Abstract
The development and experimental validation of a Machine Learning (ML) vibration based Structural Health Monitoring (SHM) prototype system for composite aerostructures operating under uncertainty is presented. Following initial training, it is intended to operate autonomously, in almost real-time, without interrupting normal operation, relying on a limited number of acceleration and strain measurements from properly selected locations on the aerostructure. It is equipped with a multi-level information fusion methodology comprising multiple sensor technologies and diagnostic algorithms for optimized SHM performance in terms of damage detection and characterization. Through its flexible software, the system provides multiple options of ML based SHM methods targeting wide applicability to various types of structures. The system is validated based on numerous experiments with healthy and damaged full-scale composite bonded spars operating under multiple uncertainty factors. Its diagnostic performance indicates that the current progress in hardware and robust vibration-based Machine Learning algorithms may lead to compact, effective, and low cost SHM systems for composite aerostructures under normal operating conditions.
Export citation and abstract BibTeX RIS
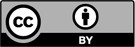
Content from this work may be used under the terms of the Creative Commons Attribution 3.0 licence. Any further distribution of this work must maintain attribution to the author(s) and the title of the work, journal citation and DOI.