- 1College of Landscape and Tourism, Hebei Agricultural University, Baoding, China
- 2National Engineering Research Center for Floriculture, School of Landscape Architecture, Beijing Forestry University, Beijing, China
- 3College of Agronomy, Inner Mongolia Minzu University, Tongliao, China
Flowering time is crucial for the survival and reproduction. Prunus genus belongs to the Rosaceae family and includes several hundred species of flowering trees and shrubs with important ornamental and economic values. However, the molecular mechanism underlying early flowering in Prunus genus is unclear. Here, we utilized the genome and transcriptome of P. mume and P. persica to explore the transcriptional regulation mechanism of early flowering. Comparative genomics found that genes accounting for 92.4% of the total P. mume genome and 91.2% of the total P. persica genome belonged to orthogroups. A total of 19,169 orthogroups were found between P. mume and P. persica, including 20,431 corresponding orthologues and 20,080 collinearity gene pairs. A total of 305 differentially expressed genes (DEGs) associated with early flowering were found, among which FT, TLI65, and NAP57 were identified as hub genes in the early flowering regulation pathway. Moreover, we identified twenty-five transcription factors (TFs) from nine protein families, including MADS-box, AP2/ERF, and MYB. Our results provide insights into the underlying molecular model of flowering time regulation in Prunus genus and highlight the utility of multi-omics in deciphering the properties of the inter-genus plants.
Introduction
Flowering time is regarded as an environmental adaptive trait of plants, which is crucial for the survival and production of offspring (Ding et al., 2020; Gaudinier and Blackman, 2020). The flowering process of plants is influenced by a number of complex external environmental conditions (nutrient conditions, ambient temperature and photoperiod, etc.) and genetic factors (Andrés and Coupland, 2012; Freytes et al., 2021). The early flowering may result in damaged floral organs, insufficient ovule fertilization and less flower production. Late flowering may be detrimental to seed ripening and dispersal (Gaudinier and Blackman, 2020). Therefore, the flowering time directly affects the yield of cash crops and the timing of ornamental plants (Gaudinier and Blackman, 2020).
Plants have evolved complex molecular mechanisms to regulate flowering time (Gaudinier and Blackman, 2020). Autonomous, photoperiod, vernalization, and gibberellin pathways have been widely studied to be involved in flowering regulation in Arabidopsis thaliana (Johansson and Staiger, 2015; Xu and Chong, 2018; Sharma et al., 2020; Wu et al., 2020; Quiroz et al., 2021). In addition, age, trehalose 6-phosphate synthase (TPS), and thermosensory pathways have been proposed to be involved in the flowering process (Blázquez et al., 2003; Halliday et al., 2003; Balasubramanian et al., 2006; Wu and Poethig, 2006; Schwarz et al., 2008; Wahl et al., 2013). These pathways have also been studied in other plants, such as Oryza sativa, Glycine max and Medicago truncatula (Weller and Macknight, 2018; Shim and Jang, 2020; Lin et al., 2021). Among the other regulators, these pathways also share some key integrators of flowering time, such as the FLOWERING LOCUS T (FT), SUPPRESSOR OF OVER EXPRESSION OF CONSTANS (SOC1), LEAFY (LFY), and FLOWERING LOCUS C (FLC) (Simpson and Dean, 2002; Posé et al., 2012; Ó'Maoiléidigh et al., 2014; Maurya et al., 2020). The flowering process of woody plants is very different from that of herbaceous plants. Most woody plants have flower buds formed before winter but need a cold duration to flower in the temperate and cold zone (Fuente et al., 2015; Goeckeritz and Hollender, 2021). Therefore, flowering regulation in most woody plants is related to flower bud dormancy and chilling requirement (Luedeling, 2012; Yu et al., 2020). Some genes that regulate bud dormancy and flowering have been identified in woody plants, such as FT, DORMANCY ASSOCIATED MADS-box (DAM 1-6), SHORT VEGETATIVE PHASE (SVP), and APETALA1 (AP1) (Bielenberg et al., 2008; Jiménez et al., 2009; Canton et al., 2021; Li et al., 2021; Zhang et al., 2021b). However, the complete digging of the molecular mechanisms of flowering in deciduous trees still require more holistic studies.
Recently, the transcriptomic analysis to detect gene expression during flower bud dynamic changes has been widely used in woody plants (Zhang et al., 2018; Rothkegel et al., 2020; Zhang et al., 2021c). Prunus genus includes the best representative species, such as P. persica, P. mume, Prunus avium, and Prunus armeniaca (Zhang et al., 2018; Rothkegel et al., 2020; Yu et al., 2020; Canton et al., 2021; Zhang et al., 2021c; Canton et al., 2022). So far, 191 candidate genes associated with flowering time traits have been identified in P. mume using integrated phenotypic data, genome-wide association study (GWAS), differentially expressed genes (DEGs), and gene co-expression studies (Zhang et al., 2021c). DAM gene family members are significantly associated with flowering time and bud dormancy (Kai et al., 2018; Zhang et al., 2018). DAM gene family members have also been discovered during flower bud development in P. persica and P. armeniaca (Bielenberg et al., 2008; Leida et al., 2012; Yu et al., 2020). In addition to DAMs, other transcription factors and regulatory genes have been found in Prunus plants, such as SVP, AP1, AGAMOUS-LIKE 24 (AGL24), APETALA3 (AP3), SEPALLATA 1, 2, 3 (SEP1, 2, 3), and PISTILLATA (PI) (Wan et al., 2020; Yu et al., 2020; Canton et al., 2021; Zhang et al., 2021c). Integrated gene mining techniques have revealed that flowering-related genes are epigenetically regulated in Prunus plants. DNA methylation pattern variations were detected in P. avium flower buds in early winter (Rothkegel et al., 2020). The chromatin marks of H3K4me3 and H3K27me3 were found in P. persica flower buds during endodormancy and ecodormancy, and the expression of DAM1, DAM3, DAM4, and DAM5 genes were inhibited (Zhu et al., 2020; Canton et al., 2022).
Prunus genus consists of over 200 species of flowering trees and shrubs that mostly are deciduous (Kole, 2011; Jung et al., 2018). P. persica is one of a few temperate fruit crops that can be grown under diverse climatic conditions (Jung et al., 2018; Wang et al., 2021b). P. mume, an early flowering species that blooms in late winter or early spring before new leaves grow (Zhang et al., 2012). Both the species are diploid and highly genetically characterized tree species (Zhang et al., 2012; Verde et al., 2013). Although P. persica and P. mume are closely related, the flowering time of P. mume is much earlier than that of P. persica (Sánchez-Pérez et al., 2014). At the same time, there are also differences in flowering time between different varieties of Prunus plants (Shi et al., 2020; Yu et al., 2020). In this study, we focused on early flowering in Prunus plants. To understand how flowering time is regulated, we integrated comparative genome between P. persica and P. mume with transcriptional outputs to obtain a potential molecular model for regulating flowering time. We selected transcriptomic data on flowering times of the two Prunus species for mutual proof. We propose that flowering time regulation includes at least three processes: cold-adaptation, transcription, and flowering. Our findings not only deepen the understanding of the flowering time control but also extend molecular model of flowering time regulation.
Materials and methods
Plant materials
Four P. persica genotypes and one P. mume cultivar ‘Zao Lve’ were selected in this study. Four P. persica genotypes, named A340, A209, A323 and A318, were derived from F2 populations constructed by crossing two P. persica cultivars (male grandparent ‘Fla.92-2C’ and female grandparent ‘Contender’) showing differences in flowering time. Floral buds from A209 and A340 genotypes were collected at October 14th to 16th, 2015, November 23th, 2015, January 28th, 2016, and February 10th, 2016, respectively. Floral buds from A318 genotype were collected at October 14th to 16th, 2015, November 23th, 2015, January 28th, 2016, February 27th, 2016, and March 12th, 2016, respectively. Floral buds from A323 genotype were collected at October 14th to 16th, 2015, November 23th, 2015, January 28th, 2016, February 27th, 2016, and March 17th, 2016, respectively (Fan et al., 2010; Zhebentyayeva et al., 2013; Yu et al., 2020). P. mume cultivar ‘Zao Lve’ had the characteristics of freezing tolerance and early flowering time. Floral buds from ‘Zao Lve’ were collected at November 22th, 2015, December 14th, 2015, January 6th, 2016, and February 18th, 2016, respectively (Zhang et al., 2018).
Comparative genome analysis
The P. persica (v1.0), P. mume (wild mei), and A. thaliana (TAIR10) genome were obtained from the Genome Database for Rosaceae (GDR), P. mume genome project and Arabidopsis Information Resource (TAIR), respectively (Zhang et al., 2012; Berardini et al., 2015; Jung et al., 2018). The longest transcripts of the genes were extracted from the genome using Python tools (https://www.python.org/). We performed comparative genome analyses using OrthoFinder software (Emms and Kelly, 2015). The cluster granularity was performed by MCL inflation with default parameters (1.5) (Theodosiou et al., 2008). Then, the sequences were aligned using MAFFT software with FFT-NS-2 method (Katoh and Standley, 2013). Phylogenetic trees of all the orthologous groups were constructed using FastTree software (Price et al., 2009). We further constructed the phylogeny of P. persica, P. mume, and A. thaliana based on single-copy genes. The divergence times of plant species were estimated using the TimeTree website (Kumar et al., 2017). The syntenic relationships of genes in the whole genomes of P. persica and P. mume were determined based on genome sequences and annotation information. We first performed multiple sequence alignments using BLASTp software (Altschul et al., 1990), and then identified the tandem and collinearity genes using MCScanX software (Wang et al., 2012).
RNA-seq and gene expression analysis
The read count of the transcriptome was defined as the number of reads compared to the exon in high-throughput sequencing. The read count was obtained using HTseq-count software (Anders et al., 2014). First, we used the GenomicFeatures package included in the R software to convert the count into Fragments Per Kilobase Million (FPKM). The relationships between samples were evaluated using hierarchical clustering analysis (HCA) and principal component analysis (PCA). The identification of differentially expressed genes (DEGs) was carried out according to the method of Audic et al. (Audic and Claverie, 1997). The expression pattern and clustering of DEG were analyzed using the Pheatmap package included in the R software. The shared genes were analyzed and extracted using UpSet included in the EVenn tool and TBtool software, respectively (Chen et al., 2020; Chen et al., 2021). Correlation of gene expression was calculated using the ggplot2 package with the ‘lm’ method included in the R project.
