Abstract
Emerging infectious diseases are increasingly recognized in species’ declines and extinctions. Landscape genetics can be used as a tool to predict disease emergence and spread. The Tasmanian devil is threatened with extinction by a nearly 100% fatal transmissible cancer, which has spread across 95% of the species’ geographic range in 20 years. Here, we present a landscape genetic analysis in the last remaining uninfected parts of the Tasmanian devil’s geographic range to: describe population genetic structure, characterize genetic diversity, and test the influence of landscape variables on Tasmanian devil gene flow to assess the potential for disease spread. In contrast to previous genetic studies on Tasmanian devils showing evidence for two genetic populations island-wide, our genetic based assignment tests and spatial principal components analyses suggest at least two, and possibly three, populations in a study area that is approximately 15% of the size of the overall species’ geographic range. Positive spatial autocorrelation declined at about 40 km, in contrast to 80 km in eastern populations, highlighting the need for range-wide genetic studies. Strong genetic structure was found between devils in the northern part of the study area and those found south of Macquarie Harbor, with weaker structure found between the northeastern and northwestern portion of our study area. Consistent with previous work, we found low overall genetic diversity, likely owing to a combination of founder effects and extreme weather events thousands of years ago that likely caused large-scale population declines. We also found possible signs of recent bottlenecks, perhaps resulting from forest clearing for dairy farming in the central part of the study area. This human disturbance also may have contributed to weak genetic structuring detected between the northeastern and northwestern part of the study area. Individual-based least cost path modeling showed limited influence of landscape variables on gene flow, with weak effects of variation in elevation in the northeast. In the northwest, however, landscape genetic models did not perform better than the null isolation-by-distance model. At the larger spatial scale of the northern part of the study area, elevation and temperatures were negatively correlated with gene flow, consistent with low dispersal suitability of higher elevation habitats that have lower temperatures and dense, wet vegetation. Overall, Tasmanian devils are a highly vagile species for which dispersal and gene flow appear to be influenced little by landscape features, and spread of devil facial tumor disease to the remaining portion of the devil’s geographic range seems imminent. Nonetheless, strong genetic structure found between the northern and southern portions of our study area, combined with low densities and limited possible colonization of DFTD from the east suggest there is some time for implementation of management strategies.
Similar content being viewed by others
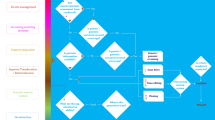
Avoid common mistakes on your manuscript.
Introduction
Predicting the emergence and spread of infectious diseases is a major challenge in ecology, evolutionary biology and conservation (Smith et al. 2006; Altizer et al. 2013). For humans, models have had some success in predicting the spread of particular diseases, such as measles (Riley 2007; Hempel and Earn 2015) and particular influenza strains to guide annual vaccine development (Shaman and Karspeck 2012). However, predicting disease transmission for populations of wild animals is far more challenging owing to the long-term data necessary to track and predict animal movements (Biek and Real 2010). Particularly for highly vagile mammals, understanding movement patterns also requires an understanding of how landscape features influence dispersal dynamics (Storfer et al. 2010; Short Bull et al. 2011; Mager et al. 2014).
Landscape genetics has become a valuable framework for understanding the ecological processes that influence population genetic structure in natural populations (Storfer et al. 2010; Manel and Holderegger 2013). Landscape genetic studies have proven valuable for generating predictions as to how landscape features may limit or enhance disease spread (Biek and Real 2010). When disease transmission is directly attributable to contact between hosts, as opposed to abiotic (e.g., wind, water) dispersal mechanisms, or biotic vectors (e.g. mosquitos), then associated landscape genetics studies should focus on spatial genetic variation in the host (Biek and Real 2010; Blanchong et al. 2016). Landscape genetics can be a powerful tool for detecting barriers to host dispersal (Crida and Manel 2007; Storfer et al. 2007, 2010; Manel et al. 2007), and by extension possible pathogen transmission across the landscape. For example, Blanchong et al. (2008) found that highways and rivers act as dispersal barriers for white-tailed deer populations in Wisconsin and thereby limit the spread of chronic wasting disease. Accordingly, higher genetic differentiation among deer translated to lower disease risk (Blanchong et al. 2008). In a similar study, Cullingham et al. (2009) found that one river acts as a barrier to raccoon movement and thereby limits rabies spread between the US and Canada, while a second river facilitates raccoon movement and consequently enhances rabies spread. This study highlighted the importance for assessing regional, in addition to local, impacts of landscape features, as the same feature that was a dispersal barrier in one area was a facilitator in another.
Furthermore, areas or habitat types that facilitate dispersal and consequent disease spread can be identified, potentially leading to focused management strategies. As an example, a landscape genetics approach was used to test models of rabies spread in Quebec in two different carrier species (Paquette et al. 2014), raccoons and skunks. Genetic analyses showed that movement was sex biased in raccoons; male movement was a function of isolation-by-distance, whereas females were restricted by the presence of agricultural fields. In contrast, movement of skunks tended to increase in edge habitats between forests and fields, regardless of sex (Paquette et al. 2014). For both species, particular corridors of high movement were identified, potentially targets for management strategies such as focused baiting.
