- 1Institute of Tuberculosis Prevention and Control, Ningbo Municipal Center for Disease Control and Prevention, Ningbo, China
- 2Center for Health Economics, Faculty of Humanities and Social Sciences, University of Nottingham, Ningbo, China
- 3Department of Disease Prevention and Health Promotion, Hwa Mei Hospital, University of Chinese Academy of Sciences, Ningbo, China
Setting: Controlling drug-resistant tuberculosis in Ningbo, China.
Objective: Whole-genome sequencing (WGS) has not been employed to comprehensively study Mycobacterium tuberculosis isolates, especially rifampicin-resistant tuberculosis, in Ningbo, China. Here, we aim to characterize genes involved in drug resistance in RR-TB and create a prognostic tool for successfully predicting drug resistance in patients with TB.
Design: Drug resistance was predicted by WGS in a “TB-Profiler” web service after phenotypic drug susceptibility tests (DSTs) against nine anti-TB drugs among 59 clinical isolates. A comparison of consistency, sensitivity, specificity, and positive and negative predictive values between WGS and DST were carried out for each drug.
Results: The sensitivities and specificities for WGS were 95.92 and 90% for isoniazid (INH), 100 and 64.1% for ethambutol (EMB), 97.37 and 100% for streptomycin (SM), 75 and 100% for amikacin (AM), 80 and 96.3%for capreomycin (CAP), 100 and 97.22% for levofloxacin (LFX), 93.33 and 90.91% for prothionamide (PTO), and 70 and 97.96% for para-aminosalicylic acid (PAS). Around 53 (89.83%) and 6 (10.17%) of the isolates belonged to lineage two (East-Asian) and lineage four (Euro-American), respectively.
Conclusion: Whole-genome sequencing is a reliable method for predicting resistance to INH, RIF, EMB, SM, AM, CAP, LFX, PTO, and PAS with high consistency, sensitivity, and specificity. There was no transmission that occurred among the patients with RR-TB in Ningbo, China.
Introduction
Rifampicin-resistant tuberculosis (RR-TB) is often diagnosed with genotypic or phenotypic techniques. RR-TB has become an important global health concern. Tracking RR-TB burden requires global efforts, including prevention, diagnosis, treatment, and surveillance (1). The medical treatment duration of RR-TB is longer compared with TB, which responds to drugs. However, more costly and toxic drugs may shorten the duration (2). According to the World Health Organization (WHO), ~0.46 × 106 new cases of RR-TB were reported worldwide in 2019. Furthermore, 78% of these cases were resistant to multiple drugs (3). China reports 66,000 cases of RR-TB yearly and ranks second among countries with high multidrug-resistant tuberculosis (MDR-TB) (3).
At the 1st stage in clinics, detecting drug resistance is critical to perform optimistic medical treatment together with avoiding the occurrence and transmission of extensively drug-resistant Mycobacterium tuberculosis (XDR-TB) (4). Over the past decades, phenotypic susceptibility has remained the primary way of diagnosing drug-resistant TB. However, phenotypic susceptibility tests only a few drugs, and the process is not quick (~6 weeks) (5). Molecular-based drug susceptibility test (DST) has recently been conducted in clinical laboratories to detect drug resistance and TB transmission dynamics (6, 7). Reports derived from recent studies are only available to a limited number of mutations and thus most likely do not identify heteroresistance (8, 9).
Whole-genome sequencing methods based on DNA sequencing platforms to reconstruct the complete genome DNA sequence can provide high-resolution genotyping and identification (10–12). The single-chromosome genome of MTBC bacteria makes WGS techniques ideal. Using DNA data from common pathways of drug resistance, WGS helps in the prediction of drug resistance (13, 14). Recently, the ease and cost-effectiveness of WGS in predicting resistance to anti-TB drugs have been established (15–17).
To date, WGS remains poorly investigated in China, and data on RR-TB remain anemic. Here, we conducted WGS for the prediction of RR-TB resistance to multiple drugs. We carried out this study in Ningbo, China, which has a relatively high annual TB incidence. Therefore, we compared WGS with the DST of 9 anti-TB drugs. We also included lesser-known drugs such as PAS and PTO.
Materials and methods
Isolates from clinical samples
We randomly selected N = 59 RR-TB isolates from our RR-TB inventory (stored at −80°C, Ningbo CDC, 1 January 2018 and 30 December 2019).
Culture-based DST was conducted for the nine anti-TB drugs. All the isolates were selected among patients with TB who have been residents of Ningbo for at least 6 months and have tested negative for human immunodeficiency virus (HIV) and tested positive for TB in local clinics. Exclusion criteria included pregnant women, minors (age <18), and patients with severe liver or renal diseases.
