- 1Division of Medical Oncology and Hematology, Princess Margaret Cancer Centre, University Health Network, University of Toronto, Toronto, ON, Canada
- 2Division of Infectious Diseases, Toronto General Hospital, University Health Network, Toronto, ON, Canada
Molecularly targeted treatments and immunotherapy are cornerstones in oncology, with demonstrated efficacy across different tumor types. Nevertheless, the overwhelming majority metastatic disease is incurable due to the onset of drug resistance. Preclinical models including genetically engineered mouse models, patient-derived xenografts and two- and three-dimensional cell cultures have emerged as a useful resource to study mechanisms of cancer progression and predict efficacy of anticancer drugs. However, variables including tumor heterogeneity and the complexities of the microenvironment can impair the faithfulness of these platforms. Here, we will discuss advantages and limitations of these preclinical models, their applicability for drug testing and in co-clinical trials and potential strategies to increase their reliability in predicting responsiveness to anticancer medications.
1 Introduction
Cancer is a genetic disease that results in cumulative alterations of molecular pathways involved in cell growth, survival and proliferation (1, 2). Until a few decades ago, chemotherapy and endocrine therapy represented the only treatment options for patients with advanced malignancies, and tumor histology was the only benchmark for drug selection (1). The identification of disrupted molecular pathways has notably broadened the therapeutic opportunities for cancer patients, allowing the development of small molecules and monoclonal antibodies exploiting oncogenic driver alterations as drug targets (3). The favorable therapeutic index demonstrated by several of these agents enabled their integration into clinical practice. A large number of such agents are now in clinical use, including epidermal growth factor receptor (EGFR) inhibitors in EGFR mutated non-small cell lung cancer (NSCLC) (4), anti-epidermal growth factor receptor 2 (HER2) agents in HER2-positive breast (5) and gastric cancer (6), B-rapidly accelerated fibro sarcoma (BRAF) inhibitors for the treatment of melanoma and other BRAF mutated tumors (7). The advent of immunotherapy has ushered in therapeutic strategies that promote immune response against neoplastic cells (8). Many different types of immune-therapeutics have now entered the clinic and some of them, such as immune checkpoint inhibitors (ICIs) and chimeric antigen receptor (CAR)-T cells, have improved patient outcomes (9, 10). In certain settings, including Hodgkin’s lymphoma, melanoma, NSCLC, head and neck, urothelial and renal cell carcinoma, ICIs have replaced previous standard therapies due to overall survival (OS) benefits. Despite the achieved improvement in patient outcomes with the introduction of targeted drugs and immunotherapy, the majority of subjects do not respond to these treatments or experience only a temporary benefit (11, 12). Primary (or intrinsic) and secondary (or acquired) resistance, led by resistance-driving factors in neoplastic tissue before the exposure to an anticancer agent or as a consequence of the antitumor treatment respectively, represent the main reasons for treatment failure with these agents (12). To distinguish between primary and acquired resistance is not always straightforward. Different subpopulations of cancer cells, characterized by specific genomic profiles, usually coexist in the same patient. The phenomenon of intra-patient tumor heterogeneity can be spatial (e.g. in different locations) or temporal (e.g. between primary tumor versus metastasis) (13). Tumor heterogeneity adds complexity to the identification of pre-existing or exposure-induced resistant clones. Multiple mechanisms can be responsible for primary and acquired resistance to specific compounds in different tumor types and their identification is a crucial step in the identification of effective, individualized treatments (14) (Figure 1). Patient-derived human cancer models have the potential to retain their distinctive molecular hallmarks, representing a unique opportunity to study cancer cell survival and resistance mechanisms (15–17). If combined with clinical studies, these tools might increase the success of experimental treatments (18, 19). This review will discuss the available preclinical models and the reliability of such platforms to predict the responsiveness to anticancer agents, focusing on patient-derived models (Figure 2).
