Abstract
The infection of human papilloma virus (HPV) poses a global public health challenge, particularly in regions with limited access to health care and preventive measures, contributing to health disparities and increased disease burden. In this research work, we present a new model to explore the transmission dynamics of HPV infection, incorporating the impact of vaccination through the Atangana–Baleanu derivative. We establish the positivity and uniqueness of the solution for the proposed model HPV infection. The threshold parameter is determined through the next-generation matrix method, symbolized by \({\mathcal {R}}_0\). Moreover, we investigate the local asymptotic stability of the infection-free steady-state of the system. The existence of the solutions of the recommended model is determined through fixed-point theory. A numerical scheme is presented to visualize the dynamical behavior of the system with variation of input factors. We have shown the impact of input parameters on the dynamics of the system through numerical simulations. The findings of our investigation delineated the principal parameters exerting significant influence for the control and prevention of HPV infection.
Similar content being viewed by others
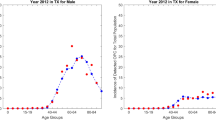
Introduction
Human papilloma virus is a group of related viruses that can infect the genital area, as well as the mouth and throat1. It is the most common sexually transmitted infection worldwide. This viral infection is primarily spread through intimate skin-to-skin contact, typically during sexual activity. Notably, there are various types of HPV, and some can cause genital warts, while others are linked to the development of cancers, including cervical, anal, penile, and oropharyngeal cancers2. In fact, HPV is a leading cause of cervical cancer in women. Preventive measures include vaccination, which is highly effective in protecting against the most common cancer-causing HPV strains. Regular screening for cervical cancer in women is also essential for early detection and treatment. Given the prevalence of HPV and its potential health implications, education about safe sexual practices, vaccination, and regular medical check-ups are crucial components of public health initiatives aimed at reducing the impact of HPV-related diseases. The HPV vaccine was developed to protect against this viral infection. Given in a series of doses, the vaccination dramatically reduces the incidence of HPV-related malignancies and genital warts3. Public health recommendations emphasize the necessity of routine vaccination for adolescents and young adults to improve health outcomes. There is no specific cure for HPV, the infections often clear on their own as the immune system fights the virus4. However, certain conditions caused by HPV, such as genital warts or abnormal cervical cells, can be treated. Always consult with a health care professional for personalized advice and treatment options based on your specific situation.
Mathematical models provide a quantitative framework for understanding complex systems and making predictions that can guide actions and policies5,6,7. These modeling typically involves defining variables, formulating equations, and using mathematical tools and techniques to interpret and understand complex phenomena, facilitating problem-solving and decision-making processes in a systematic and quantifiable manner8,9,10. Mathematical models have played a pivotal role in elucidating the dynamics of infectious disease transmission and devising efficacious interventions for disease control11,12,13. Numerous mathematical models have been formulated to conceptualize the key input factors of the dynamics of viral infection within populations14,15. Many scientific endeavors have focused on the mathematical modeling of the progression of cervical cancer in conjunction with HPV infection. The objective is to gain a comprehensive understanding of the disease and explore various approaches to managing its advancement or halting it altogether. Several mathematical models have employed a compartmental approach to study the transmission dynamics of HPV infection in the population16,17. After that, the researchers in18,19 conceptualized and studied the transmission phenomena of cervical cancer by incorporating real parameters from various geographical regions. In20, a system comprising five differential equations characterizing HPV, coupled with four compartments delineating epithelial cells healthy, infected, precancerous, and cancerous was delineated. The authors delve into examining the distinct stability of each equilibrium and scrutinize the conditions dictating disease extinction or persistence. Addressing the same issue, a recent study21 tackles optimal control by incorporating various incidence functions. The authors propose that the two treatments function by impeding new infections and diminishing the population of precancerous cells. In the present study, we formulate the dynamics of HPV to elucidate the influence of vaccination, asymptomatic carriers, and cervical cancer on the transmission pathway.
In21,22, the researchers introduced a saturated infection rate and two viral infection treatments into their model. Biologically, the saturated infection rate signifies the transmission rate of the infection, considering the viral density in the vicinity of the healthy epithelial cell23,24. Furthermore, the first treatment functions to gauge the efficacy of drug intervention in preventing new infections, while the second reflects the effectiveness of drug treatment in impeding viral production25. In epidemic models, a fractional framework holds significance as it offers a robust mechanism for integrating memory and hereditary property within systems26,27. Moreover, non-integer models have the capacity to depict the nonlocal dynamics of biological processes with greater accuracy compared to classical models28,29. Utilizing fractional operators in epidemic models yields more precise outcomes for real-world data than traditional integer models30,31,32. The complex biological processes can be accurately characterized through a fractional framework. Therefore, we choose to represent the dynamics of HPV infection within this fractional framework, aiming to offer a more precise depiction of the viral infection’s dynamics.
