Abstract
We leverage a granular representation of mobility patterns before and during the first wave of SARS-COV2 in Italy to investigate the economic consequences of various forms of lockdown policies when accounting for mobility restrictions between and within local jurisdictions, i.e. municipalities, provinces and regions. We provide an analytical characterization of the rate of economic losses using a network-based spectral method. The latter treats the spread of contagion of economic losses due to commuting restrictions as a dynamical system stability problem. Our results indicate that the interplay between lower level of smartworking and the polarization of commuting flows to fewer local labor hubs in the South of Italy makes Southern territories extremely important in spreading economic losses. We estimate an economic contraction of total income derived from commuting restrictions in the range of 10–30% depending on the economic assumptions. However, alternative policies proposed during the second wave of SARS-COV2 can pose a greater risk to Northern areas due to their higher degree of mobility between jurisdictions than Southern ones. The direction of economic losses tend to propagate from large to medium-small jurisdictions across all alternative lockdown policies we tested. Our study shows how complex mobility patterns can have unequal consequences to economic losses across the country and call for more tailored implementation of restrictions to balance the containment of contagion with the need to sustain economic output.
Similar content being viewed by others
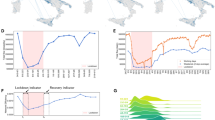
1 Introduction
Mobility reduction has been identified as a key intervention to effectively limit the spread of the epidemics during both the early stages of the virus diffusion and in subsequent waves of contagion (Aleta et al., 2020; Schlosser et al., 2020; Spelta & Pagnottoni, 2021; Giudici et al., 2023). This aspect motivated the adoption of lockdown policies grounded on mobility restrictions as mechanisms to effectively limit infections (Chinazzi et al., 2020; Hsiang et al., 2020; Xiong et al., 2020). However, such interventions cause severe disruptions on mobility patterns (Schlosser et al., 2020; Zhang et al., 2020; Smolyak et al., 2021) and have significant drawbacks to the social fabric and negative consequences for the real economy (Alfaro et al., 2020; Glaeser et al., 2020; Guan et al., 2020; Jay et al., 2020; Sarkodie & Owusu, 2021).
There exists ongoing effort to understand the balance between the impacts of mobility restrictions on the spreading of contagion and their direct and indirect effects on economic systems (Altig et al., 2020; Haug et al., 2020; Saltelli et al., 2020). For instance, recent studies have recognized how mobility reductions carried detrimental effects on economic systems during the lockdown phase (Bonaccorsi et al., 2020; Chang et al., 2020; Polyakova et al., 2020; Jay et al., 2020). In particular, mobility restrictions have been shown to be strongly correlated with the reduction of consumption (Carvalho et al., 2020; Chetty et al., 2020; Sheridan et al., 2020; Dietrich et al., 2022) and the loss of aggregate economic output (Fernández-Villaverde & Jones, 2020; Pagnottoni et al., 2021). Literature has identified some relevant factors favouring a fast and short (V-shaped) recovery vs. a slower and longer one (U- or L-shaped), highlighting the interplay between local socio-economic conditions and lockdown policies (see, e.g., (Deb et al., 2020; Eichenbaum et al., 2020; Gregory et al., 2020; Guerrieri et al., 2020; Kaplan et al., 2020; Martin et al., 2020; van Der Voet, 2021; Bonaccorsi et al., 2021; Demirgüç-Kunt et al., 2021)). More generally, lockdown measures and mobility restrictions have been shown to be relevant Non Pharmaceutical Interventions (NPIs) for contagion containment (Davies et al., 2020; Flaxman et al., 2020; Lau et al., 2020; Della Rossa et al., 2020; Maier & Brockmann, 2020; Kumar et al., 2021; Gajpal et al., 2022). The economic assessment of such mobility restriction measures is of utmost importance for policy makers and motivates a growing interest on the investigation and measurement of trade-offs between the need to limit the spread of contagion and the provision of adequate levels of economic output (Baker et al., 2020; Haug et al., 2020; Saltelli et al., 2020; Spelta et al., 2020; van Der Voet, 2021). In fact, mobility restrictions heavily affect both the configuration of mobility networks and the structure of economic systems by influencing the inter-dependencies among geographical zones. For instance, Bonaccorsi et al. (2020) find that the impact of lockdown measures on mobility is stronger in Municipalities with higher fiscal capacity and that mobility contraction is stronger in those Municipalities characterized by higher levels of inequality but lower levels of income per capita, thus indicating the potential for a segregation effect, while Bonaccorsi et al. (2021) find evidence of persistent effects even when mobility restrictions are lifted. van Der Voet (2021) discusses how policy preferences to cope with the evolution of SARS-COV2 relate to the way decision-makers interpret the nature of the pandemic in terms of the negative economic prospects and impact on health. Coibion et al. (2020) show how differential timing of local lockdowns impacts on households’ spending and macroeconomic expectations at the local level, while Goolsbee and Syverson (2020), by using mobile phone data on customer visits to more than 2.25 million businesses, find that, although lockdown measures account for only a modest share of the massive changes in consumer behavior, they had a significant role in reallocating consumer visits away from nonessential to essential businesses. Mobile phone data are also employed in Jay et al. (2020), who find that people living in high-income areas increased their “days at home” substantially more than those in low-income neighbourhoods, who instead are more likely to work outside their residence.
Our work relies on this stream of research which has already shown the efficacy of massive data collection from social networks, credit cards payment systems and mobile phone data to the study of SARS-COV2 (see, e.g., (Grantz et al., 2020; Kuchler et al., 2020; Kumar et al., 2021)). Contributing to this literature, our study integrates mobility data with local economic information to build a model of economic losses due to local mobility restrictions, which we use to evaluate heterogeneous policy interventions. Here, we opt for a parsimonious, network-based, spectral model to study the stability conditions of an economic system in which workers move across territories contributing to their aggregate level of economic output. More specifically, we investigate the impact of SARS-COV2 on the economy in terms of policy restrictions preventing workers to reach workplaces. Such restrictions can have a detrimental effect to the available economic resources of local jurisdictions due to the increasing rates of unemployment, contraction of consumption, etc., which can lead to further reductions to local production by inducing more limitations to workers commuting from other territories. In this work, such higher-order effects are nuanced by the role of smartworking that allows some workers to continue their activities remotely, thus limiting the negative impacts of lockdown restrictions. We model the economic environment as a system composed by interconnected units that are linked by agents commuting to work. By taking into account the heterogeneous business characteristics of local jurisdictions and the complex network of commuting patterns, we design a framework to assess the economic consequences of various forms of lockdown policies. To do so, we model the spread of economic losses as a dynamical system stability problem. This approach has been already employed in several domains, from biology to economic and financial systems, to study complex non-linear interdependencies (May, 1972, 1974; Newman, 2010; Markose et al., 2012, 2021; Bardoscia et al., 2017)
We design a spectral method to identify the stability of tipping point of the mobility network (see Markose et al., 2012, 2021), thus producing a signal that can support regulators in assessing the behavior of the system and help them to prevent/mitigate negative consequences of systemic events triggered by lockdowns. The application of spectral methods of stability has been well adopted in financial models of systemic risk due to the significance of their steady-state solutions and fixed point algorithms that reflect internal consistency to a high order of interconnectedness (Gauthier et al., 2012). The popular financial network model of Eisenberg and Noe (2001) and further extensions (Rogers & Veraart, 2013; Schuldenzucker et al., 2020),among others rely on a fixed-point method to assess the losses suffered by financial units in a payment network. As an example, Battiston et al. (2016) uses a similar approach to the above-mentioned spectral method by deriving a centrality fixed-point solution, although they do not adopt the concept of stability tipping point as an endogenous fixed point solution. The latter is provided by Bardoscia et al. (2017) for interbank networks and by Heath et al. (2014) for central clearing counterparts. To the best of our knowledge, the present study is the first to extend spectral methods to mobility networks. The value added of our approach is the derivation of steady-state solutions at both the macro level, i.e. whole system instability, and at the micro level, i.e. the contribution of individual local jurisdictions to the national level of instability, as well as a network-based characterization that allows for testing several NPIs.
Since Italy was among the first countries implementing mobility restrictions to contain the spread of SARS-COV2, we consider it as a relevant benchmark to test the predictions of our model. We employ a granular representation of mobility patterns in Italy using Facebook Data for Good Prevention maps see Maas et al. (2019). This data contains geo-localized daily movements across Municipalities of about four million of individuals. We then combine mobility data with socio-economic information, such as pro-capita income and number of workers in different jurisdictions, to compute economic losses derived from different levels of mobility restrictions, which we use as proxies for different lockdown policies.
The contribution of this paper is twofold. We first provide an analytical characterization of the rate of economic losses, at the jurisdiction level (Municipality or Province level), originating from commuting restrictions. Specifically, the proposed spectral method identifies the tipping point at which the extent of losses coming from mobility restrictions produce instability, i.e. when the economic resources available at the local administrative level might no longer be sufficient to absorb economic losses caused by mobility restrictions. Our model also provides both the importance and vulnerability of each jurisdiction to the overall instability of the system. These rankings shed light on the expected impact of mobility restrictions across different territories and are useful to guide policy makers in adopting the most cost-effective lockdown policies.
Second, we show how different degrees of mobility restrictions generate heterogeneous impacts at the level of Italian jurisdictions. The proposed network-based approach reveals complex mobility patterns differentiating jurisdictions across economic areas, with Southern territories appearing more penalized than Northern ones, although the former represents a much smaller proportion of all economic resources at stake. This feature is the result of the interplay between lower level of smartworking and the polarization of commuting flows to fewer industrial hubs in the South of Italy, which leave these territories extremely important in spreading economic losses. Indeed, when controlling for smartworking, we observe that more populated administrative units contribute the most to the overall system instability, regardless of their geographic location. However, mobility patterns around those large jurisdictions vary between the North and the South of Italy. The former is characterised by a high degree of mobility between neighbour jurisdictions compared to the latter, in which the vast majority of commuting is observed within the same jurisdiction.