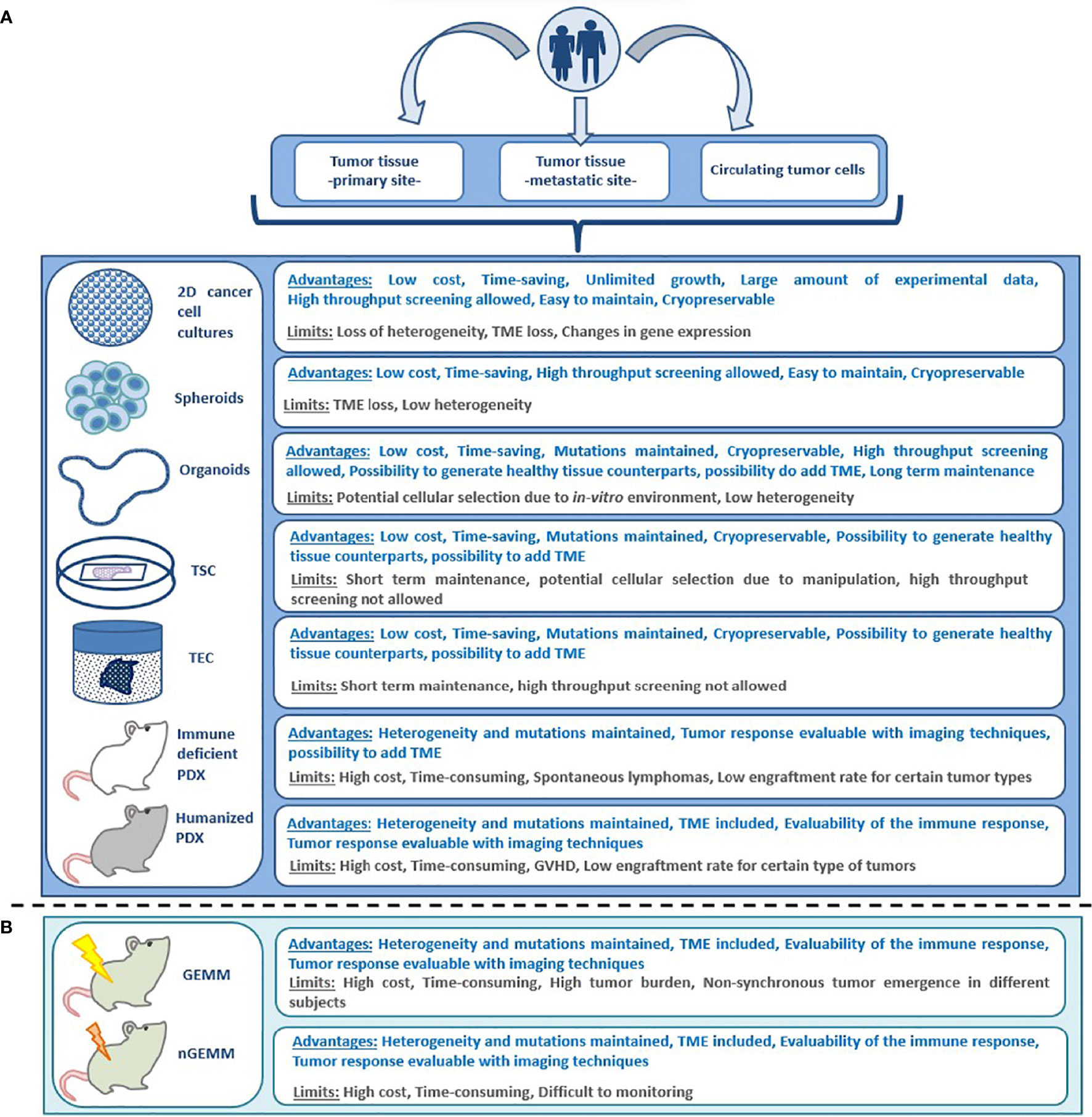
Figure 2 Advantages and limitations of different preclinical models for anticancer drug testing; (A) patient derived models, (B) non patient-derived animal models. GEMM, genetically engineered mouse model; GVHD, graft versus host disease; nGEMM, non-germinal genetically engineered mouse model; PDX, patient-derived xenograft; TEC, tumor explant culture; TME, tumor microenvironment; 2D, bi-dimensional; TSC, tumor slice culture.