Gene function annotation and enrichment analysis
We extracted the target sequences using TBtool software (Chen et al., 2020). We searched the target sequences for matching Pfam families using the HMMER website with the parameter set to E-value = 1 (Mistry et al., 2021). The relationship between homologous superfamilies and other InterPro entries was calculated by analyzing the overlap between matched sequence sets (Quevillon et al., 2005). The functional annotations and predictions for structure data of the sequences were analyzed using Protein Data Bank in Europe - Knowledge Base (PDBe-KB) (Mir et al., 2018). Gene Ontology (GO) database was used to annotate the cellular component, molecular function, and biological process of sequences (Ashburner et al., 2000). Kyoto Encyclopedia of Genes and Genomes (KEGG) database was used to enrich the pathways of the sequences (Kanehisa and Goto, 2000).
Transcription factor and gene expression analysis
Genome-wide TFs for P. persica were downloaded from the Plant Transcription Factor Database (PlantTFDB) (Jin et al., 2014). We manually retrieved the TFs from the target sequence and further confirmed TFs’ domain signatures using the Pfam database (Mistry et al., 2021). P. mume TF orthologues were retrieved based on comparative genome orthologues file. The names, functional descriptions, and GO terms of TFs were analyzed in detail using the UniProt database (The UniProt, 2021). The expression pattern of TF genes was analyzed using the boxplot package included in the R software.
Molecular model of regulating flowering time analysis
To understand the molecular model of flowering time regulation, we integrated annotation information from multiple databases and the changed into gene expression characteristics. Protein-protein interaction networks and functional enrichment analysis were analyzed according to the orthologs of A. thaliana using the STRING database (Pertea et al., 2015). Protein-protein interaction networks were visualized using Cytoscape software (Otasek et al., 2019). Here, we manually filtered KEGG and GO annotation information. The functions of the target sequences were classified based on the functional annotations of the UniProt database (The UniProt, 2021).
Results
Genomic characterization of P. mume and P. persica
To better understand the genetic background, we compared genome-wide sequences between P. mume and P. persica, and A. thaliana as the outgroup. Using OrthoFinder, 88.2% (75,090 genes) of the total sequences were assigned to 19,299 orthogroups. A total of 10,730 orthogonal groups were identified in P. mume, P. persica, and A. thaliana, of which 6,742 were composed entirely of single-copy genes (Table S1). We constructed a species tree by using 1,197 well-supported, non-terminal duplications. The estimated times of divergence for P. mume and P. persica indicated a relatively recent split (Figure 1A). 21,559 genes in A. thaliana, 29,020 genes in P. mume, and 24,511 genes in P. persica were assigned to orthologues (Figure 1B and Table S1). Moreover, the genes accounting for 92.4% of the total P. mume genome and 91.2% of the total P. persica genome belonged to orthogroups (Figure 1C and Table S1). We retrieved 1,952 species-specific orthogroups from all orthogroups, including 1,097 in A. thaliana, 569 in P. mume, and 286 in P. persica. Species-specific orthogroups included 6,120 A. thaliana genes, 3,257 P. mume genes, and 1,213 P. persica genes (Table S1). 19,173 orthogroups were found between P. mume and P. persica, including 20,435 corresponding orthologues (Table S2). At the same time, 5,902 resolved gene trees were constructed by orthogroups. 20,080 collinearity genes were identified between P. mume and P. persica (Figure 1D, Figure S1, and Table S3). 2210 and 1766 tandem duplication of gene pairs were identified in P. mume and P. persica, respectively (Tables S4). A total of 572 syntenic genomic blocks were identified between P. mume and P. persica (Figure 1E and Figure S2).
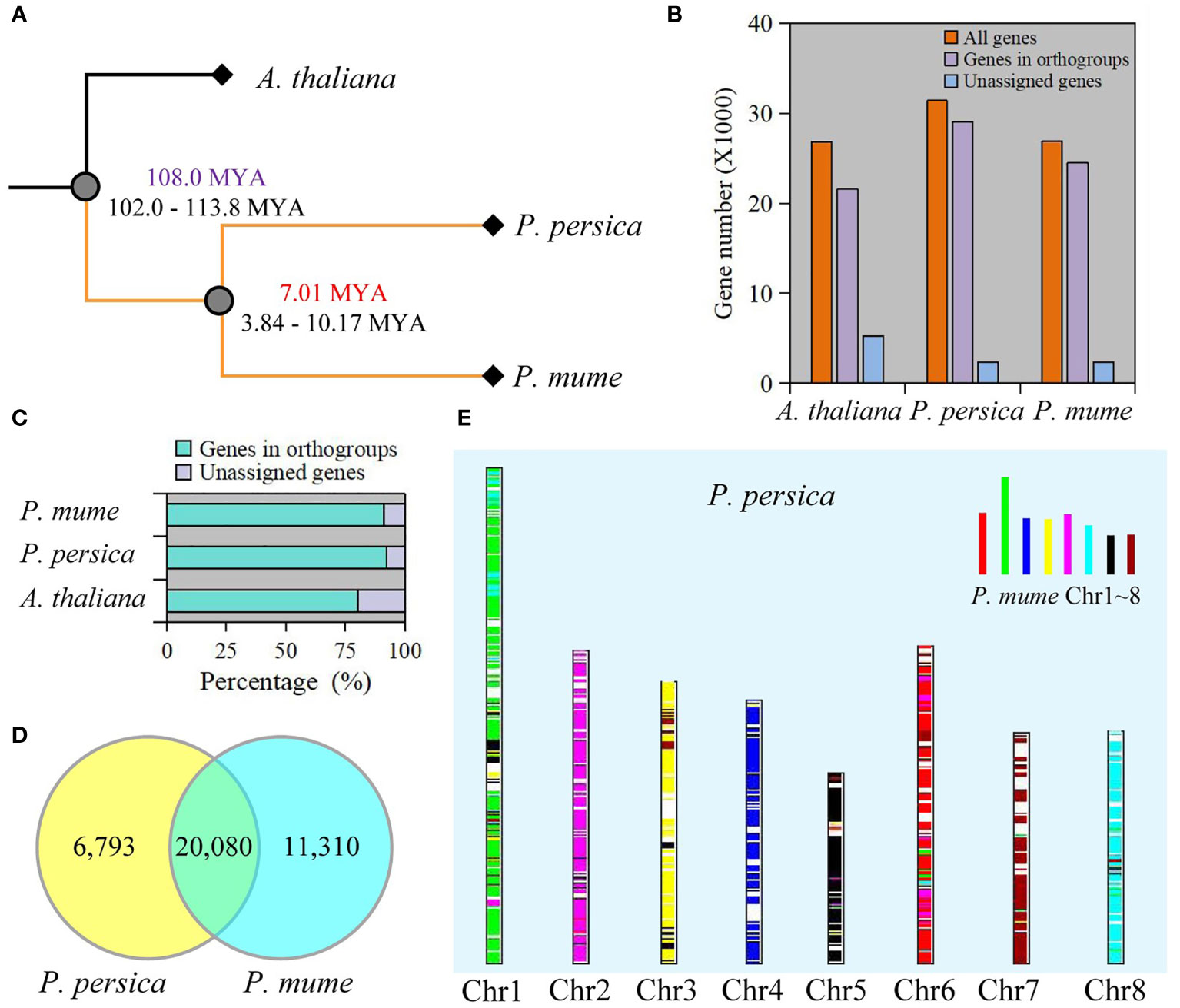
Figure 1 Comparative genomics between P. mume and P. persica. (A) The evolutionary relationships among A thaliana, P. mume, and P. persica. The evolutionary timescale of 102.0-113.8 Mya for the divergence between A thaliana and Prunus, and 3.84-10.17 Mya for the divergence between P. mume and P. persica. (B) The total number of genes in the genome, the number of genes in orthogroups, and the number of unassigned genes. (C) Percentage of genes in orthogroups and unassigned genes. (D) Collinearity genes comparison between P. mume and P. persica. (E) Comparison of the P. persica genome with the P. mume. Syntenic P. mume blocks are painted onto P. persica chromosomes.
Transcriptome profiles analysis identified DEGs from dormancy to pre-flowering stage
We compared the gene expression levels of P. mume, and P. persica at the pre-flowering stage with those at the previous stage (dormancy stage). A340, A209, A323, and A318 were derived from F2 populations obtained by crossing two P. persica cultivars (male grandparent ‘Fla.92-2C’ and female grandparent ‘Contender’) showing differences in flowering time, including A340 and A209. Here, 7,830, 6,401, 6,893, and 9,127 DEGs were identified in the A340, A209, A323, and A318 genotypes, respectively. The number of downregulated DEGs was more than upregulated DEGs in the four P. persica genotypes (Figure 2A). 1,968 downregulated DEGs and 3,025 upregulated DEGs were identified in the P. mume cultivar ‘Zao Lve’ (Figure 2A). The upregulated DEGs represented 30.8−46.3% of the total DEGs in the four P. persica genotypes from dormancy to pre-flowering. 1,080, 1,099, 1,328, and 1,360 upregulated DEGs were found in the A340, A209, A323, and A318 genotypes, respectively. We found that genotypes with similar flowering time characteristics had more shared genes (Figure 2B). 1,047 and 1,365 shared genes were obtained between A340 and A209 and between A323 and A318, respectively. 175 upregulated DEGs were shared in the four P. persica genotypes (Figure 2B). We extracted the expression data of 175 shared genes into the RNA-seq dataset and standardized the data (Figure S3 and Table S5). The shared DEGs were divided into five clusters based on gene expression patterns (Table S6). On the whole, the expression level of 13 genes in cluster I increased gradually from the S1 to P stages. At all stages, the genes in cluster II showed high expression levels, while the genes in cluster IV showed low expression levels. Most of the genes in cluster V were specifically highly expressed in the P stage (Figure 2C). Meanwhile, we searched for orthologues of P. mume corresponding to 175 shared genes from the four P. persica genotypes based on comparative genomic datasets. A total of 185 orthologues were retrieved from P. mume, including one-to-one and one-to-many (Table S7). 66 orthologues were upregulated from dormancy to pre-flowering stage in P. mume (Figure S4). Of the 175 shared genes, 114 collinearity genes were obtained between P. mume and P. persica, and the expression levels of 44 genes were upregulated from dormancy to pre-flowering (Figure 2D). These genes had similar expression patterns and were highly expressed at the P stage (Figure 2E and Table S8). The expression of the three genes (Pm010917, Pm003671, and Pm023232) was not detected in S1 and S2 stages and it gradually increased in the following stages (Table S8). Most of the genes in cluster II and III were specifically highly expressed in the P stage. 16 genes belonging to cluster V showed low expression levels (Figure 2E and Table S9). The shared genes were annotated to a variety of biological processes such as flower development, plant hormone signal transduction and defense response (Table S10).