Our approach suggests that the complexity of mobility patterns contributes to non-linear outcomes as a result of alternative lockdown restrictions. Specifically, mobility policies that treat the mobility within and between jurisdictions differently can have notably different economic consequences in different parts of the country. Those alternative policies were proposed in the recent lockdown implemented during the second wave of SARS-COV2 in Italy (Presidente del Consiglio dei Ministri, 2020), with mobility allowed within jurisdictions while enforcing harder restrictions between Provinces and Regions. Overall our results show that the effects of lockdown policies are unequally distributed across territories as a result of the observed complex mobility patterns and call for a more tailored implementation of restrictions that minimize negative economic consequences. Although the comparison between pre and post lockdown measures on economic stability helps controlling for endogenous structural weaknesses within each region, we acknowledge that endogenously mobility patterns determined by the endowment of infrastructures can affect lockdown restrictions and stability outcomes. The ability of our framework to calibrate the dynamics of the system at the local level provides a powerful tool to ad-hoc calibrations with higher expected accuracy.
The paper is organised as follows. Section 2 describes the data and estimation methodology of the network approach. The spectral dynamical model is presented in Sect. 3 along with its stability conditions. Section 4 gives the results on Italian jurisdictions. Section 5 concludes by discussing policy implications of the work.
2 The dataset
In order to study the economic consequences derived from alternative lockdown schemes and mobility patterns, we employ a massive dataset of human commuting of near real-time observations provided by Facebook Disease Prevention maps (Maas et al., 2019) through its Data for Good program.
Facebook Disease Prevention maps offer information about geo-localized movements of individuals. We consider movements in Italy over the period from February 24th to March 23rd 2020. The dataset contains about 800,000 distinct observations (recorded with a 8-hour frequency) covering daily movements across 3000 Italian Municipalities of about four million individuals.
Each observation in the dataset tracks movements of individuals across tilesFootnote 1 (Schwartz, 2018). The granularity of the data allows us to map mobility among different jurisdictions, from Municipal to Provincial and Regional level depending on the specific focus of our analysis. The aggregation process consists of summing together all data records belonging to the same territorial unit (e.g. Municipalities, Provinces), allowing for a high level of geographic scalability required when assessing different lockdown policies.
We investigate mobility patterns through the lens of network theory by interpreting the dataset records as a directed weighted graph, with nodes representing territorial units and edges being the weighted connections measuring the amount of traffic of individuals flowing between two locations. In order to investigate economic losses caused by lockdown interventions, we build up two mobility networks corresponding to Pre and Lock phases of the mobility restrictions. In particular, mobility data is aggregated over two 14-day windows, before and after the 9th of March 2020, the announcement date of the main policy mobility restriction in Italy see Presidente del Consiglio dei Ministri (2020). Mobility data is then combined with socio-economic information to assess economic losses. We gather socio-economic variables at Municipality and Province level from the Italian National Institute of StatisticsFootnote 2 (ISTAT) and from the Italian Ministry of Economy and FinanceFootnote 3 (MEF). The dataset contains information on pro-capita income and number of workers in different territories for the year 2019. We also include the percentage of smartworkers at the Regional level estimated by Barbieri et al. (2020).
In our analysis, the Pre lockdown network will serve as baseline specification of commuting patterns for building our stability model and for measuring the consequent economic losses derived from different lockdown schemes. The Pre mobility network will later be compared with the Lock network to evaluate the actual impact of lockdown to mobility and draw conclusions on how different territorial unis have been effectively exposed to economic lossesFootnote 4. The comparison of those outcomes with our estimates of losses after the first wave of lockdown measures can also provide validation of our approach.
Figure 1 shows the georeferenced mobility network at the Municipality level, before and during the national lockdown. On one hand, the mobility reduction is clearly observable in this illustrative example. On the other hand, densely connected zones, such as Torino, Milano, Bologna, Roma and Napoli areas are still connected during lockdown. Moreover, the national transportation infrastructure is not disrupted as highlighted by the presence of long distance links at the time of the lockdown phase. However, important changes in commuting patterns traveled by individuals are clearly observed.
We notice in Fig. 1 how commuting between different Municipalities decreases during the lockdown and many of them become isolated from the rest of the Italian territory, especially in the South of Italy and in the Alps and Apennines territories. This outcome is quantified in Fig. 2. Starting form the upper panel, the histograms describe the probability of observing a certain number of individuals travelling either within a Municipality (left) or between different Municipalities (right), before and during the lockdown phase. We notice that the implementation of lockdown measures has caused a right shift of the within Municipality distribution and the corresponding left shift of the curve representing movements between different Municipalities. This suggests that, while a decrease of the mobility flows is observed at the aggregate level, part of this effect is offset by a change in the proportions of people travelling within and between Municipalities. We further investigate this outcome in the lower panel of Fig. 2 by reporting the between over within mobility ratio, aggregated at Regional level. As expected, the ratio declines more than half in many cases, from highest values during the pre-lockdown phase of 40:1 to 15:1 during the national lockdown. Interestingly, Northern high populated regions, like Lombardia, Veneto and Piemonte are characterised by the highest ratios compared to Southern Regions, where the vast majority of mobility is concentrated within Municipalities. As we will see in our stability analysis of Sect. 4, heterogeneous mobility patterns across the country will play an important role in shaping the economic importance and vulnerability of different geographic areas during the lockdown.
Change of mobility patterns in Italy due to lockdown measures. The upper panel reports the distributions of the within Municipality mobility patterns (left) and the between Municipality mobility flows (right) before the intervention (blue) and during the lockdown phase (orange). The lower panel shows the between-within mobility ratio at regional aggregation level, both for the pre-lockdown and lockdown phases
The clustering coefficient is another topological measure of interest to characterize the complex structure of the Italian mobility network, measuring the probability of nodes in a graph to cluster together forming triangles. Evidence suggests that in most real-world networks, and in particular social networks, nodes tend to create tightly knit groups, characterized by a relatively high density of ties (see Holland & Leinhardt, 1971; Watts & Strogatz, 1998). Indeed, the existence of self-reinforcing loops and triadic structures between Municipalities can amplify initial shocks and exacerbate economic losses through the network, thus decreasing system stability. This quantity has been shown to contribute to the instability of social networks and relate to the spectral tipping point result we adopt in our approach (Markose et al., 2012, 2021). As will see in the result Sect. 4, different degrees of clustering at the jurisdiction level can help explaining the importance of some territories in contributing to the overall instability of the economic system.
In graph theory, the node-level clustering coefficient for directed and weighted network (see Fagiolo, 2007) is defined as:
where \({\textbf{f}}\) represents the weighted adjacency matrix of the mobility network, \(k^{in}_{i}\) and \(k^{out}_{i}\) node’s i in- and out-degree respectively and, finally, \(k_{i}\) the degree centrality of the ith node.
Between and within clustering coefficient for the top-20 Provinces. The upper panel reports the normalized value of the clustering coefficient (aggregated at Province level) where only links between Municipalities belonging to different Provinces are maintained. The lower panel shows the normalized within clustering coefficient where only links between Municipalities belonging to the same Province are maintained. The blue bars refer to the pre-lockdown phase, while red bars are associated with the lockdown period
Figure 3 shows normalized clustering coefficient for the top-20 Provinces, for both the pre-lockdown and lockdown phases. The upper panel reports the clustering coefficient computed by holding network links connecting only Municipalities belonging to different Provinces, named “between-Province clustering”. On the contrary, the lower panel shows clustering coefficient when considering links between Municipalities in the same Province, named “within-Province clustering”. In the pre-lockdown phase, which represents our baseline structure to derive stability conditions, we note that Northern Provinces, especially in Lombardia, show the highest values of the between-Province clustering coefficient. This is due to the high volume of commuting between the Milano’s Province and neighboring areas like Monza-Brianza, Lecco and Como. Interestingly, the same behavior is observable in Toscana, where the Provinces of Prato, Pistoia and to a less extent Firenze display high values of this measure. If we restrict our attention to the within-Province clustering, we see a more homogeneous distribution of clustering values across the country with Central and Southern Provinces of Roma, Napoli and Cagliari climbing top positions. The lockdown phase does not affect much the rank order of clustering: the correlation coefficient of the clustering measures computed on Pre and Lock mobility networks is indeed 0.967 and 0.966 for the between-Province and within-Province clustering, respectively. We observe a few exceptions, in particular the province of Prato that dominates the ranking. Moreover, we provide in “Appendix A” an additional robustness check about how the mobility has reacted after the lockdown policy, and in particular whether the network backbone has undergone changes.
Finally, to validate the representativity of Facebook mobility data, we carry out a comparison exercises against the 2011 commuting network provided by the Italian National Institute of Statistics (ISTAT). Such data provides movements of employees/students traveling between municipalities that were recorded in the most recent census. Although there are several limitations to this comparison since the ISTAT network only includes inhabitants who said they were traveling for business or school and it is nine years old and information refers to the Italian mobility patterns of 9 years ago, we still think it is helpful for further validating the situation of Italian mobility prior to lockdown. Indeed, because the ISTAT data is not biased towards those who own a phone and are registered on social networks, as is Facebook data, we believe it is relevant to further confirm the representativity of the Italian mobility data observed prior to entering the lockdown phase. We create an averaged mobility graph during a 14-day period just before the shutdown (9th March). After that, we ran a Pearson correlation test on the metrics for node degree, node strength, and edge weight. In every instance, we discover a sizable positive association at the level \(\alpha =0.001\) (see Fig. 4). These findings provide us with a solid background for using Facebook mobility data as proxy of communing between and within Italian jurisdictions.
3 Model
This Section presents the network-based spectral method employed to determine the stability of economic systems under lockdown. The spectral method threats the spread of contagion of economic losses, arising from restrictions of the Italian commuting mobility network, as a dynamical system stability problem. This approach has been shown to be quite effective to assess financial contagion and systemic risk (Markose et al., 2012, 2021; Heath et al., 2014; Bardoscia et al., 2017).