2 Two- and three-dimensional cell cultures modeling
2.1 Cancer cell lines
Human cancer cell lines represent the earliest and most widely used preclinical model for the investigation of tumor biology and antitumor drugs testing (20). Starting from the 1950s in vitro cultures of immortalized cancer cells have been developed from a wide variety of haematological and solid malignancies. These models have been used to assess the effectiveness of investigational anticancer compounds taking advantage of their ease of maintenance and propagation, relatively low cost, reproducibility and high-throughput evaluation (21). However, antitumor activity demonstrated with this approach is often not confirmed in clinical settings, mainly due to the low resemblance to human cancers in vivo, and lack of well-defined parameters to translate in vitro sensitivity into predicted clinical success (22). This divergence depends on several factors. Firstly, the in vitro growth process results in the selection of clones with specific features promoting their survival, outliving other subpopulations. Secondly, the progressive adaptation to culture conditions results in a loss of heterogeneity and differentiation (23). Thirdly, the absence of a natural tumor microenvironment (TME) impairs the evaluation of drugs whose mechanism of action is based on cell-cell interactions or is related to angiogenesis (24). To create platforms with a higher similarity to human cancers and to represent a broader range of tumor types, three-dimensional cultures and in vivo models have been developed.
2.2 Spheroids
Tumor-derived spheroids are self-assembled micro-aggregates of cancer cells grown in a culture medium, under low-adhesion conditions. They can be generated from cancer cell cultures, patient-derived tumor cells (tumor spheres) or from suspension of single cells from cancer cell lines (25, 26). Spheroid generation is characterized by an initial phase of exponential growth followed by a period of structural organization, which leads to the formation of an external coat of proliferating cells surrounding a necrotic core (27, 28). The cell population located in the inner, hypoxic layers displays a quiescent status. This results in resistance to anticancer drugs exploiting high proliferative rate as a target, mimicking tumor behavior in vivo (27). The source of cells used to establish the spheroids has a big impact on the model’s characteristics: for example, spheroids derived from bi-dimensional cell lines will maintain cellular clonality while tumorspheres will display a higher heterogeneity (27, 29).
2.3 Organoids
Patient-derived organoids (PDOs) are preclinical models generated from cancer tissue, mechanically or enzymatically dissociated, and then embedded in an extracellular matrix. They differ from spheroids because they are self-organized in three-dimensional structures resembling the architecture and genomic features of the original tissue and retain the capability to regenerate (26, 30). The time required to generate organoids is variable and tumor dependant (31). PDOs of a wide range of malignancies have been established, with success rates up to 80% depending on tumor types (32–40). Mutagenesis technologies such as clustered, regularly interspaced, short palindromic repeats (CRISPR)/CRISPR-associated protein (Cas9) have been used to induce cancer-driving mutations to develop tumoral organoids starting from healthy human tissue (31, 41); an approach particularly useful to study carcinogenesis. Organoids derived from both neoplastic and healthy tissues can be established from the same patient to facilitate the identification of therapeutic agents with high antitumor activity and low impact on physiological tissues (42).