The structure of the paper unfolds as follows: Section “Theory of fractional-calculus” introduces the fundamental findings and concepts associated with the Atangana–Baleanu fractional operator. In Section “Fractional order model formulation”, we construct a mathematical model for HPV infection, considering vaccination and reinfection phenomena. The stability study is detailed in Section “Analysis of the model”, and Section “Fractional-order model solution” delves into the existence and uniqueness of the solution. Section “Fractional dynamics via Newton polynomial” showcases numerical iterative methods and simulation results. Finally, Section “Conclusion” provides a summary of the entire work.
Theory of fractional-calculus
In this section, we will unveil pivotal theory of the Atangana–Baleanu operator alongside the classical Caputo derivative, as elucidated in reference33. Additionally, we will delve into the Atangana–Baleanu operator, as outlined in reference33. These basic concepts and findings will be utilized in the analysis of the model.
Definition 2.1
33. Consider a function k such that \(k:[p,q]\rightarrow {\mathbb {R}}\), then the Caputo fractional derivative of order \(\upsilon\) on k can be stated pas
where \(r \in {\textbf{Z}}\) and \(\upsilon \in (r-1,r)\).
Definition 2.2
Suppose a function k such that \(k\in {\mathcal {H}}^1(p,q)\), \(q>p\), and \(\upsilon \in [0,1],\) then AB fractional operator in Caputo structure represented by ABC is defined as
Definition 2.3
Integral of AB derivative is represented by \({}^{{ABC}}_{p}I^{\upsilon }_{t}k(t)\) and defined as
Since the fractional-order \(\upsilon \rightarrow 0\) implies that the initial function can be attained.
Theorem 2.1
33. Consider a function k such as \(k \in C[p,q]\), then the following holds
Moreover, the Lipschitz holds for the ABC derivative as
Theorem 2.2
33. The following system of fractional differential equation
has a unique solution of the form
Fractional order model formulation
In this part, we present a mathematical model of HPV transmission. In formulation of the model, we represent the total population by \({\mathcal {N}}(t)\). According to their illness state, the model splits the whole population into six sub-classes: Susceptible \({\mathcal {S}}(t)\), Vaccinated \({\mathcal {V}}(t)\), Asymptomatic \({\mathcal {A}}(t)\), Infected \({\mathcal {I}}(t)\), Recovered \({\mathcal {R}}(t)\), and Cervical cancer \({\mathcal {C}}(t)\). Here, the following presumptions are used to build a mathematical model of the human papilloma virus. A portion \(p\) are vaccinated on the onset of an outbreak and a fraction \(\alpha\) of the susceptible are vaccinated. After vaccination a portion \(\varphi\) of vaccinated individuals moves to susceptible class after losing the effectiveness of the vaccination while the recovered individuals lose the immunity with a rate \(\omega\) and become susceptible. The susceptible population is at risk of infection, whether it is from asymptomatic or symptomatic individuals, and this risk is quantified by a force of infection denoted as \(\lambda =\frac{\beta [{\mathcal {I}} + \gamma {\mathcal {A}}]}{{\mathcal {N}}}\). Here, \(\beta\) is calculated as the product of \(\kappa\)(the contact rate) and \(\tau\)(the probability that a contact leads to infection), and \(\gamma\) represents the transmission coefficient for asymptomatic individuals. In cases where \(\gamma > 1\), asymptomatic individuals are more likely to infect susceptible individuals than symptomatic ones. When \(\gamma = 1\), both asymptomatic and symptomatic individuals have an equal chance of infecting the susceptible population. However, if \(\gamma < 1\), symptomatic individuals have a greater likelihood of infecting susceptible individuals compared to asymptomatic ones.
