- 1Unit of Addiction Medicine, Department of Internal Medicine, Integrated University Hospital of Verona, Policlinico “G.B. Rossi”, Verona, Italy
- 2Department of Neurosciences, University of Verona, Verona, Italy
- 3Department of Medical Sciences and Public Health, University of Cagliari, Cagliari, Italy
- 4Department of Sport Sciences, Sports Research Centre, Miguel Hernández University, Elche, Spain
- 5Neuropharmacology on Pain and Functional Diversity (NED), Institute of Health and Biomedical Research of Alicante (ISABIAL), Alicante, Spain
- 6Padova Neuroscience Center, University of Padova, Padova, Italy
- 7Department of General Psychology, University of Padova, Padova, Italy
Benzodiazepines (BZDs) represent one of the most widely used groups of pharmaceuticals, but if used for long periods of time they are associated with dependence and an increased risk of harmful effects. High-dose (HD) BZD dependence is a specific substance use disorder associated with a poor quality of life. It is especially important to pinpoint differences in HD BZD addict subgroups in order to tailor treatment to the individual's specific needs, also considering possible comorbidities with other substance use disorders. We conducted a study to evaluate HD BZD dependence (converted doses to diazepam equivalents, mg) in an Italian sample of 1,354 participants. We also investigated if and to which extent participants co-used other substances (alcohol, tobacco, cannabis/cannabinoids, cocaine, and heroin). We then performed latent class analysis (LCA) to identify the use patterns of these substances, finding three classes: participants in Class 1 (4.3% of the sample) had the highest probability of also using cocaine and alcohol (Polysubstance BZD users); Class 2 comprised subjects with the highest probability of being former heroin, cocaine, THC, and alcohol users (Former polysubstance BZD users); Class 3 represented mono-dependence BZD users (78.5% of the sample) and was the most prevalent among women, while young men were most prevalent in Class 1.
The present study underlines different characteristics in HD BZD users both concerning other addictions and sex, and also highlights the need for a stricter control of BZD use, ranging from prescriptions to sales.
Introduction
Benzodiazepines (BZDs) are among the most commonly prescribed medications for insomnia and anxiety and are extensively used in clinical practice. BZDs act as positive allosteric modulators of the GABA-A (Gamma-Aminobutyric Acid Type A) receptor (1). A number of studies have evidenced that benzodiazepines should be considered a suitable treatment for specific clinical situations and for short-term use only (2–4): indeed, long-term use of this class of drugs increases the likelihood of adverse effects and dependence and should therefore be implemented with caution. Alternative short or intermittent treatments could have important benefits for patients and should be taken into consideration when deciding on a specific course of treatment (5).
Long-term BZD users range from 6 to 76% of total users. Fifteen to forty four percent of them present moderate-to-severe withdrawal symptoms, and 3–4% show a dependence (6).
High-dose (HD) BZD dependence is considered a specific substance use disorder (7) and it consistently reduces quality of life in patients that suffer from it (8, 9). Ohayon and Lader (10) conducted a cross-sectional survey in various European countries (France, Germany, and Italy) and in the UK, and found that an estimated 0.14% of the general population took higher-than-recommended doses of anxiolytic medications, while 0.06% reportedly abused hypnotics (10). These numbers are in line with the 0.16% of high-dose BZD users reported in Switzerland (11) and point toward HD BZD abusers being around 1.5 million in Europe and 600,000 in the United States.
Long-term BZD use was reported to be associated with abnormalities in cognitive functions, including attention, memory and learning, a higher risk of delirium, cognitive decline and accidents (12–21).
BZD withdrawal in patients is an especially discomforting experience. To alleviate withdrawal symptoms, therapeutic strategies such as gradual tapering of the dosage or substituting the target BZD with an equivalent dose of another long-acting benzodiazepine and then tapering have been developed (22, 23).
BZDs have been reported to be secondary drugs of abuse for most individuals, and a much smaller number report BZDs as the primary drugs of abuse. BZD abuse is mainly associated with opioids (54.2%) and alcohol (24.7%) abuse. As reported by Schmitz (24) in her recent review, about 1 in 5 people who abuse alcohol also abuse benzodiazepines (25).
In the last decade, research has been increasingly focused on patterns of polyabuse defined as the use of more than one drug during a specific time period. While patterns of use and characteristics of polyabusers have been examined among many substance users (such as alcohol, cocaine, heroin, etc.), not much is known about polyabuse patterns among BZD abusers. Several studies have aimed to address this complexity by identifying homogenous subgroups of patients who have similar outcomes (26, 27). The same pre-existent characteristics of the patients, indeed, do not consistently produce the same effects.
Understanding the distribution and determinants of polysubstance use is crucial for planning overdose prevention programs and policies. The problem of polydrug use has also been acknowledged as crucial in the context of treatment. There is general consensus that the effects of combining multiple substances of abuse are often problematic to predict and can increase the risks of accidents, overdose and death (28). In this respect, the last 15 years have seen an increased focus on person-centered methodologies that statistically uncover subpopulations with distinct combinations of polysubstance use (29, 30). Latent Class Analysis (LCA) is a type of finite mixture model that is used to identify and describe homogenous subgroups within a heterogeneous population based on the similarity of their response patterns (31). This method has been widely used in previous studies to examine substance use patterns (32–35).
LCA has been used in other studies to identify subgroups of substance use, misuse and addiction, e.g. to tobacco, internet etc. (36–40); notably, it has been used to differentiate problematic alcohol users from addicted users (41).
Regarding BZD addiction, LCA has been utilized by Votaw et al. (42) in a general population sample. In their study, the authors identified three distinct latent classes: limited polysubstance use class, binge alcohol and cannabis use class, and opioid use class.