Conversely, landscape genetics studies can show disease spread regardless of host genetic structuring. For example, one study showed that anthropogenic habitat modifications such as highways resulted in significant genetic structuring of bobcat (Lynx rufus) populations (Lee et al. 2012). Yet, despite genetic structuring, there was still sufficient contact of bobcats such that FIV transmission occurred throughout the study area and generally showed a lack of spatial genetic structure (Lee et al. 2012). Collectively, the varying results from these studies demonstrate the utility of a landscape genetics approach for studying disease spread and development of consequent management strategies.
The iconic Tasmanian devil (Sarcophilus harrisii) has gained worldwide attention because the species is declining dramatically owing to the rapid spread of a fatal infectious cancer (Devil facial tumor disease or DFTD; McCallum and Jones 2006; McCallum et al. 2009). The disease is spread by biting, which occurs during agonistic encounters when devils aggregate at carcasses and most frequently during male—male contests and mate guarding in the mating season (Hamede et al. 2013). Additionally, devils are capable of moving great distances and the geographic extent of positive spatial genetic correlation is large (Jones et al. 2004; Lachish et al. 2011). Genetic evidence suggests that devils experienced extensive population declines across Tasmania around the last glacial maximum (~20,000 YBP) and following unstable climate related to increased El Niño–Southern Oscillation activity approximately 3,000 years ago (Brüniche-Olson et al. 2014). Warming since the LGM resulted in ice melting and consequent sea level rise, isolating the devil population on Tasmania and perhaps enhancing their apparent universal susceptibility to DFTD owing to reduced genetic diversity (Miller et al. 2011).
Originating in northeastern Tasmania in 1996, DFTD has spread south and west across roughly 95% of Tasmania, resulting in population losses exceeding 90% in most localities (McCallum et al. 2009; Lachish et al. 2010; Hamede et al. 2013). At present, only devils in relatively small areas in northwestern and southwestern Tasmania remain uninfected (Fig. 1), and it is critical to assess how rapidly DFTD may spread through this region. Previous work suggests that there are two genetic clusters of devils across Tasmania, with the west separated from the east (Jones et al. 2004; Miller et al. 2011). However, because such coarse-scale (i.e., island-wide) genetic studies have only been conducted to date, the effects of landscape features on devil dispersal and gene flow are currently unknown. A landscape genetic study will thereby allow an assessment of whether landscape features impede or facilitate gene flow between the current disease front and the last remaining disease-free area of Tasmania. Assessments of genetic diversity in these individuals will also provide baseline information prior to disease emergence, as well as a test of whether or not these devils are genetically bottlenecked and potentially highly susceptible to DFTD. In fact, prior work suggests that devils in northwestern Tasmania are more genetically diverse than those in the east (Miller et al. 2011), and have greater MHC Class I diversity, which is responsible for presentation of tumor antigens to proliferating T-cells (Siddle et al. 2007, 2010). Thus far, however, there is no evidence that MHC diversity is related to resistance to DFTD (Lane et al. 2012).
Map of study area and individual sampling locations. a Tasmania with the study area outlined in black. The approximate location of the DFTD front at several time points is shown in red, and the area of W Tasmania with extremely low devil densities (Hawkins et al. 2006) is shown with a dashed outline and light blue shading. b Individual Tasmanian devil samples, color-coded by their Geneland population assignments. The blue color on the map indicates water bodies and the yellow–brown color denotes agricultural land. The two circled individuals indicate those for which the Geneland assignment was inconsistent with the results of a PCA (see Fig. 2A). (Color figure online)
Results of Adegenet PCA analyses. a First two principal components from standard PCA, color-coded by Geneland population assignment (Northeastern, Northwestern, and Southern populations). Two Southern individuals with similar loadings to the northern populations and one Northeastern individual with similar loadings to the Southern individuals are also marked on Fig. 1. b Map of samples, colored by the value of the first PCA from the sPCA analysis. c Distribution of values for the first PC from the sPCA analysis. (Color figure online)
At present, DFTD is spreading across the northeastern part of our study area (see Fig. 1). Here, we conducted a fine scale, individual-based landscape genetics study of Tasmanian devils of this northeastern and the remaining disease-free areas of northwestern of Tasmania using samples that were collected prior to the disease outbreak. We aimed to: (1) estimate fine-scale population genetic structure and genetic diversity using individual-based analyses; (2) assess which, if any, of 12 landscape variables previously identified as important to Tasmanian devil dispersal influences gene flow and population genetic structure in this area; and (3) use this information to infer the potential for DFTD to spread through the study area and thus to the western coast of Tasmania.
Methods
Sampling and microsatellite data
Tasmanian devils were sampled between 2002 and 2006 in NW Tasmania. Samples from the northwestern and Pieman River areas (Fig. 1) were collected as part of an intake of founders from the wild to establish a captive insurance population in the event reintroductions may be needed due to DFTD declines. The sampling pattern was intended to maximize geographic spread and genetic diversity within the area of Tasmania that was still disease-free at the time of sampling. The southern samples from south of Macquarie Harbor were collected during surveys to establish the abundance and disease status of devils in this remote area. There are large spatial gaps in the sampling between northwestern region of our study area and Macquarie Harbor because devil densities are quite low (Fig. 1a), and these are wilderness areas with no vehicular access. Although the DFTD has been progressing westward through Tasmania, it is just entering the eastern portion of our study area at present, so the sampling design herein is still relevant for assessing propensity for disease spread through the sampled area.
