Abstract
Surface ozone is problematic to air pollution. It influences respiratory health. The air quality monitoring stations measure pollutants as surface ozone, but they are sometimes insufficient or do not have an adequate distribution for understanding the spatial distribution of pollutants in an urban area. In recent years, some projects have found a connection between remote sensing, air quality and health data. In this study, we apply an empirical land use regression (LUR) model to retrieve surface ozone in Quito. The model considers remote sensing data, air pollution measurements and meteorological variables. The objective is to use all available Landsat 8 images from 2014 and the air quality monitoring station data during the same dates of image acquisition. Nineteen input variables were considered, selecting by a stepwise regression and modelling with a partial least square (PLS) regression to avoid multicollinearity. The final surface ozone model includes ten independent variables and presents a coefficient of determination (R2) of 0.768. The model proposed help to understand the spatial concentration of surface ozone in Quito with a better spatial resolution.








Similar content being viewed by others
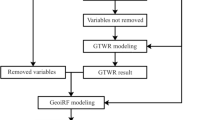
References
Adam-Poupart, A., Brand, A., Fournier, M., Jerrett, M., & Smargiassi, A. (2014). Spatiotemporal modeling of ozone levels in Quebec (Canada): a comparison of kriging, land-use regression (LUR), and combined Bayesian maximum entropy–LUR approaches. Environmental Health Perspectives, 122, 970–976. https://doi.org/10.1289/ehp.1306566.
Alvarez, C. I., Padilla Almeida, O. (2016). Estimación de la contaminación del aire por PM10 en Quito a través de índices ambientales con imágenes LANDSAT ETM+. Revista Cartográfica 135–147.
Alvarez-Mendoza, C. I., Teodoro, A., & Ramirez-Cando, L. (2018a). Improving NDVI by removing cirrus clouds with optical remote sensing data from Landsat-8 – a case study in Quito, Ecuador. Remote Sensing Applications: Society and Environment, 13, 257–274. https://doi.org/10.1016/j.rsase.2018.11.008.
Alvarez-Mendoza, C. I., Teodoro, A., Torres, N., et al. (2018b). Comparison of satellite remote sensing data in the retrieve of PM10 air pollutant over Quito, Ecuador. In: Thilo Erbertseder, Nektarios Chrysoulakis YZ (ed) Remote Sensing Technologies and Applications in Urban Environments III. Berlin, p 107930I–10793–12.
Ann Becerra, T., Wilhelm, M., Olsen, J., et al. (2013). Ambient air pollution and autism in Los Angeles County, California. Environmental Health Perspectives, 121, 380–386. https://doi.org/10.1289/ehp.1205827.
As-syakur, A. R., Adnyana, I. W. S., Arthana, I. W., & Nuarsa, I. W. (2012). Enhanced built-up and bareness index (EBBI) for mapping built-up and bare land in an urban area. Remote Sensing, 4, 2957–2970. https://doi.org/10.3390/rs4102957.
Ayres-Sampaio, D., Teodoro, A. C., Sillero, N., Santos, C., Fonseca, J., & Freitas, A. (2014). An investigation of the environmental determinants of asthma hospitalizations: an applied spatial approach. Applied Geography, 47, 10–19. https://doi.org/10.1016/j.apgeog.2013.11.011.
Bilal, M., Nichol, J. E., Bleiweiss, M. P., & Dubois, D. (2013). A simplified high resolution MODIS aerosol retrieval algorithm (SARA) for use over mixed surfaces. Remote Sensing of Environment, 136, 135–145. https://doi.org/10.1016/j.rse.2013.04.014.
Braun, D., Damm, A., Hein, L., Petchey, O. L., & Schaepman, M. E. (2018). Spatio-temporal trends and trade-offs in ecosystem services: an Earth observation based assessment for Switzerland between 2004 and 2014. Ecological Indicators, 89, 828–839. https://doi.org/10.1016/J.ECOLIND.2017.10.016.
Cattani, G., Gaeta, A., Di Menno di Bucchianico, A., di Menno di Bucchianico, A., de Santis, A., Gaddi, R., Cusano, M., Ancona, C., Badaloni, C., Forastiere, F., Gariazzo, C., Sozzi, R., Inglessis, M., Silibello, C., Salvatori, E., Manes, F., & Cesaroni, G. (2017). Development of land-use regression models for exposure assessment to ultrafine particles in Rome, Italy. Atmospheric Environment, 156, 52–60. https://doi.org/10.1016/J.ATMOSENV.2017.02.028.