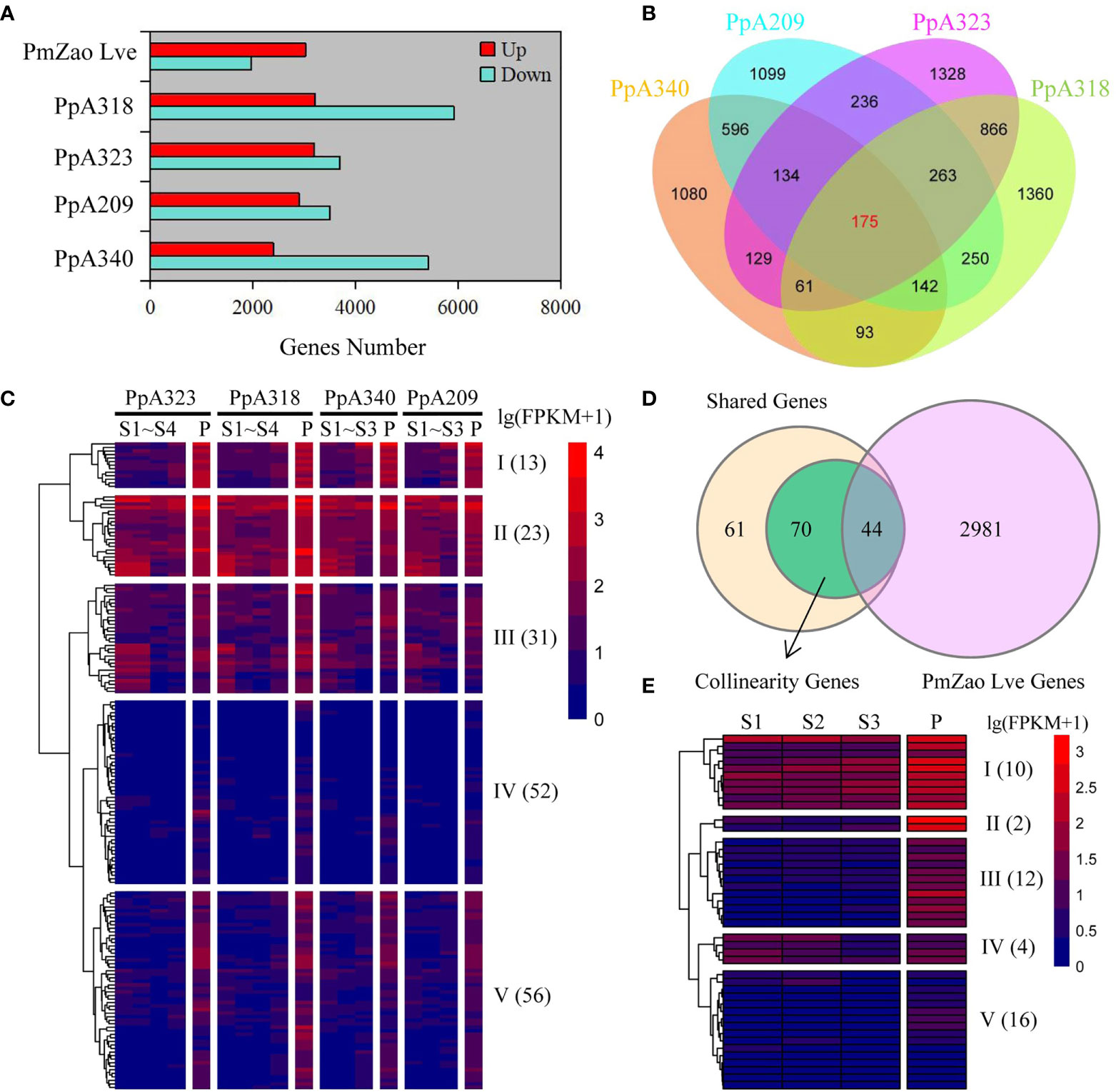
Figure 2 Identification of flowering-related DEGs and gene expression patterns. (A) DEGs from dormancy to pre-flowering stage in four P. persica genotypes (A209, A340, A318, and A323) and one P. mume cultivar (‘Zao Lve’ mei). (B) Venn diagram of four P. persica genotype DEGs from dormancy to pre-flowering stage. (C) Gene expression patterns of shared DEGs in four P. persica genotypes. (D) Overlap of shared DEGs in P. persica and P. mume from dormancy to pre-flowering stage with collinearity genes between P. persica and P. mume. (D, E) the gene expression patterns of shared genes in P. mume. The letter S stands for dormancy stage and P for pre-flowering stage.
Mining of early flowering genes from genotypic plants with significant differences in flowering time
In order to explore the regulatory genes of early flowering traits, we compared the DEGs with the same chilling requirement in different P. persica genotypes, among which A340 and A209 had the flowering ability. The upregulated DEGs accounted for 20.16% and 13.05% of the total genome-wide genes in A340 and A209, respectively (Figure 3A). From S3 to S4, although A318 and A323 did not have the flowering ability, the number of upregulated DEGs was higher than that of downregulated DEGs (Figure 3A). The fold differential expression of genes showed a positive correlation between genotypes with similar flowering times (Figures 3B, C). As representative early flowering genotypes, 439 upregulated DEGs were identified in A340 and A209. Here, 18,181 and 16,519 non-upregulated DEGs were extracted from A323 and A318, respectively. We obtained 305 shared genes based on different taxonomic groups of four genotypes (Figure 3D). To understand the biological relevance of shared genes, biological pathway enrichment and annotation of these genes were ascertained using KEGG databases. The functional category of shared genes mainly included genetic information processing, environmental information processing, carbohydrate metabolism, and metabolism of terpenoids and polyketides (Figure 3E). KEGG pathway enrichment analysis revealed numerous pathways related to environmental information processing, including plant hormone signal transduction, two-component system, MAPK signaling pathway-plant, phosphatidylinositol signaling system, and neuroactive ligand-receptor interaction. We retrieved 87 KO definitions, including methionyl aminopeptidase, plant G-box-binding factor, ABA-responsive element binding factor, MADS-box transcription factor, and protein FLOWERING LOCUS T (Table S11). 196 collinearity genes and 283 orthologues of these shared genes were identified in P. mume (Figure 3F and Table S12). Based on the orthologues of these shared genes, we constructed gene expression patterns in P. mume (Figure S5). We found that these genes had different gene expression patterns, which further narrowed the scope for screening hub genes that regulate early flowering traits.
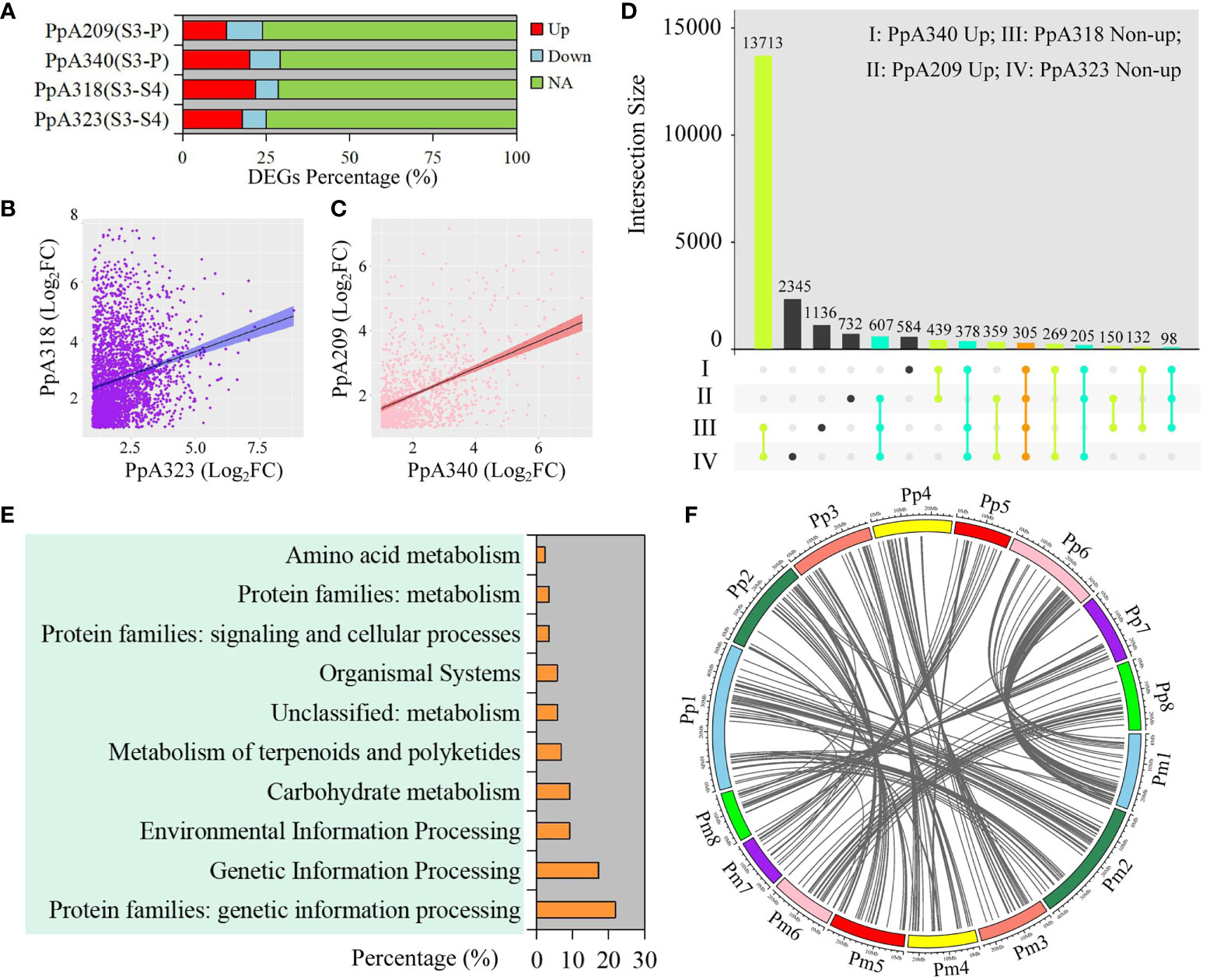
Figure 3 Identification and functional enrichment of early flowering-related DEGs. (A) Percentage of DEGs at different stages in four P. persica genotypes (A209, A340, A318, and A323). (B) Correlation of DEGs between A318 and A323 from S3 to S4 stages. (C) Correlation of DEGs between A318 and A323 from S3 to P stages. (D) Comparison of genes between pre-flowering stage in the A209 and A340 genotypes and dormancy stage in the A318 and A323 genotypes. Up represents upregulated DEGs, and non-up represents downregulated DEGs and genes with no significant difference change. (E) KEGG enrichment analysis of shared genes from (D). (F) Collinearity genes of orthologous sequences with shared genes in P. persica and P. mume.
