Abstract
Constant exploration of better performance and energy consumption has led to several hardware-based generators being used in interdependent SoC. Such generators abuse the intrinsic contrast of activities, and they also forgive inconsistencies in their outputs, such as image and optical machine learning applications. At about the same time owing to process growing and control constraints, irreversible faults are escalating, leading to incorrect outputs. Strategy to resolve this issue, which uses machine learning training tools to improve the impact of permanent fix in hardware accelerators that can withstand incorrect outputs. The suggested financial benefits do not need any accelerator details and are highly flexible with low capital areas. Furthermore, earlier work is very time intensive as it involves the introduction of fault pairs into the gate netlists to test their effect on the outputs. To solve these problems, this paper proposed to improve representation first by increasing the degree of complexity from the degree of both the gate to a stage of actions. The power to make segments and sub of the same contextual definition with specific features by establishing numerous propagation commands in the supply chain context involves. Test data demonstrate that our proposed approach is a simple and reliable way of generating different designs to secure the device.
Export citation and abstract BibTeX RIS
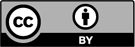
Content from this work may be used under the terms of the Creative Commons Attribution 3.0 licence. Any further distribution of this work must maintain attribution to the author(s) and the title of the work, journal citation and DOI.