DST
Drug susceptibility tests of four first-line anti-TB drugs and five second-line drugs were carried out based on WHO recommendations (18). The drug concentrations are INH 0.2 μg /ml, RIF 40 μg/ml, EMB 2 μg/ml, SM, 4 μg/ml, LFX 2 μg/ml, AMK, 30 μg/ml, CAP 40 μg /ml, PTO 40 μg/ml, and PAS 1 μg/ml (18). H37RV strains were used as a reference for quality control.
WSG procedure and analysis
Genomic DNA from MTB colonies scraped from an L–J medium was detected to conduct sequencing with the CTAB technique of DNA purification. Quantification of DNA was carried out using the Qubit 2.0 fluorometer (Invitrogen, Carlsbad, CA, United States). Preparations of the library were carried out according to the manufacturer's instructions (Illumina TruSeq DNA Nano Library Prep Kit). Illumina HiSeq equipment (Illumina, San Diego, CA, Untied States) was used for libraries with different indices. A 2 × 150 paired-end (PE) configuration was employed during sequencing. MTB H37RV (GenBank accession no. NC 000962.3) was used as the reference strain. Drug resistance and strain-specific signatures were exported directly from raw sequences in the “TB-Profiler” tool (https://tbdr.lshtm.ac.uk/)(19).
Phylogeny construction
Using the Random Accelerated Maximum Likelihood software (RAxML), a phylogenetic tree was created to evaluate evolutionary connections between 59 RR-TB strains. Single nucleotide polymorphisms (SNPs) were not included to prevent non-evolutionary effects.
Statistical analysis
The number (No.) and percentage (%) of the samples were presented as descriptive analyses. Wilson scores confidence interval method was used to measure the sensitivity, specificity, positive predictive value (PPV), and negative predictive value (NPV) of WGS after DST.
A p-value of <0.05 was considered significant. SPSS 21.0 (SPSS., USA) was employed for statistical analyses.
Results
Drug susceptibility profiles of R-R TB isolates
In total, 59 (8.38%) out of 704 clinical isolates collected between 1 January 2018 and 30 December 2019 were identified as RR-TB, including 83.05% MDR-TB, 27.12% pre-XDR, and 11.86% XDR (Table 1). Among the 59 RR-TB clinical isolates, 83.05, 100, 33.9, 64.41 13.56, 8.47, 38.98, 25.42, and 16.95% were resistant to INH, RIF, EMB, SM, AMK, CAP, LFX, PTO and PAS, respectively.
Association of gene mutations with drug resistance
The results from the 59 RR-TB clinical isolates demonstrated the following: the genome 10 × coverage and genome 1 × coverage were from 51.67 to 238.11 (mean:166.29), from 98.69 to 99.95 (mean:99.19), and from 99.12 to 99.99 (mean:99.38).
The genes and mutations identified by WGS are linked to drug resistance (nine drugs) in the RR-TB isolates, which are listed in Supplemental Table 1.
Among the 59 RR-TB isolates, 13 (22.03%) strains had >1 mutations that are linked with INH resistance. The most common (n = 38) mutation was katG Ser315Thr and the second most common (n = 9) was fabG1 −8T>C.
Mutations linked with RIF resistance were detected in the 59 isolates (100.00%), and the rpoB gene had mutations in 100% of the samples. No other mutations were found in the isolates. All the isolates had mutations in the rpoB rifampicin resistance-determining region (RRDR, 81 bp). rpoB Ser531Leu (n = 37) was the most common.
The genes linked with resistance to SM were gid (n = 2), rspL (n = 33), and rrs (n = 2). Thirty-four strains showed EMB resistance. embA and embB mutations were found in two cases, and only onecase demonstrated mutations at two embB locations. The most common were embB Met306Val [52.94% (18/34)] and Met306Ile [23.53% (8/34)].
In respect to LFX, 23 (38.98%) isolates had gyrA gene mutations, of which the most common mutation observed was gyrA Asp94Gly [65.22% (15/23)]. Only one sample had gyrB mutation.
rrs gene mutations were found in all isolates that are resistant to AMK; 100% of the mutations were 1401a>g. rrs was found in genes linked to CAP resistance with the mutation 1401a>g (n = 6).
Fifteen strains showed PTO phenotype resistance, and 44 strains showed sensitivity. A total of 14 (93.33%) isolates had genetic mutations linked to PTO resistance. Mutations in both fabG1 (t-8c) and ethA genes (ethA 11a-ins, ethA 13a-ins, and ethA 11a-dup) were the most common (57.14%) (8/14).
Folate metabolism pathways, primarily thyA, folC, ribD, and dfrA, affect PAS resistance. Seven strains were resistant and demonstrated different mutations including folC Ile43Ser (n = 1), folC Ser150Gly (n = 1), folC Glu153Ala (n = 1), folC Glu153 Gly (n = 1), thyX(c−16t) (n = 1), and thyA Thr22Ala+ thyX(c−16t) (n = 2).