Let \(\varrho _{xy}^{t}\) be the number of people commuting from location x to location y at day t, i.e. the number of individuals moving between the two locations. The estimates of entries in matrices \({\textbf{f}}^{h}\), where \(h=\{Pre,Lock\}\), represent mobility flows across local jurisdictions and are obtained as the average of mobility flows across locations after mapping each Municipality to the corresponding Province. In formulae:
Hence, elements \(\frac{f^{h}_{ij}}{\sum _{j}f^{h}_{ij}}\) represent the probability of an individual to leave district i for commuting to district j during the period h. Therefore, \(F^{h}_{ij}\) estimates the number of workers commuting from i to j as the product between the probabilities of mobility and the total number of workers \(I_i\) in each jurisdiction i.
Let the vector \({{\textbf{E}}}^{{\textbf{0}}}\) be the initial economic resources representing the total income earned by workers of each jurisdiction, \({\textbf{S}}\) the level of smart working and \({\textbf{W}}\) the average pro-capita wage of workers at the juristiction level. We define \({\textbf{X}}^h\), with entries \(X_{ij}^h=\frac{F_{ij}^h{\ W}_i}{E^0_i}\), the matrix of expected income produced by commuters from location i to j as a percentage of the economic resources of the jurisdiction i of the worker. This matrix maps the share of expected income of jurisdiction i produced by workers commuting to jurisdiction j and provides a direct economic measure of expected loss of income in the event of mobility restrictions from i to j.
3.1 Dynamical model of systemic local losses of lockdown
Following the spectral approach presented in Markose et al. (2012) and further extended by Markose et al. (2021), we define \({{\textbf{U}}^t}\) as the state vector of cumulative economic losses at the jurisdictional level, also known as the “rate of failure” of each jurisdiction. In our framework, the dynamics of the state vector \({{\textbf{U}}^t}\) is driven by the economic ties between jurisdictions and measured by \({\textbf{X}}\). Mobility restrictions caused by a lockdown in jurisdiction j can have a negative impact on the economic resources of i due to commuters \(F_{ij}^h\) not be able to travel to work, or smart working. This, in turn, can impair the economic activity in the jurisdiction i itself due to, for instance, a decline in the consumption level and local taxes, including the supply of labour with knock-on effects to other Provinces via the mobility channel.
According to Markose et al. (2012), there exist two major rates that drive the dynamics of the state vector \({{\textbf{U}}}^{t}\). The first rate is the proportion of economic resources, or buffer, that each jurisdiction can allocate to absorb losses. In our setup, these loss absorption facilities can take, for instance, the form of unemployment subsidies, tax reliefs, etc. In epidemic literature this is also known as the “cure rate” aimed at slowing down the spread of the virus. This buffer represents the stability threshold of each jurisdiction and it is denoted in our model by the vector \(\rho \). Economic losses above this threshold can lead a local unit to default, i.e. the jurisdiction is no longer able to sustain the local economy.
The second rate is the expected loss caused by other jurisdictions implementing lockdown measures and therefore limiting the accumulation of economic resources of a jurisdiction via commuting. Losses erode the economic resources of the local entity. Therefore, the state vector \({{\textbf{U}}}^{t}\) can also be interpreted as the cumulative losses measured as percentage of the initial economic resources, \(U^t_i=\frac{E^0_i-E^t_i}{E^0_i}\) for any jurisdiction i. Thus, the state vector \({{\textbf{U}}}^{t}\) monitors the cumulative losses of the local administrative unit at time t relative to the initial economic condition \({{\textbf{E}}}^{{\textbf{0}}}\).
The dynamics of \(U^t_i\) can be described by the following linear system:
where \(\left( 1-{\rho }_i\right) U^t_i\) is the contribution of the economic buffer \({\rho }_i\) in reducing its current failure rate \(U^t_i\) (i.e. the cumulative percentage losses) while the second part of the LHS of Eq. (4), \(\sum _j{\left\{ \dots \right\} }\), is the contagion channel of losses from other administrative units via the mobility matrix and weighted by their current failure rate \(U^t_j\). The higher the failure rate, the larger the contagion from other units.
Economic ties among jurisdictions, \(X_{ij}\), can cause contagion losses that will reduce the economic resources of exposed jurisdictions via a function \(g({\textbf{X}})\). This function maps the reduction of income due to commuting restrictions into economic losses of the jurisdiction. These losses aim at capturing contraction in consumption, local taxation, etc., that, in turn, will weaken the local economy, businesses and eventually the labour market. The contagion channel is also affected by the level of smart working in j (the higher \(S_j\in [0,1]\), the lower the losses propagated from unit j to i due to mobility restrictions) as well as the level of lockdown restrictions imposed in j (the higher \(L_j\in [0,1]\), the greater the losses propagated from unit j to i). Note that all of the contagion elements are weighted by the rate of failure of the local unit j, i.e. the cumulative level of losses of j at that time, namely \(U^t_j\). The higher \(U^t_j\), the more fragile the local unit j is (in terms of losses relative to the stability threshold) and therefore the higher the expected economic shocks to be propagated to other jurisdictions.
3.2 Stability analysis
The dynamical system of Eq. (4) can be described in matrix notation as:
with
It is simple to show that the dynamics of \({{\textbf{U}}}^{t}\) can either be explosive, i.e. all jurisdictions are unable to absorb losses, or converge to zero in the steady-state. In the first case, the system will be unstable to any non-negative external shock, while it will be stable in the second case as contagion losses will be internalised. The stability condition is controlled by the largest eigenvalue of \(\varvec{\theta }\) with the condition (see (Markose et al., 2021), for proof):
The corresponding left and right eigenvectors, \(\overleftarrow{{\textbf{v}}}\left( \varvec{\theta }\right) \) and \(\overrightarrow{{\textbf{v}}}\left( \varvec{\theta }\right) \), describe the systemic importance and vulnerability of each jurisdiction to the system instability, respectively. In a non-symmetric matrix, \(\overleftarrow{{\textbf{v}}}\) maps the relations from each column entry j to all row entries, while \(\overrightarrow{{\textbf{v}}}\) maps the relations from each row entry i to all column entries. According to the definition of rows and columns of the matrix \(\varvec{\theta }\) in system of Eq. (6), the direction of contagion propagates from columns j to rows i, and therefore the left eigenvector \(\overleftarrow{{\textbf{v}}}\left( \varvec{\theta }\right) \) can be interpreted as a measure of systemic importance (Importance) whereas \(\overrightarrow{{\textbf{v}}}\left( \varvec{\theta }\right) \) a measure of systemic vulnerability (Vulnerability). As a result of any non-negative initial perturbations of the matrix \(\varvec{\theta }\), such as mobility restrictions caused by lockdown, the relative importance of local units in spreading economic losses to the whole system is measured by the left eigenvector \(\overleftarrow{{\textbf{v}}}\left( \varvec{\theta }\right) \), while the relative economic exposure of each unit to the system caused by mobility restrictions is measured by \(\overrightarrow{{\textbf{v}}}\left( \varvec{\theta }\right) \). Recall that the economic interpretation of the eigenvectors relates to their contribution to the eigenvalue that measures the overall system stability. Eigenvectors are normalised by the 1-norm, making entries percentage values and allowing for comparison across different stability assessments. Note that individual levels of \(L_i\) and \(\rho _i\) allow for heterogeneous degrees of economic stability and lockdown measures across areas. This is a useful assumption in countries characterised by inherited structural differencesFootnote 5.
3.2.1 Special case
We present a special case with simplified model inputs to gauge the relationships among each of the main model inputs and the system stability. Let assume \({\rho }_i=\rho ,\ {\ S}_i=S,\ {\ L}_i=L,\ \forall i\). We also assume a simple function \(g({\textbf{X}})=\alpha {\textbf{X}}\) that maps commuting reduction to economic losses at the jurisdiction level in a linear fashion, governed by a scalar \(\alpha \). This means that, for every percentage point of income that can be lost by the worker commuting from i to j, \(X_{ij}\), its local jurisdiction i could face an economic loss in consumption, taxes and supply of labour up to \(\alpha X_{ij}\).
Since matrix \(\varvec{\theta }\) differs from \({\varvec{X}}\) only by a constant multiple of the identity matrix, by the shift eigenvector theorem we can rewrite the stability condition in Eq. (7) for this special case as follows:
leading to the stability condition of:
The stability condition in Eq. (9) for this special case provides direct implications for the role of the stability threshold, the level of lockdown and smartworking to stabilize the system. Given \({\textbf{X}}\), the higher \(\rho \) and S the better the chances of the system to be stable, vice versa for the level of lockdown L and the loss map parameter \(\alpha \).