However, to the best of our knowledge, the present study is the first to examine polysubstance use patterns among individuals who use BZDs as their primary drugs of choice in order to identify different classes of BZD users characterized by distinct substance combinations emerging from LCA.
Methods
Participants
All participants were in treatment for BZD detoxification at the Addiction Unit of the Verona University Hospital in Verona (Italy). During the study period (November 2003 to February 2020) 1,354 people were screened at the Addiction Unit of the Department of Internal Medicine at the Verona University Hospital for high-dose BZD dependence. Inclusion criteria were: being over 18 years of age; meeting the DSM-IV (43) criteria for benzodiazepine dependence, with more than 6 months' use; high dose benzodiazepines use (HDU). The DSM-IV criteria was applied by the clinician.
All patients also had to have so-called problematic use, defined by either mixing BZDs, escalating dosage, and/or using BZDs for recreational purposes (8, 9, 44). In accordance with previous literature (45, 46), the proposed detoxification program they enlisted in consisted in a 7-day continuous slow infusion of flumazenil (FLU-SI) in an inpatient setting, followed by interventions such as counseling, cognitive-behavioral therapy, and pharmacological therapy to prevent BZD relapse.
Patient demographics, the type of BZD they used and what it had been prescribed for, the duration of its use and its mean daily dose in the previous 3 months, its preferred administration route, comorbid abuse of other substances or other psychiatric disorders and detoxification attempts were assessed upon admission to our Unit.
The definition of what constitutes a “high dose” is still controversial and no real consensus exists about the appropriate clinical criteria that should be applied; in our study, we recommended inpatient treatment if a patient's BZD intake was at least 5 times higher than the maximum defined daily dose (DDD). Among the BZDs considered, we also included so-called Z-drugs. BZD use was quantified as standardized as diazepam dose equivalents.
The study was conducted according to the Declaration of Helsinki. Its protocol was approved by the ethics committee of the Verona University Hospital (approval code 683CESC) and fully adhered to its guidelines. Patients and controls gave written informed consent to participate in the study and to receive off-label administration of flumazenil (patients only).
Measures
Participants were asked questions regarding their demographic profile, including sex, age, age of first use, education, marital status, and employment status. BZD dependence duration was considered by converting doses to diazepam equivalents (DDDE, mg) (47) and calculating the mean diazepam dose/day.
Furthermore, participants' history of drug addiction and simultaneous drug use was assessed, considering alcohol, tobacco, cannabis/cannabinoids, amphetamines/methamphetamines, barbiturates/sedatives, cocaine, and heroin. To better quantify these variables, we assigned the following: (0) no drugs/alcohol used in the past 12 months, (1) previous history of drug/alcohol addiction, (2) addicted.
Information on these variables was mainly obtained from medical records. DDDE data were based on self-report.
Data Analysis
LCA was implemented to identify the use patterns of seven substances (other than BZDs): alcohol, tobacco, cannabis/cannabinoids, cocaine, and heroin. As the usage rate of amphetamines/methamphetamines and barbiturates/sedatives was low, we removed them from the analyses. LCA including one to six latent classes was estimated by employing the robust maximum-likelihood estimator (MLR) MPlus 7. The LCA was conducted by using 5,000 random sets of start values and 1,000 iterations, and the 500 best solutions were retained for final stage optimization (48, 49). In deciding how many classes should be retained, we considered the statistical appropriateness and consistency with respect to the theoretical meaning and conformity of the extracted classes (50–53).
Different information criteria (IC)-based fit statistics were examined in selecting numbers of classes. ICs follow the principle of parsimony controlling for overfitting and providing a standardized way to balance sensitivity and specificity (54). The following ICs were considered: the Bayesian Information Criterion [BIC; (55)], the Akaike Information Criterion [AIC; (56)], the Constant AIC (CAIC), the Sample Adjusted Bayesian information criterion (SABIC), and the bootstrapped likelihood ratio [BLRT; (57)]. The BLRT test compares the improvement between K-class model with a K-1 class model, providing p-values that can be used to justify the inclusion of one more class. Finally, we examined the accuracy with which models classify individuals into their most likely class by considering the entropy of each model. Entropy values range from 0 to 1 and indicate the clarity of class specification, with scores closer to 1 indicating better fit of the data into the prescribed class structure. According to the recommended fit indices (52), the optimal class solution would have the lowest BIC values, lowest AIC values, lowest CAIC values, lowest SABIC values, a significant BLRT p value, relatively higher entropy values, and conceptual and interpretive meaning. Furthermore, when comparing a K-class model with a K-1 class model, a significant BLRT test indicates that the model with K classes is optimal.
Furthermore, information criteria were depicted through “elbow plots” showing the improvements related with additional classes (53). More specifically, the optimal number of classes should be the value at which the slope flattens, plus and minus a class.
Then, we analyzed the associations between the identified classes and the sociodemographic variables of the participants. In this sense, the consideration of predictors should not qualitatively change the classes (49). More specifically, we regressed the latent classes on age, age of first use, sex, and employment (yes/no) in a series of multinomial logistic regressions. The R3STEP method in MPlus (58–60) was used.
Results
Participant Characteristics
A cross-sectional survey study was carried out. From the starting 1,354 questionnaires, 265 were removed because of missing responses (> 5%) about relevant variables to this study. The final sample comprised 1,088 subjects.
As shown in Table 1, slightly more than half (51%) were female and the mean age was 45.85 (SD ± 10.82) years. Regarding employment, 53.5% were employed. Characteristics of the sample are summarized in Table 1.
Latent Class Analysis
Fit indices resulting from the latent profile models containing up to 6 classes are provided in Table 2.