A total of 433 tissue samples were collected across an approximately 250,000 km2 area (Fig. 1b shows the origin and spread of DFTD by year across Tasmania) by taking 2 mm ear biopsies, and DNA was extracted using the HotSHOT salt extraction method (Truett et al. 2000). Because individuals were often trapped quite close together, we retained one individual per 100 m x 100 m meter GIS grid cell (the scale of our GIS layer resolution) for further analyses, resulting in a total of 276 individuals. We amplified ten microsatellite loci previously developed for S. harrisii (Jones et al. 2003), and then submitted the products to be run on an ABI 3730 automated sequencer (Applied Biosystems, Inc., Foster City, CA) at the Washington State University LBB1 core facility. We used GeneMapper 3.7 software to genotype all samples; genotypes were called based on peaks that passed process quality values (PCV >0.75) and were verified with visual inspection. Individuals with fewer than seven scorable microsatellites were discarded from the analyses.
To explore the basic properties of our microsatellite loci and ensure they meet the assumptions of population genetic analyses, we used Genepop (v4.2.1; Rousset 2008). We tested for linkage disequilibrium (LD), and for departure from Hardy–Weinberg equilibrium within each sub-population (later identified by Geneland; Guillot et al. 2008). In addition, we used Micro-checker (van Oosterhout et al. 2004) to test for the presence of null alleles. Although there was evidence for null alleles in only a few locus/population combinations, we calculated FST using the null allele correction in FreeNA (Chapuis and Estoup 2007).
Population structure and genetic diversity
All analyses were conducted between individuals, as opposed to a priori population level clustering, due to the large dispersal distances and extent of gene flow previously observed in Tasmanian devils (Lachish et al. 2011; Miller et al. 2011). To identify the number of genetic clusters in our data set, five separate Geneland (v4.04; Guillot et al. 2008) runs were conducted with 5,000,000 iterations each on all 276 individuals with GPS locality data. Considered individually, all runs detected three subpopulations, so we ran Geneland again on each of these subpopulations, but we detected no further population structure. Combining all five chains, the posterior distribution is three populations 59% of the time, four populations 21% of the time, more than four 12% of the time, and never fewer than three populations. Further, we conducted a principal components analysis (PCA) and spatial PCA (sPCA) using the R (R Core Team 2015) package adegenet (Jombart 2008). Unlike PCA, sPCA finds linear combinations of alleles that maximize the product of the genetic variation and spatial autocorrelation (Jombart 2008).
To further explore population structure and demographic history, we conducted three additional analyses on all three subpopulations identified by Geneland. First, we used Genepop to generate estimates of genetic diversity, including F-statistics. Second, to determine genetic neighborhood size, we calculated Moran’s I (Hardy and Vekemans 1999)—a measure of spatial autocorrelation using SPAGeDi (v1.4; Hardy and Vekemans 2002). We repeated the analysis after splitting the dataset by sex to account for any effects of sex-biased dispersal (see Lachish et al. 2011). Given the fact that there still could be genetic structuring among devils despite extensive disease dispersal (e.g., Lee et al. 2012), estimates of spatial autocorrelation could capture potential rare long-distance dispersal events not detected using other analysis methods. Third, we tested for signatures of a population bottleneck using the Wilcoxon sign rank test for heterozygote excess in bottleneck (Piry et al. 1999) with a two-phase mutation model and default settings. Because this may be sensitive to false homozygosity, we ran this test both with and without the loci that have null alleles. We also used the software Bottleneck to test for shifted allele frequency distributions, which can be caused due to disproportionate effects of genetic drift on rare alleles during recent bottleneck events (Cornuet and Luikart 1996; Luikart et al. 1998).
Landscape genetic analysis
Considering that Geneland provided support for two genetic clusters in NE and NW respectively, whereas sPCA analyses showed little substructure between these two regions, we conducted landscape genetic analyses on all individuals in the northern portion of the study area combined, as well as separately in the NE and NW areas to determine whether analyses at different spatial scale yielded different results. Individuals south of Macquarie Harbor were excluded from our analyses due to small population size. Throughout the study area, we examined seven continuous and four categorical environmental variables (at a pixel size of 100 × 100 m2; Supplementary Table 1). The continuous variables included compound topographic index (CTI—a steady state wetness index that is commonly used to quantify topographic control on hydrological processes), elevation relief ratio (ERR—a measure of the extent of altitudinal relief between two points), maximum annual temperature, minimum annual temperature, annual rainfall, heat-load index (HLI—which estimates heat load on the substrate as a combination of solar radiation and slope), and elevation. These variables were chosen based on assessment of Tasmanian devil movement patterns using mark-recapture studies (Lachish et al. 2008, 2011) and radiocollaring studies (Jones unpubl. data). We used a window size of 15 pixels for ERR, after comparing models with 3, 15, or 27 pixels. For CTI, HLI, and temperature, we transformed the data by subtracting the raw value from the maximum; for all other continuous variables, we used the raw values. The categorical variables included were road type, vegetation type, large rivers and iron ore pipelines (see Supplementary Table 1). Dispersal/movement costs for the different categorical variables were assigned using expert judgment and based on data from radio-collaring studies of localized individual dispersal (M. Jones, unpubl. data). Pipelines were assigned ten times the cost of non-pipeline pixels; pipeline costs were chosen based on radiocollaring studies that showed strong aversion of Tasmanian devils to movement via pipelines. Paved roads, non-road, and unpaved roads were assigned costs of ten, five, and one, respectively; paved roads are considered extremely costly for devil movement due to the large numbers of road kills observed island-wide each year. Eucalypt forest, rainforest, scrub, and heathland were assigned a cost of one; agriculture and grassland were assigned a cost of five; and all other vegetation cover types were assigned a cost of ten. These costs were chosen because devils strongly prefer movement through forest and scrub, with lower preference for movement through grassland and even less movement through other habitat types, such as developed areas. These costs were based on extensive mark-recapture studies showing devil trapping in areas other than forest resulted in extremely low capture rates (Hamede et al. 2009, 2012, 2013). Cost-distance values were calculated using UNICOR (Landguth et al. 2011), which implements Dijkstra’s shortest path algorithm between all pair-wise individuals for each landscape layer. Genetic distances between individuals were calculated as the proportion of shared alleles (DPS) (Bowcock et al. 1994). We created a null model of isolation-by-distance by calculating Euclidean distance between individuals.