4 Results
We present two main sets of results concerning the economic stability of the Italian territory, derived from the spectral model. The first ensemble estimates the stability conditions of the economic system in the pre-lockdown phase, for which we test different scenarios of lockdown policies by controlling for the level of smartworking and by differentiating among restrictions “within” and “between” jurisdictions. Within this framework, we also investigate importance and vulnerability conditions at both Province and Municipality level to emphasize local heterogeneity between the North and South of the Italian territory. The second set of results present a stability analysis grounded on actual (real) lockdown data estimated via the mobility reduction observed during the first wave of SARS-COV2 in Italy. We discuss the implications of heterogeneous reactions to national lockdown by jurisdictions characterised by different mobility patterns pre and during lockdown. Throughout the subsections, we keep the assumption of \({\rho }_i=\rho \) for all i and interpret this value as stability threshold, with \(\left[ \rho |\lambda _{max}^h\left( \varvec{\theta } \right) =1\right] \) being the minimum cost of income the jurisdictions on average need to absorb in order to keep the system stable.Footnote 6
4.1 Pre-lockdown analysis of Italian Provinces and Municipalities
We first evaluate the system stability of the Italian territory at the Province level, based on the mobility matrix \({\textbf{F}}^{Pre}\in {\mathbb {R}}^{N\times N}\), in which \(N=106\) denotes the Italian Provinces. Figure 5 shows the \({\lambda }_{max}\left( {\varvec{\theta }}^{Pre}\right) \) for different combinations of lockdown levels (L) and stability threshold (\(\rho \)). Similar to the special case in Sect. 3.2.1, we adopt a linear cost function \(g({\textbf{X}})=\alpha {\textbf{X}}\). Note that \(\alpha _i\) is quite difficult to estimate at local level because it is dependent on dimensions such as the marginal rate of consumption, the taxation level, etc. of each jurisdiction. We therefore avoid to attempt this calibration in our study and discuss the sensitivity of our outcomes when varying \(\alpha \in [0.25, 0.75]\). Depending on how the economic cost is mapped (from a very conservative \(\alpha =0.25\) to a more severe case of \(\alpha =0.75\)), the model predicts different areas of stability identified by the colder colors in the top-left quadrant of the plot. This area represents (L, \(\rho \)) combinations for which \({\lambda }_{max}\left( {\varvec{\theta }}^{Pre}\right) <1\). For an average level of lockdown of about 50%, that would translate into a reduction of half of the mobility flows.Footnote 7 Provinces face, on average, economic losses from about 10% up to 30% of the total income, depending on the parameter \(\alpha \). This is consistent with the stability analysis at the Municipality level reported in Fig. 15.
We then investigate the impact at micro level for each Province. The topological analysis of single Provinces reported in Table 1 reveals that the most important and vulnerable ones are all placed in the South of Italy. This can be explained by the fact that larger Provinces in lower income Regions (mostly in the South of Italy) show relevant mobility flows coming from neighbour Provinces due to the labour supply concentrated in just one or two local areas. As a result, those Provinces become extremely important as labour hubs. This phenomenon is clearly depicted in Fig. 6, which reports the distribution of the importance (panel A) and vulnerability (panel B) rankings at Province level where lighter colors correspond to higher rankings. Note, however, how these centrality scores refer to the network configuration of jurisdictions relative to their own economic resources, the latter being very low in the South of Italy (see, e.g., panel C). This means that the most important or vulnerable jurisdictions in our framework are identified with respect to their structure of economic interdependencies based on the flows of workers relative to their economic resources, while other economic dimensions (e.g., GDP or total income at stake) could suggest alternative rankings of importance.
The relevance of the neighborhood of each jurisdiction in the computation of the scores of importance and vulnerability is clearly highlighted by rankings reported in Fig. 7 and Table 1. Network configuration in southern regions is typically fragmented, with a few larger Provinces attracting workers from closer territories, thus determining an almost star-like system at regional level. As a consequence, such Provinces play a role similar to a hub for the local labor market, being therefore very central in our topological assessment. For instance, the Province of Potenza in the Southern region of Basilicata is by far the most critical unit, accounting for 86.8% and 75.3% of the whole system importance and vulnerability, respectively, although representing a very small proportion of total economic resources at stake (see Fig. 6 panel C and Table 1 last column).
Stability Analysis of the Italian Provinces in the Pre-Lockdown phase. The colormap represents different levels of \(\lambda _{max}\left( \varvec{\theta }\right) \) for each (\(L,\rho \)) combinations, given the vector \({\textbf{S}}\) from Sect. 3.1. The stability tipping line is plotted in black and represents all combinations of (L,\(\ \rho \)) such that \(\lambda _{max}\left( \varvec{\theta }\right) =1\). The colormap tones range from cold to warm tones, the warmer the tone the higher the value of \({\lambda }_{max}\left( \varvec{\theta }\right) \). The top-left corner identifies the region of system stability with \(\lambda _{max}\left( \varvec{\theta }\right) <1\) (cold color tones), while the bottom-right the region of instability with \({\lambda }_{max}\left( \varvec{\theta }\right) >1\) (warm color tones)
On a deeper investigation, it turns out that Potenza is one of the Province with the highest rate of “within” commuting, along with the lowest level of smartworking due to more traditional economic activities. Figure 7 panel (a) plots the weighted mobility network in the Basilicata Region showing strong commuting flows from peripheral Provinces to the few main labour hubs, the largest being in the Province of Potenza. As an example, this is compared with the Northern Region of Lombardia in panel (b), which instead is characterised by a more spread distribution of links that have lower weights and connect many more industrial areas. Hence, in Lombardia there are several large Provinces connected with each other, thus determining a very dense network in which it is more difficult to identify only one single Province that is deeply more relevant than the rest of the local territory. Hence, the local area of the Basilicata region is mainly dominated by a few Provinces, mainly Potenza and, to a lesser extent, Matera; by contrast, in Lombardia along with the Province of Milano, which is obviously relevant for the labour market, there are several other relevant nodes (e.g., Bergamo, Brescia, Monza e della Brianza, Varese, to name a few). In Sect. 4.2 we will discuss additional elements differentiating Northern and Southern regions and that contribute to such differences in the levels of centrality.
Importance and vulnerability of Italian Provinces. The figure reports the Italian Provinces colored according to their ranking of importance (panel A) and vulnerability (panel B). Panel C reports the wage ranking. Colder colors represent lower ranking in the importance/vulnerability or wage scores, warmer colors are associated to high ranked Provinces
Mobility patterns around local economic hubs. The figure reports an enlargement on mobility patterns in Basilicata and Lombardia as representative examples of commuting flows in South and North of Italy. Municipalities are reported as blue triangle, while links represent the flows between them. While mobility flows in South of Italy form a tree like structure (left panel), mobility between Municipalities in the North of Italy is much more dense and characterized by a high number of redundant links (right panel)
We also observe that the direction of the economic shocks is usually propagated from large to medium-small Provinces. As expected, when shifting from the importance to the vulnerability rankings, highly densely populated Provinces such as Bari, Napoli and Salerno move from 3rd to 5th, 4th to 16th and 5th to 13th place, respectively. Overall, we can observe from Table 1 that the most important Provinces tend to show lower levels of vulnerability, with only a few exceptions in the top positions.
Since the vast majority of commuting is recorded within Provinces, this level of aggregation could lead to loss of information. In order to avoid this effect, we also propose a more granular assessment of the impacts of mobility restrictions at the level of Italian Municipalities, where \({\textbf{F}}^{Pre}\in {\mathbb {R}}^{M\times M}\), and \(M=2790\) denotes the Italian Municipalities. We confirm that the overall system stability at the Municipality level is in line with what discussed for Provinces (see Fig. 15). We therefore focus our attention on the importance and vulnerability rankings in order to detect the emergence of specific patterns within Provinces.
Table 2 confirms the importance of Southern local units, specifically in Municipalities located in the Potenza Province, which dominates this ranking, although representing a very small proportion of total economic resources. More specifically, the scalability from Provinces to Municipalities helps to pint-point those specific Municipalities and the interactions between them in contributing to the Province level of importance and vulnerability.
The next Section investigates the role of smartworking in skewing the importance and vulnerability results towards Southern regions.
4.2 The role of smart working
To better understand the causes leading to the high rankings of territories located in the South of Italy, we turn our attention to the distribution of smartworking. On average, the national level of smartworking during the lockdown was assessed at about 33% (see (Barbieri et al., 2020)), with below average values among Southern Provinces compared to above average values in the Northern and few Central ones (e.g., Rome and neighbours). These differences are clearly represented in Fig. 8. Specifically, the left tail of the distribution in panel (a) is mainly represented by Southern Provinces as highlighted in panel (b).
We know, from Eq. (4), and the special case Eq. (9), that there exists a positive relationship between smartworking and system stability. In particular, the distribution of the different levels of smartworking has been shown to be inversely correlated with the vulnerability index (see, e.g., Table 1), skewing the results towards the South of Italy. In order to assess the impact of a more homogeneous level of smartworking across Italian territories, or alternatively considering the policy implication of aligning Southern regions to the same national average of smart working, we reassessed the vulnerability of both Provinces and Municipalities by imposing the same level \(S_i=S\) for all i. In so doing, we actually control for the local level of smartworking by reducing the national stability to a linear combination of S as in Eq. (8). By factoring for \(\rho \), we can rewrite Eq. (8) as \(\rho =\alpha L \left( 1-S\right) \lambda _{max}\left( {\textbf{X}}\right) \), which provides the minimum level of economic resources for the system to be stable, i.e. the minimum buffer needed to absorb all the losses. Setting smartworking at its average value, \(S=0.33\), considering as an example \(L=0.5\) and knowing that \(\lambda _{max}\left( {\textbf{X}}^{Pre}\right) =1\),Footnote 8 we can derive the analytical expected minimum value of \(\rho \) that is in the range 8.25–24.5% depending on the value of \(\alpha \in [0.25, 0.75]\). A more homogeneous distribution of smartworking, although targeting the same average level, leads to a higher stability of the economic system by few percentage points compared to what estimated in Sect. 4.1. This provides some valuable indication on the negative role of heterogeneous distributions of smartworking on system stability.
Smartworking across Italian Provinces and its relationship with importance and vulnerability. The figure reports in the left panel the smartworking distribution across Italian Provinces. The right panel shows the scatter plot of the importance and vulnerability scores against the level of smart. The legend displays the correlation among these quantities
When controlling for smartworking in Table 3, the skew effect towards Southern Provinces visible in Sect. 4.1 disappears. The most densely populated Provinces are now the most important ones, regardless of the geographic location and economic characteristics. This is confirmed by Fig. 9 compared to the initial lockdown scenario of Fig. 6. Those Provinces include the major Italian cities with Milano, Napoli and Roma in the top 3 positions. This result is expected by the volume of commuters in those areas, but also confirms how favouring smartworking might prevent the concentration of importance in specific areas, which are instead now structurally penalized by the absence of adequate technologies and infrastructures.
