Abstract
Zero insulators directly reduce the electrical performance of insulator strings, affecting the safe and reliable operation of transmission lines. The grounding current of the insulator string contains the characteristic information of insulator electrical performance. Extracting the characteristic information of grounding current can realize the detection of zero value insulator, which is of great significance to ensure the safe operation of transmission lines. In this paper, the composite insulator used on ultra-high-voltage (UHV) lines is studied to analyze the influence of zero insulators on the electric field distribution. Furthermore, the corresponding characteristics of the zero insulators at different locations are extracted. The fitting relationship between the characteristic quantity and the zero insulator position is analyzed. The effective characteristics of grounding current are selected as training sample to establish the zero insulator position prediction model based on probabilistic neural network (PNN). The research result is meaningful for the detection of zero insulators.









Similar content being viewed by others
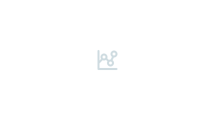
References
Du BX, Liang HC, Li J (2019) Surface coating affecting charge distribution and flashover voltage of cone-type insulator under DC stress. IEEE Trans Dielectr Electr Insul 26(3):706–713
Liu HR, He Li, Wei DJ, Li Q (2019) Experimental study of multiple-reignition features of secondary arcs on EHV/UHV transmission lines. IEEE Trans Industr Electron 66(4):3247–3255
Wang H, Cheng L, Liao R, Zhang S, Yang L (2020) Nonlinear ultrasonic nondestructive detection and modelling of kissing defects in high voltage composite insulators. IEEE Trans Dielectr Electr Insul 27(3):924–931
He H, Hu Z, Wang B, Luo D, Lee WJJ, Li (2020) A contactless zero-value insulators detection method based on infrared images matching. IEEE Access 8:133882–133889
Bi J, Hao Y, Yang L, Zheng Y, Li L (2020) Impact of hydrophobicity on wetting characteristics of composite insulators. IEEE Access 8:159316–159323
Gao Q, Yang W, Li Q (2016) Algorithm of insulator fault recognition based on sparse difference depth belief network. Electr Meas Instrum 1:19–25
Huang L, Yang Y, Zhao H, Wang X, Zheng H (2017) Time series modeling and filtering method of electric power load stochastic noise. Prot Control Modern Power Syst 2(1):1–7
Tu Y, Gong B, Wang C, Xu K, Xu Z, Wang S, Zhang F, Li R (2015) Effect of moisture on temperature rise of composite insulators operating in power system. IEEE Trans Dielectr Electr Insul 22(4):2207–2213
Park KC, Motai Y, Yoon JR (2017) Acoustic fault detection technique for high-power insulators. IEEE Trans Ind Electron 64(12):9699–9708
Jiang H, Qiu X, Chen J, Liu X, Zhuang S (2019) Insulator fault detection in aerial images based on ensemble learning with multi-level perception. IEEE Access 7:61797–61810
Nandi S, Subba BR, Sharma D (2020) Understanding performance of transmission and distribution insulators at very low temperature conditions. Electr Eng 102(4):2255–2268
Koo JH, Shin WJ, Oh DH, Hwang R, Lee BW (2017) Comparison of surface flashover characteristics of rod and rib type post insulator for extra-high voltage superconducting fault current limiter. IEEE Trans Appl Supercond 27(4):1–5
Ouyang X, Jia Z, Yang S, Shang X, Liu R (2018) Influence of algae growth on the external insulation performance of HVDC insulators. IEEE Trans Dielectr Electr Insul 25(1):263–271
Wang B, Dong M, Ren M, Wu Z, Guo C, Zhuang T et al (2020) Automatic fault diagnosis of infrared insulator images based on image instance segmentation and temperature analysis. IEEE Trans Instrum Meas 69(8):5345–5355
Zhang X, Zhang Y, Liu J, Zhang C, Xue C, Zhang H, Zhang W (2021) InsuDet: a fault detection method for insulators of overhead transmission lines using convolutional neural networks. IEEE Trans Instrum Meas 70:1–12
Madhiarasan M (2020) Accurate prediction of different forecast horizons wind speed using a recursive radial basis function neural network. Prot Control Modern Power Syst 5(1):1–9
Liu W, Liu Z, Wang H, Han Z (2020) An automated defect detection approach for catenary rod-insulator textured surfaces using unsupervised learning. IEEE Trans Instrum Meas 69(10):8411–8423
Uckol HI, Karaca B, Ilhan S (2020) DC and AC electric field analysis and experimental verification of a silicone rubber insulator. Electr Eng 102(1):503–514
Zhao W, Xu M, Cheng X, Zhao Z (2021) An insulator in transmission lines recognition and fault detection model based on improved faster RCNN. IEEE Trans Instrum Meas 70:1–8
Klimenta D, Jevtić M, Andriukaitis D, Mijailović V (2021) Increasing the transmission performance of a conventional 110 kV cable line by combining a hydronic concrete pavement system with photovoltaic floor tiles. Electr Eng 103:1401–1415
Zhai Y, Chen R, Yang Q, Li X, Zhao Z (2018) Insulator fault detection based on spatial morphological features of aerial images. IEEE Access 6:35316–35326
Jiang XL, Xia QF, Hu Q, Shu LC, Sun CX (2010) Influence of deteriorated insulator on the electric field distribution of overhang string. Proc CSEE 30(16):118–124
Jahed Armaghani D, Harandizadeh H, Momeni E (2021) Load carrying capacity assessment of thin-walled foundations: an ANFIS–PNN model optimized by genetic algorithm. Eng Comput. https://doi.org/10.1007/s00366-021-01380-0
Lin W, Wang X, Zheng Y, Xue L (2018) Research of feature extraction and recognition of partial discharge based on the probabilistic neural network. Power Syst Autom 40(3):60–63
Yan H, Chen J (2020) Insulator string positioning and state recognition method based on improved YOLOv3 algorithm. High Volt Eng 46(02):423–432
Funding
This work was supported in part by the Key Laboratory of Special Machine and High Voltage Apparatus (Shenyang University of Technology), Ministry of Education under Grant KFKT202111; Shandong Provincial Natural Science Foundation under Grant ZR2021ME057.
Author information
Authors and Affiliations
Contributions
He was a major contributor in writing the manuscript. Wu and Wang performed the simulation examination. Meng and Liu analyzed and interpreted the simulation results. Zhang is responsible for data recording, and Lin is responsible for checking. All authors reviewed and approved the final manuscript.
Corresponding author
Ethics declarations
Conflict of interests
The authors declare that they have no competing interests.
Additional information
Publisher's Note
Springer Nature remains neutral with regard to jurisdictional claims in published maps and institutional affiliations.
Rights and permissions
Springer Nature or its licensor (e.g. a society or other partner) holds exclusive rights to this article under a publishing agreement with the author(s) or other rightsholder(s); author self-archiving of the accepted manuscript version of this article is solely governed by the terms of such publishing agreement and applicable law.
About this article
Cite this article
He, B., Wu, S., Wang, L. et al. Location prediction model of zero value insulator based on PNN. Electr Eng 105, 2347–2360 (2023). https://doi.org/10.1007/s00202-023-01794-7
Received:
Accepted:
Published:
Issue Date:
DOI: https://doi.org/10.1007/s00202-023-01794-7