Taken as a whole, the 2-, and 3- class solutions showed the better fit as they were supported by the BIC and ABIC values, and BLRT tests (Table 2). Then, we compared the 2-, and 3- class solutions. Comparisons of the AIC, BIC, and ABIC values for all the models were contrasted in an elbow plot (Figure 1). Nylund et al. (52) suggest that lower BIC, AIC, CAIC and ABIC values indicate a better fit in class selection. However, for both 2- and 3- class solutions those values did not differ greatly across models. In addition, although BLRT distinguishes between class models (52), BLRT significance values did not differ across the two solutions, so we examined both options considering whether classes were theoretically meaningful and interpretable. We inspected the proportion of participants in each class finding that, concerning the 3-class solution, the smallest class drastically dropped to <5%. Although the principle of parsimony is generally to be followed, in our case adding a third class resulted in the addition of a well-defined, qualitatively distinct and theoretically meaningful class. Thus, the interpretability and clinical utility of the 3-class model was superior. This solution provided a reasonable level of classification accuracy, with an entropy value of 0.861. These results clearly suggest the high level of classification accuracy of these solutions, with average posterior probabilities of class membership varying from 0.69 to 0.96 (M = 0.85), with low cross-probabilities, ranging from 0.014–0.189 (M = 0.072).
The retained 3-class solution is represented in Table 2. Class 1 represents 4.3% of the sample (n = 47, latent class membership probability = 0.69) and participants in this class had the highest probabilities of using cocaine (53%), and alcohol (56%). Thus, this class was labeled as Polysubstance BZD users. Class 2 represents 17.2% of the sample (n = 186, latent class membership probability = 0.91) and participants in this class had the highest probabilities of being former heroin (62%), cocaine (77%), THC (66%), and alcohol users (36%). Thus, this class was labeled as Former polysubstance BZD users. Finally, class 3 represents 78.5% of the sample (n = 855, latent class membership probability = 0.96) and participants in this class had the highest probabilities of not using heroin (99%), cocaine (94%), THC (95%), and alcohol (77%). Thus, this class was labeled as mono-dependence BZD users.
Table 3 shows the sociodemographic predictors of the LC membership, including sex, age, age of first use, and employment. We performed a series of logistic regression analyses (R3STEP) where the categorical latent class variable was regressed on sex (0 = male; 1 = female), employment (yes/no), and the continuous age variables (Table 4). This analysis showed that males were more likely than females to be in class 1 (Polysubstance BZD users; OR = 17.83), and class 2 (Former polysubstance BZD users OR = 7.69) compared to class 3 (BZD users). Younger individuals were more likely to be in class 1 (Polysubstance BZD users; OR = 1.13) and class 2 (Former polysubstance BZD users OR = 1.09) compared to class 3. Finally, employed individuals were less likely to be in class 2 (Former polysubstance BZD users OR = 1.62) compared to class 3 (BZD users). Concerning the age of first use, no statistical differences were found among the three classes.
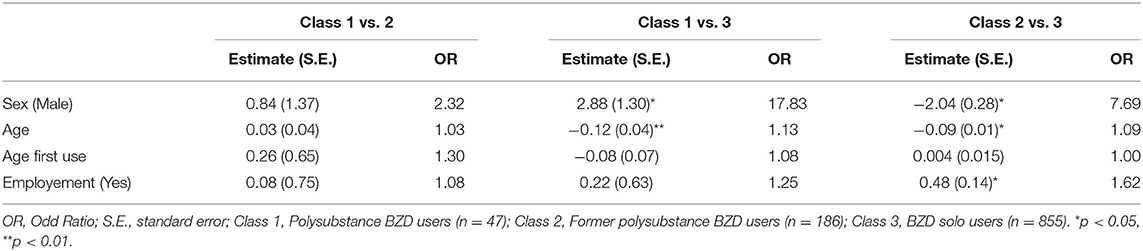
Table 4. Odds coefficients for the 3-class model with sex, age, age first use, and employment as covariates.
Discussion
Over the past 40 years, BZD dependence has been on the rise as a public health concern around the world (61). The present study aims to examine patterns of polysubstance use among a sample of Italian adults with BZD dependence.
Given its clinical relevance, we aimed to disentangle the patterns of polysubstance use among a sample of Italian adults that were also misusing BZDs. Our findings revealed three main types of BZD-HD users: of these, the vast majority only abused BZDs (78.5% of the sample), while the other two groups were either more likely polydrug users or former polydrug users. These results highlight that, while the overlap between BZD use and the abuse of other substances is well-documented, a large portion of BZD users are actually less likely to also be using heroin, cocaine and alcohol, as was also reported in other studies (42, 61).
However, the percentage of users who showed a high probability of polyabuse or of being former polyabusers is far from negligible. The simultaneous use of BZDs and other substances is especially concerning given that it may increase the risk of overdose (25), and these two classes of subjects present high treatment complexity.
It is important to note that biological, psychological and social factors influence personal prognoses and treatment responses (62, 63). Many adverse consequences are associated with polydrug abuse, such as an increased fatal and non-fatal overdose (64, 65), self-harm (66), infectious disease (67, 68), risky sexual behavior/risky injection practices (68, 69), criminal involvement (70–72), suicidal ideation/attempt (73, 74), violence and reckless driving (39, 75), mental and physical impairment (75, 76) and social dysfunction (77).
However, the association between these risks and polyabuse (also including BZDs) is not completely clear. Some studies point toward BZD dependence increasing them by markedly increasing disinhibition (73, 74).
Another important aspect is the fact that BZD dependence with greater psychological severity also have poorer treatment outcomes (73, 74, 78).
The most frequent type of patients that we treat in our Addiction Unit is assignable to Class 3, which has the highest probability of not using any substance other than high doses of BZDs. For these patients, treatment with FLU-SI was shown to be efficacious (7).