2.4 Ex-vivo models: Organotypic tumor slice and tumor explant cultures
The term ex-vivo is referred to models generated by tissue samples collected from an individual and then preserved in an artificial environment, outside the original organism (43). Organotypic tumor slice cultures (TSCs) are obtained by incubation of thin slices of tissue in controlled conditions allowing oxygen and nutrient distribution (44). This approach has been used to develop models of different types of malignancies, including breast, gastric, head and neck and pancreatic cancer (45–51). When compared to other preclinical models, TSCs offer some advantages including the preservation of an intact tumour microenvironment and a quicker set up, allowing timely drug testing (47). TSCs however, have main limitations such as rapid deterioration of cell viability and tissue architecture as well as unfeasibility of culture propagation (52). Moreover, the resemblance of these models to original tissue is highly influenced by procedural manipulation and sample processing (52). Tumour slice cultures represent only one of the multiple approaches attempted for the generation of ex-vivo models. Explanted cancer tissue can be preserved by submerging it in culture media or using a support to keep it in contact with the media such as gelatine sponges (53), grids or culture wells coated by a matrix (54, 55). One of the main advantages of these techniques as compared to tumor slice cultures is that they minimize tissue manipulation and assure higher tissue integrity. On the other hand, tissue slice models may allow a better distribution of anticancer drugs for testing. As for tumor slice cultures, short tissue viability is one of the main limitations for all tumor explant platforms (56). Several attempts have been made in order to delay models deterioration for example through the integration of microfluidic systems allowing prolonged tissue viablity (56).
3 In vivo models
3.1 Non-patient-derived in vivo models: The role of engineered mouse models
In vivo models enable the evaluation of cancer biology and treatment strategies in a complex organism. Genetically engineered mouse models (GEMMs) are transgenic mice harbouring alleles which lead to the spontaneous development of malignancies in immunocompetent animals (57). The development of GEMMs represented an important milestone in cancer research, as these models have been used to demonstrate that oncogene expression and tumor suppressor gene loss can induce neoplastic transformation of normal cells (58, 59). GEMMs have several limitations. The presence of pathogenic mutations affecting many target cells at an organism or tissue level can result in simultaneous development of multiple malignancies and consequently early death of the model. Furthermore, if present in germline cells, these mutations can affect embryonic viability, cause developmental abnormalities or impair normal tissue development (60). On the other hand, cancer onset in GEMMs can be delayed due to incomplete penetrance of the mutations, resulting in non-synchronous tumor occurrence in different mice and thus impairing the simultaneous evaluation of multiple anticancer agents. The development of non-germline GEMMs and conditional GEMMs, together with novel technologies for genome editing including CRISPR-Cas9 have helped overcome these limitations, enhancing the reliability of engineered mouse models in predicting drug responsiveness (59, 61, 62).
3.2 Patient-derived in-vivo models
Patient Derived Xenograft (PDXs) are preclinical models established from human neoplastic cells injection or tumor tissue implantation in immune-deficient animal hosts. PDXs are characterized by the maintenance of molecular and cellular heterogeneity of the primary tumor (63). The success rate of PDX establishment depends on multiple variables including the animal recipient, cancer type, and the technique used to implant the tumor (64). Metastatic tumors showing aggressive behaviour more frequently result in successful engraftment. Specific tumor types, such as colorectal or gastric cancer demonstrate a higher probability of engraftment compared with malignancies originating from other sites such as breast (particularly hormone dependent) or kidney (64–66). Many techniques have been used to optimize engraftment, including orthotopic transplant or, in the case of hormone-dependant cancers, the addition of human hormones (53, 67). Furthermore, the probability of obtaining successful engraftment increases with the degree of immunosuppression in the animal host. A greater rate of success can be achieved using animal models lacking functions of both B and T lymphocytes and of natural killer (NK) cells such as non-obese diabetic (NOD)/severe combined immunodeficient (SCID), in particular NOD/SCID/IL-2 receptor-γ deficient (NOG and NSG) and NOD/SCID/Janus kinase 3 deficient (NOJ) mouse models (64). Mice represent the most common type of host used for PDX generation however, other species can be used for this purpose (68–73). In-vivo models can also be generated in non-mammalian species, such as zebrafish (68). Both transgenic and xenograft models have been established for different tumor types including endocrine pancreatic cancer (69), multiple myeloma (74), head and neck squamous cell cancer (75), sarcoma (76)and melanoma (70–72), demonstrating some advantages in comparison with traditional mouse models. These include a higher rate of breeding, lower costs of maintenance and the possibility to track malignant cells with fluorescent labelling in the transparent casper zebrafish strain. Moreover, the process of engraftment for zebrafish PDXs is easier and faster compared to their murine counterparts (72, 73). To better recapitulate the original TME, humanized animal models have been developed (77, 78). These models can be obtained by xenotransplanting human immune cells or by engineering the host to express specific human genes. Humanized in vivo models, hosting not only human cancer cells but also a human-like TME are particularly suitable to test different anticancer strategies, including immunotherapy.