To determine the environmental variables most closely associated with gene flow, we fit linear mixed models with maximum-likelihood population effects (MLPE; Clarke et al. 2002) with all combinations of landscape variables as fixed effects. All possible combinations of landscape variables were considered in models, and those models with lowest AICc values were retained. As Geneland detected three populations (Southern: 20 individuals, Northeastern: 109 individuals, and Northwestern: 147 individuals), models were fit for the NE and NW populations separately. The analysis was conducted in R (v2.14; R Core Team 2014) using the MuMIn (Bartón 2015), and lme4 (Bates et al. 2014) packages, following the method described by van Strien et al. (2012). We calculated an R2 value for the top model using the method proposed by Nakagawa and Schielzeth (2013; see also http://mbjoseph.github.io/blog/2013/08/22/r2/). All variables were standardized (subtracted mean, divided by standard deviation) before fitting the model, and models were chosen by Akaike Information Criterion corrected for finite sample size score (AICc; MuMIn package).
Results
Basic microsatellite results
There was no significant linkage disequilibrium or departure from Hardy–Weinberg equilibrium in any of the microsatellite loci after a Bonferonni correction for multiple tests. Micro-checker indicated the presence of null alleles in some populations (at the 95% confidence level). However, no locus had null alleles consistently across all three populations (as estimated by Geneland, below), and estimated null allele frequencies were low (<0.1 for the southern population and <0.05 for both northern populations where we conducted the landscape genetics analysis). Thus, we retained all loci for further analyses, except for the Bottleneck test, which may be especially sensitive to increased levels of homozygosity because it searches for excess heterozygosity (Piry et al.1999).
Population structure and genetic diversity
We identified three populations consistently across five runs in Geneland, corresponding to the southern, northwestern, and northeastern portions of the sampling localities (Fig. 1). Additional Geneland runs within the populations individually revealed no further substructure. A standard PCA analysis of the first and second principal components (explaining 14 and 7.5% of the variance, respectively), also showed clear genetic structuring between the S and NE and NW populations, as well as some genetic separation between NE and NW, albeit less than that found with Geneland (Fig. 2a). The sPCA analysis identified one principal component that explained almost all of the variation (Supplemental Fig. 1). This component clearly separated the northern populations from the southern, and there was evidence for subdivision of the northern samples into two populations (Fig. 2b, c). Thus, our analyses indicated that there are clearly at least two, and most likely three, genetic clusters in our study area.
When considering three clusters, mean pairwise FST was low between the NE and the NW (0.021 [0.012–0.034; 95% CI]), yet high between the NE and the S (0.26 [0.143–0.364]) and the NW and the S (0.31 [0.178–0.455]). FIS was similar among the three populations (NW: 0.056 [0.022–0.086]; NE: 0.037 [0.004–0.073]; S: 0.022 [−0.093 to 0.195]; Tables 1, 2, 3). Genetic neighborhood size extended to approximately 40 km, as indicated by positive spatial correlation values in Moran’sI at this spatial scale (Fig. 3). Spatial autocorrelation distances decayed at approximately 40 km for both males and females, indicating no sex-bias in dispersal. Finally, using Bottleneck, we found significant evidence of heterozygote excess only in the northeastern sub-population (one-sided p = 0.039), with weak evidence in the northwestern sub-population (p = 0.055; Table 4). No allele frequency shifts were detected.
Landscape genetic analysis
At the larger spatial scale of the whole northern portion of our study area considered together, the top three models included elevation and minimum and maximum temperature, which were only separated by a ΔAIC of 1.2 (Table 4). When NE and NW portions of the study area were considered separately, as indicated by the Geneland analyses, different variables were supported. In the NE population, model selection supported a single best model (ΔAIC ~3) which showed a positive relationship between elevation relief ratio (ERR) and genetic distance, such that areas with large ERR values acted as barriers to gene flow (Table 4). This model performed better than the null model of isolation-by-Euclidean distance (IBD) alone (ΔAIC = 4.7), but the parameter value for ERR was very small. However, the other variables in the top five supported models—elevation, vegetation type, and pipelines—did not perform better than the null model. In the NW population, there was no clear best model. The top five models included roads, vegetation, pipelines, and slope, and but it questionable the effects that these landscape variables had on population genetic structure as parameter values were extremely small, but significantly greater than zero. Additionally, ΔAIC between the best model and the null (IBD) model was only 1.1. In both geographic partitions of the data, the top models had low R2 values (0.098–0.11), suggesting that all models tested explained only a small proportion of the variance in genetic distances.