Importance and vulnerability of Italian Regions when controlling for S. The figure reports the Italian Provinces colored according to their ranking of importance (left panel) and vulnerability (right panel). Colder colors represent lower ranking in the importance/vulnerability scores, warmer colors are associated to high ranked Provinces
4.3 Between versus within mobility
As the aforementioned clustering analysis highlights (see Sect. 2), the contributions of between-Province and within-Province mobility look different from Regions to Regions. Specifically, there exists a higher mobility between Northern Italian Provinces, while higher within-Province commuting is observed in the South. This Section will investigate the effect of heterogeneous commuting patterns across the Italian territory on the economic system stability. As reported in Sect. 2, commuting in Southern Regions is heavily concentrated within the area itself while other territories, specifically the more industrially developed zones of the North of Italy, show mobility flows both within and between neighbour territories.
Competing lockdown restrictions have been put in place in Italy during the second wave of SARS-COV2, targeting more severe restrictions between jurisdictions than within. Hence, we are interested in assessing the impact of these lockdown measures to the overall stability of the system as well as the changes in importance/vulnerability rankings. To this aim we set all diagonal values \(X_{ii}=0\) for all i. Imposing a zero diagonal has the effect of eliminating any potential loss coming from commuting restrictions within the local area. The resulting matrix \({\widehat{\varvec{\theta }}}^{Pre}\) is therefore identical to \({\varvec{\theta }}^{Pre}\) with the exception of the diagonal elements and allows for direct comparison between alternative lockdown restrictions. Note, however, that this exercise does not include extra infrastructure costs and safety measures that could be required to keep the virus reproductive number at comparable levels.Footnote 9 For this reason, we focus our analysis only on the changes in the rankings among jurisdictions.
Stability Analysis of the Italian Provinces in the Pre-Lockdown phase – Between Mobility only. The colormap represents different levels of \(\lambda _{max}\left( \varvec{\theta }\right) \) for each (\(L,\rho \)) combinations, given the vector \({\textbf{S}}\) from Sect. 3.1. The stability tipping line is plotted in black and represents all combinations of (L,\(\ \rho \)) such that \(\lambda _{max}\left( \varvec{\theta }\right) =1\). The colormap tones range from cold to warm tones, the warmer the tone the higher the value of \({\lambda }_{max}\left( \varvec{\theta }\right) \). The top-left corner identifies the region of system stability with \(\lambda _{max}\left( \varvec{\theta }\right) <1\) (cold color tones), while the bottom-right the region of instability with \({\lambda }_{max}\left( \varvec{\theta }\right) >1\) (warm color tones)
We briefly show the stability/instability areas of the system when controlling for the mobility within Provinces in Fig. 10. This is achieved by plotting \(\lambda _{max}\left( {\widehat{\varvec{\theta }}}^{Pre}\right) \) values for different combinations of lockdown levels (\({\textbf{L}}\)) and economic buffer (\(\varvec{\rho }\)). In line with the previous assessments, areas of stability are identified by colder colors in the top-left quadrant of the plot while instability areas by warmer colors in the bottom-right. For the same indicative level of lockdown of 50%, on average Provinces would have to sustain economic losses from 4% to about 10% of total income to preserve stability, depending on the economic cost map (see Fig. 16 for the stability analysis at Municipality level). As expected, the system appears much more stable at different (L, \(\rho \)) combinations than the more conservative framework of Sect. 4.1 that penalises both within and between jurisdictions mobility. Nevertheless, as already mentioned, these costs do not account for other infrastructure and safety measures required to keep SARS-COV2 reproductive numbers at the same level among different lockdown scenarios. It is therefore up to policy makers to evaluate cost-benefit trade-offs of each of these alternative policy restrictions for specific local areas.
An interesting comparison would be on the importance/vulnerability rankings when assessing between-Province-only lockdown scenarios, as presented in Table 4. As predicted by the mobility patterns discussed above, important Provinces responsible for propagating economic losses are those in the Northern part of Italy, specifically in Lombardia and Piemonte Regions. Those are also the areas accounting for the largest proportion of total economic resources. Lockdown measures that only target mobility between Provinces can have a limited impact on the risk of contagion in Southern regions while Northern areas, where mobility patterns between Provinces are more developed, would be more exposed to economic losses. In line with the other lockdown scenarios presented in Sect. 4.1, the economic shocks tend to propagate from the largest Provinces to the others in the same Region. Indeed, Milano is the most important jurisdiction but ranks only 5th in vulnerability. For the other Provinces, mixed results are observed on the rankings within the top 20 most important ones.
The scalability of our mobility network allows us to test alternative lockdown restrictions at different jurisdiction levels, specifically Province and Municipality ones. We can thus relax mobility restrictions only within Municipalities, while still maintaining restrictions between different Municipalities. We can consider this scenario as a mid-ground approach that would still allow for a certain degree of mobility within the same local jurisdiction. On the other hand, this policy would disadvantage those Provinces with high degree of inner mobility between their Municipalities.
Since Toscana is one of the Italian Region with the largest volume of mobility between neighbouring Municipalities (see Sect. 2), lockdown policies targeting only commuting between Municipalities, would make this Region the most important and vulnerable local area in the country, with Florence as a key local hub for commuting and the most important Province in this framework (see Table 5). This compares to what assessed in the previous scenario of Table 4 in which Florence was barely making the top 20st more important Provinces. More generally, Municipalities in Toscana dominate by far the top 100 rankings under this scenario. This is also confirmed by Fig. 11 that presents the connectivity of Provinces in Toscana (right panel) as well as plotting the importance and vulnerability of Provinces on the Italian map (left panel) in this scenario. The former clearly underlines the characteristics of jurisdictions in Toscana with large volume of between mobility and high levels of clustering.
Province Between-Mobility Importance and Vulnerability. The figure shows, in the left panel, the importance and vulnerability scores associated to each Municipally relative to between mobility patterns only. Square size is proportional to the importance of the Municipality while color, from warm to cold, represent the vulnerability. The right panel displays an enlargement of the mobility flow in the Toscana Region from which the high number of clusters is visible
More in general, the above discussed outcomes are likely the result of the impact of different lockdown protocols to the fragility of local areas that are characterised by different mobility patterns. These features would support the use of bespoke lockdown measures that would reflect the specific characteristics of each Region as the one-rule-fit-all approach can heavily discriminate between territories so heterogeneous.
4.4 Lockdown assessment
This Section investigates the impact of the actual lockdown measures imposed to Italian territories during the first wave of SARS-COV2. By comparing the change of mobility patterns before (\({\textbf{F}}^{pre}\)) and during the lockdown (\({\textbf{F}}^{lock}\)), we can derive the vector \({\textbf{L}}\) and therefore assess the estimated economic losses and compare with those predicted in the pre-lockdown analysis of Sect. 4.1. Figure 12 reports the distribution of the estimated \({\textbf{L}}\). The relative change in mobility patterns (pre vs. post lockdown measures) can net out endogenous structural weaknesses within each region. We, however, acknowledge this potential issue of endogeneity on local infrastructure differences that can affect lockdown restrictions and stability outcomes and leave further calibration to individual dynamical properties to future works.
Distribution of Lockdown Restrictions on Commuting in Italian Provinces. The histogram displays the distribution of the percentage reduction in commuting caused by the lockdown measures on the Italian Provinces. It is computed by comparing mobility data before and after the implementation of the restrictions
The prediction of economic consequences of the Italian lockdown during the first wave of SARS-COV2 is plotted in Fig. 13. Depending on the cost parameter \(\alpha \), we predict an average economic loss in the range of about 15% to 40% of the total income among Italian Provinces, which is few percentage points above the equivalent estimation with a constant level lockdown of 60–65% to all jurisdiction (see Fig. 5). Hence, the analysis of economic losses due to lockdown restrictions highlights the severe economic consequences of the mobility restrictions put in place to limit the spread of the virus, with additional emphasis on heterogeneous changes in mobility that, similar to what we observed with smartworking, exacerbate instability.
In line with the results derived from the pre-lockdown analysis, Southern Provinces are the ones facing higher vulnerability to lockdown restrictions as shown in Table 6. We also learn from the lockdown analysis that they are showing the largest reduction in mobility. In fact, although the same lockdown restrictions have been implemented in all Italian regions during the first wave of the contagion, the actual change in mobility can shed a light on how those restrictions have been effectively implemented and monitored. They also reflect the proportion of workers that were providing essential services as lockdown restrictions rolled out. Specifically, Provinces in the Southern regions of Calabria and Sicily were the most affected, showing extremely low values of smartworking and lockdown percentages above the national average. In addition, similar to all other scenarios, larger Provinces are more likely to spread losses to medium-small ones relative to their own economic resources. Note, however, that these areas represent a very small proportion of total economic resources as shown in Table 6 last column. The unequal distribution of actual mobility reduction towards the South of Italy, which was already top ranked in the importance and vulnerability scores in our pre-lockdown analysis, has contributed to boost the level of instability of the whole national system even further.
5 Conclusions and policy implications
We propose a network-based spectral method to assess the spread of contagion of economic losses coming from restrictions on the commuting mobility network. The latter is provided by Facebook Disease Prevention maps covering about 3000 Italian Municipalities of about four million individuals over the period from February 24th to March 23rd 2020. Our preliminary network analysis shows complex mobility patterns differentiating jurisdictions across the country. We observe higher degree of mobility flows between Northern areas compared to those in the South of Italy. The latter are characterised by mobility mainly concentrated within the jurisdiction itself. When combined mobility data with socio-economic variables provided by the Italian National Institute of Statistics (ISTAT), the Italian Ministry of Economy and Finance and the Bank of Italy, we notice a heterogeneous distribution of smartworking that penalizes Southern regions during lockdown. This evidence, combined with the concentration of commuting flows on fewer industrial hubs in the same regions, makes Southern jurisdictions quite dependent on local commuting to support economic outputs. The stability analysis confirms our intuition towards the importance of Southern jurisdictions in contributing to instability. Specifically, we observe the Region of Basilicata that dominates importance and vulnerability rankings as a result of the labour supply concentrated in very few local central areas with the lowest level of smartworking in the country.