The HPV vaccine is considered to provide only temporary immunity, and individuals who have been vaccinated may still have a chance of being infectious or asymptomatic, although this likelihood is relatively low. The force of infection for the vaccinated population is denoted as \(\lambda _v = \varepsilon \lambda\), where \(\varepsilon\) falls within the range of 0 to 1. \(\varepsilon\) represents the proportion of the serotype not covered by the vaccine. Individuals acquiring new infections due to the force of infection face dual potential outcomes. They can either undergo asymptomatic infection, characterized by a probability denoted as \(\rho\), and subsequently join the asymptomatic class. Alternatively, there is a probability of \(1-\rho\) that they progress to the infected class. Within the asymptomatic class, individuals encounter two distinct trajectories. They may either manifest disease symptoms or opt for screening, triggering a transition into the infected class at a rate represented as \(\theta\). Alternatively, they may naturally recover, acquiring immunity at a rate denoted as \(\phi\). Individuals within the infected class undergo transitions based on treatment. At a rate of \(\eta\), some individuals move to the recovered compartment through effective treatment, where a proportion \(q\) successfully joins the recovered class. Others, constituting the remaining \((1- q )\) proportion, opt for an alternative treatment path, joining the asymptomatic class. Unfortunately, in cases where the treatment fails, individuals may progress to develop cervical cancer at a rate \(\delta\), leading to a shift into the cervical cancer compartment. Individuals who are afflicted with cervical cancer may face mortality due to the infection, occurring at a rate denoted as \(\xi\). Within all compartments, \(\mu\) represents the natural mortality rate of individuals. The flow chart of the transmission dynamics of HPV with the above assumptions is illustrated in Fig. 1. Then, the model of HPV with the above assumptions in the form of mathematical expression is as follows:
with appropriate initial condition
The initial conditions for the system of model (1) are all non-negative and represented as follows: \({\mathcal {S}}(0) = {\mathcal {S}}_0, {\mathcal {V}}(0) = {\mathcal {V}}_0, {\mathcal {A}}(0) = {\mathcal {A}}_0, {\mathcal {I}}(0) = {\mathcal {I}}_0, {\mathcal {R}}(0) = {\mathcal {R}}_0, {\mathcal {C}}(0) = {\mathcal {C}}_0\). The above model (1) in fractional form can written as:
where \(0< \upsilon \le 1.\) The adoption of fractional derivatives in epidemic modeling enhances the models’ ability to reflect the complexity of real-world scenarios, making them more effective tools for predicting and managing the spread of infectious diseases. The Atangana–Baleanu derivative is known for its ability to model non-local and non-singular behaviors, which may be crucial for accurately describing certain physical processes. The Atangana–Baleanu derivative provides a flexible mathematical framework that can be adapted to describe systems with memory and long-range dependencies. Its versatility makes it suitable for a wide range of applications.
Theorem 3.1
The solutions the system (3) of the disease are nonnegative and bounded for nonnegative initial vales of state variables of the system.
The solutions of our fractional system (3) of the disease is evidently constrained and remains nonnegative for nonnegative initial values of state variables. Consequently, the system is biologically valid. Further analysis of the model will be presented in the upcoming investigation of the system.
Analysis of the model
In this section of the, we will investigate our model of HPV for disease-free steady-state, reproduction number and local asymptotic stability. Let the disease-free steady-state is denoted by \({\mathcal {E}}_0\) and can be determined by taking the steady-state of system (3) without infection, then, we have
Here, we assume that the basic reproduction number is indicated by \({\mathcal {R}}_0\) which can be calculated through different technique. We take the following step to determined \({\mathcal {R}}_0\) of our model:
putting \(\lambda =\frac{\beta [{\mathcal {I}} + \gamma {\mathcal {A}}]}{{\mathcal {N}}}\), we have
Taking the Jacobian of the above, we have \({\mathcal {F}}\) and \({\mathcal {V}}\) as given below
in which \(q_1 = \frac{\varphi - \mu + \mu p}{\varphi + \mu }\), \(q_2 = \frac{ \mu p}{ \varphi + \mu }\), \(k_1 = q_1 + \varepsilon q_2\), \(k_2 = cd - \theta \eta (1 - q)\), \(k_3 = d\gamma + \theta\), \(k_4 = (1 - q)\eta \gamma + c\), \(a = ( \lambda + \mu )\), \(b = (\varphi + \epsilon \lambda + \mu )\), \(c = (\theta + \varphi + \mu )\), \(d = (\delta + \eta + \mu )\), \(e = (\omega + \mu )\) and \(f = (\xi + \mu )\). From the above, we have \({\mathcal {F}} {\mathcal {V}}^{-1}\) as
Here, assume that the greatest eigenvalue of \({\mathcal {F}} {\mathcal {V}}^{-1}\) is denoted by \(\rho ({\mathcal {F}} {\mathcal {V}}^{-1})\) which is \(\frac{\kappa \tau k_1 k_2 k_3\rho + (1 - \rho )k_4}{k_2}\) and the basic reproduction number through next-generation matrix method is the greatest eigenvalue of \({\mathcal {F}} {\mathcal {V}}^{-1}\), thus, we have
Theorem 4.1
If \({\mathcal {R}}_0<1\), then the steady-state \({\mathcal {E}}_0\) is locally asymptotically stable and is unstable in other cases.