The phenotypic DST found resistance-related mutations in some of the samples. Mutations were discovered for INH at katG Ser315Gly (n = 1); for EMB at embB Met306Ile (n = 4), embB Met306Val (n = 6), embB Asp354Ala (n = 1), embB Gly406Asp (n = 2), and embB His1002Arg (n = 1); for CAP at rrs a1401g (n = 2); for LFX at gyrA Asp94Ala (n = 1); for PTO at fabG1 t-8c (n = 1), fabG1 c-15t (n = 1), ethA 403–424del (n = 1), ethA 61del (n = 1); for PAS at thyX c-16t (n = 1).
Comparison of WGS and phenotypic DST results
The properties of each drug are listed in Table 2. A mean consistency of 93.79% (n = 59 isolates) was found for all the nie drugs, [76.27% (EMB) to 100% (RIF)]. The overall range of sensitivity and specificity for WGS were 70%−100% and 64.10%−100.00%, respectively. For both the highest sensitivity and specificity, 100% was found in 3 (RIF, EMB, and LFX) and 2 (SM and AMK) of the drugs respectively. The lowest sensitivity and specificity were found at 70% (PAS) and 64.1% (EMB), respectively. The phenotypic DST showed drug resistance in 10 samples (n = 2 for INH, n = 1 for SM, n = 2 for AMK, n = 1 for CAP, n = 1 for PTO, and n = 3 for PAS); however, the WGS results did not demonstrate any known drug-resistant mutations in the samples (Table 2).
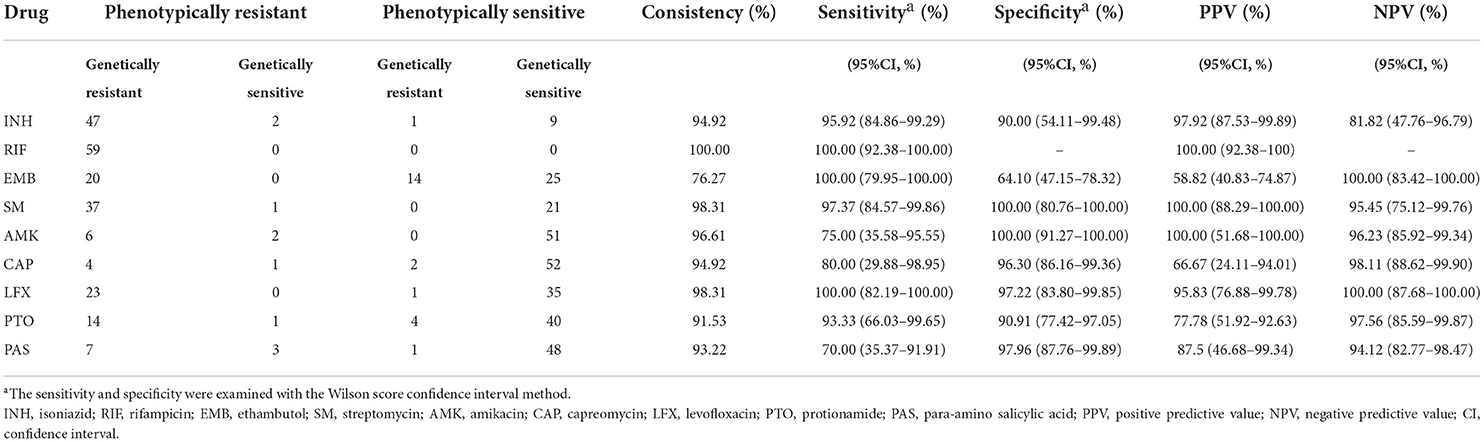
Table 2. Whole-genome sequencing compared with phenotypic DST for detection of drug resistance in RR-TB.
Phylogenetic pattern of drug resistance
A phylogenetic tree showing profiles and lineages related to drug resistance is shown in Figure 1. No transmission occurred among the 59 RR-TB strains, which is shown in Figure 2. Around 53 (89.83%) and 6 (10.17%) belonged to lineage two (East-Asian) and lineage four (Euro-American), respectively.