There seems to be a gender gap concerning the prescription of psychoactive drugs, with BZDs more frequently prescribed to women (79, 80). This study suggests differences in the psychopathology underlying high-dose BZD use: on one hand males tend toward polydrug use, and on the other hand BZD-only users are for the most part female. The latter scenario might be due to several causes, including females being more prone toward anxiety and mood disorders requiring medication, and the tendency to prescribe BZDs more to women (81, 82). Women, in order to soothe psychological distress, tend to call on medical attention more than men, who more frequently resort to other means outside the healthcare system, e.g. alcohol use (83).
The third class is the most numerous among women, also suggesting that BZD addiction could be a cross phenomenon, not only concerning subjects with a history of polyaddictions. This is interesting, because our results suggests that BZD addiction could be a problem that involves a large segment of the general population.
Concerning both age and sex, we found that young men had a higher probability of being included in classes 1 (Poly-substance BZD users) and 2 (Former polysubstance BZD users). Several studies in the general population support the fact that the male sex and a younger age are associated with binge alcohol and cannabis use (33, 84). Women with cocaine and heroin addiction seem less likely than men to develop a comorbidity to alcohol (85). Since the 1980s, studies on heroin and cocaine users have indicated that women present a shorter-lasting addiction than men, and they enter in treatment at a younger age (86, 87). Westermeyer and Boedicker (88), regarding the abuse of multiple substances and their respective treatment, indicated that women progressed more quickly from drug use to dependence: that is, women used each drug (except cocaine) for a shorter period, while rates of dependence remained constant. Moreover, women entering treatment exhibited a more severe clinical profile due to the greater consequences of drug use/abuse in women relative to men (85, 89–91).
The present analysis had several methodological limitations. First, data from the present study are cross-sectional, therefore we cannot make causal conclusions about findings. Second, data were from retrospective, self-report measures. There is the risk that substance use could be underreported when comparing self-report measures with biological markers (92). In fact, despite the self-report measures finding a wide use in the context of substance abuse problems (93, 94), their use is still a matter of debate, due to the limitations related to their use, such as the patients' desire to show a positive self-image or difficulties in remembering consumption episodes and dosages taken (95). Third, information on lifetime or past year use was not available for all substances except BZDs. Fourth, actually there is no a clear definition of high dose of BZD. Finally, we were unable to examine subgroup differences between types of non-medical prescription BZD use.
Conclusion
The present study underlines three different classes of BZD high dose abusers. The third class is the most represented and presents a mono-addiction (high dose BZD addiction). Our results and clinical experience highlight the need for a stricter control of BZD use, ranging from prescriptions to sales. While other BZD abuser studies show a female prevalence, our sample was more balanced regarding sex, but this is the first study with this peculiarity. This study also underlines the potential of LCA in improving knowledge of BZD abusers. Since LCA identifies homogeneous subgroups, this division could be used to plan and choose different and specific treatments. Further studies with LCA could be crucial especially in the field of BZD addiction, which would greatly benefit from more detailed studies.
Data Availability Statement
The raw data supporting the conclusions of this article will be made available by the authors, without undue reservation.
Ethics Statement
The studies involving human participants were reviewed and approved by 2822CESC. The patients/participants provided their written informed consent to participate in this study.
Author Contributions
FL and RC were responsible for the study concept and design. IP, RC, and LZ contributed to the data acquisition. IP assisted with the data analysis and interpretation of findings. LZ, IP, FF, TZ, AB, and AC drafted the manuscript. All authors critically reviewed the content and approved the final version of the manuscript for publication.
Conflict of Interest
The authors declare that the research was conducted in the absence of any commercial or financial relationships that could be construed as a potential conflict of interest.
Publisher's Note
All claims expressed in this article are solely those of the authors and do not necessarily represent those of their affiliated organizations, or those of the publisher, the editors and the reviewers. Any product that may be evaluated in this article, or claim that may be made by its manufacturer, is not guaranteed or endorsed by the publisher.
References
1. Soyka M. Treatment of benzodiazepine dependence. N Engl J Med. (2017) 376:1147–57. doi: 10.1056/NEJMra1611832
2. Lader M. Benzodiazepine harm: how can it be reduced? Br J Clin Pharmacol. (2014) 77:295–301. doi: 10.1111/j.1365-2125.2012.04418.x
3. López-Muñoz F, Alamo C, García-García P. The discovery of chlordiazepoxide and the clinical introduction of benzodiazepines: half a century of anxiolytic drugs. J Anxiety Disord. (2011) 25:554–62. doi: 10.1016/j.janxdis.2011.01.002