The overall system expected loss is estimated in the range of 10–30% of total income from commuting depending on the economic assumptions. We then investigate the role of smartworking and alternative lockdown policies to understand the impact of mobility patterns to the overall system stability as well as the contribution, in terms of network importance and vulnerability, to instability. Across all alternative restriction policies, the direction of the economic shocks is usually propagated from the largest to other medium-small jurisdictions as expected (large areas are the major labor supplier of the Regions). When controlling for smartworking, the skew effect penalising Southern Provinces disappears, making the most densely populated Provinces the most important ones, regardless of the geographic location and economic characteristics, while the aggregate expected loss of the system is also slightly improved by few percentage points, supporting a more homogeneous distribution of smartworking nationwide.
Our estimates of the economic losses induced by the lockdown phase confirm how mobility restrictions, put in place to contain the spread of the contagion during the first wave, penalized more those territories located in the South of the country. These findings are in line with official statistics released by Bank of Italy (Banca d’Italia, 2020) which indicate how, in the first semester of 2020, the highest reduction of employed people was registered in Calabria (\(-\)4.8%), with the South accounting for an aggregate reduction equal to \(-\)2.6%, compared to the North of the country (\(-\)1.5%) and the average level of Italy (\(-\)1.7%). Hence, the effects of the lockdown affected most those areas already very fragile from a socio-economic perspective, with the South characterized by an unemployment rate in the first quarter of 2020 equal to 16.3%, much higher than the average of the country (9.2%). These Southerner areas are much smaller in terms of economic resources compared to the Northern areas and therefore might be overlooked if assessed in absolute economic terms.
Our results suggest that important emphasis should be given to coordinate the development of smartworking solutions that can mitigate the spread of economic losses among local units, especially when this distribution discriminates certain Regions. For instance, promoting targeted policies aimed at limiting these differences would have positive results for the system stability. Finally, the heterogeneous reduction in mobility flows should inform policy makers regarding the most cost-effective implementation of lockdown measures. Since economic integration implies that a more homogeneous impact on mobility favours system stability, coordinated policies tailored at the regional level should be promoted to balance the spreading of the pandemic with the need to sustain economic output. When looking at alternative lockdown restrictions that are closely related to those proposed to contain the second wave of SARS-COV2 in Italy, we notice a shift in economic risk towards Northern jurisdictions. Specifically, policy restrictions that treat mobility between jurisdictions more severely that within can have notably different economic consequences in different parts of the country. Our results are confirmed by further stability analysis using actual mobility restrictions data during lockdown, echoing the importance of supporting lockdown policies that, although aiming at containing the spread of the virus, are tailored to the complex structure of mobility flows and their contribution to local economic outputs.
There are some limitations in this study. First, our sample does not include commuting flows of those workers that did not enable the social network platform to map movements, thus potentially bias our findings in terms of generational and geographical coverage. Second, we impose economic impacts in terms of losses of salary as measured by the local administrative unit average value, which may hidden relevant heterogeneity in the distribution especially when dealing with commuter workers. Third, the estimates of economic losses do not take into account the presence of different values of the economic local buffers \(\rho _i\), which may indicate pre-existing local socio-economic fragility. The assessment of the economic consequences should also consider the impact of fiscal interventions and local policies to support economic output. Future works may deal with these aspects to calibrate cost-benefits analysis of competing NPIs on real data.
Notes
Geographical and georeferenced polygons of size 0.6 km \(\times \) 0.6 km.
In Italy, for example, the historical divide between the North and the South and the inner fragilities suffered by Italian underdeveloped areas are well documented (see among others (Vecchi, 2017)).
By assuming that the resilience of the Italian labor market is homogeneous across areas, we potentially oversight the impact of lockdown measures in certain areas that, for example, might rely on an underground economy (which accounts for almost 12% of the Italian GDP), and that some 20% of workers in specific areas are undocumented (Giovannini, 2010; Morvillo, 2012) Since the estimation of this undocumented portion of the labor market goes beyond the scope of this paper, we leave this development to future works.
In reality, lockdown restrictions affect industry sectors differently depending on the policy aim. A clear example is related to workers providing essential services for which restrictions did not apply.
Since we assume that the probability of mobility between jurisdictions maps all workers, the row sum of matrix \({\textbf{X}}\) tends to be equal to 1 for all jurisdictions, making the largest eigenvalue equal to 1.
For instance, Spelta et al. (2020) propose a scenario based analysis to evaluate economic impacts including hospitalization costs when salary losses refer to both mobility restrictions and contagion.
References
Aleta, A., Martín-Corral, D., & Pastore y Piontti, A., Ajelli, M., Litvinova, M., Chinazzi, M., Dean, N.E., Halloran, M.E., Longini Jr, I. M., Merler, S., Pentland, A., Vespignani, A., Moro, E., & Moreno, Y. (2020). Modelling the impact of testing, contact tracing and household quarantine on second waves of COVID-19. Nature Human Behaviour, 4, 964–971. https://doi.org/10.1038/s41562-020-0931-9
Alfaro, L., Faia, E., Lamersdorf, N., & Saidi, F. (2020). Social interactions in pandemics: Fear, altruism, and reciprocity. Technical Report, National Bureau of Economic Research.
Altig, D., Baker, S., Barrero, J. M., Bloom, N., Bunn, P., Chen, S., Davis, S. J., Leather, J., Meyer, B., Mihaylov, E., et al. (2020). Economic uncertainty before and during the covid-19 pandemic. Journal of Public Economics, 191, 104274.
Baker, S. R., Bloom, N., Davis, S. J., & Terry, S. J. (2020). Covid-induced economic uncertainty. Technical Report, National Bureau of Economic Research.
Banca d’Italia. (2020). Economie regionali: L’economia delle regioni italiane, dinamiche recenti e aspetti strutturali, Economie Regionali series 22.
Barbieri, T., Basso, G., & Scicchitano, S. (2020). Italian workers at risk during the covid-19 epidemic, Banca d’Italia occasional paper no. 569. https://doi.org/10.2139/ssrn.3572065.
Bardoscia, M., Battiston, S., Caccioli, F., & Caldarelli, G. (2017). Pathways towards instability in financial networks, Nature Communications,8, 1–7. https://doi.org/10.1038/ncomms14416. arXiv:1602.05883
Battiston, S., Caldarelli, G., D’Errico, M., & Gurciullo, S. (2016). Leveraging the network: A stress-test framework based on DebtRank. Statistics and Risk Modeling. https://doi.org/10.1515/strm-2015-0005. arXiv:1503.00621
Bonaccorsi, G., Pierri, F., Cinelli, M., Flori, A., Galeazzi, A., Porcelli, F., Schmidt, A. L., Valensise, C. M., Scala, A., Quattrociocchi, W., et al. (2020). Economic and social consequences of human mobility restrictions under COVID-19. Proceedings of the National Academy of Sciences, 117, 15530–15535. https://doi.org/10.1073/pnas.2007658117
Bonaccorsi, G., Pierri, F., Scotti, F., Flori, A., Manaresi, F., Ceri, S., & Pammolli, F. (2021). Socioeconomic differences and persistent segregation of Italian territories during covid-19 pandemic. Scientific Reports, 11, 1–15.
Bucci, M., Ivaldi, G., Messina, G., Moller, L., & Gennari, E. (2021). I divari infrastrutturali in italia: una misurazione caso per caso [infrastructure gaps in Italy: A case-by-case measurement], Bank of Italy Occasional Paper.
Carvalho, V. M., Garcia, J. R., Hansen, S., Ortiz, A., Rodrigo, T., & Mora, J. V. R. (2020). Tracking the COVID-19 Crisis with High-Resolution Transaction Data. Technical Report, CEPR Discussion Paper No. DP14642.
Chang, S., Pierson, E., Koh, P. W., Gerardin, J., Redbird, B., Grusky, D., & Leskovec, J. (2020). Mobility network models of COVID-19 explain inequities and inform reopening. Nature. https://doi.org/10.1038/s41586-020-2923-3
Chetty, R., Friedman, J. N., Hendren, N., Stepner, M., et al. (2020). How did covid-19 and stabilization policies affect spending and employment? a new real-time economic tracker based on private sector data. Technical Report, National Bureau of Economic Research.
Chinazzi, M., Davis, J. T., Ajelli, M., Gioannini, C., Litvinova, M., Merler, S., & Pastore y Piontti, A., Mu, K., Rossi, L., Sun, K., Viboud, C., Xiong, X., Yu, H., Halloran, M. E., Longini, I. M., Vespignani, A. (2020). The effect of travel restrictions on the spread of the 2019 novel coronavirus (COVID-19) outbreak. Science. https://doi.org/10.1126/science.aba9757
Coibion, O., Gorodnichenko, Y., & Weber, M. (2020). The cost of the covid-19 crisis: Lockdowns, macroeconomic expectations, and consumer spending. Technical Report, National Bureau of Economic Research.
Davies, N. G., Kucharski, A. J., Eggo, R. M., Gimma, A., Edmunds, W. J., Jombart, T., O’Reilly, K., Endo, A., Hellewell, J., Nightingale, E. S., et al. (2020). Effects of non-pharmaceutical interventions on COVID-19 cases, deaths, and demand for hospital services in the UK: a modelling study. The Lancet Public Health. https://doi.org/10.1016/S2468-2667(20)30133-X
Deb, P., Furceri, D., Ostry, J. D., Tawk, N. (2020). The economic effects of COVID-19 containment measures. Technical Report, CEPR Discussion Paper No. DP15087.