Protein-protein interaction networks of early flowering associated proteins
In order to explore the gene functions and interactions that potentially regulate early flowering traits, proteins encoded by these candidate genes were annotated and their interactions were predicted. Here, annotation information was obtained for 220 proteins, including transcription factors, kinases, and functional proteins (Table S13). The predicted protein-protein interaction network for early flowering traits consisted of 111 proteins, around half of which were transcription factors. In total, 324 protein-protein interactions were found (Figure 4). Low-temperature-induced 65 kDa protein (LTI65) was the most interacting protein (13), followed by 60S ribosomal protein L26-1 (RPL26A) and H/ACA ribonucleoprotein complex subunit 4 (NAP57). There were fifteen proteins in the network that interacted with at least six proteins. We found that most proteins were associated with low-temperature stress, protein synthesis, and flowering pathways. Several proteins were involved in the CBF-COR signal transduction pathway, including four members of the dehydration-responsive element-binding protein (DREB) transcription factor family. Ribosomal components represented by members of the RPL family were responsible for the protein synthesis in the cell. FT, as a member of the phosphatidylethanolamine-binding protein (PEBP) family, played a key role in the mobile flower-promoting signal. We identified ten proteins interacting with FT, including floral homeotic protein AGAMOUS (AG), and AGL24. PI, AG, and AGL are the members of the MAD-box family, suggesting that MAD-box and PEBP family members played important roles in regulating early flowering traits. Glutaredoxin-C7 (ROXY1), as a regulator of petal primordia initiation and further petal morphogenesis, forms complex with the AG and regulates flower development. In addition, members of the bZIP transcription factor family (ABF4, GBF4) were also identified in the FT interaction network.
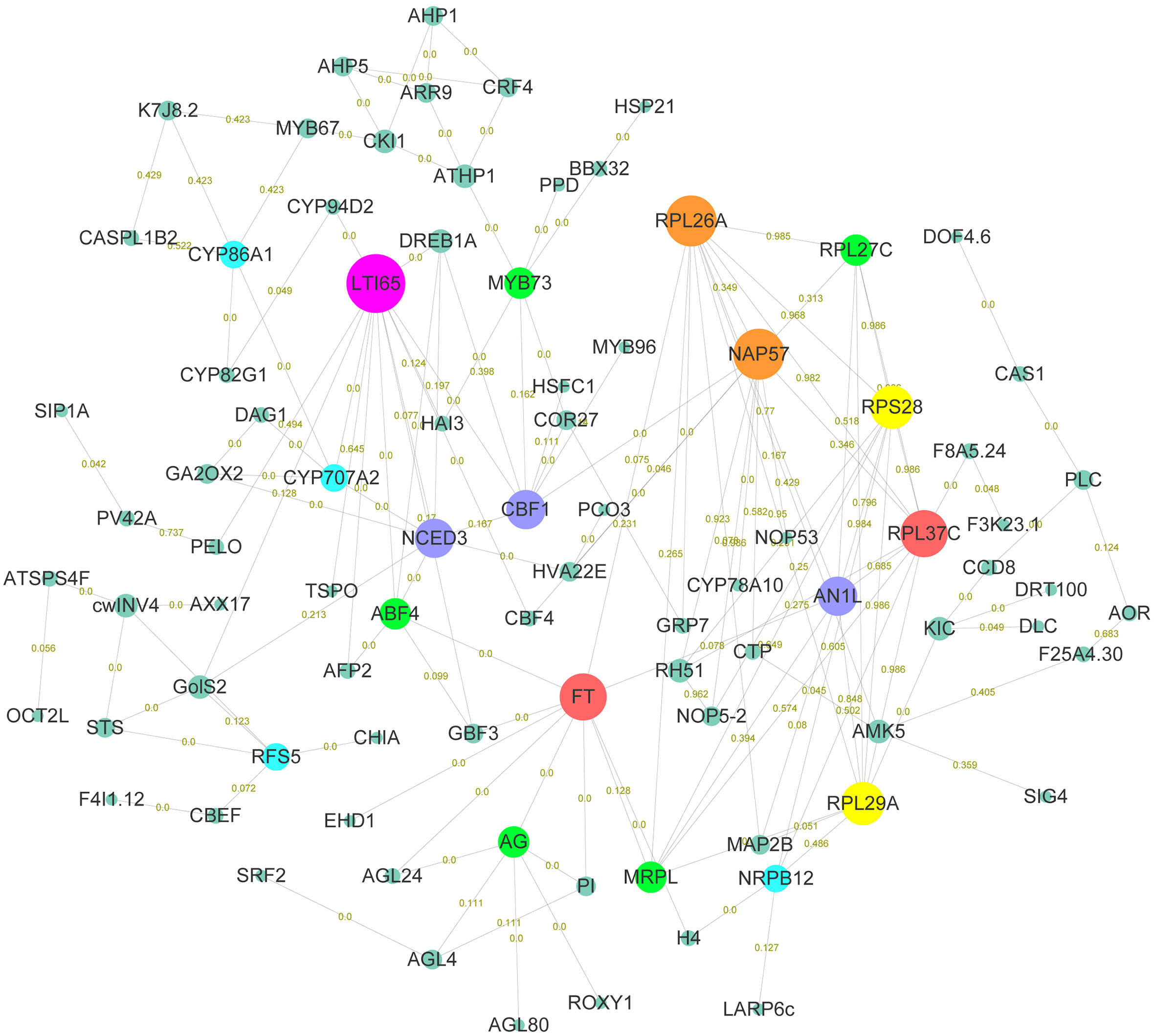
Figure 4 Functional enrichment analysis of early flowering-related genes and protein-protein interaction networks. Proteins are named based on the orthologous sequence of A. thaliana. The size of the circle represents the combined score of the STRING database rating. The connectedness is distinguished by different colors. The numbers on the connecting lines represent the coexpression of genes.
Transcription factor enrichment
TFs are a class of gene expression regulatory proteins, which play an important role in the regulation of plant flowering. Among the proteins associated with early flowering, TFs were inferred through the PlantTFDB and Pfam databases, respectively. Here, twenty-five TFs covering nine protein families were retrieved, and these TFs were named according to the orthologues following the rules for A. thaliana (Table 1). We identified six MADS-box family members, including AGL4, AGL24, AGL80, AG, PI, and SVP. MADS-box TFs interact with several transcriptional targets to mediate a diverse range of plant processes, including differentiation, flowering, transcription, and transcription regulation (Table 1 and Table S14). Twenty-four percent of all the identified TFs were apetala2/ethylene response factor (AP2/ERF) homologs, including ERF, RAV, and CBF/DREB subfamilies. Other TFs, including MYB, WRKY, bZIP, heat shock factor (HSF), homeobox protein (ATH), dof zinc finger protein (DOF), and auxin response factor (ARF), comprised 12%, 4%, 8%, 8%, 4%, 8%, and 4% of the total candidate TFs, respectively. These TFs regulate hormone signal transduction and synthesis pathways, including auxin, abscisic acid, ethylene, cytokinin-activated signaling pathway, and gibberellin biosynthetic process (Table 1 and Table S14). DAG1 and FAR1 were involved in response to red or far-red light. FAR1 negatively regulates leaf senescence and positively regulates circadian rhythm (Table S14). Among TFs involved in abiotic stress response, WRKY, MYB, and AP2/ERF represented a large protein family, respectively, which displayed diverse roles in various biological processes (Table S14). ATH1, as a specific activator of FLC expression, controls floral competency. ATH1 was involved in several biological processes, such as floral organ abscission, photomorphogenesis, regulation of gibberellin biosynthetic process, and vegetative to the reproductive phase transition of the meristem (Table S14).
MADS-box family members are the key TFs for flowering, and AP2/ERF family members play an important role in plant response to cold stress. Here, the expression patterns of MADS-box and AP2/ERF TF coding genes were analyzed in P. persica (Figure 5). The expression level of PI and AGL4 genes showed an upward trend from S1 to P stages in the early and late flowering genotypes. SVP gene showed different expression patterns in the early and late flowering genotypes. The expression of SVP was the highest in A340 genotype at the P stage, while at S4 stage the highest expression was observed in A318 genotype. The expressions of AG gene showed a changing trend from dropping at first to rising afterwards in early flowering genotypes. However, the expression of AG gene in late flowering genotypes showed a continuous downward trend. The expression of AGL80 gene showed the opposite trend in the two genotypes of P. persica. The expression of AGL80 gene in the P stage was the highest, which was 6.9 and 2.3 times higher than the expression in S1 and S3 stages, respectively (Figures 5A, B). ERF061 gene had a high basic expression level in the early and late flowering genotypes. The expressions of ERF061, CBF4, CBF1, CRF4, and DREB1A gene showed a changing trend from dropping at first to rising afterwards in early flowering genotypes. The expression pattern of these genes in late flowering was not as regular as that in early flowering genotypes. Overall, AP2/ERF TF coding genes were upregulated from S3 to P stages in early flowering genotypes. In late flowering genotypes, the expression of these genes showed a downward or constant trend from S3 to S4 stages and continued to the P stage (Figures 5A, B).