4. Sirdifield C, Anthierens S, Creupelandt H. General practitioners'experiences and perceptions of benzodiazepine prescribing: systematic review and meta-synthesis. BMC Fam Pract. (2013) 14:191. doi: 10.1186/1471-2296-14-191
5. Baldwin DS, Aitchison K, Bateson A. Benzodiazepines: risks and benefits. A reconsideration. J Psychopharmacol. (2013) 27:967–71. doi: 10.1177/0269881113503509
6. Faccini M, Leone R, Opri S, Casari R, Resentera C, Morbioli L, et al. Slow subcutaneous infusion of flumazenil for the treatment of long-term, high-dose benzodiazepine users: a review of 214 cases. J Psychopharmacol. (2016) 30:1047–53. doi: 10.1177/0269881116647505
7. Tamburin S, Faccini M, Casari R, Federico A, Morbioli L, Franchini E, et al. Low risk of seizures with slow flumazenil infusion and routine anticonvulsant prophylaxis for high-dose benzodiazepine dependence. J Psychopharmacol. (2017) 31:1369–73. doi: 10.1177/0269881117714050
8. Lugoboni F, Mirijello A, Faccini M, Casari R, Cossari A, Musi Bissoli G, et al. Quality of life in a cohort of high-dose benzodiazepine dependent patients. Drug Alcoh Depend. (2014) 142:105–9. doi: 10.1016/j.drugalcdep.2014.06.020
9. Tamburin S, Federico A, Faccini M, Casari R, Morbioli L, Sartore V. Determinants of quality of life in high-dose benzodiazepine misusers. Int J Environ Res Public Health. (2017) 14:38. doi: 10.3390/ijerph14010038
10. Ohayon MM, Lader MH. Use of psychotropic medication in the general population of France, Germany, Italy, and the United Kingdom. J Clin Psychiatry. (2002) 63:817–25. doi: 10.4088/JCP.v63n0912
11. Petitjean S, Ladewig D, Meier CR, Amrein R, Wiesbeck GA. Benzodiazepine prescribing to the Swiss adult population: results from a national survey of community pharmacies. Int Clin Psychopharmacol. (2007) 22:292–8. doi: 10.1097/YIC.0b013e328105e0f2
12. Barker MJ, Greenwood KM, Jackson M, Crowe SF. Cognitive effects of long-term benzodiazepine use: A meta-analysis. CNS Drugs. (2004) 18:37–48. doi: 10.2165/00023210-200418010-00004
13. Boeuf-Cazou O, Bongue B, Ansiau D, Marquié JC, Lapeyre-Mestre M. Impact of long-term benzodiazepine use on cognitive functioning in young adults: the VISAT cohort. Eur J Clin Pharmacol. (2011) 67:1045–52. doi: 10.1007/s00228-011-1047-y
14. Finkle WD, Der JS, Greenland S, Adams JL, Ridgeway G, Blaschke T, et al. Risk of fractures requiring hospitalization after an initial prescription for zolpidem, alprazolam, lorazepam, or diazepam in older adults. J Am Geriatr Soc. (2011) 59:1883–90. doi: 10.1111/j.1532-5415.2011.03591.x
15. Fond G, Berna F, Boyer L, Godin O, Brunel L, Andrianarisoa M. Benzodiazepine long-term administration is associated with impaired attention/working memory in schizophrenia: Results from the national multicentre FACE-SZ data set. Eur Arch Psychiatry Clin Neurosci. (2018) 268:17–26. doi: 10.1007/s00406-017-0787-9
16. Helmes E, Østbye T. Associations between benzodiazepine use and neuropsychological test scores in older adults. Can J Aging. (2015) 34:207–14. doi: 10.1017/S0714980815000082
17. Kok L, Slooter AJ, Hillegers MH, van Dijk D, Veldhuijzen DS. Benzodiazepine use and neuropsychiatric outcomes in the ICU: a systematic review. Critic Care Med. (2018) 46:1673–80. doi: 10.1097/CCM.0000000000003300
18. Picton JD, Marino AB, Nealy KL. Benzodiazepine use and cognitive decline in the elderly. Am J Health Syst Pharm. (2018) 75:e6–e12. doi: 10.2146/ajhp160381
19. Puustinen J, Lähteenmäki R, Polo-Kantola P, Salo P, Vahlberg T, Lyles A, et al. Effect of withdrawal from long-term use of temazepam, zopiclone or zolpidem as hypnotic agents on cognition in older adults. Eur J Clin Pharmacol. (2014) 70:319–29. doi: 10.1007/s00228-013-1613-6
20. van der Sluiszen NNJJM, Vermeeren A, Jongen S, Jongen S, Vinckenboschm F, Ramaekers JG. Influence of long-term benzodiazepine use on neurocognitive skills related to driving performance in patient populations: a review. Pharmacopsychiatry. (2017) 50:189–96. doi: 10.1055/s-0043-112755
21. Wedmann F, Himmel W, Nau R. Medication and medical diagnosis as risk factors for falls in older hospitalized patients. Eur J Clin Pharmacol. (2019) 75:1117–24. doi: 10.1007/s00228-019-02668-3
22. Ashton H. The diagnosis and management of benzodiazepine dependence. Curr Opin Psychiatry. (2005) 18:249–55. doi: 10.1097/01.yco.0000165594.60434.84
23. Lader M. Benzodiazepines revisited – will we ever learn? Addiction. (2011) 106:2086–109. doi: 10.1111/j.1360-0443.2011.03563.x
24. Schmitz A. Benzodiazepine use, misuse, and abuse: a review. Ment Health Clin 6. (2016) 120–6. doi: 10.9740/mhc.2016.05.120
25. Jones JD, Mogali S, Comer SD. Polydrug abuse: a review of opioid and benzodiazepine combination use. Drug Alcohol Depend. (2012) 125:8–18. doi: 10.1016/j.drugalcdep.2012.07.004
26. Fairbank J, Gwilym SE, France JC, Daffner SD, Dettori J, Hermsmeyer J, et al. The role of classification of chronic low back pain. Spine. (2011) 36:19–42. doi: 10.1097/BRS.0b013e31822ef72c
27. Foster NE, Hill JC, Hay EM. Subgrouping patients with low back pain in primary care: Are we getting any better at it? Man Ther. (2011) 16:3–8. doi: 10.1016/j.math.2010.05.013
29. Lanza ST, Rhoades BL. Latent class analysis: an alternative perspective on subgroup analysis in prevention and treatment. Prev Sci. (2013) 14:157–68. doi: 10.1007/s11121-011-0201-1
30. Shaw SY, Shah L, Jolly AM, Wylie JL. Identifying heterogeneity among injection drug users: a cluster analysis approach. Am J Public Health. (2008) 98:1430–7. doi: 10.2105/AJPH.2007.120741