Della Rossa, F., Salzano, D., Di Meglio, A., De Lellis, F., Coraggio, M., Calabrese, C., Guarino, A., Cardona-Rivera, R., De Lellis, P., Liuzza, D., Lo Iudice, F., Russo, G., & di Bernardo, M. (2020). A network model of Italy shows that intermittent regional strategies can alleviate the COVID-19 epidemic. Nature Communications, 11, 5106. https://doi.org/10.1038/s41467-020-18827-5
Demirgüç-Kunt, A., Lokshin, M., & Torre, I. (2021). The sooner, the better: The economic impact of non-pharmaceutical interventions during the early stage of the covid-19 pandemic, Economics of Transition and Institutional. Change, 29, 551–573.
Dietrich, A. M., Kuester, K., Müller, G. J., Schoenle, R. (2022). News and uncertainty about covid-19: Survey evidence and short-run economic impact. Journal of Monetary Economics.
Eichenbaum, M. S., Rebelo, S., Trabandt, M. (2020). Epidemics in the Neoclassical and New Keynesian Models, Working Paper number27430, National Bureau of Economic Research. http://www.nber.org/papers/w27430. https://doi.org/10.3386/w27430.
Eisenberg, L., & Noe, T. H. (2001). Systemic risk in financial systems. Management Science, 47, 236–249. https://doi.org/10.1287/mnsc.47.2.236.9835
Fagiolo, G. (2007). Clustering in complex directed networks. Physical Review E, 76, 026107.
Fernández-Villaverde, J., Jones, C. I. (2020). Macroeconomic Outcomes and COVID-19: A Progress Report, Working Paper number28004, National Bureau of Economic Research. http://www.nber.org/papers/w28004. https://doi.org/10.3386/w28004.
Flaxman, S., Mishra, S., Gandy, A., Unwin, H. J. T., Mellan, T. A., Coupland, H., Whittaker, C., Zhu, H., Berah, T., Eaton, J. W., et al. (2020). Estimating the effects of non-pharmaceutical interventions on COVID-19 in Europe. Nature. https://doi.org/10.1038/s41586-020-2405-7
Gajpal, Y., Appadoo, S., Shi, V., & Hu, G. (2022). Optimal multi-stage group partition for efficient coronavirus screening. Annals of Operations Research 1–17.
Gauthier, C., Lehar, A., & Souissi, M. (2012). Macroprudential capital requirements and systemic risk. Journal of Financial Intermediation, 21, 594–618. https://doi.org/10.1016/j.jfi.2012.01.005
Giovannini, E. (2010). Dossier (allegato 1): l’economia sommersa: stime nazionali e regionali, Technical Report, Audizione del Presidente dell’Istituto nazionale di statistica Enrico Giovannini presso la Commissione parlamentare di vigilanza sull’Anagrafe tributaria Roma, 22 luglio 2010, ISTAT.
Giudici, P., Pagnottoni, P., & Spelta, A. (2023). Network self-exciting point processes to measure health impacts of COVID-19. Journal of the Royal Statistical Society Series A: Statistics in Society, 186, 401–421. https://doi.org/10.1093/jrsssa/qnac006
Glaeser, E. L., Gorback, C. S., & Redding, S. J. (2020). How much does COVID-19 increase with mobility? Technical Report, National Bureau of Economic Research: Evidence from New York and four other US cities.
Goolsbee, A., & Syverson, C. (2020). Fear, lockdown, and diversion: Comparing drivers of pandemic economic decline 2020. Journal of Public Economics, 193, 104311.
Grady, D., Thiemann, C., & Brockmann, D. (2012). Robust classification of salient links in complex networks. Nature Communications, 3, 1–10.
Grantz, K. H., Meredith, H. R., Cummings, D. A., Metcalf, C. J. E., Grenfell, B. T., Giles, J. R., Mehta, S., Solomon, S., Labrique, A., Kishore, N., et al. (2020). The use of mobile phone data to inform analysis of covid-19 pandemic epidemiology, Nature. Communications, 11, 1–8.
Gregory, V., Menzio, G., & Wiczer, D. G. (2020). Pandemic Recession: L or V-Shaped? Technical Report, National Bureau of Economic Research.
Guan, D., Wang, D., Hallegatte, S., Davis, S. J., Huo, J., Li, S., Bai, Y., Lei, T., Xue, Q., Coffman, D., Cheng, D., Chen, P., Liang, X., Xu, B., Lu, X., Wang, S., Hubacek, K., & Gong, P. (2020). Global supply-chain effects of COVID-19 control measures. Nature Human Behaviour, 4, 577–587. https://doi.org/10.1038/s41562-020-0896-8
Guerrieri, V., Lorenzoni, G., Straub, L., & Werning, I. (2020). Macroeconomic implications of COVID-19: Can negative supply shocks cause demand shortages? Technical Report, National Bureau of Economic Research.
Haug, N., Geyrhofer, L., Londei, A., Dervic, E., Desvars-Larrive, A., Loreto, V., Pinior, B., Thurner, S., & Klimek, P. (2020). Ranking the effectiveness of worldwide COVID-19 government interventions. Nature Human Behaviour. https://doi.org/10.1038/s41562-020-01009-0
Heath, A., Kelly, G., Manning, M., Markose, S., & Shaghaghi, A. R. (2014). CCPs and network stability in OTC derivatives markets. Journal of Financial Stability, 27(2014), 217–233. https://doi.org/10.1016/j.jfs.2015.12.004
Holland, P. W., & Leinhardt, S. (1971). Transitivity in structural models of small groups. Comparative Group Studies, 2, 107–124.
Hsiang, S., Allen, D., Annan-Phan, S., Bell, K., Bolliger, I., Chong, T., Druckenmiller, H., Huang, L. Y., Hultgren, A., & Krasovich, E. (2020). The effect of large-scale anti-contagion policies on the COVID-19 pandemic. Nature. https://doi.org/10.1038/s41586-020-2404-8
ISTAT. (2006). The infrastructure in Italy. Technical Report, ISTAT. http://www3.istat.it/dati/catalogo/20060512_00/inf_0607_infrastrutture_in_Italia.pdf.
Jay, J., Bor, J., Nsoesie, E. O., Lipson, S. K., Jones, D. K., Galea, S., & Raifman, J. (2020). Neighbourhood income and physical distancing during the COVID-19 pandemic in the United States. Nature Human Behaviour. https://doi.org/10.1038/s41562-020-00998-2
Kaplan, G., Moll, B., Violante, G. L. (2020). The Great Lockdown and the Big Stimulus: Tracing the Pandemic Possibility Frontier for the U.S., Working Paper number27794, National Bureau of Economic Research. https://doi.org/10.3386/w27794.
Kuchler, T., Russel, D., & Stroebel, J. (2020). The geographic spread of COVID-19 correlates with structure of social networks as measured by Facebook. Technical Report, National Bureau of Economic Research.
Kumar, A., Choi, T.-M., Wamba, S. F., Gupta, S., & Tan, K. H. (2021). Infection vulnerability stratification risk modelling of covid-19 data: A deterministic seir epidemic model analysis. Annals of Operations Research 1–27.
Kumar, S., Xu, C., Ghildayal, N., Chandra, C., & Yang, M. (2021) Social media effectiveness as a humanitarian response to mitigate influenza epidemic and covid-19 pandemic. Annals of Operations Research, 1–29.
Lau, H., Khosrawipour, V., Kocbach, P., Mikolajczyk, A., Schubert, J., Bania, J., & Khosrawipour, T. (2020). The positive impact of lockdown in Wuhan on containing the covid-19 outbreak in China. Journal of Travel Medicine, 27, taaa037.
Maas, P., Iyer, S., Gros, A., Park, W., McGorman, L., Nayak, C., & Dow, P. A. (2019) Facebook disaster maps: Aggregate insights for crisis response and recovery. In Proceedings of the 16th international conference on information systems for crisis response and management (ISCRAM), Valencia, Spain.
Maier, B. F., & Brockmann, D. (2020). Effective containment explains subexponential growth in recent confirmed COVID-19 cases in China. Science, 368, 742–746.
Markose, S. M., Giansante, S., Eterovic, N. A., & Gatkowski, M. (2021). Early warning of systemic risk in global banking: Eigen-pair R number for financial contagion and market price-based methods. Annals of Operations Research. https://doi.org/10.1007/s10479-021-04120-1
Markose, S., Giansante, S., & Shaghaghi, A. R. (2012). ‘Too interconnected to fail’ financial network of US CDS market: Topological fragility and systemic risk. Journal of Economic Behavior and Organization, 83, 627–646.
Martin, A., Markhvida, M., Hallegatte, S., & Walsh, B. (2020). Socio-economic impacts of covid-19 on household consumption and poverty. Economics of Disasters and Climate Change, 4, 453–479.
May, R. M. (1972). Will a large complex system be stable? Nature, 238, 413–414.
May, R. M. (1974). Stability and complexity in model ecosystems. Princeton: Princeton University Press.
Morvillo, C. (2012). Evoluzione delle determinanti dell’economia sommersa: analisi panel di regioni italiane, Nota tecnica 1–2 agosto 2016. Technical Report, Ministero dell’economia e delle finanze, dipartimento del tesoro.
Newman, M. E. J. (2010). Networks. New York: Oxford University Press.
Pagnottoni, P., Spelta, A., Pecora, N., Flori, A., & Pammolli, F. (2021). Financial earthquakes: SARS-COV-2 news shock propagation in stock and sovereign bond markets. Physica A: Statistical Mechanics and its Applications, 582, 126240.
Polyakova, M., Kocks, G., Udalova, V., & Finkelstein, A. (2020). Initial economic damage from the COVID-19 pandemic in the United States is more widespread across ages and geographies than initial mortality impacts. Proceedings of the National Academy of Sciences, 117, 27934–27939. https://doi.org/10.1073/pnas.2014279117
Presidente del Consiglio dei Ministri, Ulteriori disposizioni attuative del decreto-legge 23 febbraio 2020, n. 6, recante misure urgenti in materia di contenimento e gestione dell’emergenza epidemiologica da covid-19, Gazzetta Ufficiale, 62 (2020).