Discussion
Here, we show four main results. First, there is more population structure in Tasmanian devils than has been shown in previous studies. That is, despite very large home ranges and dispersal capabilities in Tasmanian devils, there is localized genetic structure along the western coast of Tasmania. Second, genetic diversity analyses suggest weak evidence for a genetic bottleneck across northwest Tasmania. These results raise possible concern about reduced adaptive potential for DFTD resistance in the last remaining disease-free area of devils. Nonetheless, Tasmanian devil genetic diversity is quite low island-wide with near universal DFTD susceptibility, so this result may not be important as disease moves through our study area. Third, depending on the spatial scale of the landscape genetics analyses, results vary. At the largest spatial scale, only elevation and temperature (min and max temperatures), consistent with the influence these have on vegetation type and thus habitat suitability and prey availability, which influence juvenile dispersal decisions, affect landscape genetic structure. However, at the finer spatial scale of considering landscape genetic structure within the northeastern and northwestern populations in our study area, elevation relief is only significant in the NE, because the NW area has comparatively little topographic relief. In the NW, landscape variables did not explain variance in gene flow any better than a straight line isolation-by-distance model. Fourth, our landscape genetics analyses suggest that DFTD spread throughout the northwestern disease-free region of Tasmania is imminent. This result is attributable to the location of the present disease front at the eastern edge of our study area, and that our top landscape genetic models showed that key landscape features throughout the disease-free area are unlikely to impede gene flow.
Population structure and genetic diversity
Similar to other larger mammals that range widely (Montgelard et al. 2014; Mager et al. 2014), Tasmanian devils show little evidence of population genetic structure across northwestern Tasmania. Spatial genetic clustering analyses suggest two to three genetic clusters across a study area that is approximately 100 km wide and 250 km long. The Pieman River, a deep, and at times strongly flowing water body between 30 and 100 m wide (Fig. 1), does not appear to be a barrier to gene flow because there is no detectable genetic structure between devils captured north and south of the river. The only major barrier to devil gene flow throughout our study area appears to be Macquarie Harbour (see Fig. 1), consistent with previous work (Brüniche-Olsen et al. 2014). The harbour is a glacial feature currently an over 1 km deep saltwater body which perhaps presented a barrier to devil movement when sea levels were at their lowest during the last glacial maximum. Indeed, FST values between devils found south of the harbor and either of the two northern genetic clusters are greater than 0.26, indicating high genetic substructure.
Geneland did detect population subdivision between the NE and NW portions of our study area, although pairwise FST between the two is 0.021. This portion of our study area is dominated by dairy farms, clear-fell logging and increased road density. Devils avoid completely open areas, and roads are a major source of devil mortality, which could contribute to the weak genetic subdivision observed in this area.
Our findings contrast with previous genetic studies of Tasmanian devils that have shown an island-wide K of two, with all western devils belonging to one genetic cluster (Jones et al. 2004; Miller et al. 2011). With a likely K of three, our study demonstrates that there is more genetic structure in Tasmanian devils than has been shown in previous coarse scale, island-wide studies. On a finer spatial scale, we observed positive spatial autocorrelation up to distances of approximately 40 km, which is about half the size than that found by Lachish et al. (2011) in east-central Tasmania. Discrepancies in genetic neighborhood sizes in different parts of species’ geographic ranges is not uncommon (Short Bull et al. 2011; Trumbo et al. 2013). Variation in the extent of spatial autocorrelation in eastern versus western Tasmanian devils may be influenced by relatively high human disturbance in the west, or by higher rainfall in the west that supports wetter, denser vegetation that may influence food availability, population density, and consequent dispersal decisions. Indeed, diet and habitat choice have been documented to affect dispersal in other animals, such as coyotes (Sacks et al. 2005). Given there is some discrepancy as to support for two (sPCA) or three (Geneland) genetic clusters, additional fine-scale studies with a greater number of molecular markers will help to understand better how Tasmanian devils are subdivided across Tasmania.
Throughout the area in northwestern Tasmania, genetic diversity in the Tasmanian devil is low, supporting previous work. Previously, Miller et al. (2011) showed that Tasmanian devils have the second lowest level of mtDNA diversity of any mammal studied (the lowest being found in the thylacine or Tasmanian tiger (Thylacinus cynocephalus), the largest marsupial carnivore that went extinct in the 1930s). Low genetic diversity in devils is consistent with their demographic history with major periods of population decline at the end of the last glacial maximum, as well as around El Niño events between 3,000 and 6,000 years ago (Brüniche-Olsen et al. 2014). Levels of genetic diversity in this study are consistent with those previously reported from the east, suggesting DFTD susceptibility in this region will likely be similar to that of devils in other parts of Tasmania.
We also found weak evidence of genetic bottlenecks in the northeastern and northwestern portions of our study area. Although FIS values within each genetic cluster are significantly greater than zero, they are small (<0.1). Additionally, we found weak evidence of heterozygote excess in the two northern clusters; heterozygote excess is theorized to be transient for several generations following a bottleneck due to loss of rare alleles (Cornuet and Luikart 1996; Luikart et al. 1998). It is possible that widespread clear-fell logging for forest production and increases in dairy farming in the last 20 years could have contributed to the observed bottlenecks. Although devils appear to be rapidly evolving in response to DFTD east of this area (Epstein et al. 2016; Pye et al. 2016), reduced genetic diversity may compromise future adaptive genetic potential. Future genome-wide studies will help to assess the extent of adaptive genetic diversity in Tasmanian devils.
