stitching polarimetric data of a cold cut of chicken meat and gelatine.
Figure 14 shows the resulting MMs after stitching the raw data with three different techniques for the cold cut.
Figure 14. MMs of the stitched data of a cold cut sample consisting of pork gelatine and chicken meat laying on paper. At the top, a photograph of the sample is displayed. Some polarimetric artefacts are marked exemplary with white circles.
Download figure:
Standard image High-resolution imageFigure 14 illustrates the effectiveness of image stitching techniques in significantly extending the field of view, thereby enabling an integrated, complete visualization of the sample. This expanded view considerably aids in discerning and distinguishing the various components of the sample. It's important to note that without these stitching techniques, single measurements were unable to capture the entire cold cut slice, complicating the task of comparing and differentiating the various parts of the sample. Figure 15 on the other side displays a comparison of the MMs for ex vivo pork skin after stitching with three different stitching techniques.
Figure 15. Comparison of the MM entries for different stitching techniques on porc skin (ex vivo).
Download figure:
Standard image High-resolution imageDespite the sample not displaying pronounced polarimetric contrast, it illustrates the potential for improperly chosen stitching techniques to introduce extraneous polarimetric features or artefacts. These unwanted intrusions can create unnecessary distractions and potentially confound accurate interpretation, thus emphasizing the importance of careful and precise execution of stitching techniques in polarimetric imaging.
Figure 16 shows the MM result after stitching of raw data obtained from an ex vivo scar on porcine skin.
Figure 16. Resulting MMs after stitching of polarimetric data of an ex vivo porcine skin scar. The white circles indicate polarimetric artefacts. The scar region is exemplarly marked in of the overwriting result.
Download figure:
Standard image High-resolution imageThe scar on the ex vivo sample of porcine skin exhibits minimal polarimetric contrast. Only with the employment of the overwriting technique is it possible to ascertain the position of the scar. The other stitching procedures introduce artefacts, which render the scar indiscernible, thereby illustrating the critical role of appropriate imaging and stitching techniques in accurately capturing and representing skin features.
3.3. In vivo human skin analysis
However, in the clinical setting, where patient motion can occur randomly, the algorithm's effectiveness can be affected. Thus, it is essential to investigate the algorithm's robustness to patient motion to evaluate its practicality in real-world scenarios. In the following we study the stitching of polarimetric data for an in vivo measurement of two human fingers to provide insight into the algorithm's capabilities in handling the negative impact of a relatively small degree of random patient motion. The motion is minimized by pressing the fingers onto the optical table during the measurement.
Figure 17. Comparison of Mueller matrices from stitched polarimetric data of two human fingers for different stitching techniques. Both alpha blending techniques generate significant undesirable polarimetric artefacts in the form of horizontal lines. Some examples of artefacts are marked with white circles.
Download figure:
Standard image High-resolution imageOur findings indicate that, in the context of stitching polarimetric images, overwrite blending may prove more effective than both overlaying blending and seamless blending. We demonstrate this through a comparative analysis of Mueller matrices derived from stitched polarimetric data of two human fingers, processed through different stitching techniques. Both alpha blending techniques were observed to generate significant polarimetric artefacts, compromising the clarity of the resulting image. Remarkably, the individual fingers were discernible exclusively in the overwrite blending case. The significant artefact production associated with other blending techniques masks the true number of fingers present in the measurement, highlighting the superior performance of overwrite blending in this scenario.
Figure 18 presents the results of a polarimetric analysis following the implementation of a stitching process on a pattern drawn on human skin, specifically an arm. This pattern serves as an in vivo phantom, demonstrating the practical application and feasibility of the stitching method in a realistic context. The intricacy of the drawing provides an abundance of key points, facilitating the ease and efficiency of the stitching process. Additionally, figure 18 underscores the variable outcomes achieved when different stitching techniques are employed, showing that the choice of technique significantly influences the final result.