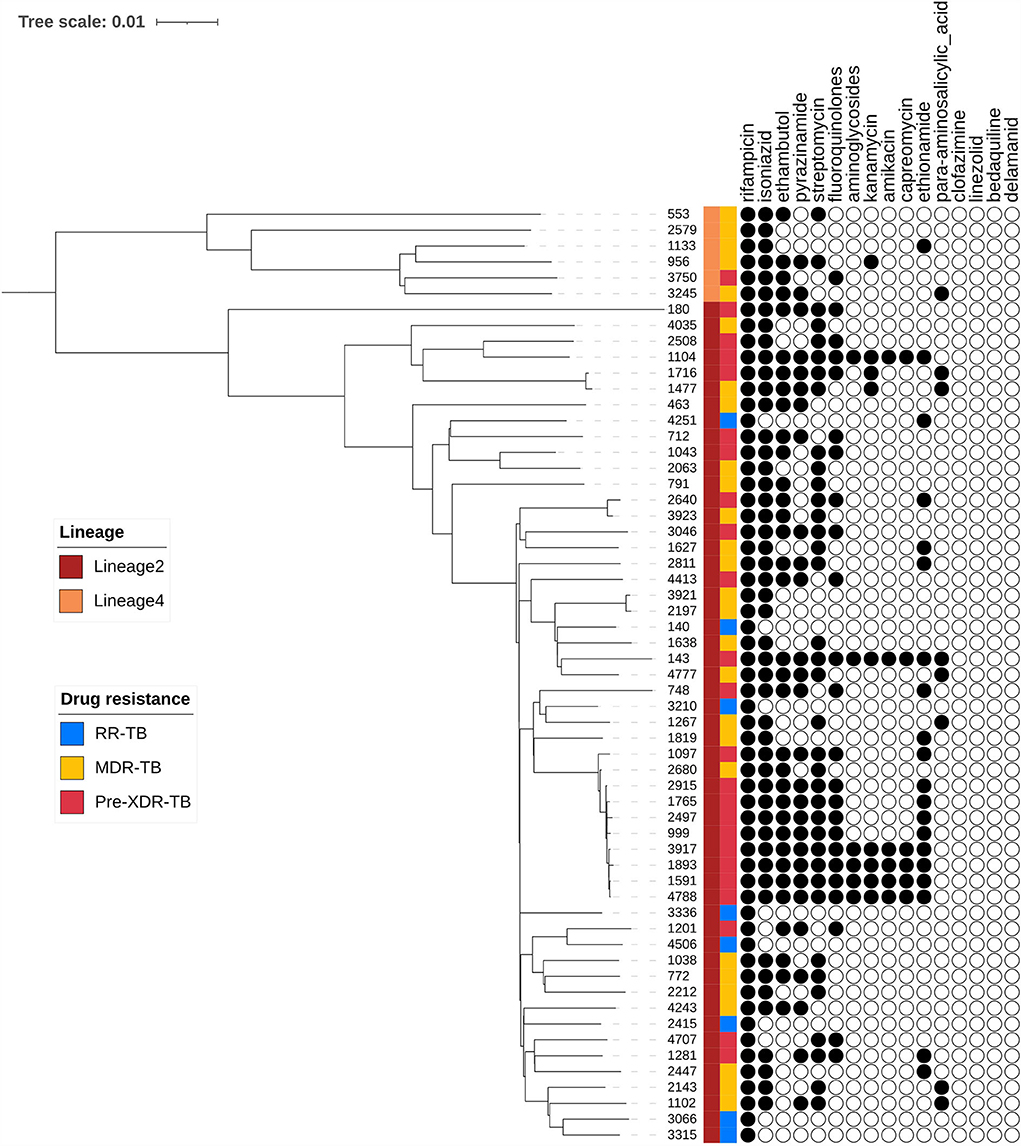
Figure 1. Phylogenetic tree of 59 R-R MTB clinical samples showing drug resistance profiles and lineages.
Discussion
TB control and prevention, including diagnosis, treatment, and surveillance, can be guided by rapid, reliable, and increasingly affordable WGS technology. It effectively creates a genetic signature for drug resistance. Recently, WGS has been a critical player in predicting resistance to drugs (20–22). To the best of our knowledge, this study is the first to investigate gene mutations that impart resistance to nine anti-TB medications through the WGS method in Ningbo, China, and show that WGS is a reliable method for predicting resistance to INH, RIF, EMB, SM, AMK, CAP, LFX, PTO, and PAS with high accuracy, sensitivity, and specificity. The laboratories of Public Health England and the New York Department of Health have approved the use of WGS for testing susceptibility to TB drugs (23). Although WGS is widely conducted in research, its use in the clinical setting faces roadblocks since bioinformatics specialists are required for data analysis. Using raw sequences and the online software known as the TB-Profiler can predict drug resistance more accurately than other online platforms (24). In our study, WGS and the TB- Profiler were employed for prediction of drug resistance in 59 RR-TB clinical isolates. Mutations in katG and fabG1 were discovered in 93.88% (46/49) of INH genotype-resistant isolates in our study, showing the role of the two locations in prediction of INH resistance. Ser315Thr was the most prevalent mutation in katG; Ser315Thr previously has been shown to impart a significant level of INH resistance (25–27).
One previous review study showed that mutations in the 81-bp region of rpoB (RRDR) were responsible for more than 96% of RIF resistance (28), which was in line with our findings. Our study showed that no RIF-resistant bacteria were in this region. Outside the RRDR, mutations may not be detected by GeneXpert MTB/RIF (molecular test). Additionally, other mutations in the RRDR, such as a synonymous or silent mutations, may cause RIF resistance to be misdiagnosed (29).
In clinical isolates resistant to EMB, embB codon 306 mutations were the most prevalent (30). The most common mutations in this analysis were embB Met306Val and Met306Ile, which accounted for 52.94 and 23.53%, respectively.