Cazorla, M. (2016). Air quality over a populated andean region: Insights from measurements of ozone, NO, and boundary layer depths. Atmospheric Pollution Research, 7, 66–74. https://doi.org/10.1016/j.apr.2015.07.006.
Chen, G., & Meentemeyer, R. (2016). Remote sensing of forest damage by diseases and insects. In Q. Weng (Ed.), Remote Sensing for Sustainability (2017th ed., p. 357). Boca Raton: CRC Press.
Chen, L., Bai, Z., Kong, S., Han, B., You, Y., Ding, X., du, S., & Liu, A. (2010). A land use regression for predicting NO2 and PM10 concentrations in different seasons in Tianjin region, China. Journal of Environmental Sciences, 22, 1364–1373. https://doi.org/10.1016/S1001-0742(09)60263-1.
Chen, Y., Han, W., Chen, S., & Tong, L. (2014). Estimating ground-level PM2.5 concentration using Landsat 8 in Chengdu, China. Proceedings of SPIE, 9259, 925917–925931. https://doi.org/10.1117/12.2068886.
Chi, Y., Shi, H., Zheng, W., & Sun, J. (2018). Simulating spatial distribution of coastal soil carbon content using a comprehensive land surface factor system based on remote sensing. Science of the Total Environment, 628–629, 384–399. https://doi.org/10.1016/j.scitotenv.2018.02.052.
Daac, N. L. P, Falls, S., March, S. D. (2012). MODIS land products quality assurance tutorial: Part-1 how to find , understand , and use the quality assurance information for MODIS land products. 1–15.
de Mesnard, L. (2013). Pollution models and inverse distance weighting: some critical remarks. Computational Geosciences, 52, 459–469. https://doi.org/10.1016/J.CAGEO.2012.11.002.
Department of the Interior U.S. Geological Survey (2016). Landsat 8 (L8) Data Users Handbook http://www.crisp.nus.edu.sg/~research/tutorial/optical.htm. Accessed 21 Jul 2017.
Gholizadeh, H., & Robeson, S. M. (2016). Revisiting empirical ocean-colour algorithms for remote estimation of chlorophyll-a content on a global scale revisiting empirical ocean-colour algorithms for remote estimation of chlorophyll-a content on a global scale. International Journal of Remote Sensing, 37, 2682–2705. https://doi.org/10.1080/01431161.2016.1183834org/10.1080/01431161.2016.1183834.
Gillespie, A. (2014). Land surface emissivity. In E. Njoku (Ed.), Encyclopedia of remote sensing (p. 939). New York: Springer-Verlag.
Habermann, M., Billger, M., & Haeger-Eugensson, M. (2015). Land use regression as method to model air pollution. Previous results for Gothenburg/Sweden. Procedia Engineering, 115, 21–28. https://doi.org/10.1016/J.PROENG.2015.07.350.
Instituto Nacional de Meteorología e Hidrología (2016). Boletín Climatológico Anual 2015.
Jia, K., Liang, S., Zhang, L., Wei, X., Yao, Y., & Xie, X. (2014). Forest cover classification using Landsat ETM+ data and time series MODIS NDVI data. International Journal of Applied Earth Observation and Geoinformation, 33, 32–38. https://doi.org/10.1016/j.jag.2014.04.015.
Larkin, A., Geddes, J. A., Martin, R. V., Xiao, Q., Liu, Y., Marshall, J. D., Brauer, M., & Hystad, P. (2017). Global land use regression model for nitrogen dioxide air pollution. Environmental Science & Technology, 51, 6957–6964. https://doi.org/10.1021/acs.est.7b01148.
Lee, P., Saylor, R., & McQueen, J. (2018). Air quality monitoring and forecasting. Atmosphere (Basel), 9, 89. https://doi.org/10.3390/atmos9030089.
Liang, C.-S., Liu, H., He, K.-B., Ma, Y.-L. (2016). Assessment of regional air quality by a concentration-dependent Pollution Permeation Index OPEN. doi: https://doi.org/10.1038/srep34891.
Liew, S. C. (2001). Principles of remote sensing. In: Cent. Remote Imaging, Sens. Process. Cris.
