- 1Department of Clinical Pharmacy, Key Laboratory of Chemical Biology (Ministry of Education), School of Pharmaceutical Sciences, Cheeloo College of Medicine, Shandong University, Jinan, China
- 2NMPA Key Laboratory for Clinical Research and Evaluation of Innovative Drug, Qilu Hospital of Shandong University, Shandong University, Jinan, China
- 3Division of Clinical Pharmacology, Children’s National Hospital, Washington, DC, United States
- 4Departments of Pediatrics, Pharmacology & Physiology, Genomics & Precision Medicine, The George Washington University School of Medicine and Health Sciences, Washington, DC, United States
- 5Department of Pediatric Pharmacology and Pharmacometrics, University of Basel Children’s Hospital, Basel, Switzerland
Editorial on the Research Topic
Model-based evaluation of antimicrobial agents in children—volume II
Introduction
Off-label drug use is very common in neonatal and pediatric patients (Tang B H et al., 2023). The pediatric population shows major changes in the way they can handle drugs due to their continuous growth and development. It is therefore very challenging to determine the most effective and safe way to use drugs in neonates, infants, and children. To improve this situation increasing numbers of scholars are working tirelessly to achieve model-based pediatric drug development as well as individualized treatment.
In “Model-based evaluation of antimicrobial agents in children—volume II,” the articles focus on studies of model-based drug development of antimicrobial agents in the pediatric population as well as model-based individualized antimicrobial therapy in neonates, infants, children, and adolescents.
Model-based antimicrobial therapy in severe infectious disease
Model-based evaluation of antimicrobial agents has been achieved in severe infectious diseases including pneumonia, critically illnesses and central nervous system infections in children. For antibiotic therapy, most studies are still happening at the level of pharmacokinetics (PK) and pharmacodynamics (PD). The clinical use of individualized therapy and the promotion of precision dosing software has, until now, only be applied in a few antibiotics. Girdwood et al. emphasized the dilemma between endorsing and implementing precision dosing of β-lactam antibiotics in critically ill children without knowing which subgroups would really benefit from precision dosing.
In applying knowledge of model-based PK of antibiotics to clinical practice, some scholars are experimenting by conducting randomized controlled trials. Based on a previously published population pharmacokinetic (PopPK) model, Tian et al. designed a randomized controlled trial of meropenem in pediatric patients with severe pneumonia with the aim of comparing the efficacy and safety of the conventional and model-based dosing regimens of meropenem. Compared to the conventional treatment group (20 mg/kg, q8 h, infusion for 0.5–1 h), the model treatment group was optimized using an extended infusion time of 4 h. From a pharmacokinetic perspective, the extended infusion time contributed to the 70% fT > MIC (the free plasma concentration of meropenem exceeds the minimum inhibitory concentration during 70% of the dosing interval) target, and in terms of clinical effectiveness, this model-based dosing regimen should be evaluated.
However, for some diseases, the first step to achieve model-based dose optimization is still to supplement the basic data for efficacy, safety, and pharmacokinetics. Liu et al. reviewed the efficacy, safety, and pharmacokinetics of intravenous and intraventricular vancomycin for central nervous system infection including meningitis, ventriculitis, and central nervous system (CNS) device-associated infections. They found significant differences in dosages, duration, and concentrations of vancomycin in the cerebrospinal fluid for intravenous and intraventricular vancomycin administration, respectively. The optimal dose for the treatment of CNS remains unclear and needs to be achieved by high-level clinical trials assisted by modeling techniques.
Bayesian techniques play a crucial role in model-based antimicrobial therapy. Bunn et al. described the challenges associated with designing and implementing dose optimization strategies and provided evidence that Bayesian-model informed precision dosing can address these challenges. They also described the practice of optimizing vancomycin dose using a software Lyv (Pumas-AI, Inc., Centreville, VA).
Model-based evaluation of antimicrobial agents in children: state of the art and future
The PopPK model is a traditional method for achieving dose optimization and has largely improved the phenomenon of off-label drug use in pediatric populations. Despite its widespread use, there are significant problems with this approach: 1) Bayesian estimation based on the PopPK model is not sufficiently accurate and cumbersome; 2) the software often relied upon, such as NONMEM, is limited by the software itself and does not allow for easy application; and 3) traditional models incorporate limited variables and cannot handle complex clinical scenarios. Machine learning is a data-driven technique that can make accurate judgements and predictions about real-world events. It is now widely used in aspects of disease diagnosis (Esteva et al., 2017; Ding et al., 2019), creating many new clinical possibilities and showing great potential in the field of individualized treatment of pediatric patients with antimicrobial agents. Machine learning can handle more complex variable factors and enable initial dose optimization and subsequent dose adjustment, as well as making it easier to convert formulation information into application software or web-based applications for individualized treatment in clinical practice (Figure 1).