Three resistance genes were found to be related to SM, notably rrs, rpsL, and gid, which encode 16srRNA, ribosomal protein S12, and 16srRNA-specific methyltransferase, respectively (31, 32). Mutations in the rpsL gene in our study, linked with high levels of resistance to SM, were predominant among SM-resistant isolates. We found that only isolates that did not have rrs or rpsL mutations carried gid mutations. gid mutations linked with low resistance to SM were rare and only occurred in two (5.26%) SM genotype-resistant strains.
AMK and CAP genotype-resistant samples showed mutation 1401a>g in rrs linked to 70%−80% of MTB samples that are resistant to AMK and CAP globally (33).
Chromosome changes in the quinolone resistance determining region of gyrA or gyrB are the main way of fluoroquinolones resistance in MTB (34). Similar to previous studies (35, 36), the most prevalent mutations were found in positions 90 and 94 of gyrA in the LFX-resistant isolates, and lesser mutations were found in positions 46 and 91.
It is well known that the inhA c-15t mutation is associated with PTO resistance (37). We found that fabG1 t-8c and ethA genes mutation were most prevalent, and that no isolates harbored an inhA mutation in our study.
Regarding PAS, DST could not be successfully replaced by WGS in our study, but WGS may be more useful in better interpretation of gene mutations compared with DST. Despite high specificities, WGS failed to successfully diagnose PAS resistance because of the limited number of resistant strains.
The majority of drugs showed > 90% sensitivity and specificity, indicating that WGS is a reliable method for predicting drug resistance. The WGS results were consistent with the phenotypic DST for INH, RIF, SM, AMK, CAP, LFX, PTO, and PAS, showing that all were >90.00%, which was consistent with other studies with the same methods (38). A lower specificity for predicting EMB resistance than other drugs was found in our study. Around 35.9% of the EMB phenotype-sensitive isolates harbored mutations associated with EMB resistance. This could be because phenotypic DST for EMB is unreliable (39). However, it is unclear whether EMB resistance is affected by resistance mechanisms to other drugs (40).
The differences between WGS and phenotypic DST are explained by the following reasons. First, phenotypic DST may not catch samples that have low resistance to drugs. Recently, it was shown that phenotypic DST failed to diagnose EMB resistance, especially in the case of INH resistance (39, 40). Second, WGS may not be able to diagnose certain types of non-specific resistance, e.g., resistance that develops because of efflux pumps (41, 42).
Whole-genome sequencing has significant advantages over both phenotypic DST and molecular DST. WSG can detect specific genetic mutations promptly, which may be helpful for the most accurate treatment method. The detection capacity of WGS will also increase with time as novel genes involved in drug resistance are identified and the database is updated. Additionally, if certain laboratories do not have appropriate biosafety hoods, inactivation of strains may be performed and the inactivated strains may be sent to WGS labs, significantly reducing the time (months for DST vs. weeks for WGS). WGS will also become quicker and less expensive with the development of sequencing technology.
To our best knowledge, this was the first study on the agreement of phenotypic DST and WGS DST among rifampicin-resistant Mycobacterium tuberculosis (RR-TB) isolates in Ningbo. Our study may help in management of patients with TB and contribute to the development of novel anti-TB drugs and TB prevention strategies. Nonetheless, although the advanced sequencing technology of WGS and benefits were derived from WGS, some limitations of this study need to be considered. First, the study investigated a small number of anti-TB drugs, and pyrazinamide and linezolid were not included. Second, the number of amikacin-resistant isolates was small since AMK shows a low level of resistance. As a result, our results may be biased. Third, the small sample size number limited the statistical power of the study. There results must be interpreted with caution. However, our cohort represented the largest consecutive cohort of RR-TB in the region and the patients were representative thanks to the high detection rates of endemic TB. However, the findings of this study provided critical evidence for research and clinics to diagnose drug resistance by WGS and may help treat patients with high RR-TB burden.
Data availability statement
The datasets presented in this study can be found in online repositories. The names of the repository/repositories and accession number(s) can be found at: http://www.ncbi.nlm.nih.gov/bioproject/862152, PRJNA862152.
Ethics statement
Studies involving human participants were reviewed and approved by Ningbo CDC IBR and Hwa Mei Hospital IBR. Written informed consent for participation was not required for this study in accordance with the national legislation and institutional requirements.
Author contributions
YC and YL conceived the study and were involved in the design, analysis, report writing, and drafting of the manuscript. TY, TC, GS, QC, and TH were involved in the conception and design, and supervised the study. QC and TH were involved in manuscript review. All authors contributed to the article and approved the submitted version.
Funding
This study was funded by the Medical Technology Program Foundation of Zhejiang (Grant nos: 2021KY334 and 2022KY1189) and Ningbo Health Branding Subject Fund (Grant no: PPXK2018-10). The findings and conclusions of our study are not official representations of the Natural Science Foundation of Ningbo.