Landscape genetic analyses
We conducted landscape genetic analyses at two spatial scales. First, we considered landscape genetic structure at the finer spatial scale within the northeastern and northwestern clusters as identified by Geneland. Second, given that spatial PCA analyses did not support two genetic clusters in this area and there was a gap in sampling that may have resulted in mis-identification of a genetic break in Geneland (Schwartz and McKelvey 2009), we also tested the effects of landscape variables at the larger spatial scale of NE and NW combined.
At the smaller spatial scale, elevation relief ratio was the only significant variable in the top model for the NE. The northeastern portion of the study area contains topographic relief coming from the steep upper catchment of the Arthur River system, which may limit gene flow. In contrast, there was no clear top model for the northwestern part of the study area based on ΔAIC values, and models that included landscape variables did not perform better than the null model of IBD alone. The northwestern portion lacks any significant topographic relief.
At the larger spatial scale of the whole northern portion of our study area considered together, top models included elevation and temperature (minimum and maximum temperatures) (Table 4). These results are consistent with the demography and life history of Tasmanian devils. That is, large-scale movements that lead to gene flow result almost exclusively from post-natal dispersal by juveniles (Lachish et al. 2011). Juveniles leave their den within weeks of weaning at 9 months of age and travel for 6–8 months before establishing an adult home breeding range. Dispersal decisions are likely influenced by suitable vegetation communities that support adequate prey; thus devils avoid dense, wet vegetation at altitude. Differences in effects of landscape variables and fine and broad spatial scales in our study suggest that analyses at different spatial scales may yield important insights into more localized effects of landscape variables on gene flow, as well as more generalized effects at larger spatial extents.
Overall, however, landscape genetic models only explained a small proportion of variation in gene flow (Table 4). These results suggest that unmeasured environmental variables could be responsible for genetic structuring in Tasmanian devils. While we included available environmental variables expected to affect devil gene flow based on previous mark-recapture and radiocollaring studies in our landscape genetic models, variables that proximately affect devil abundance and dispersal decisions, such as prey availability, are difficult to measure and are not available in GIS layers.
Given the large spatial extent of observed spatial autocorrelation throughout the study area, however, it is also quite possible that most landscape variables have little effect on devil dispersal and gene flow. That is, Tasmanian devils generally disperse large distances, and at the spatial scale of our study area, there is limited influence of landscape processes on devil gene flow. Generally parameter values were low, even for top models in the NE, and landscape variables in the NW area did not explain spatial genetic variation any better than straight line Euclidean distance alone.
Inferring propensity for disease spread
Estimations of host genetic structure can potentially be used as a coarse proxy for estimating disease spread in cases when diseases are directly transmitted (Biek and Real 2010). This may be particularly the case for DFTD, which cannot live outside an individual host and is transmitted via biting during social contact (Hamede et al. 2013). In the case of Tasmanian devils, given that we found weak genetic structure overall and positive spatial genetic autocorrelation exceeding 40 km, DFTD spread throughout the northwestern disease-free region is likely inevitable. On a more positive note, however, we found a high degree of population subdivision between Macquarie Harbor and the precipitous ravines and dense wet rainforests of the lower Gordon river system, which should slow or impede the spread of DFTD to devils in this southwestern region. Risk of DFTD arriving in the southwest region of our study area from more eastern populations remains low, as devils are quite sparsely distributed for at least 100 km to the east of this part of our study area (see Fig. 1, dashed outline). Although DFTD has caused localized declines exceeding 90% in many localities, recent evidence suggests that Tasmanian devils may be evolving resistance to DFTD insomuch as some long-term diseased populations are increasing in size and some individuals are showing signs of recovering from infection (Epstein et al. 2016; Pye et al. 2016).