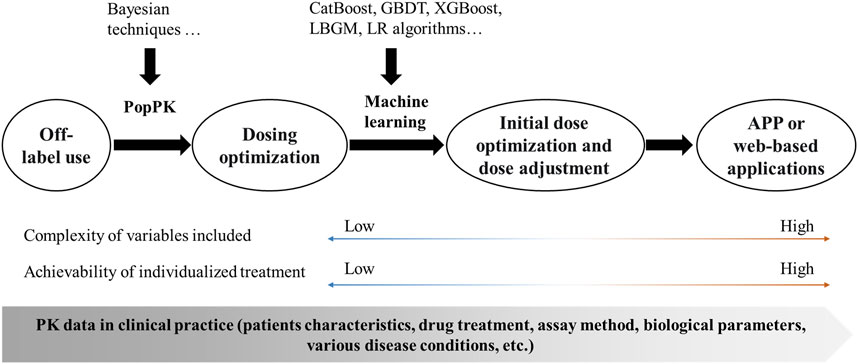
FIGURE 1. Framework for the implementation of individualized treatment. PopPK, population pharmacokinetics; CatBoost, Categorical boosting; GBDT, Gradient boosting decision tree; XGBoost, Extreme gradient boosting; LBGM, Light gradient boosting machine; LR, Logistic Regression; APP, application; PK, pharmacokinetics.
By studying six drugs that are primarily eliminated by the kidney (vancomycin, latamoxef, cefepime, azlocillin, ceftazidime, and amoxicillin) as “proof-of-concept” compounds, Tang et al. validated that a combination of PopPK and machine learning approaches could more accurately predict the clearance of drugs for renal elimination in neonates (Tang et al., 2021). In case of vancomycin, steady-state trough concentration (C0) and area under the steady-state curve (AUC0-24) targets are often used for dose optimization of vancomycin. C0-based and AUC0-24-based machine learning models of vancomycin have been successfully developed and can achieve individualized initial dose prediction and further optimization based on therapeutic drug monitoring results in neonates by accurately predicting C0 and AUC0-24 (Tang L et al., 2023).
To summarize, “Model-based evaluation of antimicrobial agents in children—volume II” provides current knowledge and future research ideas for model-based drug development and individualized treatment of antimicrobial drugs, in the hope of opening new horizons for the rational use of antimicrobial drugs in children.
Author contributions
WZ: Funding acquisition, Writing–original draft, Writing–review and editing. JV: Supervision, Writing–review and editing.
Funding
This work was supported by the National Key R&D Program of China (2020YFC2008303, 2020YFC2008306), National Natural Science Foundation of China (82173897), Taishan Scholar Program of Shandong Province (No. tstp20230660), Distinguished Young and Middle-aged Scholar of Shandong University.
Conflict of interest
The authors declare that the research was conducted in the absence of any commercial or financial relationships that could be construed as a potential conflict of interest.
The author(s) declared that they were an editorial board member of Frontiers, at the time of submission. This had no impact on the peer review process and the final decision.
Publisher’s note
All claims expressed in this article are solely those of the authors and do not necessarily represent those of their affiliated organizations, or those of the publisher, the editors and the reviewers. Any product that may be evaluated in this article, or claim that may be made by its manufacturer, is not guaranteed or endorsed by the publisher.
References
Ding, Y., Sohn, J. H., Kawczynski, M. G., Trivedi, H., Harnish, R., Jenkins, N. W., et al. (2019). A deep learning model to predict a diagnosis of alzheimer disease by using (18)F-fdg PET of the brain. Radiology 290 (2), 456–464. doi:10.1148/radiol.2018180958
Esteva, A., Kuprel, B., Novoa, R. A., Ko, J., Swetter, S. M., Blau, H. M., et al. (2017). Dermatologist-level classification of skin cancer with deep neural networks. Nature 542 (7639), 115–118. doi:10.1038/nature21056
Tang, B. H., Guan, Z., Allegaert, K., Wu, Y. E., Manolis, E., Leroux, S., et al. (2021). Drug clearance in neonates: a combination of population pharmacokinetic modelling and machine learning approaches to improve individual prediction. Clin. Pharmacokinet. 60 (11), 1435–1448. doi:10.1007/s40262-021-01033-x
Tang, B. H., Zhang, J. Y., Allegaert, K., Hao, G. X., Yao, B. F., Leroux, S., et al. (2023). Use of machine learning for dosage individualization of vancomycin in neonates. Clin. Pharmacokinet. 62, 1105–1116. doi:10.1007/s40262-023-01265-z
Keywords: model-based drug development, model-based individualized antimicrobial therapy, children, antimicrobial agents, machine learning
Citation: Zhao W and Van Den Anker J (2023) Editorial: Model-based evaluation of antimicrobial agents in children—volume II. Front. Pharmacol. 14:1272996. doi: 10.3389/fphar.2023.1272996
Received: 05 August 2023; Accepted: 09 August 2023;
Published: 14 August 2023.
Edited and reviewed by:
Catherine M. T. Sherwin, Wright State University, United StatesCopyright © 2023 Zhao and Van Den Anker. This is an open-access article distributed under the terms of the Creative Commons Attribution License (CC BY). The use, distribution or reproduction in other forums is permitted, provided the original author(s) and the copyright owner(s) are credited and that the original publication in this journal is cited, in accordance with accepted academic practice. No use, distribution or reproduction is permitted which does not comply with these terms.
*Correspondence: John Van Den Anker, jvandena@childrensnational.org