Conflict of interest
The authors declare that the research was conducted in the absence of any commercial or financial relationships that could be construed as a potential conflict of interest.
Publisher's note
All claims expressed in this article are solely those of the authors and do not necessarily represent those of their affiliated organizations, or those of the publisher, the editors and the reviewers. Any product that may be evaluated in this article, or claim that may be made by its manufacturer, is not guaranteed or endorsed by the publisher.
Supplementary material
The Supplementary Material for this article can be found online at: https://www.frontiersin.org/articles/10.3389/fpubh.2022.956171/full#supplementary-material
References
1. Dixit A, Freschi L, Vargas R, Calderon R, Sacchettini J, Drobniewski F, et al. Whole genome sequencing identifies bacterial factors affecting transmission of multidrug-resistant tuberculosis in a high-prevalence setting. Sci Rep. (2018) 9:5602–11. doi: 10.1038/s41598-019-41967-8
2. Katale BZ, Mbelele PM, Lema NA, Campino S, Mshana SE, Rweyemamu MM, et al. Whole genome sequencing of Mycobacterium tuberculosis isolates and clinical outcomes of patients treated for multidrug-resistant tuberculosis in Tanzania. BMC Genomics. (2020) 21:174–88. doi: 10.1186/s12864-020-6577-1
3. WHO. Global tuberculosis report 2021. Geneva: WHO (2021). Available online at: https://apps.who.int/iris/rest/bitstreams/1379788/retrieve/9789240037021-eng.pdf (accessed October 14, 2021).
4. Zumla AI, Gillespie SH, Hoelscher M, Philips PP, Cole ST, Abubakar I, et al. New antituberculosis drugs, regimens, and adjunct therapies: needs, advances, and future prospects. Lancet Infect Dis. (2014) 14:327–40. doi: 10.1016/S1473-3099(13)70328-1
5. Van Deun A, Aung KJ, Bola V, Lebeke R, Hossain MA, Rijk DE, et al. Drug resistance tests for tuberculosis: challenging the gold standard. J Clin Microbiol. (2013) 51:2633–40. doi: 10.1128/JCM.00553-13
6. Siddiqui S, Brooks MB, Malik AA, Fuad J, Nazish A, Bano S, et al. Evaluation of GenoType MTBDRplus for the detection of drug-resistant Mycobacterium tuberculosis on isolates from Karachi, Pakistan. PloS ONE. (2019) 14:e0221485. doi: 10.1371/journal.pone.0221485
7. Zhang Q, Zhang Q, Sun BQ, Liu C, Su AN, Wang XH, et al. GeneXpert MTB/RIF for rapid diagnosis and rifampin resistance detection of endobronchial tuberculosis. Respirology. (2018) 23:950–5. doi: 10.1111/resp.13316
8. Cohen KA, Manson AL, Desjardins CA, Abeel T, Earl AM. Deciphering drug resistance in Mycobacterium tuberculosis using whole-genome sequencing: progress, promise, and challenges. Genome Med. (2019) 11:45. doi: 10.1186/s13073-019-0660-8
9. Miotto P, Tessema B, Tagliani E, Chindelevitch L, Starks AM, Emerson C, et al. A standardised method for interpreting the association between mutations and phenotypic drug resistance in Mycobacterium tuberculosis. Eur Respir J. (2017) 50:1701354. doi: 10.1183/13993003.01354-2017
10. van der Werf MJ, Ködmön C. Whole-genome sequencing as tool for investigating international tuberculosis outbreaks: a systematic review. Front Public Health. (2019) 7:87. doi: 10.3389/fpubh.2019.00087
11. Walker TM, Merker M, Kohl TA, Crook DW, Niemann S, Peto TE, et al. Whole genome sequencing for M/XDR tuberculosis surveillance and for resistance testing. Clin Microbiol Infect. (2017) 23:161–6. doi: 10.1016/j.cmi.2016.10.014
12. Witney AA, Cosgrove CA, Arnold A, Hinds J, Stoker NG, Butcher PD, et al. Clinical use of whole genome sequencing for Mycobacterium tuberculosis. BMC Med. (2016) 14:46. doi: 10.1186/s12916-016-0598-2
13. Takiff HE, Feo O. Clinical value of whole-genome sequencing of Mycobacterium tuberculosis. Lancet Infect Dis. (2015) 15:1077–90. doi: 10.1016/S1473-3099(15)00071-7
14. Walker TM, Kohl TA, Omar SV, Hedge J, Del Ojo Elias C, Bradley P, et al. Whole-genome sequencing for prediction of Mycobacterium tuberculosis drug susceptibility and resistance: a retrospective cohort study. Lancet Infect Dis. (2015) 15:1193–202. doi: 10.1016/S1473-3099(15)00062-6
15. Nikolayevskyy V, Niemann S, Anthony R, van Soolingen D, Tagliani E, Ködmön C, et al. Role and value of whole genome sequencing in studying tuberculosis transmission. Clin Microbiol Infect. (2019) 25:1377–82. doi: 10.1016/j.cmi.2019.03.022
16. O'Donnell MR, Larsen MH, Brown TS, Jain P, Munsamy V, Wolf A, et al. Early detection of emergent extensively drug-resistant tuberculosis by flow cytometry-based phenotyping and whole-genome sequencing. Antimicrob Agents Chemother. (2019) 63:e01834-18. doi: 10.1128/AAC.00764-19
17. Satta G, Lipman M, Smith GP, Arnold C, Kon OM, McHugh TD, et al. Mycobacterium tuberculosis and whole-genome sequencing: how close are we to unleashing its full potential? Clin Microbiol Infect. (2018) 24:604–9. doi: 10.1016/j.cmi.2017.10.030
18. Barrera L, Cooreman E, Dieu Iragena DE, Drobniewski J, Duda F, Havelkova P, et al. WHO guidelines approved by the guidelines review committee. In: Policy Guidance on Drug-Susceptibility Testing (DST) of Second-Line Antituberculosis Drugs. Geneva: World Health Organization (2008). Available online at: https://www.ncbi.nlm.nih.gov/books/NBK310860/pdf/Bookshelf_NBK310860.pdf (accessed October 12, 2021).