31. Lubke GH, Muthén B. Investigating population heterogeneity with factor mixture models. Psychol Methods. (2005) 10:21–39. doi: 10.1037/1082-989X.10.1.21
32. Blow FC, Walton MA, Barry KL, Murray RL, Cunningham RM, Massey LS, et al. Alcohol and drug use among patients presenting to an inner-city emergency department: a latent class analysis. Addict Behav. (2011) 36:793–800. doi: 10.1016/j.addbeh.2010.12.028
33. Chen LY, Crum RM, Martins SS, Kaufmann CN, Strain EC, Mojtabai R. Patterns of concurrent substance use among nonmedical ADHD stimulant users: results from the National Survey on Drug Use and Health. Drug Alcohol Depend. (2014) 142:86–90. doi: 10.1016/j.drugalcdep.2014.05.022
34. Scherer M, Harrell P, Romano E. Marijuana and other substance use among motor vehicle operators: a latent class analysis. J Stud Alcohol Drugs. (2015) 76:916–23. doi: 10.15288/jsad.2015.76.916
35. Shiu-Yee K, Brincks AM, Feaster DJ, Frimpong JA, Nijhawan A, Mandler RN, et al. Patterns of substance use and arrest histories among hospitalized HIV drug users: a latent class analysis. AIDS Behav. (2018) 22:2757–65. doi: 10.1007/s10461-017-2024-y
36. McCarthy DE, Ebssa L, Witkiewitz K, Shiffman S. Repeated measures latent class analysis of daily smoking in three smoking cessation studies. Drug Alcohol Depend. (2016) 165:132–42. doi: 10.1016/j.drugalcdep.2016.05.025
37. Mok JY, Choi SW, Kim DJ, Choi JS, Lee J, Ahn H, et al. Latent class analysis on internet and smartphone addiction in college students. Neuropsychiatr Dis Treat. (2014) 10:817–28. doi: 10.2147/NDT.S59293
38. Monga N, Rehm J, Fischer B, Brissette S, Bruneau J, El-Guebaly N, et al. Using latent class analysis (LCA) to analyze patterns of drug use in a population of illegal opioid users. Drug Alcohol Depend. (2007) 17:88. doi: 10.1016/j.drugalcdep.2006.08.029
39. Scherer JN, Silvestrin R, Ornell F, Roglio V, Sousa TRV, Von Diemen L, et al. Prevalence of driving under the influence of psychoactive substances and road traffic crashes among Brazilian crack-using drivers. Drug Alcohol Depend. (2016) 168:255–62. doi: 10.1016/j.drugalcdep.2016.09.017
40. Wainstein L, Victorri-Vigneau C, Sébille V, Hardouin JB, Feuillet F, Pivette J, et al. Pharmacoepidemiological characterization of psychotropic drugs consumption using a latent class analysis. Int Clin Psychopharmacol 26. (2011) 54–62. doi: 10.1097/YIC.0b013e3283400cb2
41. Jemberie WB, Padyab M, Snellman F, Lundgren LA. Multidimensional latent class analysis of harmful alcohol use among older adults: subtypes within the swedish addiction severity index registry. J Addict Med. (2020) 14:e89–99. doi: 10.1097/ADM.0000000000000636
42. Votaw VR, McHugh RK, Vowles KE, Witkiewitz K. Patterns of polysubstance use among adults with tranquilizer misuse. Subst Use Misuse. (2020) 55:861–70. doi: 10.1080/10826084.2019.1708118
43. American Psychiatric Association (1994). Diagnostic and Statistical Manual of Mental Disorders, Fourth Edition (DSM-IV). Washington, DC: APA.
44. Liebrenz M, Schneider M, Buadze A, Gehring MT, Dube A, Caflisch C. High-dose benzodiazepine dependence: a qualitative study of patients'perceptions on initiation, reasons for use, and obtainment. PLoS ONE. (2015) 10:e0142057. doi: 10.1371/journal.pone.0142057
45. Gerra G, Zaimovich A, Giusti F, Moi G, Brewer C. Intravenous flumazenil versus oxazepam tapering in the treatment of benzodiazepine withdrawal: a randomized, placebo-controlled study. Addict Biol. (2002) 7:385–95. doi: 10.1080/1355621021000005973
46. Hood SD, Norman A, Hince DA, Melichar JK, Hulse GK. Benzodiazepine dependence and its treatment with low dose flumazenil. Br J Clin Pharmacol. (2014) 77:285–94. doi: 10.1111/bcp.12023
47. Drug Alcohol Services South Australia. (2014). Benzodiazepines: Information for GPs. Available online at: https://www.sahealth.sa.gov.au/wps/wcm/connect/dbd6d500407749f193d6bb222b2948cf/Benzodiazepines+-+GPs+2017.pdf?MOD=AJPERES&CACHEID=ROOTWORKSPACE-dbd6d500407749f193d6bb222b2948cf-nwKa1Rl (accessed November 5, 2021).
48. Asparouhov T, Muthén B. Exploratory structural equation modeling. Struct Equ Model. (2009) 16:397–438. doi: 10.1080/10705510903008204
49. Morin AJS, Arens AK, Tran A, Caci H. Exploring sources of construct-relevant multidimensionality in psychiatric measurement: a tutorial and illustration using the composite scale of morningness. Int J Methods Psychiatr Res. (2016) 25:277–88. doi: 10.1002/mpr.1485
50. Litalien D, Guay F, Morin AJS. Motivation for PhD studies: scale development and validation. Learn Individ Diff. (2015) 41:1–13. doi: 10.1016/j.lindif.2015.05.006
51. Morin AJ, Meyer JP, Creusier J, Biétry F. Multiple-group analysis of similarity in latent profile solutions. Org Res Methods. (2016) 19:231–54. doi: 10.1177/1094428115621148
52. Nylund KL, Asparouhov T, Muthén B. Deciding on the number of classes in latent class analysis and growth mixture modeling. A Monte Carlo simulation study. Struct Equ Model. (2007) 14:535–69. doi: 10.1080/10705510701575396
53. Morin AJS, Arens AK, Marsh HW. A bifactor exploratory structural equation modeling framework for the identification of distinct sources of construct-relevant psychometric multidimensionality. Struct Equ Model. (2016) 23:116–39. doi: 10.1080/10705511.2014.961800
54. Dziak JJ, Coffman DL, Lanza ST, Li R, Jermiin LS. Sensitivity and specificity of information criteria. Brief Bioinformatics. (2020) 21:553–65. doi: 10.1093/bib/bbz016
55. Schwartz G. Estimating the dimension of a model. Ann Stat. (1978) 6:461–4. doi: 10.1214/aos/1176344136
58. Muthén LK, Muthén BO. Mplus User's Guide: Statistical Analysis With Latent Variables, 7th Edn. Los Angeles, CA: Muthén and Muthén (2012).