4 Key features of valuable platforms for antitumor drug testing
4.1 Genomic and transcriptomic fidelity
Faithful recapitulation of the molecular profile of the original tumor is one of the key characteristics to predict responsiveness to antitumor compounds. PDXs have been considered the most reliable reproduction of human cancers for a long time, retaining more than 80% of the genomic alterations harboured by the engrafted neoplastic tissue (79–81). However, several potential discrepancies have been identified. PDXs often demonstrate a higher aggressiveness with increased proliferation rate -especially at later passages- than human tumors in situ. Moreover, some evidence suggests that these models may acquire or select for copy number alterations and single nucleotide variants, or exhibit transcriptional alterations, which can affect the anticancer drug sensitivity (82, 83). Organoids and spheroids have emerged as cost-effective alternatives to animal models, with high genomic concordance with primary tumors (81, 84–88). Multiple factors however, can reduce their resemblance to original cancer tissue or affect their long-term preservation. The purity and the viability of the cancer cells selected to initiate the culture are of crucial importance to ensure successful generation of the model (42). The composition of the culture medium is another key factor. Appropriate nutrient and growth factor modulation are needed to avoid overgrowth of normal cells that would counteract the development of the cancer models (41). The composition of the medium has also demonstrated to influence epigenomic modulation and gene expression (89, 90) and to affect the consumption of glutamine, alanine and other elements from cancer cells (89). In vitro culture itself can lead to significant transcriptomic changes, resulting in the upregulation of several growth and metabolism-related pathways such as PI3K, glycolysis and oxidative phosphorylation (90). Some attempts have been made to compare the transcriptional faithfulness of different types of models. As an example, Da Peng et al. have developed the CancerCellNet (CCN), a computational tool evaluating the transcriptomic fidelity of cancer cell lines, PDXs, GEMMs and 3D cultures, through comparison with The Cancer Genome Atlas dataset (91). This approach is burdened by significant limitations, including the small number of models for specific tumor types, the lack of proteomic and epigenomic information, and the fact that the transcriptomic profiles are compared with bulk RNA that includes also non-cancer cells. However, CCN provides some interesting insights indicating that the suitability of different models in proving a faithful reproduction of the original tissue may vary across tumors originating from different sites. This should be considered in studies where generating preclinical models across different tumor types is planned. The application of machine learning algorithms to large datasets collecting genomic and transcriptomic profiles from thousands of patient-derived models can partially overcome the discrepancy with original tumours and optimize drug testing in preclinical studies (92–95). This approach has indeed facilitated the identification of genomic signatures predicting drug sensitivity with a greater precision than single-gene biomarkers (96). Moreover, integrating tumor profiling with analysis of other components of TME, such as the T-cells deep learning models can identify multidimensional biomarker signatures and unveil mechanisms of resistance to anticancer drugs (97, 98).