Presidente del Consiglio dei Ministri, Ulteriori disposizioni attuative del decreto-legge 25 marzo 2020, n. 19, convertito, con modificazioni, dalla legge 25 maggio 2020, n. 35, recante misure urgenti per fronteggiare l’emergenza epidemiologica da covid-19, e del decreto-legge 16 maggio 2020, n. 33, convertito, con modificazioni, dalla legge 14 luglio 2020, n. 74, recante ulteriori misure urgenti per fronteggiare l’emergenza epidemiologica da covid-19, Gazzetta Ufficiale, 275 (2020).
Rogers, L. C. G., & Veraart, L. A. M. (2013). Failure and rescue in an interbank network. Management Science, 59, 882–898. https://doi.org/10.1287/mnsc.1120.1569
Saltelli, A., Bammer, G., Bruno, I., Charters, E., Di Fiore, M., Didier, E., Nelson Espeland, W., Kay, J., Lo Piano, S., Mayo, D., et al. (2020). Five ways to ensure that models serve society: a manifesto. Nature, 582, 482–484. https://doi.org/10.1038/d41586-020-01812-9
Sarkodie, S. A., & Owusu, P. A. (2021). Global assessment of environment, health and economic impact of the novel coronavirus (covid-19). Environment, Development and Sustainability, 23, 5005–5015.
Schlosser, F., Maier, B. F., Jack, O., Hinrichs, D., Zachariae, A., & Brockmann, D. (2020). Covid-19 lockdown induces disease-mitigating structural changes in mobility networks. Proceedings of the National Academy of Sciences.
Schuldenzucker, S., Seuken, S., & Battiston, S. (2020). Default ambiguity: Credit default swaps create new systemic risks in financial networks. Management Science. https://doi.org/10.2139/ssrn.3043708
Schwartz, J. (2018). Bing maps tile system. https://docs.microsoft.com/en-us/bingmaps/articles/bing-maps- tile-system.
Sheridan, A., Andersen, A. L., Hansen, E. T., & Johannesen, N. (2020). Social distancing laws cause only small losses of economic activity during the covid-19 pandemic in Scandinavia. Proceedings of the National Academy of Sciences, 117, 20468–20473.
Smolyak, A., Bonaccorsi, G., Flori, A., Pammolli, F., & Havlin, S. (2021). Effects of mobility restrictions during covid19 in Italy. Scientific Reports, 11, 1–15.
Spelta, A., Flori, A., Pierri, F., Bonaccorsi, G., & Pammolli, F. (2020). After the lockdown: Simulating mobility, public health and economic recovery scenarios. Scientific Reports, 10, 1–13.
Spelta, A., & Pagnottoni, P. (2021). Mobility-based real-time economic monitoring amid the Covid-19 pandemic. Scientific Reports, 11, 13069.
van Der Voet, J. (2021) Policy preferences in response to negative economic prospects of covid-19: A survey-experiment among local politicians in four European countries, Public Administration.
Vecchi, G. (2017). Measuring wellbeing: A history of Italian living standards. https://doi.org/10.1111/ecaf.12256
Watts, D. J., & Strogatz, S. H. (1998). Collective dynamics of ‘small-world’ networks. Nature, 393, 440–442.
Xiong, C., Hu, S., Yang, M., Luo, W., & Zhang, L. (2020). Mobile device data reveal the dynamics in a positive relationship between human mobility and COVID-19 infections. Proceedings of the National Academy of Sciences, 117, 27087–27089. https://doi.org/10.1073/pnas.2010836117
Zhang, J., Litvinova, M., Liang, Y., Wang, Y., Wang, W., Zhao, S., Wu, Q., Merler, S., Viboud, C., Vespignani, A., Ajelli, M., & Yu, H. (2020). Changes in contact patterns shape the dynamics of the COVID-19 outbreak in China. Science,368, 1481–1486. https://doi.org/10.1126/science.abb8001. arXiv:3235.0060.
Funding
Open access funding provided by Universitá degli Studi di Pavia within the CRUI-CARE Agreement.
Author information
Authors and Affiliations
Corresponding author
Ethics declarations
Ethical approval
This article does not contain any studies with human participants or animals performed by any of the authors.
Conflict of interest
Simone Giansante declares that he has no conflict of interest. Andrea Flori declares that he has no conflict of interest. Alessandro Spelta declares that he has no conflict of interest.
Additional information
Publisher's Note
Springer Nature remains neutral with regard to jurisdictional claims in published maps and institutional affiliations.
Appendices
A Network dismantling robustness
In this Appendix we check how the mobility data has reacted to different forms of lockdown. In particular we aim at discovering whether the network backbone has undergone changes after lockdowns. In so doing, we introduce the concept of link salience (Grady et al., 2012). Salience has been shown to be a robust approach to classifying network elements based on a consensus estimate of all nodes. Although a number of filtering techniques have been proposed in many contexts, such as statistical tests, Minimum Spanning Tree algorithms or Planar Maximally Filtered Graphs, all of these approaches come with several drawbacks. This is due to the fact that link weights are typically continuously distributed, thus making thresholding not only arbitrary but also problematic, as key properties of the reduced networks can sensitively depend on the chosen threshold. To cope with this, we employ link salience, which can successfully classify links into distinct groups without external parameters or arbitrary thresholds. The central idea of this approach is based on the notion of the average shortest path tree, which is the collection of shortest paths from a node to all other nodes in the network. This measure summarizes the most effective routes from the reference node to the rest of the network and can be used to derive the salience adjacency matrix, namely, the backbone structure mobility network.
Link salience is based on the concept of effective proximity \(d_{i,j}\) defined by the reciprocal coupling strength \(d_{i,j}=1 / w_{i,j}\). Effective proximity captures the intuitive notion that strongly (weakly) coupled nodes are close to (distant from) each other. It also provides one way to define the length of a path p that connects two terminal nodes \(\left( n_{1}, n_{k}\right) \) and consists of a sequence of \(K-1\) intermediate nodes \(n_{i}\), and connections \(w_{n_{i} n_{i+1}}>0\). The shortest path minimizes the total effective distance \(l=\sum _{i=1}^{K-1} \mathrm {~d}_{n_{i} n_{i+1}}\) and can be interpreted as the most efficient route between its terminal nodes. Given the notion of shortest path that originates at node k and terminates at node l, one can introduce the indicator function:
A shortest path tree (SPT) \(\varvec{\Upsilon }(k)\) rooted at node k can be represented as a matrix with elements
and the salience \(\textrm{s}_{\textrm{i,j}}\) of link \(\textrm{i} \rightarrow \textrm{j}\) is given by
The salience \({\varvec{S}} = [s_{i,j}]\) as defined by Eq. (12) permits an intuitive definition of the skeleton of a network. We thus extract the skeleton of the Italian mobility network pre and during the lockdown to see whether this policy has effectively changed the distribution of the commuters among municipalities. Results are shown in Fig. 14, which reports the weights distributions of the salient, non-salient and excluded links for the pre-lockdown phase (upper panel) and lockdown phase (lower panel). Results suggest a general decreasing in the number of commuters (link weights) despite the distributions retains approximately the same shape in the two sub-sample. Only the salient link weight distribution displays a decreasing right tail thus suggesting the efficiency of the policy in dismantling the commuter network backbone.
B Supplementary figures
Stability analysis of Italian municipalities pre-lockdown. The colormap represents different levels of \({\lambda }_{max}\left( \varvec{\theta }\right) \)for each (L,\(\ \rho \)) combinations, given the vector Sfrom Section 4. The stability tipping line is plotted in black and represents all combinations of (L,\(\ \rho \)) such that \({\lambda }_{max}\left( \varvec{\theta }\right) \mathbf {=}{\textbf{1}}\). The colormap tones ranges from cold to warm tones, the warmer the tone the higher the value of \({\lambda }_{max}\left( \varvec{\theta }\right) \). The top-left corner identifies the region of system stability with \({\lambda }_{max}\left( \varvec{\theta }\right) \mathbf {<}{\textbf{1}}\) (cold color tones), while the bottom-right the region of instability with \({\lambda }_{max}\left( \varvec{\theta }\right) \mathbf {>}{\textbf{1}}\) (warm color tones)
Stability analysis of Italian municipalities pre-lockdown—between mobility only. The colormap represents different levels of \({\lambda }_{max}\left( \varvec{\theta }\right) \) for each (L,\(\ \rho \)) combinations, given the vector Sfrom Section 4. The stability tipping line is plotted in black and represents all combinations of (L,\(\ \rho \)) such that \({\lambda }_{max}\left( \varvec{\theta }\right) \mathbf {=}{\textbf{1}}\). The colormap tones ranges from cold to warm tones, the warmer the tone the higher the value of \({\lambda }_{max}\left( \varvec{\theta }\right) \). The top-left corner identifies the region of system stability with \({\lambda }_{max}\left( \varvec{\theta }\right) \mathbf {<}{\textbf{1}}\) (cold color tones), while the bottom-right the region of instability with \({\lambda }_{max}\left( \varvec{\theta }\right) \mathbf {>}{\textbf{1}}\) (warm color tones)
Rights and permissions
Open Access This article is licensed under a Creative Commons Attribution 4.0 International License, which permits use, sharing, adaptation, distribution and reproduction in any medium or format, as long as you give appropriate credit to the original author(s) and the source, provide a link to the Creative Commons licence, and indicate if changes were made. The images or other third party material in this article are included in the article’s Creative Commons licence, unless indicated otherwise in a credit line to the material. If material is not included in the article’s Creative Commons licence and your intended use is not permitted by statutory regulation or exceeds the permitted use, you will need to obtain permission directly from the copyright holder. To view a copy of this licence, visit http://creativecommons.org/licenses/by/4.0/.
About this article
Cite this article
Giansante, S., Flori, A. & Spelta, A. On the fragility of the Italian economic territories under SARS-COV2 lockdown policies. Ann Oper Res (2023). https://doi.org/10.1007/s10479-023-05635-5
Received:
Accepted:
Published:
DOI: https://doi.org/10.1007/s10479-023-05635-5