References
Altizer S, Ostfeld RS, Johnson PTJ, Kutz S, Harvell CD (2013) Climate change and infectious diseases: from evidence to a predictive framework. Science 341:514–519
Bartón K (2015) MuMIn: R code package. https://cran.rproject.org/web/packages/MuMIn/MuMIn.pdf
Bates D, Maechler M, Bolker B, Walker S (2014) R package lme4 https://cran.r-project.org/package=lme4
Biek R, Real LA (2010) The landscape genetics of disease emergence and spread. Mol Ecol 19:3515–3531
Blanchong JA, Samuel MD, Scribner KT, Weckworth BV, Langenberg JA, Filcek KB (2008) Landscape genetics and the spatial distribution of chronic wasting disease. Biol Letts 4:130–133
Blanchong JA, Robinson SJ, Samuel MD, Foster JT (2016) Application of genetics and genomics to wildlife epidemiology. J Wildl Manag 80:593–608
Bowcock AM, Ruiz-Linares A, Tomfohrde J et al (1994) High resolution of human evolutionary trees with polymorphic microsatellites. Nature 368:455–457
Brüniche-Olsen A, Jones ME, Austin JJ, Burridge CP, Holland BR (2014) Extensive population decline in the Tasmanian devil predates European settlement and devil facial tumor disease. Biol Lett 10:20140619
Chapuis MP, Estoup A (2007) Microsatellite null alleles and estimation of population differentiation. Mol Biol Evol 24:621–631
Clarke RT, Rothery P, Raybould AF (2002) Confidence limits for regression relationships between distance matrices: estimating gene flow with distance. J Agric Biol Environ Stat 7:361–372
Cornuet JM, Luikart G (1996) Description and power analysis of two tests for detecting recent population bottlenecks from allele frequency data. Genetics 144:2001–2014
Crida A, Manel S (2007) Wombsoft: an r package that implements the Wombling method to identify genetic boundary. Mol Ecol Res 7:588–591
Cullingham CI, Kyle CJ, Pond BA, Rees EE, White BN (2009) Differential permeability of rivers to raccoon gene flow corresponds to rabies incidence in Ontario, Canada. Mol Ecol 18:43–53
Epstein B, Jones M, Hamede R, Hendricks S, McCallum H, Murchison EP, Schönfeld B, Wiench C, Hohenlohe P, Storfer A (2016) Rapid evolutionary response to a transmissible cancer in Tasmanian devils. Nat Commun. doi:10.1038/ncomms12684
Guillot G, Estoup A, Mortier F, Cosson JF (2005) A spatial statistical model for landscape genetics. Genetics 170:1261–1280
Guillot G, Santos F, Estoup A (2008) Analysing georeferenced population genetics data with Geneland: a new algorithm to deal with null alleles and a friendly graphical user interface. Bioinformatics 24:1406–1407
Hamede RK, Bashford J, Jones M, McCallum H (2012) Simulating devil facial tumour disease outbreaks across empirically derived contact networks. J Appl Ecol 49:447–456
Hamede RK, McCallum H, Jones ME (2013) Biting injuries and transmission of Tasmanian devil facial tumour disease. J Anim Ecol 82:182–190
Hardy OJ, Vekemans X (1999) Isolation by distance in a continuous population: reconciliation between spatial autocorrelation analysis and population genetics models. Heredity 83:145–154
Hardy OJ, Vekemans X (2002) SPAGeDi: a versatile computer program to analyse spatial genetic structure at the individual or population levels. Mol Ecol Notes 2:618–620
Hawkins CE, Baarsc C, Hestermana H, Hocking GJ, Jonesa ME, Lazenbya B, Manna D, Mooney N, Pemberton D, Pyecroft S, Restani M, Wiersma J (2006) Emerging disease and population decline of an island endemic, the Tasmanian devil Sarcophilus harrisii. Biol Conserv 131:307–324
Hempel K, Earn JD (2015) A century of transitions in New York city’s measles dynamics. J R Soc Interface 12:20150024
Jombart T (2008) adegenet: a R package for the multivariate analysis of genetic markers. Bioinformatics 24:1403–1405
Jones ME, Paetkau D, Geffen E, Moritz C (2003) Microsatellites for the Tasmanian devil (Sarcophilus laniarius). Mol Ecol Notes 3:277–279
Jones ME, Paetkau D, Geffen E, Moritz C (2004) Genetic diversity and population structure of Tasmanian devils, the largest marsupial carnivore. Mol Ecol 13:2197–2209
Lachish S, McCallum H, Mann D, Pukk C, Jones M (2010) Evaluating selective culling of infected individuals to control Tasmanian devil Facial Tumor Disease. Conserv Biol 24:841–851
Lachish S, Miller KJ, Storfer A, Goldzien AW, Jones ME (2011) Evidence that disease-induced population decline changes genetic structure and alters dispersal patterns in the Tasmanian devil. Heredity 106:172–182
Landguth EL, Hand BK, Glassy J, Cushman SA, Sawaya MA (2011) UNICOR: a species connectivity and corridor network simulator. Ecography 35:9–14
Lane A, Cheng Y, Wright B, Hamede R, Levan L, Jones M, Ujvari B, Belov K (2012) New insights into the role of MHC diversity in devil facial tumour disease. PLoS ONE. doi:10.1371/journal.pone.0036955
Lee JS, Ruell EW, Boydston EE, Lyern LM, Alonzo RS, Troyer JL, Crooks KR, VandeWoode S (2012) Gene flow and pathogen transmission among bobcats (Lynx rufus) in a fragmented landscape. Mol Ecol 21:1617–1631
Luikart G, Allendorf FW, Cornuet J-M, Sherwin WB (1998) Distortion of allele frequency distributions provides a test for recent population bottlenecks. J Hered 89:238–247
Mager KH, Colson KE, Groves P et al (2014) Population structure over a broad spatial scale driven by non anthropogenic factors in a wide-ranging migratory mammal, Alaskan caribou. Mol Ecol 23:6045–6057