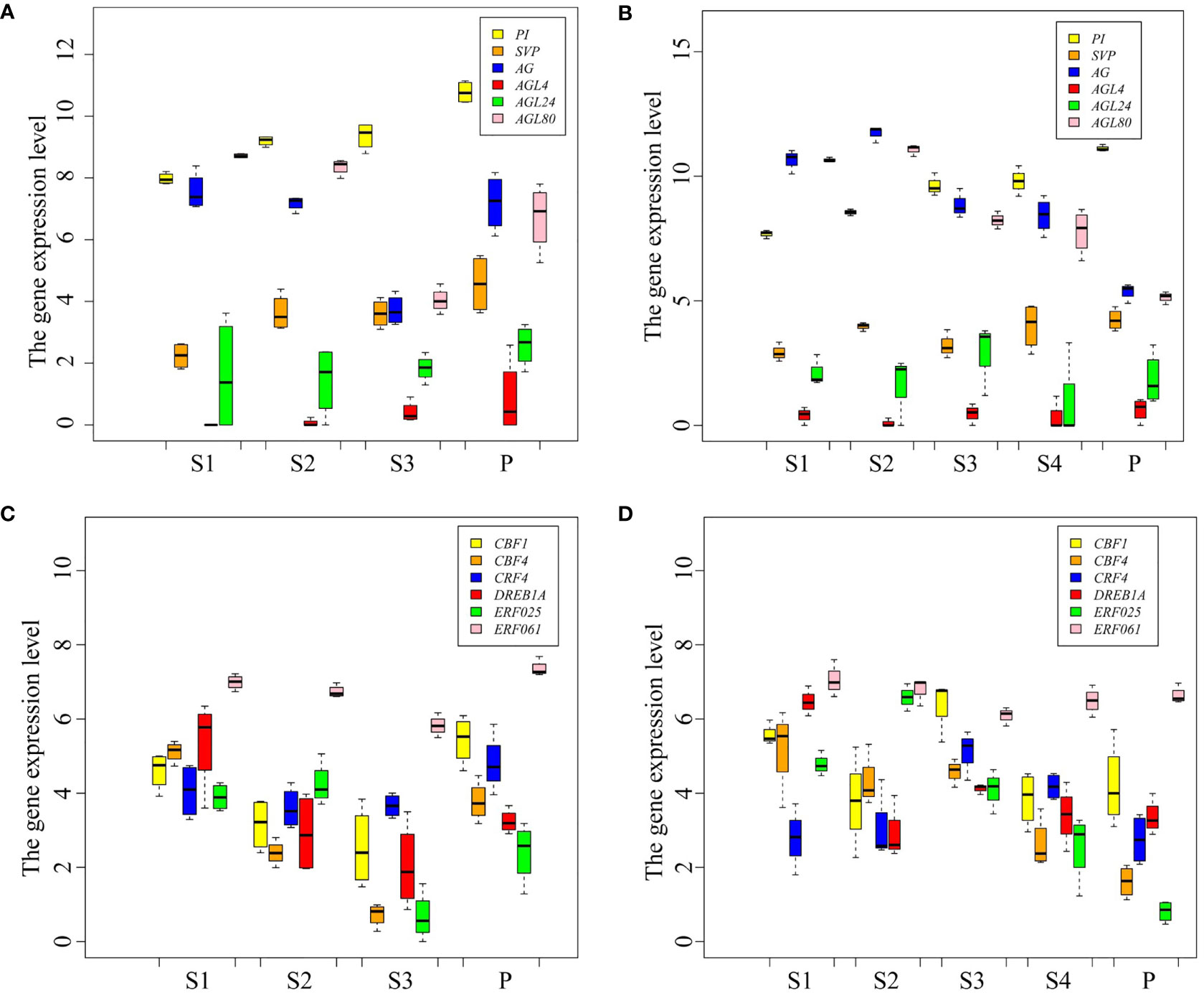
Figure 5 Expression patterns of transcription factor genes. (A, B) Expression levels of MAD-box TF genes in the early and late flowering genotypes, respectively. (C, D) Expression levels of AP2/ERF TF genes in the early and late flowering genotypes, respectively.
Potential molecular model of flowering time regulation
To investigate the molecular model of flowering time regulation, we selected three hub protein sets via connectivity in protein-protein interaction networks. FT belonged to the phosphatidylethanolamine-binding protein family and was involved in biological processes such as flower development, meristem determinacy, photoperiodism, flowering, and cell differentiation. FT had protein-protein interaction with ten proteins, including three MADS-box TFs (AG, AGL24, and PI) and two bZIP TFs (ABF4 and GBF3) (Figure 6A). GBF3 TF also had protein-protein interaction with LTI65 protein. Most proteins in LTI65 protein-protein interaction were closely related to abiotic stress. We identified three AP2/ERF TFs (CBF1, CBF4, and DREB1A), in addition to ninja-family protein (AFP2), 9-cis-epoxycarotenoid dioxygenase (NCED3), translocator protein homolog (TSPO) and Galactinol synthase 2 (GolS-2) proteins (Figure 6B). Nopp-140-associated protein of 57 kDa homolog (NAP57) catalyze pseudouridylation of rRNA, which plays a central role in ribosomal RNA processing. The NAP57 protein-protein interaction network shared CBF1, CBF4, and 60S ribosomal protein L26-A (RPL26A) with the FT and LTI65 protein-protein interaction networks. RPL26A, RPL27C, and RPL37C were annotated as the ribosomal proteins for molecular function and were involved in the biological process of transcription. Ribosome biogenesis proteins (NOP53 and NOP5-2) were associated with numerous RNAs including the 27S and 7S pre-rRNAs and the box H/ACA snoRNA snR37 (Figure 6C). FT, LTI65, and NAP57 protein-protein interaction networks were mainly involved in the molecular regulation of flowering, cold response and RNA transcription, respectively. Here, we constructed a potential molecular model for flowering time regulation. The molecular model of flowering time regulation included at least three processes: cold-adaptation, transcription, and flowering (Figure 6D).
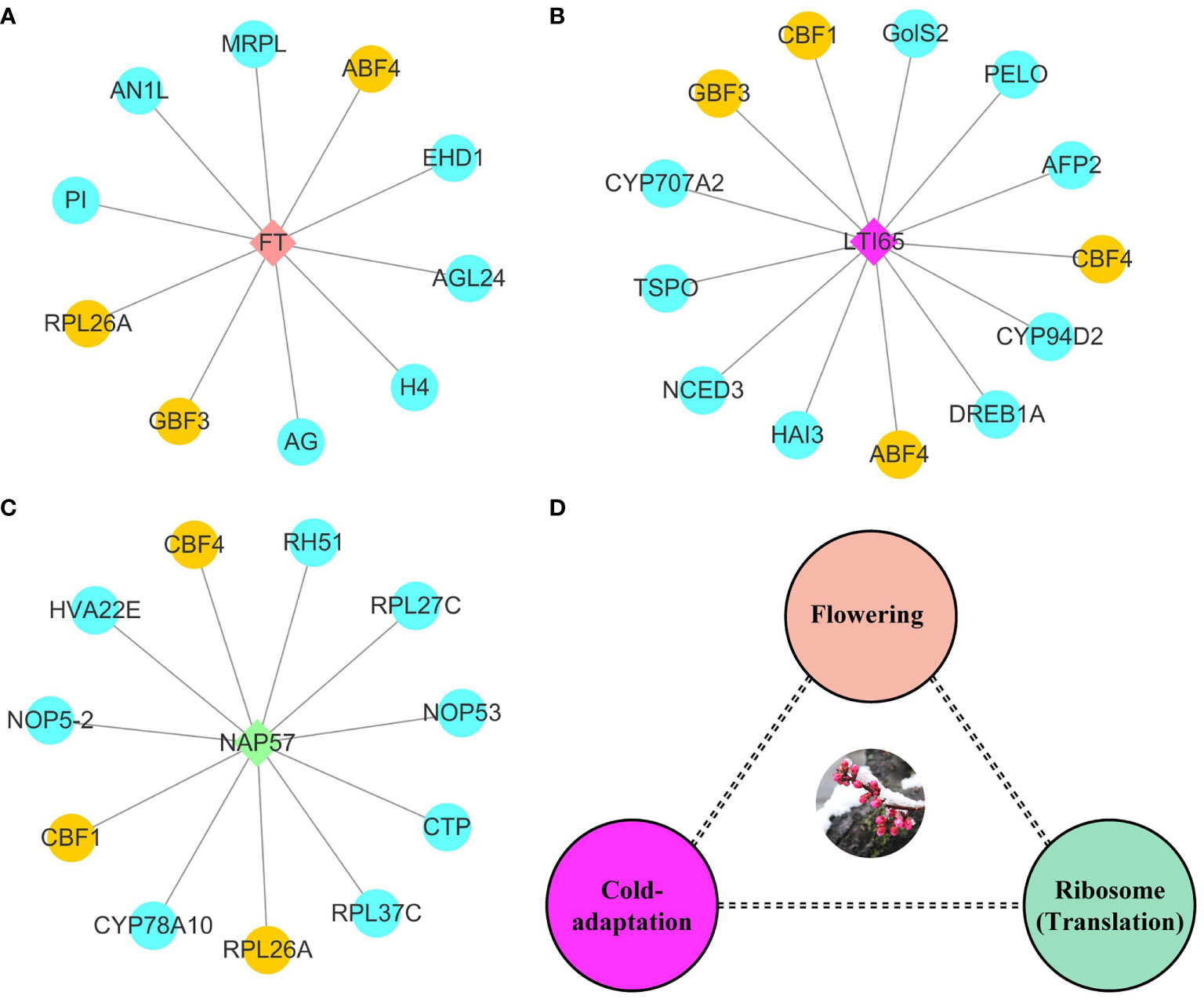
Figure 6 Potential hub regulatory protein interactions and models for early flowering traits. (A) Interaction network with FT as hub protein in early flowering associated proteins. (B) Interaction network with LTI65 as hub protein in early flowering associated proteins. (C) Interaction network with NAP57 as hub protein in early flowering associated proteins. The yellow represents shared proteins in the three interaction networks. The blue represents the unique proteins in each interaction network. (D) Potential models for regulating flowering time.
Discussion
Flowering time is regarded as an environmental adaptive trait of plants, which is crucial for the survival and reproduction (Ding et al., 2020; Gaudinier and Blackman, 2020). The flowering regulation of Prunus is closely related to the heat and chilling requirements, and bud dormancy, including endodormancy and ecodormancy (Sánchez-Pérez et al., 2012; Castède et al., 2014; Zhang et al., 2018; Calle et al., 2019; Yu et al., 2020). The dormancy release is the starting point of flowering process (Bielenberg et al., 2008; Zhang et al., 2018). The dormancy process is also accompanied by the chilling requirement in winter (Lang et al., 1987; Campoy et al., 2012). The plant can bloom under suitable environmental conditions when chilling requirements are met (Campoy et al., 2012; Castède et al., 2014). The flowering time varies by species and cultivars (Dirlewanger et al., 2012). Prunus mume, an early flowering plant, blooms in late winter or early spring before new leaves grow (Zhang et al., 2012). The P. persica genotypes are derived from F2 populations constructed by crossing two P. persica cultivars (male grandparent ‘Fla.92-2C’ and female grandparent ‘Contender’) showing differences in flowering time (Yu et al., 2020).