Proof
For the required stability result, we take the the Jacobian matrix at \({\mathcal {E}}_0\) as
For the required result, we will show that all the eigenvalues of \({\mathcal {J}}(E_0)\) are negative. For which, we take the characteristic equation \(\det [{\mathcal {J}}(E_0) - \chi I] = 0\) as:
From the above, the first and second eigenvalue are \(-\mu\) and \(-f\) which are negative while the other eigenvalues can be determined from
here, we have the third and fourth eigenvalue are \(-b\) and \(-e\) which are negative. The remaining eigenvalues can be calculated from
Here, if \(det({\mathcal {J}}(E_1))<0\) and \(trc({\mathcal {J}}(E_1))>0\) for \({\mathcal {R}}_0<1\), then the disease-free steady-state of our model of HPV is locally asymptotically stable.
Fractional-order model solution
In this section of the manuscript, we will utilize fixed-point theory to confirm the uniqueness and existence of solutions of our model of the disease. The described system for HPV with the Atangana-Baleno derivative is provided as follows
this can be further expressed as follows:
In this context, we have the state variables represented by \(w(t)=({\mathcal {S}},{\mathcal {V}},{\mathcal {A}},{\mathcal {I}},{\mathcal {R}},{\mathcal {C}})\), and \({\mathcal {J}}\) is a continuous function. To clarify, the vector function \({\mathcal {J}}\) can be more clearly expressed as follows:
with appropriate initial conditions specified as \(w_0(t)=({\mathcal {S}}(0),{\mathcal {V}}(0),{\mathcal {A}}(0),{\mathcal {I}}(0),{\mathcal {R}}(0),{\mathcal {C}}(0))\), and furthermore, the function \({\mathcal {J}}\) satisfies the Lipschitz condition as outlined below:
Subsequently, we will explore the uniqueness and existence of system (4) in the following outcome.
Theorem 5.1
A unique solution for the suggested system (4) of HPV is present if the following condition is met
Proof
To establish the intended result, we apply the AB fractional integral (2.3) to the system (5) which provide the following
Consider the interval \((0, {\mathcal {T}})\) represented as I, and the operator \(\Lambda :{\mathcal {P}}(I,\textrm{R}^6)\rightarrow {\mathcal {P}}(I,\textrm{R}^6)\) is defined as follows:
Then, Eq. (8) can be written as
the supremum norm over the set I is represented by \(\Vert .\Vert _I\), and defined as
One can observe with patience that \({\mathcal {P}}(I,\textrm{R}^6)\) forms a Banach space equipped with the norm \(\Vert .\Vert _I\). Moreover, it is evident that
both w(t) and \({\mathcal {K}}(t,\varpi )\) are members of \({\mathcal {P}}(I,\textrm{R}^6)\) and \({\mathcal {P}}(I^2,\textrm{R})\), respectively, in a way that
Using the definition of \(\Lambda\) as outlined in (10), we obtain the following result
Furthermore, by making use of the Lipschitz condition (6) and the outcome from (12), the following is derived
As a result, we get the following
where
It is clear that if condition (7) holds, then \(\Lambda\) is a contraction. This, in turn, implies that the HPV system (4) possesses a unique solution.