Liu, Y., Franklin, M., Kahn, R., & Koutrakis, P. (2007). Using aerosol optical thickness to predict ground-level PM2.5 concentrations in the St. Louis area: a comparison between MISR and MODIS. Remote Sensing of Environment, 107, 33–44. https://doi.org/10.1016/j.rse.2006.05.022.
Meng, X., Chen, L., Cai, J., Zou, B., Wu, C. F., Fu, Q., Zhang, Y., Liu, Y., & Kan, H. (2015). A land use regression model for estimating the NO2 concentration in Shanghai, China. Environmental Research, 137, 308–315. https://doi.org/10.1016/j.envres.2015.01.003.
Meng, X., Fu, Q., Ma, Z., Chen, L., Zou, B., Zhang, Y., Xue, W., Wang, J., Wang, D., Kan, H., & Liu, Y. (2016). Estimating ground-level PM10 in a Chinese city by combining satellite data, meteorological information and a land use regression model. Environmental Pollution, 208, 177–184. https://doi.org/10.1016/J.ENVPOL.2015.09.042.
Mok, K. M., Yuen, K. V., Hoi, K. I., Chao, K. M., & Lopes, D. (2018). Predicting ground-level ozone concentrations by adaptive Bayesian model averaging of statistical seasonal models. Stochastic Environmental Research and Risk Assessment, 32, 1283–1297. https://doi.org/10.1007/s00477-017-1473-1.
Monks, P. S., Archibald, A. T., Colette, A., Cooper, O., Coyle, M., Derwent, R., Fowler, D., Granier, C., Law, K. S., Mills, G. E., Stevenson, D. S., Tarasova, O., Thouret, V., von Schneidemesser, E., Sommariva, R., Wild, O., & Williams, M. L. (2015). Tropospheric ozone and its precursors from the urban to the global scale from air quality to short-lived climate forcer. Atmospheric Chemistry and Physics, 15, 8889–8973. https://doi.org/10.5194/acp-15-8889-2015.
NASA EOSDIS (2018). Remote Sensors In: Earthdata. https://earthdata.nasa.gov/user-resources/remote-sensors. Accessed 21 Jul 2018 NCSS, LLC Stepwise Regression.
North, G. R. (2015). Climate and climate change - Greenhouse effect. Encyclopedia of Atmospheric Sciences, 80–86. https://doi.org/10.1016/B978-0-12-382225-3.00470-9.
Olmanson, L. G., Brezonik, P. L., Finlay, J. C., & Bauer, M. E. (2016). Comparison of Landsat 8 and Landsat 7 for regional measurements of CDOM and water clarity in lakes. Remote Sensing of Environment, 185, 119–128. https://doi.org/10.1016/j.rse.2016.01.007.
Secretaria del Ambiente de Quito (2018). Red Metropolitana de Monitoreo Atmosférico de Quito. http://www.quitoambiente.gob.ec/ambiente/index.php/generalidades. Accessed 26 Jun 2018.
Sicard, P., Anav, A., De Marco, A., & Paoletti, E. (2017). Projected global ground-level ozone impacts on vegetation under different emission and climate scenarios. Atmospheric Chemistry and Physics, 17, 12177–12196. https://doi.org/10.5194/acp-17-12177-2017.
Sobrino, J. A., Jiménez-Muñoz, J. C., Sòria, G., et al. (2008). Land surface emissivity retrieval from different VNIR and TIR sensors. IEEE Transactions on Geoscience and Remote Sensing, 46, 316–327. https://doi.org/10.1109/TGRS.2007.904834.
Stafoggia, M., Schwartz, J., Badaloni, C., Bellander, T., Alessandrini, E., Cattani, G., de' Donato, F., Gaeta, A., Leone, G., Lyapustin, A., Sorek-Hamer, M., de Hoogh, K., di, Q., Forastiere, F., & Kloog, I. (2017). Estimation of daily PM10 concentrations in Italy (2006–2012) using finely resolved satellite data, land use variables and meteorology. Environment International, 99, 234–244. https://doi.org/10.1016/J.ENVINT.2016.11.024.
Teodoro, A. (2015). A study on the quality of the vegetation index obtainded from MODIS data (pp. 3365–3368). Yokohama: IGARSS.
U.S. Geological Survey (2016). Product Guide: Landsat 8 Surface Reflectance Product
US Department of Commerce (2018). NOAA ESRL ESRL Global Monitoring Division - Ozone and Water Vapor Group. https://www.esrl.noaa.gov/gmd/ozwv/surfoz/. Accessed 23 May 2018.