With all the important advances in genomic sequencing, it is extremely important to understand the biological significance of a variety of sequence and structure data in the post-genome era. So far, the genomes of seventeen Prunus species have been published according to the plaBiPD database (https://www.plabipd.de/). The P. persica and P. mume genomes have been sequenced and assembled in five and three versions, respectively (Zhang et al., 2012; Verde et al., 2013; Guan et al., 2021; Wang et al., 2021b; Zhang et al., 2021a; Zheng et al., 2021; Lian et al., 2022). 10,704 shared orthologues have been obtained across the six Rosaceae species (Malus×domestica, Rosa chinensis, Prunus yedoensis, P. persica, P. armeniaca and P. mume) (Zheng et al., 2021). Species evolution suggests that P. persica and P. mume are closely related and lacks recent whole genome duplication (WGD) events (Verde et al., 2017; Jiang et al., 2019; Zheng et al., 2021). In this study, we identified 19,173 orthogroups and 20,080 collinearity genes between P. mume and P. persica. 20,435 and 25,185 orthologues were retrieved in the P. mume and P. persica genomes, accounting for 76.04% and 80.23% of the total genome, respectively. In addition, the Pan-genome of Prunus was assembled based on the 377 accessions of Prunus germplasm in the Himalayas (Wang et al., 2021b). The molecular mechanism of endodormancy to ecodormancy transition is studied by combining P. persica and P. armeniaca (Yu et al., 2020). These results provide further evidence that P. mume and P. persica are closely related.
In the past 20 years, the research and application of transcriptome technology have gained tremendous importance in the mining of key genes and transcriptional regulation mechanism related to specific traits. The combined genomic and transcriptome strategy provides insight into the molecular mechanism underlying important plant-specific traits, such as the carotenoid metabolism of Lonicera japonica, tanshinones synthesis of Salvia miltiorrhiza, and tortuous-branch phenotype of P. mume (Pu et al., 2020; Ma et al., 2021; Zheng et al., 2021). 59 candidate genes related to flowering time and flower development were screened from the flower development processes by comparing the transcriptomes of the two Solanum lycopersicum genotypes (Wang et al., 2021a). 4,871 and 5,319 DEGs were identified during endodormancy release in P. armeniaca low and high chilling requirement genotypes, respectively (Canton et al., 2021). Meanwhile, 5,912 and 7,048 DEGs were identified during endodormancy release in P. persica low and high chilling requirement genotypes, respectively (Canton et al., 2021). We identified 7,830, 6,401, 6,893, and 9,127 DEGs in the four P. persica genotypes from dormancy to pre-flowering. 1,968 downregulated DEGs and 3,025 upregulated DEGs were identified in the P. mume cultivar ‘Zao Lve’. DEGs represented 20.6% to 33.0% of the total number of genes in the genome from dormancy to pre-flowering. A large number of genes were differentially expressed in the flowering process of Prunus plants, suggesting that the flowering is regulated by a complex mechanism regulated by multiple genes.
Flowering pathways are constantly being developed in plants. The integrators of flowering time play a crucial role in this process (Lloret et al., 2018). At present, FT, LFY, FLC, and SOC1 are thought to be important integrators of floral meristem genes, such as the CAULIFLOWER (CAL), FRUITFUL (FUL), and AP1 (Simpson and Dean, 2002; Posé et al., 2012; Khan et al., 2014; Ó'Maoiléidigh et al., 2014). In Prunus plant, MADS-box TF family members are significantly associated with flowering time and dormancy (Bielenberg et al., 2008; Kai et al., 2018; Zhang et al., 2018; Quesada-Traver et al., 2020). SVP, PI, AG, and AGL24 TFs, belonging to the MADS-box family, directly or indirectly regulate FT gene. Meanwhile, many plant hormone related genes have been identified in the flowering pathway, such as the pyrabactin resistance (PYR) and 9-cis epoxycarotenoid dioxygenase (NCED) genes of the abscisic acid pathway, GA 20-oxidase (GA20ox) and GA 3-oxidase (GA3ox) genes of the gibberellin pathway, and 3-epi-6-deoxocathasterone 23-monooxygenase (CYP) gene of brassinosteroid pathway (Canton et al., 2021; Zhang et al., 2021c). In this study, we identified six MADS-box family members, including AGL4, AGL24, AGL80, AG, PI, and SVP from dormancy to pre-flowering. MADS-box TFs interact with several transcriptional targets to mediate a diverse range of plant processes. Twenty-four percent of all the identified TFs were AP2/ERF homologs, including ERF, RAV, and CBF/DREB subfamilies. Other TFs included MYB, WRKY, bZIP, HSF, ATH, DOF, and ARF TFs. These TF genes have been shown to involve flowering regulation, especially AP2/ERF TFs (Reinoso et al., 2002; Julian et al., 2011; Goeckeritz and Hollender, 2021).
Usually, Prunus plants flower during transition from endodormancy to ecodormancy (Castède et al., 2014; Gaudinier and Blackman, 2020; Canton et al., 2022). Flower buds complete their chilling requirements during endodormancy (Fan et al., 2010; Castède et al., 2014). Although low temperature inhibited the expression of SVP and FLC genes, which were negative regulators of FT, it also inhibited other genes that promoted flowering. The ability of plants to adapt to cold environment was one of the key factors for flowering. The effect of low temperature on plant flowering plays different roles in different stages. Toward the end of winter, low temperature is the main cause of growth arrest during ecodormancy (Lang et al., 1987; Luedeling, 2012; Khan et al., 2014). Moreover, temperature affects the transcriptional ability of genes, which is essential for the morphological transformation of plant tissues during flowering. In this study, the hub proteins, including FT, LTI65 and NAP57 were enriched in CBF-COR pathway, transcription, and flowering. We, therefore, propose a molecular model that covers three aspects of flowering time regulation: cold-adaptation, transcription, and flowering. These results suggest that the regulation of flowering time in plants is influenced by both hereditary substances and environmental conditions.
Data availability statement
The original contributions presented in the study are publicly available. This data can be found here: NCBI BioProject PRJNA714446 and NGDC (https://bigd.big.ac.cn/) BioProject PRJCA000291.
Author contributions
PL and XZ conceived and designed the experiments. JW and HY provided the data collection and analysis platform. PL analyzed the data and wrote the manuscript. LL and BS provided help with the experiments. QZ, LL, BS, HY, JW, and XZ examined and finalized the manuscript. All authors read and approved the final manuscript.
Funding
This research was funded by the Natural Science Foundation of Hebei Province under grant No. C2021204113 and C2021204184.
Conflict of interest
The authors declare that the research was conducted in the absence of any commercial or financial relationships that could be construed as a potential conflict of interest.
Publisher’s note
All claims expressed in this article are solely those of the authors and do not necessarily represent those of their affiliated organizations, or those of the publisher, the editors and the reviewers. Any product that may be evaluated in this article, or claim that may be made by its manufacturer, is not guaranteed or endorsed by the publisher.
Supplementary material
The Supplementary Material for this article can be found online at: https://www.frontiersin.org/articles/10.3389/fpls.2022.1036221/full#supplementary-material
References
Altschul, S. F., Gish, W., Miller, W., Myers, E. W., Lipman, D. J. (1990). Basic local alignment search tool. J. Mol. Biol. 215 (3), 403–410. doi: 10.1016/S0022-2836(05)80360-2
Anders, S., Pyl, P., Huber, W. (2014). HTSeq - a Python framework to work with high-throughput sequencing data. Bioinformatics 31 (2), 166-169. doi: doi: 10.1093/bioinformatics/btu638
Andrés, F., Coupland, G. (2012). The genetic basis of flowering responses to seasonal cues. Nat. Rev. Genet. 13 (9), 627–639. doi: 10.1038/nrg3291
Ashburner, M., Ball, C. A., Blake, J. A., Botstein, D., Butler, H., Cherry, J. M., et al. (2000). Gene ontology: tool for the unification of biology. the gene ontology consortium. Nat. Genet. 25 (1), 25–29. doi: 10.1038/75556
Audic, S., Claverie, J. M. (1997). The significance of digital gene expression profiles. Genome Res. 7 (10), 986–995. doi: 10.1101/gr.7.10.986
Balasubramanian, S., Sureshkumar, S., Lempe, J., Weigel, D. (2006). Potent induction of arabidopsis thaliana flowering by elevated growth temperature. PloS Genet. 2 (7), e106. doi: 10.1371/journal.pgen.0020106
Berardini, T. Z., Reiser, L., Li, D., Mezheritsky, Y., Muller, R., Strait, E., et al. (2015). The arabidopsis information resource: Making and mining the “gold standard” annotated reference plant genome. genesis 53 (8), 474–485. doi: 10.1002/dvg.22877
Bielenberg, D. G., Wang, Y., Li, Z., Zhebentyayeva, T., Fan, S., Reighard, G. L., et al. (2008). Sequencing and annotation of the evergrowing locus in peach [Prunus persica (L.) batsch] reveals a cluster of six MADS-box transcription factors as candidate genes for regulation of terminal bud formation. Tree Genet. Genomes 4 (3), 495–507. doi: 10.1007/s11295-007-0126-9
Blázquez, M. A., Ahn, J. H., Weigel, D. (2003). A thermosensory pathway controlling flowering time in arabidopsis thaliana. Nat. Genet. 33 (2), 168–171. doi: 10.1038/ng1085
Calle, A., Cai, L., Iezzoni, A., Wunsch, A. (2019). Genetic dissection of bloom time in low chilling sweet cherry (Prunus avium l.) using a multi-family QTL approach. Front. Plant Sci. 10 1647. doi: 10.3389/fpls.2019.01647
Campoy, J., Ruiz, D., Allderman, L., Cook, N., Egea, J. (2012). The fulfilment of chilling requirements and the adaptation of apricot (Prunus armeniaca l.) in warm winter climates: An approach in murcia (Spain) and the Western cape (South Africa). Eur. J. Agron. 37, 43–55. doi: 10.1016/j.eja.2011.10.004
Canton, M., Forestan, C., Bonghi, C., Varotto, S. (2021). Meta-analysis of RNA-seq studies reveals genes with dominant functions during flower bud endo- to eco-dormancy transition in prunus species. Sci. Rep. 11 (1), 13173. doi: 10.1038/s41598-021-92600-6
