Abstract
The latest research progress of the theory of compressive sensing (CS) over graphs makes it possible that the advantage of CS can be utilized by data ferries to gather data for wireless sensor networks. In this paper, we leverage the non-uniform distribution of the sensing data field to significantly reduce the required number of data ferries, yet ensuring the recovered data quality. Specially, we propose an intelligent compressive data gathering scheme consisting of an efficient stopping criterion and a novel learning strategy. The proposed stopping criterion is based only on the gathered data, without relying on the priori knowledge on the sparsity of unknown sensing data. Our learning strategy minimizes the number of data ferries while guaranteeing the data quality by learning the statistical distribution of the gathered data. Simulation results show that the proposed scheme improves the reconstruction accuracy and stability compared to the existing ones.











Similar content being viewed by others
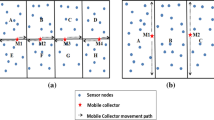
References
Akyildiz, I. F., Su, Y. S. W., & Cayirci, E. (2002). A survey on sensor networks. IEEE Communication Magazine, 40(8), 102–114.
Culler, D., Estrin, D., & Srivastava, M. (2004). Overview of sensor networks. IEEE Computer Society, 37(8), 41–49.
Rajagopalan, R., & Varshney, P. K. (2006). Data aggregation techniques in sensor networks: A survey. IEEE Communications Surveys and Tutorials, 8(4), 48–63.
Zhang, H., & Shen, H. (2009). Balancing energy consumption to maximize network lifetime in data-gathering sensor networks. IEEE Transactions on Parallel and Distributed Systems, 20(10), 1526–1539.
Jiang, H., Jin, S., & Wang, C. (2011). Prediction or not? An energy-efficient framework for clustering-based data collection in wireless sensor networks. IEEE Transactions on Parallel and Distributed Systems, 22(6), 1064–1071.
Tang, X., & Xu, J. (2008). Optimizing lifetime for continuous data aggregation with precision guarantees in wireless sensor networks. IEEE Transactions on networking, 16(4), 904–917.
Cands, E., & Tao, T. (2006). Near-optimal signal recovery from random projections: Universal encoding strategies? IEEE Transaction on Information Theory, 52(12), 5406–5425.
Donoho, D. L. (2006). Compressed sensing. IEEE Transaction on Information Theory, 52(4), 1289–1306.
Xu, X., Ansari, R., Khorkhar, A., & Vasilakos, A. V. (2015). Hierarchical data aggregation using compressive sensing(HDACS). ACM Transaction on Sensor Networks, 11(3), Article 45.
Xu, X., Ansari, R., & Khorkhar, A. (2014). Adaptive hierarchical data aggregation using compressive sensing (A-HDACS) for non-smooth data field. In Proceedings of IEEE ICC.
Fazel, F., Fazel, M., & Stojanovic, M. (2011). Random access compressed sensing for energy-efficient underwater sensor networks. IEEE Journal on Selected Areas in Communications, 29(8), 1660–1670.
Wang, J., Tang, S., Yin, B., & Li, X.-Y. (2012). Data gathering in wireless sensor networks through intelligent compressive sensing. In Proceedings of IEEE INFOCOM.
Zheng, H., Xiao, S., Wang, X., & Tian, X. (2012). Energy and latency analysis for in-network computation with compressive sensing in wireless sensor networks. In Proceedings of IEEE INFOCOM.
Sartipi, M., & Fletcher, R. (2011). Energy-efficient data acquisition in wireless sensor networks using compressed sensing. In Proceedings of IEEE Data Compression Conference (DCC) (pp. 223–232).
Sugihara, R., & Gupta, R. K. (2010). Speed control and scheduling of data mules in sensor networks. ACM Transactions on Sensor Networks, 7(1), Article 4.
Sugihara, R., & Gupta, R. K. (2010). Path planning of data mules in sensor networks. ACM Transactions on Sensor Networks, 8(1), Article 1.
He, L., Pan, J., & Xu, J. (2013). Progressive approach to reducing data collection latency in wireless sensor networks with mobile elements. IEEE Transactions on Mobile Computing, 12(7), 1308–1320.