Figure 18. Comparison of the MM entries for different stitching techniques. The sample is a drawing on human skin and was measured in vivo. The whit circles exemplarily mark the polarimetric artefacts.
Download figure:
Standard image High-resolution imageThe application of stitching to in vivo polarimetric data is carried out on a phantom for human skin. The application of the polarimetric stitching method significantly expands the field of view and allows for a comprehensive analysis of large skin patches, which was previously not possible due to the inherent limitations of traditional polarimetric setups. The results of the in vivo measurements show the algorithm's effectiveness in the clinical setting, where patient motion is inevitable. During our examination procedure, the initial measurement obtains a particular FoV. With each additional measurement, we incorporate an additional area of about 37% of the original FoV, as depicted in figure 18. Thus, the total FoV grows substantially in the course of the five measurements.
In figures 13–18, we demonstrate that the Mueller matrices calculated for the overwrite images show far fewer polarimetric artefacts than with other overlaying and seamless blending. Therefore, overwrite blended raw data is ideal for these types of MMP applications.
4. Discussion
Stitching has an added advantage when dealing with motion artefacts. In in vivo polarimetric imaging, especially on human subjects, minor unintended movements are inevitable. By combining multiple overlapping images, the method can not only expand the FoV but also compensate for inconsistencies or misalignments introduced due to patient movement during the acquisition. In our studies, the magnitude of motion artefacts varied based on the specific scenarios and subjects under consideration. However, previous work included a detailed study with quantifications on motion artefacts in polarimetry, showing the improvement of the quantitative motion correction [23].
To ensure repeatability, we employed a data acquisition protocol. The initial step involved adjusting the position of the sample. We located and centered the sample within the FoV to ensure optimal imaging. To capture high-quality images with accurate details, we calibrated the exposure time specific to the sample's optical properties. This ensured that our images were neither overexposed nor underexposed. Upon optimizing the settings, the system was set to automatically acquire the set of 36 raw images needed to derive the MM. After the raw data acquisition, a voluntary motion either of the system or of the sample was initiated. We ensured that the FoVs have sufficient overlap and acquired another set of raw images.
Furthermore, stitching offers the potential to average out measurement errors or uncertainties present in individual measurements, leading to more accurate and consistent results. The accuracy of MM measurements as a polarization imaging technique is sensitive to the signal-to-noise ratio (SNR). Polarization imaging, being SNR-dependent, can be affected by various factors such as ambient light, speckle noise, camera noise, and calibration errors. In our system, we took several measures to mitigate such issues. The system was carefully calibrated according to the Compain method [17]. We employed a controlled environment with minimized ambient light interference and checked the calibration regularly with optical samples of known polarization (i.e., linear polarizers, waveplates, air and diffusers). The deviations are found to be well within the acceptable limits for the applications discussed in our study. Previously published data showed that our system handles the SNR challenges effectively [1]. Calibration errors arise from its non-perfect polarization properties and its non-optimal alignment to the setup.
The FOV of our system is approximately 3 cm by 2 cm. While this is sufficient for many applications, the limited FOV can sometimes be restrictive, especially when studying larger skin patches. Stitching allows for a more comprehensive and holistic view of the skin, enabling clinicians to compare the region of interest with surrounding benign areas, which aids in diagnosis. Certain skin conditions, such as large nevi, span a substantial area. Capturing the entire lesion with sufficient spatial resolution in a single shot is often challenging. Stitching offers a viable solution to this challenge.
A white light image-guided stitching method could potentially improve polarimetric analyses and address the effect of artefacts by aiding in more accurate alignment of the polarimetric images. The detailed resolution available in a white light image can provide a more comprehensive reference framework. White light image guidance might help in compensating for artefacts by providing a 'true' representation of the sample. This could assist in identifying and mitigating any inconsistencies or distortions introduced during the stitching of polarimetric images. However, there are some challenges and considerations. Integrating a white light image acquisition into the workflow will inevitably increase the total acquisition time. This could be a limitation in scenarios where time-efficiency is paramount. If a second camera is necessary, it introduces complexities related to calibration, synchronization, and additional costs. White light imaging, being a more 'native' representation of the sample, might offer a reference frame that could lead to more consistent stitching results and should be tested in future work.