Canton, M., Forestan, C., Marconi, G., Carrera, E., Bonghi, C., Varotto, S. (2022). Evidence of chromatin and transcriptional dynamics for cold development in peach flower bud. New Phytol. doi: 10.1111/nph.18393
Castède, S., Campoy, J. A., García, J. Q., Le Dantec, L., Lafargue, M., Barreneche, T., et al. (2014). Genetic determinism of phenological traits highly affected by climate change in prunus avium: flowering date dissected into chilling and heat requirements. New Phytol. 202 (2), 703–715. doi: 10.1111/nph.12658
Chen, C., Chen, H., Zhang, Y., Thomas, H. R., Frank, M. H., He, Y., et al. (2020). TBtools: An integrative toolkit developed for interactive analyses of big biological data. Mol. Plant 13 (8), 1194–1202. doi: 10.1016/j.molp.2020.06.009
Chen, T., Zhang, H., Liu, Y., Liu, Y.-X., Huang, L. (2021). EVenn: Easy to create repeatable and editable Venn diagrams and Venn networks online. J. Genet. Genomics 48 (9), 863–866. doi: 10.1016/j.jgg.2021.07.007
Ding, Y., Shi, Y., Yang, S. (2020). Molecular regulation of plant responses to environmental temperatures. Mol. Plant 13 (4), 544–564. doi: 10.1016/j.molp.2020.02.004
Dirlewanger, E., Quero-García, J., Le Dantec, L., Lambert, P., Ruiz, D., Dondini, L., et al. (2012). Comparison of the genetic determinism of two key phenological traits, flowering and maturity dates, in three prunus species: peach, apricot and sweet cherry. Heredity 109 (5), 280–292. doi: 10.1038/hdy.2012.38
Emms, D. M., Kelly, S. (2015). OrthoFinder: solving fundamental biases in whole genome comparisons dramatically improves orthogroup inference accuracy. Genome Biol. 16 (1), 157. doi: 10.1186/s13059-015-0721-2
Fan, S., Bielenberg, D. G., Zhebentyayeva, T. N., Reighard, G. L., Okie, W. R., Holland, D., et al. (2010). Mapping quantitative trait loci associated with chilling requirement, heat requirement and bloom date in peach (Prunus persica). New Phytol. 185 (4), 917–930. doi: 10.1111/j.1469-8137.2009.03119.x
Freytes, S. N., Canelo, M., Cerdán, P. D. (2021). Regulation of flowering time: When and where? Curr. Opin. Plant Biol. 63, 102049. doi: 10.1016/j.pbi.2021.102049
Fuente, L., Conesa, A., Lloret, A., Badenes, M., Rios, G. (2015). Genome-wide changes in histone H3 lysine 27 trimethylation associated with bud dormancy release in peach. Tree Genet. Genomes 11, 45. doi: 10.1007/s11295-015-0869-7
Gaudinier, A., Blackman, B. K. (2020). Evolutionary processes from the perspective of flowering time diversity. New Phytol. 225 (5), 1883–1898. doi: 10.1111/nph.16205
Goeckeritz, C., Hollender, C. (2021). There is more to flowering than those DAM genes: the biology behind bloom in rosaceous fruit trees. Curr. Opin. Plant Biol. 59, 101995. doi: 10.1016/j.pbi.2020.101995
Guan, J., Xu, Y., Yu, Y., Fu, J., Ren, F., Guo, J., et al. (2021). Genome structure variation analyses of peach reveal population dynamics and a 1.67 Mb causal inversion for fruit shape. Genome Biol. 22 (1), 13. doi: 10.1186/s13059-020-02239-1
Halliday, K. J., Salter, M. G., Thingnaes, E., Whitelam, G. C. (2003). Phytochrome control of flowering is temperature sensitive and correlates with expression of the floral integrator FT. Plant J. 33 (5), 875–885. doi: 10.1046/j.1365-313X.2003.01674.x
Jiang, F., Zhang, J., Wang, S., Yang, L., Luo, Y., Gao, S., et al. (2019). The apricot (Prunus armeniaca l.) genome elucidates rosaceae evolution and beta-carotenoid synthesis. Horticult Res. 6, 128. doi: 10.1038/s41438-019-0215-6
Jiménez, S., Lawton-Rauh, A. L., Reighard, G. L., Abbott, A. G., Bielenberg, D. G. (2009). Phylogenetic analysis and molecular evolution of the dormancy associated MADS-box genes from peach. BMC Plant Biol. 9 (1), 81. doi: 10.1186/1471-2229-9-81
Jin, JP, Zhang, H., Kong, L., Gao, G., Luo, J.. (2014). PlantTFDB 3.0: a portal for the functional and evolutionary study of plant transcription factors. Nucleic Acids Res 42, D1182–D1187. doi: 10.1093/nar/gkt1016
Johansson, M., Staiger, D. (2015). Time to flower: interplay between photoperiod and the circadian clock. J. Exp. Bot. 66 (3), 719–730. doi: 10.1093/jxb/eru441
Julian, C., Rodrigo, J., Herrero, M. (2011). Stamen development and winter dormancy in apricot (Prunus armeniaca). Ann. Bot. 108 (4), 617–625. doi: 10.1093/aob/mcr056
Jung, S., Lee, T., Cheng, C.-H., Buble, K., Zheng, P., Yu, J., et al. (2018). 15 years of GDR: New data and functionality in the genome database for rosaceae. Nucleic Acids Res. 47 (D1), D1137–D1145. doi: 10.1093/nar/gky1000
Kai, Z., Zhou, Y., Ahmad, S., Xu, Z., Li, Y., Yang, W., et al. (2018). Comprehensive cloning of prunus mume dormancy associated MADS-box genes and their response in flower bud development and dormancy. Front. Plant Sci. 9. doi: 10.3389/fpls.2018.00017
Kanehisa, M., Goto, S. (2000). KEGG: kyoto encyclopedia of genes and genomes. Nucleic Acids Res. 28 (1), 27–30. doi: 10.1093/nar/28.1.27
Katoh, K., Standley, D. M. (2013). MAFFT multiple sequence alignment software version 7: Improvements in performance and usability. Mol. Biol. Evol. 30 (4), 772–780. doi: 10.1093/molbev/mst010
Khan, M. R. G., Ai, X.-Y., Zhang, J.-Z. (2014). Genetic regulation of flowering time in annual and perennial plants. WIREs RNA 5 (3), 347–359. doi: 10.1002/wrna.1215
Kole, C. (2011). Wild crop relatives: Genomic and breeding resources: Temperate fruits (Berlin:Springer). doi: 10.1007/978-3-642-14255-0
Kumar, S., Stecher, G., Suleski, M., Hedges, S. B. (2017). TimeTree: A resource for timelines, timetrees, and divergence times. Mol. Biol. Evol. 34 (7), 1812–1819. doi: 10.1093/molbev/msx116
Lang, G. A., Early, J. D., Darnell, R., Martin, G. C. (1987). Endo-, para-, and eco-dormancy: Physiological terminology and classification for dormancy research. Hortscience 22, 371–377. doi: 10.21273/HORTSCI.22.3.371
Leida, C., Conesa, A., Llácer, G., Badenes, M. L., Ríos, G. (2012). Histone modifications and expression of DAM6 gene in peach are modulated during bud dormancy release in a cultivar-dependent manner. New Phytol. 193 (1), 67–80. doi: 10.1111/j.1469-8137.2011.03863.x
Lian, X., Zhang, H., Jiang, C., Gao, F., Yan, L., Zheng, X., et al. (2022). De novo chromosome-level genome of a semi-dwarf cultivar of prunus persica identifies the aquaporin PpTIP2 as responsible for temperature-sensitive semi-dwarf trait and PpB3-1 for flower type and size. Plant Biotechnol. J. 20 (5), 886–902. doi: 10.1111/pbi.13767
Lin, X., Liu, B., Weller, J. L., Abe, J., Kong, F. (2021). Molecular mechanisms for the photoperiodic regulation of flowering in soybean. J. Integr. Plant Biol. 63 (6), 981–994. doi: 10.1111/jipb.13021
Li, P., Tangchun, Z., Zhang, Z., Liu, W., Qiu, L., Wang, J., et al. (2021). Integrative identification of crucial genes associated with plant hormone-mediated bud dormancy in prunus mume. Front. Genet. 12. doi: 10.3389/fgene.2021.698598
Lloret, A., Badenes, M. L., Rios, G. (2018). Modulation of dormancy and growth responses in reproductive buds of temperate trees. Front. Plant Sci. 9 1368. doi: 10.3389/fpls.2018.01368
Luedeling, E. (2012). Climate change impacts on winter chill for temperate fruit and nut production: A review. Scientia Hortic. 144, 218–229. doi: 10.1016/j.scienta.2012.07.011
Ma, Y., Cui, G., Chen, T., Ma, X., Wang, R., Jin, B., et al. (2021). Expansion within the CYP71D subfamily drives the heterocyclization of tanshinones synthesis in salvia miltiorrhiza. Nat. Commun. 12 (1), 685. doi: 10.1038/s41467-021-20959-1
Maurya, J. P., Miskolczi, P. C., Mishra, S., Singh, R. K., Bhalerao, R. P. (2020). A genetic framework for regulation and seasonal adaptation of shoot architecture in hybrid aspen. Proc. Natl. Acad. Sci. 117 (21), 11523–11530. doi: 10.1073/pnas.2004705117
Mir, S., Alhroub, Y., Anyango, S., Armstrong, D. R., Berrisford, J. M., Clark, A. R., et al. (2018). PDBe: towards reusable data delivery infrastructure at protein data bank in Europe. Nucleic Acids Res. 46 (D1), D486–D492. doi: 10.1093/nar/gkx1070
Mistry, J., Chuguransky, S., Williams, L., Qureshi, M., Salazar, G. A., Sonnhammer, E. L. L., et al. (2021). Pfam: The protein families database in 2021. Nucleic Acids Res. 49 (D1), D412–D419. doi: 10.1093/nar/gkaa913
Ó'Maoiléidigh, D. S., Graciet, E., Wellmer, F. (2014). Gene networks controlling arabidopsis thaliana flower development. New Phytol. 201 (1), 16–30. doi: 10.1111/nph.12444
Otasek, D., Morris, J. H., Bouças, J., Pico, A. R., Demchak, B. (2019). Cytoscape automation: empowering workflow-based network analysis. Genome Biol. 20 (1), 185–185. doi: 10.1186/s13059-019-1758-4
Pertea, M., Pertea, G. M., Antonescu, C. M., Chang, T.-C., Mendell, J. T., Salzberg, S. L. (2015). StringTie enables improved reconstruction of a transcriptome from RNA-seq reads. Nat. Biotechnol. 33 (3), 290–295. doi: 10.1038/nbt.3122
Posé, D., Yant, L., Schmid, M. (2012). The end of innocence: flowering networks explode in complexity. Curr. Opin. Plant Biol. 15 (1), 45–50. doi: 10.1016/j.pbi.2011.09.002
Price, M. N., Dehal, P. S., Arkin, A. P. (2009). FastTree: Computing Large minimum evolution trees with profiles instead of a distance matrix. Mol. Biol. Evol. 26 (7), 1641–1650. doi: 10.1093/molbev/msp077
Pu, X., Li, Z., Tian, Y., Gao, R., Hao, L., Hu, Y., et al. (2020). The honeysuckle genome provides insight into the molecular mechanism of carotenoid metabolism underlying dynamic flower coloration. New Phytol. 227(3), 930-943. doi: 10.1111/nph.16552
Quesada-Traver, C., Guerrero, B., Badenes, M., Rodrigo, J., Rios, G., Lloret, A. (2020). Structure and expression of bud dormancy-associated MADS-box genes (DAM) in European plum. Front. Plant Sci. 11 1288. doi: 10.3389/fpls.2020.01288
Quevillon, E., Silventoinen, V., Pillai, S., Harte, N., Mulder, N., Apweiler, R., et al. (2005). InterProScan: protein domains identifier. Nucleic Acids Res. 33 (suppl_2), W116–W120. doi: 10.1093/nar/gki442
Quiroz, S., Yustis, J. C., Chávez-Hernández, E. C., Martínez, T., Sanchez, M. D., Garay-Arroyo, A., et al. (2021). Beyond the genetic pathways, flowering regulation complexity in arabidopsis thaliana. Int. J. Mol. Sci. 22 (11). doi: 10.3390/ijms22115716
Reinoso, H., Luna, V., Pharis, R. P., Bottini, R. (2002). Dormancy in peach (Prunus persica) flower buds. v. anatomy of bud development in relation to phenological stage. Can. J. Bot. 80 (6), 656–663.