4.2 Preserving tumor heterogeneity
The capability of a model to replicate the molecular features of the original tissue is not enough to ensure success in predicting treatment response. Tumor heterogeneity plays a crucial role in promoting the onset and selection of genomic alterations that lead to cancer cell survival from targeted and immunotherapeutic agents (90). Both PDXs and 3D cultures have been originated from different portions of a same tumor lesion or from different metastatic sites to investigate this phenomenon (99–101). As an example, Li et al. generated PDOs from the primary tumour and paired liver metastases in two patients with colorectal cancer (100). The organoids derived from the metastases demonstrated a more aggressive phenotype with a greater propensity for invasion and higher replication index. Despite providing useful information, this approach is expensive, complex and requires multiple invasive procedures. More importantly, the understanding of genomic aberrations is still limited to specific biopsy sites, hence not practical to predict drug responsiveness. Circulating tumor cells (CTCs), released into the bloodstream from the primary tumour and secondary lesions represent a unique opportunity for the development of complex models for broad evaluation of the genomic landscape of metastatic tumors. CTCs are challenging to isolate as they occur at low frequencies. Data regarding the generation of 3D cultures from CTCs are still limited, however, some successful attempts have been reported (33, 102, 103). In 2014 Gao et al. generated PDOs from CTCs in one patient with prostate cancer with extensive metastatic disease (33). Whole-exome sequencing of PDOs and of a metastatic lymph node resected from the same patient one year before were compared. Only 67% of the point mutations found in the PDOs were identified in the archival tissue. While some of the mutations might be acquired during the culturing process this can reflect tumor heterogeneity. CTCs have also been used to establish animal models, known as cell line derived tumour xenografts (CDXs). Hodgkinson et al. generated CDX models by injecting blood obtained from 4 patients with small cell lung cancer (SCLC), enriched with CTCs (104). Genomic analysis of CDXs shown preservation of original mutational profile, moreover these models mimicked patients responses to chemotherapy. Despite representing a possible strategy to overcome tumor heterogeneity, this approach has some limitations including technical challenges in isolation and expansion of CTCs, lack of stromal and immune components, uncertain representation of different metastatic sites and possible selection of specific clones (105). Despite using CTCs to initiate a patient-derived model the preservation of tumor heterogeneity might be challenged by the fact that cancer cells with particular molecular alterations are more difficult to expand. As an example Li et al. reported a lower rate of success for organoids generation from colorectal cancer with microsatellite instability (MSI) or BRAF mutations (106). Interestingly when looking at the possible causes contributing to the failure of these models authors observed that cancer cells harbouring MSI or BRAF mutations were more dependant for their survivorship on other components of the tumor microenvironment such as immune cells. This indicate that the maintenance of a proficient TME aside from enabling the evaluation of anticancer therapies such as immunotherapy might be a key factor also for the maintenance of tumor heterogeneity.
4.3 Tumor Microenvironment Preservation
4.3.1 Tumor microenvironment preservation in 3D cultures
There has been much recent effort to develop preclinical models with an intact TME preserving functional immune effectors (25, 107). 3D models hosting competent immune cells can be obtained by co-culturing previously expanded immune cells (108). Both spheroid (109–111), and organoid (112, 113) cultures have been developed with this technique. T cells generated using this process were shown to efficiently kill cancer cells, while they did not show activity against organoids originated from healthy tissue, confirming the maintenance of self-tolerance (112). Courau et al. used this approach to generate immune-competent spheroids from colorectal cancer by co-culturing these models with immune cells obtained from healthy donors (111). They observed rapid infiltration from allogenic transferred T and NK cells, resulting in immune-related cell death. Moreover, they used this platform to test the activity of antibodies targeting natural killer group 2 member D (NKG2D) and its ligands. They observed an enhancement of spheroids immune-mediated killing, driven by an increase of NK infiltration, supporting the utility of this type of model to identify new therapeutic strategies. The co-culturing approach, however, does not fully recapitulate the complexity of the TME, as it lacks native infiltrating immune populations and other key factors, regulating the interaction between