5. Conclusion and outlook
In this work, we undertook an extensive investigation into the feasibility and effectiveness of image stitching techniques for expanding the field of view in Mueller Matrix Polarimetry. Our studies have shown that image stitching, particularly the overwrite technique, can serve as a powerful tool for generating comprehensive polarimetric images, significantly enhancing our ability to visualize and analyze large skin patches. Stitching is an important technique in polarimetry that can enable a wider range of applications. The creation of larger and more comprehensive, panoramic views of the skin provides a more immersive and intuitive experience for the dermatologist. This can be particularly useful in cases where it is necessary to study large patches of skin, such as in the case of inflammatory skin diseases or scars. Additionally, the development and application of advanced imaging technologies that can accommodate larger nevi are crucial. These should be able to capture comprehensive, high-resolution images of these larger skin lesions, ensuring that physicians have the complete picture when making a diagnosis.
Overwrite stitching appears to be a superior option for Mueller Matrix Polarimetry applications. The alpha blending techniques (fused and seamless), while potentially beneficial in certain contexts, were observed to introduce significant polarimetric artefacts. These artefacts can interfere with the accurate interpretation of images, potentially impeding diagnosis and treatment decisions in clinical settings. The overwrite blending method, on the other hand, preserved the essential characteristics of the Mueller matrix data, reducing such artefact-induced complexities.
The observed blurring effect, which seems to arise as a byproduct of the geometric transformation and warping processes, offers an interesting avenue for future research. While not intended to serve as a noise reduction measure, this effect seems to influence the noise levels within the stitched images by half. It is important to explore this phenomenon further to develop a more nuanced understanding of its implications.
We evaluated the presented algorithm's ability to stitch polarimetric data accurately under the presence of random patient motion. Through our experiments, we provide insights into the algorithm's robustness and practicality for in vivo measurements showing that the field of view can be expanded by about a third with each additional in vivo measurement.
In summary, our results validate the effectiveness of the proposed stitching technique for polarimetric data in the context of dermatology, showing its utility in improving the accuracy, robustness, and comprehensiveness of in vivo polarimetric measurements. Our technique enables the assessment of larger skin patches, and its robustness against patient movement makes it an ideal tool for practical, clinical applications.
Future work will continue to explore and optimize these techniques, with particular focus on handling real-world challenges such as patient motion and noise reduction. The advancements made in this field hold significant potential to transform our abilities to accurately diagnose and treat a range of skin conditions in clinical practice.
In the next stages, we intend to employ the polarimetry-based system for systematic tests in a clinical environment. Finally, it is worth noting that the proposed stitching technique showed promise not only for the diagnosis but also for the therapy monitoring of inflammatory skin conditions.
Acknowledgments
This work has been supported by iToBoS (Intelligent Total Body Scanner for Early Detection of Melanoma), project funded by the European Union's Horizon 2020 research and innovation programme, under grant agreement No 965221. Also, financial support by the Deutsche Forschungsgemeinschaft (DFG, German Research Foundation) under Germany's Excellence Strategy within the Cluster of Excellence PhoenixD (EXC 2122, Project ID 390833453) is acknowledged.
Data availability statement
The data cannot be made publicly available upon publication because no suitable repository exists for hosting data in this field of study. The data that support the findings of this study are available upon reasonable request from the authors.
Ethical statement
The measurements conducted on human skin in this study were performed exclusively on human volunteers (the authors). No external participants were involved. Additionally, the non-human biological samples used were sourced from products readily available at local supermarkets or obtained as slaughterhouse waste, ensuring no animals were harmed specifically for the purposes of this research. The non-biological phantoms used posed no ethical concerns.
Disclosures
The authors declare no conflict of interests.