19. Coll F, McNerney R, Preston MD, Guerra-Assunção JA, Warry A, Hill-Cawthorne G, et al. Rapid determination of anti-tuberculosis drug resistance from whole-genome sequences. Genome Med. (2015) 7:51. doi: 10.1186/s13073-015-0164-0
20. Nikolayevskyy V, Kranzer K, Niemann S, Drobniewski F. Whole genome sequencing of Mycobacterium tuberculosis for detection of recent transmission and tracing outbreaks: a systematic review. Tuberculosis. (2016) 98:77–85. doi: 10.1016/j.tube.2016.02.009
21. Wu X, Gao R, Shen X, Guo Y, Yang J, Wu Z, et al. Use of whole-genome sequencing to predict Mycobacterium tuberculosis drug resistance in Shanghai, China. Int J Infect Dis. (2020) 96:48–53. doi: 10.1016/j.ijid.2020.04.039
22. Yang C, Luo T, Shen X, Wu J, Gan M, Xu P, et al. Transmission of multidrug-resistant Mycobacterium tuberculosis in Shanghai, China: a retrospective observational study using whole-genome sequencing and epidemiological investigation. Lancet Infect Dis. (2017) 17:275–84. doi: 10.1016/S1473-3099(16)30418-2
23. Allix-Béguec C, Arandjelovic I, Bi L, Beckert P, Bonnet M, Bradley P, et al. Prediction of susceptibility to first-line tuberculosis drugs by DNA sequencing. N Engl J Med. (2018) 379:1403–15. doi: 10.1056/NEJMoa1800474
24. Macedo R, Nunes A, Portugal I, Duarte S, Vieira L, Gomes JP, et al. Dissecting whole-genome sequencing-based online tools for predicting resistance in Mycobacterium tuberculosis: can we use them for clinical decision guidance? Tuberculosis. (2018) 110:44–51. doi: 10.1016/j.tube.2018.03.009
25. Jabbar A, Phelan JE, Sessions DE, Khan PF, Rahman TA, Khan H, et al. Whole genome sequencing of drug resistant Mycobacterium tuberculosis isolates from a high burden tuberculosis region of North West Pakistan. Sci Rep. (2019) 9:14996. doi: 10.1038/s41598-019-51562-6
26. Pym AS, Saint-Joanis B, Cole ST. Effect of katG mutations on the virulence of Mycobacterium tuberculosis and the implication for transmission in humans. Infect Immun. (2002) 70:4955–60. doi: 10.1128/IAI.70.9.4955-4960.2002
27. Saint-Joanis B, Souchon H, Wilming M, Johnsson K, Alzari PM, Cole ST, et al. Use of site-directed mutagenesis to probe the structure, function and isoniazid activation of the catalase/peroxidase, KatG, from Mycobacterium tuberculosis. Biochem J. (1999) 338:753–60. doi: 10.1042/bj3380753
28. Musser JM. Antimicrobial agent resistance in mycobacteria: molecular genetic insights. Clin Microbiol Rev. (1995) 8:496–514. doi: 10.1128/CMR.8.4.496
29. McAlister AJ, Driscoll J, Metchock B. DNA sequencing for confirmation of rifampin resistance detected by Cepheid Xpert MTB/RIF assay. J Clin Microbiol. (2015) 53:1752–3. doi: 10.1128/JCM.03433-14
30. Enkirch T, Werngren J, Groenheit R, Alm E, Advani R, Lind Karlberg M, et al. Systematic review of whole-genome sequencing data to predict phenotypic drug resistance and susceptibility in Swedish Mycobacterium tuberculosis isolates, 2016 to 2018. Antimicrob Agents Chemother. (2020) 64:e02550-19. doi: 10.1128/AAC.02550-19
31. Dookie N, Rambaran S, Padayatchi N, Mahomed S, Naidoo K. Evolution of drug resistance in Mycobacterium tuberculosis: a review on the molecular determinants of resistance and implications for personalized care. J Antimicrob Chemother. (2018) 73:1138–51. doi: 10.1093/jac/dkx506
32. Gygli SM, Keller PM, Ballif M, Blöchliger N, Hömke R, Reinhard M, et al. Whole-genome sequencing for drug resistance profile prediction in Mycobacterium tuberculosis. Antimicrob Agents Chemother. (2019) 63:e02175-18. doi: 10.1128/AAC.02175-18