59. Asparouhov T, Muthén B. Multiple-group factor analysis alignment. Struct Equ Model. (2014) 21:1–14. doi: 10.1080/10705511.2014.919210
60. Asparouhov T, Muthén B. Auxiliary variables in mixture modeling: three-step approaches using Mplus. Struct Equ Model. (2014) 21:329–41. doi: 10.1080/10705511.2014.915181
61. Votaw VR, Geyer R, Rieselbach MM, McHugh RK. The epidemiology of benzodiazepine misuse: a systematic review. Drug Alcohol Depend. (2019) 200:95–114. doi: 10.1016/j.drugalcdep.2019.02.033
62. Croft P, Altman DG, Deeks JJ, Dunn KM, Hay AD, Hemingway H, et al. The science of clinical practice: disease diagnosis or patient prognosis? Evidence about “what is likely to happen” should shape clinical practice. BMC Med. (2015) 13:20. doi: 10.1186/s12916-014-0265-4
63. Pincus T, Kent P, Bronfort G, Loisel P, Pransky G, Hartvigsen J. Twenty-five years with the biopsychosocial model of low back pain-is it time to celebrate? A report from the twelfth international forum for primary care research on low back pain. Spine (Phila Pa 1976). (2013) 38:2118–123. doi: 10.1097/BRS.0b013e3182a8c5d6
64. European Monitoring Centre for Drugs and Drug Addiction. (2009). Selected Issue, Poly-Drug Use: Patterns and Responses. Luxembourg: Office for Official Publications of the European Communities.
65. Origer A, Lopes da Costa S, Baumann M. Opiate-and cocaine-related fatal overdoses in Luxembourg from 1985 to 2011: a study on gender differences. Eur Addict Res. (2014) 20:87–93. doi: 10.1159/000355170
66. Park S, Song H. Factors that affect adolescent drug users' suicide attempts. Psychiatry Investig. (2016) 13:360–3. doi: 10.4306/pi.2016.13.3.360
67. Bach P, Walton G, Hayashi K, Milloy MJ, Dong H, Kerr T, et al. Benzodiazepine use and hepatitis c seroconversion in a cohort of persons who inject drugs. Am J Public Health. (2016) 106:1067–72. doi: 10.2105/AJPH.2016.303090
68. Ickowicz S, Hayashi K, Dong H, Milloy MJ, Kerr T, Montaner JSG, et al. Benzodiazepine use as an independent risk factor for HIV infection in a Canadian setting. Drug Alcohol Depend. (2015) 155:190–4. doi: 10.1016/j.drugalcdep.2015.07.017
69. Tucker D, Hayashi K, Milloy MJ, Nolan S, Dong H, Kerr T, et al. Risk factors associated with benzodiazepine use among people who inject drugs in an urban Canadian setting. Addict Behav 52. (2016) 103–7. doi: 10.1016/j.addbeh.2015.10.002
70. Comiskey CM, Stapleton R, Kelly PA. Ongoing cocaine and benzodiazepine use: effects on acquisitive crime committal rates amongst opiate users in treatment. Drugs. (2012) 19:406–14. doi: 10.3109/09687637.2012.668977
71. Darke S, Ross J, Mills K, Teesson M, Williamson A, Havard A. Benzodiazepine use among heroin users: baseline use, current use and clinical outcome. Drug Alcohol Rev. (2010) 29:250–5. doi: 10.1111/j.1465-3362.2009.00101.x
72. Horyniak D, Dietze P, Degenhardt L, Agius P, Higgs P, Bruno R, et al. Age-related differences in patterns of criminal activity among a large sample of polydrug injectors in Australia. J Subst Use. (2016) 21:48–56. doi: 10.3109/14659891.2014.950700
73. Artenie AA, Bruneau J, Roy É, Zang G, Lespérance F, Renaud J, Tremblay J, et al. Licit and illicit substance use among people who inject drugs and the association with subsequent suicidal attempt. Addiction (Abingdon, England). (2015) 110:1636–43. doi: 10.1111/add.13030
74. Artenie AA, Bruneau J, Zang G, Lespérance F, Renaud J, Tremblay J, et al. Associations of substance use patterns with attempted suicide among persons who inject drugs: can distinct use patterns play a role? Drug Alcohol Depend. (2015) 147:208–14. doi: 10.1016/j.drugalcdep.2014.11.011
75. Herbeck DM, Brecht ML, Lovinger K, Raihan A, Christou D, Sheaff P. Poly-drug and marijuana use among adults who primarily used methamphetamine. J Psychoact Drugs. (2013) 45:132–140. doi: 10.1080/02791072.2013.785824
76. Hakansson A, Schlyter F, Berglund M. Associations between polysubstance use and psychiatric problems in a crim-inal justice population in Sweden. Drug Alcohol Depend. (2011) 118:5–11. doi: 10.1016/j.drugalcdep.2011.02.014
77. Martinotti G, Carli V, Tedeschi D, Di Giannantonio M, Roy A, Janiri L, et al. Mono- and polysubstance dependent subjects differ on social factors, childhood trauma, personality, suicidal behaviour, and comorbid Axis I diagnoses. Addict Behav. (2009) 34:790–793. doi: 10.1016/j.addbeh.2009.04.012
78. Naji L, Dennis BB, Bawor M, Plater C, Pare G, Worster A, et al. A prospective study to investigate predictors of relapse among patients with opioid use disorder treated with methadone. Subst Abuse. (2016) 10:9–18. doi: 10.4137/SART.S37030
79. Greenfield SF, Back SE, Lawson K, Brady KT. Substance abuse in women. Psychiatr Clin North Am. (2010) 33:339–55. doi: 10.1016/j.psc.2010.01.004
80. Olfson M, King M, Schoenbaum M. Benzodiazepine use in the United States. JAMA Psychiatry. (2015) 72:136–42. doi: 10.1001/jamapsychiatry.2014.1763
81. Simoni-Wastila L. Gender and psychotropic drug use. Med Care. (1998) 36:88–94. doi: 10.1097/00005650-199801000-00010
82. Lagro-Janssen AL. Medicine is not gender-neutral: influence of physician sex on medical care. Ned Tijdschr Geneeskd. (2008) 152:1141–5.