Manel S, Berthoud F, Bellemain E, Gaudeul M, Luikart G, Swenson JE, Waits LP, Taberlet P, Intrabiodiv Consortium (2007) A new individual-based spatial approach for identifying genetic discontinuities in natural populations. Mol Ecol 10:2031–2043
Manel S, Holderegger R (2013) Ten years of landscape genetics. Trends Ecol Evol 28:614–621
McCallum H, Jones ME (2006) To lose both would look like carelessness: Tasmanian devil facial tumour disease. PLoS Biol 4:1671–1674
McCallum H, Jones ME, Hawkins C, Hamede R, Lachish S, Sinn DL, Beeton N, Lazenby B (2009) Transmission dynamics of Tasmanian devil facial tumor disease may lead to disease-induced extinction. Ecology 90:3379–3392
Miller W, Hayes VM, Ratan A et al (2011) Genetic diversity and population structure of the endangered marsupial Sarcophilus harrisii (Tasmanian devil). Proc Natl Acad Sci USA 108:12348–12353
Montgelard C, Zenboudji S, Ferchaud A-L et al (2014) Landscape genetics in mammals. Mammalia 78:139–157
Nakagawa S, Schielzeth H (2013) A general and simple method for obtaining R2 from generalized linear mixed-effects models. Methods Ecol Evol. doi:10.1111/j.2041-210x.2012.00261.x
Paquette SR, Talbot B, Garant D, Mainguy J, Pelletier F (2014) Modelling the dispersal of the two main hosts of the raccoon rabies variant in heterogeneous environments with landscape genetics. Evol Appl 7:734–749
Piry S, Luikart G, Cornuet J-M (1999) BOTTLENECK: A program for detecting recent effective population size reductions from allele data frequencies. http://www1.montpellier.inra.fr/CBGP/software/Bottleneck/pub.html
Pye R, Hamede R, Siddle HV, Caldwell A, Knowles GW, Swift K, Kreiss A, Jones ME, Lyons B, Woods GM (2016) Demonstration of immune responses against devil facial tumour disease in wild Tasmanian devils. Biol Lett 12:20160553
Riley S (2007) Large-scale spatial-transmission models of infectious disease. Science 316:1298–1301
Rousset F (2008) Genepop’007: a complete reimplementation of the Genepop software for Windows and Linux. Mol Ecol Res 8:103–106
Sacks BN, Mitchell BR, Williams CL, Ernest HB (2005) Coyote movements and social structure along a cryptic population genetic subdivision. Mol Ecol 14:1241–1249
Schwartz MK, McKelvey KS (2009) Why sampling scheme matters: the effect of sampling scheme on landscape genetic results. Conserv Genet 10:441–452
Shaman J, Karspeck A (2012) Forecasting seasonal outbreaks of influenza. Proc Natl Acad Sci USA 50:20425–20430
Short Bull RA, Cushman SA, Mace R et al (2011) Why replication is important in landscape genetics: American black bear in the Rocky Mountains. Mol Ecol 20:1092–1107
Siddle HV, Kreiss A, Eldridge MDB, Noonan E, Clarke CJ, Pyecroft S, Woods GM, Belov K (2007) Transmission of a fatal clonal tumor by biting occurs due to depleted MHC diversity in a threatened carnivorous marsupial. Proc Natl Acad Sci USA 104:16221–16226
Siddle HV, Marzek J, Cheng Y, Jones ME, Belov K (2010) MHC gene copy number variation in Tasmanian devils: implications for the spread of a contagious cancer. Proc R Soc B 277: 2001–2006
Smith KF, Sax DF, Lafferty KD (2006) Evidence for the role of infectious disease in species extinction and endangerment. Conserv Biol 20:1349–1357
Storfer A, Murphy MA, Evans JS, Goldberg CS, Robinson S, Spear SF, Dezzani R, Demelle E, Vierling L, Waits LP (2007) Putting the “landscape” in landscape genetics. Heredity 98:128–142
Storfer A, Murphy MA, Spear SF, Holderegger R, Waits LP (2010) Landscape genetics: where are we now? Mol Ecol 19:3496–3514
Truett GE, Heeger P, Mynatt RL, Truett AA, Walker JA, Warman ML (2000) Preparation of PCR-quality mouse genomic DNA with hot sodium hydroxide and tris (HotSHOT). Biotechniques 29:52–54
Trumbo DR, Spear SF, Baumsteiger J, Storfer A (2013) Rangewide landscape genetics of an endemic Pacific northwestern salamander. Mol Ecol 22:1250–1266
van Oosterhout C, Hutchison B, Willis D, Shipley P (2004) MICRO-CHECKER: software for identifying and correcting genotyping errors in microsatellite data. Mol Ecol Notes 4:535–538
van Strien MJ, Keller D, Holderegger R (2012) A new analytical approach to landscape genetic modelling: least-cost transect analysis and linear mixed models. Mol Ecol 21:4010–4023
Acknowledgements
This work was supported by NSF DEB-1316549 to AS and MJ, and MJ was also supported by an ARC Future Fellowship FT100100250. Animal use was approved under IACUC protocol ASAF# 04392 from Washington State University and by the Tasmanian Department of Primary Industries, Parks, Water and Environment (DPIPWE) Animal Ethics Committee. Samples were collected by the Save the Tasmanian Devil Program (DPIPWE), particularly by Jason Wiersma, Jim Richley, Billie Lazenby, Stewart Huxtible, Clare Hawkins, Harko Werkman, and Dydee Mann. We thank Sarah Emel for help with analyses and four anonymous reviewers for comments that helped improve the manuscript.
Author information
Authors and Affiliations
Corresponding author
Electronic supplementary material
Below is the link to the electronic supplementary material.
Rights and permissions
About this article
Cite this article
Storfer, A., Epstein, B., Jones, M. et al. Landscape genetics of the Tasmanian devil: implications for spread of an infectious cancer. Conserv Genet 18, 1287–1297 (2017). https://doi.org/10.1007/s10592-017-0980-4
Received:
Accepted:
Published:
Issue Date:
DOI: https://doi.org/10.1007/s10592-017-0980-4