Rothkegel, K., Sandoval, P., Soto, E., Ulloa, L., Riveros, A., Lillo-Carmona, V., et al. (2020). Dormant but active: Chilling accumulation modulates the epigenome and transcriptome of prunus avium during bud dormancy. Front. Plant Sci. 11. doi: 10.3389/fpls.2020.01115
Sánchez-Pérez, R., Del Cueto, J., Dicenta, F., Martínez-Gómez, P. (2014). Recent advancements to study flowering time in almond and other prunus species. Front. Plant Sci. 5. doi: 10.3389/fpls.2014.00334
Sánchez-Pérez, R., Dicenta, F., Martínez-Gómez, P. (2012). Inheritance of chilling and heat requirements for flowering in almond and QTL analysis. Tree Genet. Genomes 8 (2), 379–389. doi: 10.1007/s11295-011-0448-5
Schwarz, S., Grande, A. V., Bujdoso, N., Saedler, H., Huijser, P. (2008). The microRNA regulated SBP-box genes SPL9 and SPL15 control shoot maturation in arabidopsis. Plant Mol. Biol. 67 (1), 183–195. doi: 10.1007/s11103-008-9310-z
Sharma, N., Geuten, K., Giri, B. S., Varma, A. (2020). The molecular mechanism of vernalization in arabidopsis and cereals: role of flowering locus c and its homologs. Physiol Plantarum 170 (3), 373–383. doi: 10.1111/ppl.13163
Shi, T., Luo, W., Li, H., Huang, X., Ni, Z., Gao, H., et al. (2020). Association between blooming time and climatic adaptation in prunus mume. Ecol. Evol. 10 (1), 292–306. doi: 10.1002/ece3.5894
Shim, J. S., Jang, G. (2020). Environmental signal-dependent regulation of flowering time in rice. Int. J. Mol. Sci. 21 (17), 6155. doi: 10.3390/ijms21176155
Simpson, G. G., Dean, C. (2002). Arabidopsis, the Rosetta stone of flowering time? Science 296 (5566), 285–289. doi: 10.1126/science.296.5566.285
Theodosiou, T., Darzentas, N., Angelis, L., Ouzounis, C. A. (2008). PuReD-MCL: a graph-based PubMed document clustering methodology. Bioinformatics 24 (17), 1935–1941. doi: 10.1093/bioinformatics/btn318
The UniProt, C. (2021). UniProt: the universal protein knowledgebase in 2021. Nucleic Acids Res. 49 (D1), D480–D489. doi: 10.1093/nar/gkaa1100
Verde, I., Abbott, A. G., Scalabrin, S., Jung, S., Shu, S., Marroni, F., et al. (2013). The high-quality draft genome of peach (Prunus persica) identifies unique patterns of genetic diversity, domestication and genome evolution. Nat. Genet. 45 (5), 487–494. doi: 10.1038/ng.2586
Verde, I., Jenkins, J., Dondini, L., Micali, S., Pagliarani, G., Vendramin, E., et al. (2017). The peach v2.0 release: high-resolution linkage mapping and deep resequencing improve chromosome-scale assembly and contiguity. BMC Genomics 18 (1), 225. doi: 10.1186/s12864-017-3606-9
Wahl, V., Ponnu, J., Schlereth, A., Arrivault, S., Langenecker, T., Franke, A., et al. (2013). Regulation of flowering by trehalose-6-Phosphate signaling in arabidopsis thaliana. Science 339 (6120), 704–707. doi: 10.1126/science.1230406
Wang, X., Liu, S., Zuo, H., Zheng, W., Zhang, S., Yue, H., et al. (2021b). Genomic basis of high-altitude adaptation in Tibetan prunus fruit trees. Curr. Biol. 31 (17), 3848–3860.e8. doi: 10.1016/j.cub.2021.06.062
Wang, Y., Tang, H., Debarry, J. D., Tan, X., Li, J., Wang, X., et al. (2012). MCScanX: a toolkit for detection and evolutionary analysis of gene synteny and collinearity. Nucleic Acids Res. 40 (7), e49–e49. doi: 10.1093/nar/gkr1293
Wang, H., Yang, Y., Zhang, Y., Zhao, T., Jiang, J., Li, J., et al. (2021a). Transcriptome analysis of flower development and mining of genes related to flowering time in tomato (Solanum lycopersicum). Int. J. Mol. Sci. 22 (15), 8128. doi: 10.3390/ijms22158128
Wan, J., Jiu, S., Xu, Y., Sabir, I., Wang, L., Ma, C., et al. (2020). SVP-like gene PavSVP potentially suppressing flowering with PavSEP, PavAP1, and PavJONITLESS in sweet cherries (Prunus avium l.). Plant Physiol. Biochem. 159, 277–284. doi: 10.1016/j.plaphy.2020.12.013
Weller, J. L., Macknight, R. C. (2018). “"Functional genomics and flowering time in medicago truncatula: An overview,",” in In functional genomics in medicago truncatula: Methods and protocols. Eds. Cañas, L. A., Beltrán, J. P. (New York, NY: Springer New York), 261–271.
Wu, Z., Fang, X., Zhu, D., Dean, C. (2020). Autonomous pathway: FLOWERING LOCUS c repression through an antisense-mediated chromatin-silencing Mechanism1 [CC-BY]. Plant Physiol. 182 (1), 27–37. doi: 10.1104/pp.19.01009
Wu, G., Poethig, R. S. (2006). Temporal regulation of shoot development in arabidopsis thalianaby miR156 and its target SPL3. Development 133 (18), 3539–3547. doi: 10.1242/dev.02521
Xu, S., Chong, K. (2018). Remembering winter through vernalisation. Nat. Plants 4 (12), 997–1009. doi: 10.1038/s41477-018-0301-z
Yu, J., Conrad, A., Decroocq, V., Zhebentyayeva, T., Williams, D., Bennett, D., et al. (2020). Distinctive gene expression patterns define endodormancy to ecodormancy transition in apricot and peach. Front. Plant Sci. 11. doi: 10.3389/fpls.2020.00180
Zhang, Q., Chen, W., Sun, L., Zhao, F., Huang, B., Yang, W., et al. (2012). The genome of prunus mume. Nat. Commun. 3, 1318. doi: 10.1038/ncomms2290
Zhang, M., Li, P., Yan, X., Wang, J., Cheng, T., Zhang, Q. (2021b). Genome-wide characterization of PEBP family genes in nine rosaceae tree species and their expression analysis in p. mume. BMC Ecol. Evol. 21 (1), 32. doi: 10.1186/s12862-021-01762-4
Zhang, M., Yang, Q., Yuan, X., Yan, X., Wang, J., Cheng, T., et al. (2021c). Integrating genome-wide association analysis with transcriptome sequencing to identify candidate genes related to blooming time in prunus mume. Front. Plant Sci. 12. doi: 10.3389/fpls.2021.690841
Zhang, A., Zhou, H., Jiang, X., Han, Y., Zhang, X. (2021a). The draft genome of a flat peach (Prunus persica l. cv. ‘124 pan’) provides insights into its good fruit flavor traits. Plants 10 (3), 538. doi: 10.3390/plants10030538
Zhang, Z., Zhuo, X., Zhao, K., Zheng, T., Han, Y., Yuan, C., et al. (2018). Transcriptome profiles reveal the crucial roles of hormone and sugar in the bud dormancy of prunus mume. Sci. Rep. 8 (1), 5090. doi: 10.1038/s41598-018-23108-9
Zhebentyayeva, T. N., Fan, S., Chandra, A., Bielenberg, D. G., Reighard, G. L., Okie, W. R., et al. (2013). Dissection of chilling requirement and bloom date QTLs in peach using a whole genome sequencing of sibling trees from an F2 mapping population. Tree Genet. Genomes 10 (1), 35–51. doi: 10.1007/s11295-013-0660-6
Zheng, T., Li, P., Zhuo, X., Liu, W., Qiu, L., Li, L., et al. (2021). The chromosome-level genome provides insight into the molecular mechanism underlying the tortuous-branch phenotype of prunus mume. New Phytol. 235 (1), 141–156. doi: 10.1111/nph.17894
Keywords: Prunus genus, early flowering, gene mining, multi-omics, molecular model
Citation: Li P, Zhang Q, Shi B, Liu L, Zhang X, Wang J and Yi H (2022) Integration of genome and transcriptome reveal molecular regulation mechanism of early flowering trait in Prunus genus (Prunus mume and Prunus persica). Front. Plant Sci. 13:1036221. doi: 10.3389/fpls.2022.1036221
Received: 04 September 2022; Accepted: 20 September 2022;
Published: 06 October 2022.
Edited by:
Penghui Li, Anhui Agricultural University, ChinaReviewed by:
Yongming Fan, North China University of Water Conservancy and Electric Power, ChinaBaijun Li, Zhejiang University, China
Copyright © 2022 Li, Zhang, Shi, Liu, Zhang, Wang and Yi. This is an open-access article distributed under the terms of the Creative Commons Attribution License (CC BY). The use, distribution or reproduction in other forums is permitted, provided the original author(s) and the copyright owner(s) are credited and that the original publication in this journal is cited, in accordance with accepted academic practice. No use, distribution or reproduction is permitted which does not comply with these terms.
*Correspondence: Xiaoman Zhang, zhangxiaoman1977@163.com; Jia Wang, wangjia8248@163.com; Haihui Yi, yihaihui2004@163.com