Chen, L., Wang, W., Huang, H., & Lin, S. (2015). Time-constrained data harvesting in WSNs: Theoretical foundation and algorithm design. In Proceedings of IEEE INFOCOM.
Qi, H., Xu, Y., & Wang, X. (2003). Mobile agent based collaborative signal and information processing in sensor networks. Proceedings of the IEEE, 91(8), 1172–1183.
Wang, M., Xu, W., Mallada, E., & Tang, A. (2015). Sparse recovery with graph constraints. IEEE Transactions on Information Theory, 61(2), 1028–1044.
Wang, M., Xu, W., Mallada, E., & Tang, A. (2012). Sparse recovery with graph constraints: Fundamental limits and measurement construction. In Proceedings of IEEE INFOCOM (pp. 1871–1879).
Xu, W., Mallada, E., & Tang, A. (2011). Compressive sensing over graphs. In Proceedings of IEEE INFOCOM (pp. 2087–2095).
Brabham, D. C. (2008). Crowdsourcing as a model for problem solving: An introduction and cases. Convergence: The International Journal of Research into New Media Technologies, 14(1), 75C90.
Luo, C., Wu, F. Sun, J., & Chen, C. W. (2009). Compressive data gathering for large-scale wireless sensor networks. In Proceedings of ACM MobiCom (pp. 145–156).
Luo, C., Wu, F., Sun, J., & Chen, C. W. (2010). Efficient measurement generation and pervasive sparsity for compressive data gathering. IEEE Transaction on Wireless Communication, 9(12), 3728–3738.
Luo, J., Xiang, L., & Rosenberg, C. (2010). Does compressed sensing improve the throughput of wireless sensor networks? In Proceedings of the IEEE ICC, no. 1–6. Cape Town, South Africa.
Xiang, L., Luo, J., & Vasilakos, A. (2011). Compressed data aggregation for energy efficient wireless sensor networks. In Proceedings of IEEE SECON.
Malioutov, D. M., Sanghavi, S. R., & Willsky, A. S. (2010). Sequential compressed sensing. IEEE Journal of Selected Topics in Signal Processing, 4(2), 435–444.
Zheng, H., Yang, F., Tian, X., Gan, X., Wang, X., & Xiao, S. (2015). Data gathering with compressive sensing in wireless sensor networks: A random walk based approach. IEEE Transactions on Parallel and Distributed Systems, 26(1), 35.
Figueiredo, M., Nowak, R., & Wright, S. (2007). Gradient projection for sparse reconstruction: Application to compressed sensing and other inverse problems. IEEE Journal of Selected Topics in Signal Processing, 1(4), 586C598.
Kim, S. J., Koh, K., Lustig, M., Boyd, S., & Gorinevsky, D. (2007). A method for large-scale l1-regularized least squares. IEEE Journal of Selected Topics in Signal Processing, 1(6), 606–617.
Daubechies, I., De Friese, M., & De Mol, C. (2004). An iterative thresholding algorithm for linear inverse problems with a sparsity constraint. Communications on Pure and Applied Mathematics, 57, 1413–1457.
Shannon, C. E. (2001). A mathematical theory of communication. ACM SIGMOBILE Mobile Computing and Communications Review, 5(1), 3–55.
Chen, S., Donoho, D. L., & Saunders, M. A. (1999). Atomic Decomposition by basis pursuit. Siam Journal on Scientific computing, 20(l), 33–61.
National Oceanic and Atmospheric Administration is National Data Buoy Center. http://tao.ndbc.noaa.gov/refreshed/ctd_delivery.php.
Liu, Y., He, Y., Li, M., et al. (2013). Does wireless sensor network scale? A measurement study on GreenOrbs. IEEE Transactions on Parallel and Distributed Systems, 24(10), 1983–1993.
Acknowledgements
Funding was provided by CERNET Innovation Project (NGII20160323).
Author information
Authors and Affiliations
Corresponding author
Rights and permissions
About this article
Cite this article
Zhou, S., Zhong, Q., Ou, B. et al. Data ferries based compressive data gathering for wireless sensor networks. Wireless Netw 25, 675–687 (2019). https://doi.org/10.1007/s11276-017-1584-0
Published:
Issue Date:
DOI: https://doi.org/10.1007/s11276-017-1584-0