US EPA (2014). Report of the Environment: Ozone Concentrations
USGS (2017). Product guide: Landsat surface reflectance-derived spectral indices
Vermote, E., Justice, C., Claverie, M., & Franch, B. (2016). Preliminary analysis of the performance of the Landsat 8/OLI land surface reflectance product. Remote Sensing of Environment, 185, 46–56. https://doi.org/10.1016/J.RSE.2016.04.008.
Vieira, D., Teodoro, A., & Gomes, A. (2016). Analysing land surface temperature variations during Fogo Island (Cape Verde) 2014-2015 eruption with Landsat 8 images. Proceedings of SPIE, 10005, 1000508. https://doi.org/10.1117/12.2241382.
Wang, M., Sampson, P. D., Hu, J., Kleeman, M., Keller, J. P., Olives, C., Szpiro, A. A., Vedal, S., & Kaufman, J. D. (2016). Combining land-use regression and chemical transport modeling in a spatiotemporal geostatistical model for ozone and PM 2.5. Environmental Science & Technology, 50, 5111–5118. https://doi.org/10.1021/acs.est.5b06001.
WHO (World Health Organization). (2013). Health risks of ozone from long-range transboundary air pollution. Journal of Chemical Information and Modeling, 53, 1689–1699. https://doi.org/10.1017/CBO9781107415324.004.
Williams, L. J., Abdi, H., & Williams, L. J. (2013). Partial least squares methods: Partial least squares correlation and partial Least Square regression. In B. Reisfeld & A. N. Mayeno (Eds.), Computational toxicology (Vol. II, pp. 549–579). Totowa: Humana Press.
Wolf, K., Cyrys, J., Harciníková, T., Gu, J., Kusch, T., Hampel, R., Schneider, A., & Peters, A. (2017). Land use regression modeling of ultrafine particles, ozone, nitrogen oxides and markers of particulate matter pollution in Augsburg, Germany. Science of the Total Environment, 579, 1531–1540. https://doi.org/10.1016/J.SCITOTENV.2016.11.160.
Yang, X., Zheng, Y., Geng, G., Liu, H., Man, H., Lv, Z., He, K., & de Hoogh, K. (2017). Development of PM2.5 and NO2 models in a LUR framework incorporating satellite remote sensing and air quality model data in Pearl River Delta region, China. Environmental Pollution, 226, 143–153. https://doi.org/10.1016/J.ENVPOL.2017.03.079.
Zhang, J., Hu, J., Lian, J., Fan, Z., Ouyang, X., & Ye, W. (2016). Seeing the forest from drones: testing the potential of lightweight drones as a tool for long-term forest monitoring. Biological Conservation, 198, 60–69. https://doi.org/10.1016/j.biocon.2016.03.027.
Zhang, X., Chu, Y., Wang, Y., & Zhang, K. (2018). Predicting daily PM2.5 concentrations in Texas using high-resolution satellite aerosol optical depth. Science of the Total Environment, 631–632, 904–911. https://doi.org/10.1016/J.SCITOTENV.2018.02.255.
Zheng, S., Zhou, X., Singh, R., Wu, Y., Ye, Y., & Wu, C. (2017). The spatiotemporal distribution of air pollutants and their relationship with land-use patterns in Hangzhou city, China. Atmosphere (Basel), 8, 110. https://doi.org/10.3390/atmos8060110.
Acknowledgements
This study is part of a PhD thesis in Surveying Engineering at the University of Porto, Portugal, supported by the Salesian Polytechnic University, Ecuador. This work was supervised at the University of Porto by Prof. Ana Cláudia Teodoro. The statistical analysis and regression models were supervised by Prof. Lenin Ramirez. We thank to Kathy Copo and Michelle Burgos for assistance with data acquisition to evaluate some initial parts of paper.
Author information
Authors and Affiliations
Corresponding author
Additional information
Publisher’s note
Springer Nature remains neutral with regard to jurisdictional claims in published maps and institutional affiliations.
Rights and permissions
About this article
Cite this article
Alvarez-Mendoza, C.I., Teodoro, A. & Ramirez-Cando, L. Spatial estimation of surface ozone concentrations in Quito Ecuador with remote sensing data, air pollution measurements and meteorological variables. Environ Monit Assess 191, 155 (2019). https://doi.org/10.1007/s10661-019-7286-6
Received:
Accepted:
Published:
DOI: https://doi.org/10.1007/s10661-019-7286-6