33. Bouzouita I, Cabibbe AM, Trovato A, Daroui H, Ghariani A, Midouni B, et al. Whole-genome sequencing of drug-resistant Mycobacterium tuberculosis Strains, Tunisia, 2012-2016. Emerg Infect Dis. (2019) 25:538–46. doi: 10.3201/eid2503.181370
34. Ruiz J. Mechanisms of resistance to quinolones: target alterations, decreased accumulation and DNA gyrase protection. J Antimicrob Chemother. (2003) 51:1109–17. doi: 10.1093/jac/dkg222
35. Sun Z, Zhang J, Zhang X, Wang S, Zhang Y, Li C, et al. Comparison of gyrA gene mutations between laboratory-selected ofloxacin-resistant Mycobacterium tuberculosis strains and clinical isolates. Int J Antimicrob Agents. (2008) 31:115–21. doi: 10.1016/j.ijantimicag.2007.10.014
36. Zhang Z, Lu J, Wang Y, Pang Y, Zhao Y. Prevalence and molecular characterization of fluoroquinolone-resistant Mycobacterium tuberculosis isolates in China. Antimicrob Agents Chemother. (2014) 58:364–9. doi: 10.1128/AAC.01228-13
37. Moreno-Molina M, Comas I, Furió V. The future of TB resistance diagnosis: the essentials on whole genome sequencing and rapid testing methods. Arch Bronconeumol. (2019) 55:421–6. doi: 10.1016/j.arbres.2019.01.002
38. Chaidir L, Ruesen C, Dutilh BE, Ganiem AR, Andryani A, Apriani L, et al. Use of whole-genome sequencing to predict Mycobacterium tuberculosis drug resistance in Indonesia. J Glob Antimicrob Resist. (2019) 16:170–7. doi: 10.1016/j.jgar.2018.08.018
39. Meehan CJ, Goig GA, Kohl TA, Verboven L, Dippenaar A, Ezewudo M, et al. Whole genome sequencing of Mycobacterium tuberculosis: current standards and open issues. Nat Rev Microbiol. (2019) 17:533–45. doi: 10.1038/s41579-019-0214-5
40. Heyckendorf J, Andres S, Köser CU, Olaru ID, Schön T, Sturegård E, et al. What is resistance? Impact of phenotypic versus molecular drug resistance testing on therapy for multi- and extensively drug-resistant tuberculosis. Antimicrob Agents Chemother. (2018) 62:e01550-17. doi: 10.1128/AAC.01550-17
41. Kanji A, Hasan R, Hasan Z. Efflux pump as alternate mechanism for drug resistance in Mycobacterium tuberculosis. Indian J Tuberc. (2019) 66:20–5. doi: 10.1016/j.ijtb.2018.07.008
Keywords: drug resistance, drug susceptibility test, whole-genome sequencing, rifampicin-resistant tuberculosis, gene mutation
Citation: Che Y, Lin Y, Yang T, Chen T, Sang G, Chen Q and He T (2022) Evaluation of whole-genome sequence to predict drug resistance of nine anti-tuberculosis drugs and characterize resistance genes in clinical rifampicin-resistant Mycobacterium tuberculosis isolates from Ningbo, China. Front. Public Health 10:956171. doi: 10.3389/fpubh.2022.956171
Received: 30 May 2022; Accepted: 28 July 2022;
Published: 18 August 2022.
Edited by:
Francisco Gonzalez-Salazar, Mexican Social Security Institute (IMSS), MexicoReviewed by:
Ning Sun, Hong Kong Polytechnic University, Hong Kong SAR, ChinaWenchang Yuan, Guangzhou Medical University, China
Ruyang Zhang, Nanjing Medical University, China
Copyright © 2022 Che, Lin, Yang, Chen, Sang, Chen and He. This is an open-access article distributed under the terms of the Creative Commons Attribution License (CC BY). The use, distribution or reproduction in other forums is permitted, provided the original author(s) and the copyright owner(s) are credited and that the original publication in this journal is cited, in accordance with accepted academic practice. No use, distribution or reproduction is permitted which does not comply with these terms.
*Correspondence: Qin Chen, 148119107@qq.com; Tianfeng He, hetf@nbcdc.org.cn
†These authors have contributed equally to this work