Fractional dynamics via Newton polynomial
In this section of the paper, our focus is on the numerical solution of our system (4) of the infection. To do this, we consider the below stated Atangana–Baleanu derivative system
transform the previously stated equation into the subsequent form according to34:
the above at \(t_{r+1}=(r+1)\Delta t\) can be stated as
this can be further transformed into:
In the subsequent phase, we employ the Newton polynomial method to estimate f(t, g(t)) as follows
Utilizing the above stated polynomial in (20), we get that
Moreover, we get
the following result is achieved after simplification
the integrals above can be evaluated using the following method
where \(V_1=2(r-\imath )^2+(3\upsilon +10) (r-\imath )+2\upsilon ^2+9 \upsilon +12,\) and \(V_2=2(r-\imath )^2+(5\upsilon +10) (r-\imath )+6\upsilon ^2+18 \upsilon +12\). After simplification, we get that
We will employ the aforementioned approach to depict the time series of the proposed infection model. Time series analysis holds significant importance in comprehending, monitoring, and managing diseases. It furnishes valuable insights into the dynamics of the disease, aids in the early detection of outbreaks, and enables the assessment of intervention effectiveness. This, in turn, contributes to more informed and targeted public health initiatives. The numerical values of system parameters and state variables will be assumed for computational purposes. Various simulations will be conducted to illustrate how these parameters impact the infection system.
In the initial simulation, illustrated in Figs. 2 and 3, we scrutinized the impact of the fractional parameter \(\upsilon\) on the dynamics of HPV. In Fig. 2, we consider the values of \(\upsilon\) to be 1.00, 0.95, 0.90, and 0.85, while in Fig. 3, the value of \(\upsilon\) is varied as 0.80, 0.70, 0.60, and 0.50. This systematic exploration of diverse values for the input parameter \(\upsilon\) allows us to thoroughly investigate the characteristic solution pathways of the system. The outcomes of these simulations unequivocally highlight the substantial influence exerted by the fractional parameter on the dynamics of the infection. Notably, \(\upsilon\) emerges as a promising tool for effectively managing the spread of the infection within the community. Therefore, we strongly advocate for a more in-depth exploration and analysis of this fractional parameter by policymakers to enhance their understanding of its potential in mitigating the impact of the infection on public health. This comprehensive investigation can contribute valuable insights for developing targeted strategies in the control and prevention of the infection. Figure 4 depicts the impact of the input parameter \(\beta\) on the dynamics of HPV infection. In this simulation, we considered \(\beta\) values of 0.20, 0.40, 0.60, and 0.80. Our observations highlight the crucial role of this parameter, indicating a direct association with an increased risk of the infection.
In Figs. 5 and 6, we have illustrated the biological implications of varying input parameters \(\rho\) and \(\theta\) on the dynamics of HPV. In Fig. 5, we explored the effects of different values of \(\rho\) (0.45, 0.55, 0.65, and 0.75), while maintaining \(\theta\) at values of 0.2, 0.3, 0.4, and 0.5 in Fig. 6. Our investigation specifically focuses on discerning how changes in these parameters influence the behaviors of asymptomatic and infected individuals within the HPV system. In the conclusive simulation, depicted in Fig. 7, we investigated the impact of the input parameter \(\eta\) on the solution pathways of HPV infection. For this analysis, we considered values of \(\eta\) as 0.25, 0.30, 0.35, and 0.40. The observation centered on understanding how variations in \(\eta\) contribute to the dynamics of the asymptomatic and infected classes within the model. These insights hold significant relevance for informing public health strategies, intervention measures, and the formulation of effective control policies aimed at managing and mitigating the repercussions of infectious diseases on populations. Understanding the intricate relationships between input parameters and the dynamics of HPV infection is essential for the development of targeted and efficient approaches to tackle such public health challenges.
Conclusion
The infection HPV had posed a global public health challenge, especially in regions with limited access to health-care and preventive measures, contributing to health disparities and an increased disease burden. In our research, we structured a mathematical model for the transmission dynamics of HPV infection with the effect of vaccination, asymptomatic carrier and cervical cancer. We have shown that the solution of the recommended model are positive and bounded for positive initial values of state variables. We utilized the next-generation matrix method for the calculation of the basic reproduction number \({\mathcal {R}}_0\). In addition to this, we proved that the infection-free steady-state of the system are locally asymptotically stable for \({\mathcal {R}}_0<1\) and unstable in other cases. The existence of the solution has been investigated with the help of fixed-point theory. We introduced a numerical scheme to elucidate the dynamic behavior of the system, aiming to demonstrate the influence of the system’s input parameters. The most critical factors of the proposed system has been visualized and are recommended to the policy makers for the control and management of the infection. In the future research work, we will examine the impact of pulse vaccination on the dynamics of HPV infection. Additionally, we intend to incorporate the dynamics of HPV infection within a stochastic framework and conduct a comparative analysis of their respective outcomes.
Ethical approval
There is no ethical issue in this work. All the authors actively participated in this research and approved it for publication.