83. Franconi F, Campesi I. Farmacologia di genere. Boll SIFO. (2011) 57:157–74. doi: 10.1704/932.10219
84. Moss HB, Goldstein RB, Chen CM, Yi HY. Patterns of use of other drugs among those with alcohol dependence: associations with drinking behavior and psychopathology. Addict Behav. (2015) 50:192–8. doi: 10.1016/j.addbeh.2015.06.041
85. Center for Substance Abuse Treatment. Substance Abuse Treatment: Addressing the Specific Needs of Women.. Treatment Improvement Protocol (TIP) Series, No. 51, HHS Publication No. (SMA) 15-4426. Rockville, MD: Center for Substance Abuse Treatment (2009).
86. Hser YM, Anglin MD, Booth MW. Sex differences in addict careers: addiction. Am. J. Drug Alcohol Abuse. (1987) 13:231–51. doi: 10.1007/s002130050278
87. Denier CA, Thevos AK, Latham PK, Randall CL. Psycosocial and psychopathology differences in hospitalized male and female cocaine abusers: a retrospective chart review, Addict. Behav. (1991) 16:489–96. doi: 10.1016/0306-4603(91)90056-N
88. Westermeyer J, Boedicker AE. Course, severity, and treatment of substance abuse among women versus men. Am. J. Drug Alcohol Abuse. (2000) 26:523–35. doi: 10.1081/ADA-100101893
89. Jarque-Lopez A, Gonzalez-Reimers E, Rodriguez-Moreno F, Santolaria-Fernandez F, Lopez-Lirola A, Ros-Vilamajo R, et al. Prevalence and mortality of heavy drinkers in a general medical hospital unit. Alcohol Alcohol. (2001) 36:335–8. doi: 10.1093/alcalc/36.4.335
90. Perkins KA. Smoking cessation in women. Special considerations. CNS Drugs. (2001) 15:391–411. doi: 10.2165/00023210-200115050-00005
91. Hernandez-Avila CA, Roundsaville BJ, Kranzier HR. Opioid, cannabis, and alcohol-dependent women show more rapid progression to substance abuse treatment. Drug Alcohol Depend. (2004) 74:265–72. doi: 10.1016/j.drugalcdep.2004.02.001
92. Kim S, Puisis M. Conducting substance abuse research: incarcerated populations. In: VanGeest JB, Johnson TP, and Alemagno SA, editors., Research Methods in the Study of Substance Abuse. Cham: Springer (2017). p. 357–378. doi: 10.1007/978-3-319-55980-3_17
93. Finney JW. Assessing treatment and treatment processes. In: Allen J and Columbus R, editors. Assessing Alcohol Problems: A Guide for Clinicians and Researchers. Rockville: US Department of Health and Human Services, National Institute on Alcohol Abuse and Alcoholism (1995). p. 123–142.
94. Rosay AB, Najaka SS, Hertz DC. Differences in the validity of self-reported drug use across five factors: gender, race, age, type of drug, and offense seriousness. J Quant Criminol. (2007) 23:41–58. doi: 10.1007/s10940-006-9018-7
Keywords: benzodiazepine, addiction, latent class analysis, polyabusers, anxiety
Citation: Zamboni L, Portoghese I, Congiu A, Zandonai T, Casari R, Fusina F, Bertoldi A and Lugoboni F (2022) Polysubstance Use Patterns Among High Dose Benzodiazepine Users: A Latent Class Analysis and Differences Between Male and Female Use. Front. Psychiatry 13:811130. doi: 10.3389/fpsyt.2022.811130
Received: 08 November 2021; Accepted: 03 January 2022;
Published: 25 January 2022.
Edited by:
Danilo De Gregorio, Vita-Salute San Raffaele University, ItalyReviewed by:
Liana Fattore, CNR Neuroscience Institute (IN), ItalyB. N. Subodh, Post Graduate Institute of Medical Education and Research (PGIMER), India
Copyright © 2022 Zamboni, Portoghese, Congiu, Zandonai, Casari, Fusina, Bertoldi and Lugoboni. This is an open-access article distributed under the terms of the Creative Commons Attribution License (CC BY). The use, distribution or reproduction in other forums is permitted, provided the original author(s) and the copyright owner(s) are credited and that the original publication in this journal is cited, in accordance with accepted academic practice. No use, distribution or reproduction is permitted which does not comply with these terms.
*Correspondence: Lorenzo Zamboni, lorenzo.zamboni88@gmail.com