Data availability
The datasets used and analysed during the current study available from the corresponding author on reasonable request.
References
Rosalik, K., Tarney, C. & Han, J. Human papilloma virus vaccination. Viruses 13(6), 1091 (2021).
Brianti, P., De Flammineis, E. & Mercuri, S. R. Review of HPV-related diseases and cancers. New Microbiol. 40(2), 80–85 (2017).
Brianti, P., De Flammineis, E. & Mercuri, S. R. Review of HPV-related diseases and cancers. New Microbiol. 40(2), 80–85 (2017).
Hathaway, J. K. HPV: diagnosis, prevention, and treatment. Clin. Obstet. Gynecol. 55(3), 671–680 (2012).
Arnous, A. H. et al. Investigating solitary wave solutions with enhanced algebraic method for new extended Sakovich equations in fluid dynamics. Results Phys. 57, 107369 (2024).
Ahmad, I., Ali, I., Jan, R., Idris, S. A. & Mousa, M. Solutions of a three-dimensional multi-term fractional anomalous solute transport model for contamination in groundwater. PLoS ONE 18(12), e0294348 (2023).
Shah, K. & Abdeljawad, T. On complex fractal-fractional order mathematical modeling of CO\(_2\) emanations from energy sector. Phys. Scr. 99(1), 015226 (2023).
Thirthar, A. A., Majeed, S. J., Shah, K. & Abdeljawad, T. The dynamics of an aquatic ecological model with aggregation, fear and harvesting effects. AIMS Mathematics 7(10), 18532–18552 (2022).
Thirthar, A. A. A mathematical modelling of a plant-herbivore community with additional effects of food on the environment. Iraqi J. Sci. 64, 3551–3566 (2023).
Thirthar, A. A., Panja, P., Khan, A., Alqudah, M. A. & Abdeljawad, T. An ecosystem model with memory effect considering global warming phenomena and an exponential fear function. Fractals 31(10), 2340162. https://doi.org/10.1142/S0218348X2340162X (2023).
Tang, T. Q. et al. Analysis of the dynamics of a vector-borne infection with the effect of imperfect vaccination from a fractional perspective. Sci. Rep. 13(1), 14398 (2023).
Deebani, W., Jan, R., Shah, Z., Vrinceanu, N. & Racheriu, M. Modeling the transmission phenomena of water-borne disease with non-singular and non-local kernel. Comput. Methods Biomech. Biomed. Eng. 26(11), 1294–1307 (2023).
Jan, R., Boulaaras, S., Alyobi, S. & Jawad, M. Transmission dynamics of hand-foot-mouth disease with partial immunity through non-integer derivative. Int. J. Biomath. 16(06), 2250115 (2023).
Jan, R., Boulaaras, S., Alyobi, S., Rajagopal, K. & Jawad, M. Fractional dynamics of the transmission phenomena of dengue infection with vaccination. Discrete Contin. Dyn. Syst. S 16(8), 2096–2117 (2023).
Jan, A., Boulaaras, S., Abdullah, F. A. & Jan, R. Dynamical analysis, infections in plants, and preventive policies utilizing the theory of fractional calculus. Eur. Phys. J. Spec. Top. 232(14), 2497–2512 (2023).
Barnabas, R. V. et al. Epidemiology of HPV 16 and cervical cancer in Finland and the potential impact of vaccination: Mathematical modelling analyses. PLoS Med. 3(5), e138 (2006).
Myers, E. R., McCrory, D. C., Nanda, K., Bastian, L. & Matchar, D. B. Mathematical model for the natural history of human papillomavirus infection and cervical carcinogenesis. Am. J. Epidemiol. 151(12), 1158–1171 (2000).
Goldie, S. J., Goldhaber-Fiebert, J. D. & Garnett, G. P. Public health policy for cervical cancer prevention: The role of decision science, economic evaluation, and mathematical modeling. Vaccine 24, S155–S163 (2006).
Lee, S. L. & Tameru, A. M. A mathematical model of human papillomavirus (HPV) in the United States and its impact on cervical cancer. J. Cancer 3, 262 (2012).
Asih, T. S. N. et al. The dynamics of HPV infection and cervical cancer cells. Bull. Math. Biol. 78, 4–20 (2016).
Chakraborty, S., Cao, X., Bhattyacharya, S. & Roy, P. K. The role of HPV on cervical cancer with several functional response: A control based comparative study. Comput. Math. Model. 30, 439–453 (2019).
Asih, T. S. N., Aryati, L. & Kusumo, F. A. Cusp bifurcation on cervical cancer mathematical model. J. Phys. Conf. Ser. 1321(2), 022087 (2019).
Allali, K., Danane, J. & Kuang, Y. Global analysis for an HIV infection model with CTL immune response and infected cells in eclipse phase. Appl. Sci. 7(8), 861 (2017).
Sun, Q., Min, L. & Kuang, Y. Global stability of infection-free state and endemic infection state of a modified human immunodeficiency virus infection model. IET Syst. Biol. 9(3), 95–103 (2015).
Bratcher, L. F. & Sahasrabuddhe, V. V. RTehvieew impact of antiretroviral therapy on HPV andcervical intraepithelial neoplasia: Current evidence and directions for future research. Infect. Agents Cancer 5(1), 1–13 (2010).
Yadav, P., Jahan, S., Shah, K., Peter, O. J. & Abdeljawad, T. Fractional-order modelling and analysis of diabetes mellitus: Utilizing the Atangana–Baleanu Caputo (ABC) operator. Alex. Eng. J. 81, 200–209 (2023).
Khan, Z. A., Shah, K., Abdalla, B. & Abdeljawad, T. A numerical study of complex dynamics of a chemostat model under fractal-fractional derivative. Fractals 31(08), 2340181 (2023).
Sadek, L. et al. Fractional order modeling of predicting COVID-19 with isolation and vaccination strategies in Morocco. CMES-Comput. Model. Eng. Sci 136, 1931–1950 (2023).
Sinan, M. et al. Analysis of the mathematical model of cutaneous leishmaniasis disease. Alex. Eng. J. 72, 117–134 (2023).
Jan, R. et al. Fractional perspective evaluation of chikungunya infection with saturated incidence functions. Alex. Eng. J. 83, 35–42 (2023).
Jan, R. et al. Optimization of the fractional-order parameter with the error analysis for human immunodeficiency virus under Caputo operator. Discrete Contin. Dyn. Syst. S 16, 2118–2140 (2023).
Shah, Z., Bonyah, E., Alzahrani, E., Jan, R. & Aedh Alreshidi, N. Chaotic phenomena and oscillations in dynamical behaviour of financial system via fractional calculus. Complexity 2022, 1–14 (2022).
Atangana, A. & Baleanu, D. New fractional derivatives with nonlocal and non-singular kernel: Theory and application to heat transfer model. Therm. Sci. 20, 763–769 (2016).
Toufik, M. & Atangana, A. New numerical approximation of fractional derivative with non-local and non-singular kernel: Application to chaotic models. Eur. Phys. J. Plus 132, 444 (2017).
Funding
No funding is associated with the current research work.
Author information
Authors and Affiliations
Contributions
M.C.B. and S.B. conceptualized, formulated, validated the model and performed the analysis. R.J. and S.B. supervised, investigated and find out numerical results of the work. H.A. and R.F. analyzed, validated, conceptualized the model and wrote the initial draft. All the authors revised and approved the final draft of the work.
Corresponding author
Ethics declarations
Competing interests
The authors declare no competing interests.
Additional information
Publisher's note
Springer Nature remains neutral with regard to jurisdictional claims in published maps and institutional affiliations.
Rights and permissions
Open Access This article is licensed under a Creative Commons Attribution 4.0 International License, which permits use, sharing, adaptation, distribution and reproduction in any medium or format, as long as you give appropriate credit to the original author(s) and the source, provide a link to the Creative Commons licence, and indicate if changes were made. The images or other third party material in this article are included in the article’s Creative Commons licence, unless indicated otherwise in a credit line to the material. If material is not included in the article’s Creative Commons licence and your intended use is not permitted by statutory regulation or exceeds the permitted use, you will need to obtain permission directly from the copyright holder. To view a copy of this licence, visit http://creativecommons.org/licenses/by/4.0/.
About this article
Cite this article
Bahi, M.C., Bahramand, S., Jan, R. et al. Fractional view analysis of sexual transmitted human papilloma virus infection for public health. Sci Rep 14, 3048 (2024). https://doi.org/10.1038/s41598-024-53696-8
Received:
Accepted:
Published:
DOI: https://doi.org/10.1038/s41598-024-53696-8
Comments
By submitting a comment you agree to abide by our Terms and Community Guidelines. If you find something abusive or that does not comply with our terms or guidelines please flag it as inappropriate.