cancer and immune system. The extracellular matrix (ECM), the complex of proteins, polysaccharides and other elements surrounding the cells, give structure and sustain both normal and neoplastic tissues. Cancer-associated fibroblasts producing collagen as well as alterations of other elements of ECM, such as hyaluronic acid, metalloproteases and lysyl oxidases are known to promote cancer initiation and invasion through ECM remodeling (114) and have been related to resistance to multiple anticancer agents (115). Moreover, the recognition of the key role played by ECM in cancer resulted in the development of antitumor drugs directly targeting ECM molecules or the cell-matrix crosstalk (115). This led to an urgent need of preclinical models for drug testing retaining a functional ECM. Multiple synthetic and biological materials have been used to develop scaffolds to support the generation of 3D cultures, mimicking ECM. Examples include hydroxyapatite-graphene (116), polyethylene glycol oxide (117), chitosan alginate (118–120), collagen (76, 121) and matrigel (117, 122). Different materials present specific advantages but also limitations. As an example hydrogels are highly biocompatible and recapitulate the biochemical composition of original matrix but offer low mechanical resistance (122). To include original immune populations different 3D models have been developed (123). The feasibility of this approach has been initially demonstrated in healthy human epithelial breast tissue (124). Zumwalde et al. observed the presence of T cells in mammary ductal epithelial PDOs, producing interferon (IFN)-γ and proliferating in response to zoledronic acid. Interestingly, these lymphocytes showed cytotoxic activity towards a triple negative breast carcinoma cell line. The air-liquid interface technique allows to generate PDOs from both healthy and neoplastic tissue with preserved epithelial and mesenchymal components, retaining proficient immune effectors and ECM (123, 125, 126). This method enables organoids to be propagated as epithelial-mesenchymal hybrids using an inner collagen gel–containing transwell with direct air exposure (127). Using this approach Neal et al. generated PDOs from 28 distinct tumor types of human and murine malignancies including colorectal, kidney, lung and pancreatic cancer (123). Human PDO analysis demonstrated the presence of CD3+ tumor infiltrating lymphocytes (TILs), macrophages, B and NK cells. Single-cell gene expression profiling indicated that TILs present within PDOs maintained the original TCR repertoire observed in the tumor biopsy. Protein death 1 (PD-1) expression was observed on the surface of immune cells included in the cultures and the exposure to anti-PD/PD-ligand 1 (PD-L1) agents resulted in the expansion of TILs and in the promotion of neoplastic cells killing. The air-liquid interface technique is not the only possible approach to preserve original TME. Jacob et al. successfully generated PDOs from glioblastoma patients performing microdissection of original tissue into pieces of ≈1 mm diameter instead of dissociation, in order to preserve native cell-cell interactions (128). Single-cell transcriptome analysis shown similar cytokine expression in macrophage and microglia from original tissue and PDOs. Moreover, a similar distribution of cells in PDOs generated at later time points was observed, indicating the capability of this model to preserve and maintain at least in part the features of parental TME. Once established, these models have been used to test multiple antitumor compounds, including CAR-T cells. The resemblance of PDO models to the parental cancer can be further implemented by the organs-on-a-chip technology (129). Through the use of customized microfluidic cell culture devices, this approach allows the vascularization of 3D cultures, mimicking physiological delivery of drugs through the blood vessels (130). Chemo- and biosensors can be integrated in this type of models to optimize the control of oxygen and metabolites levels (131). 3D bioprinting techniques can also be used to develop preclinical models fully recapitulating the architecture of parental tumors, including a functional vascular system and enabling a uniform distribution of different cellular components (132, 133). This approach consists in the controlled deposition of layers of patient-derived cancer and stromal cells, signalling molecules and other biomaterials to generate spheroids or organoids with a functional TME. The use of 3D bioprinting is rapidly expanding and different systems are currently available including extrusion-based bioprinting, laser-based bioprinting, and droplet-based bioprinting (134). 3D bioprinted PDOs and spheroids have already been successful established for multiple cancer types including glioblastoma, neuroblastoma, multiple myeloma, melanoma and cholangiocarcinoma and used to test novel anticancer treatments such as oncolytic viruses (135–141). TEC and TSC represent another possible approach to preserve TME and test immune-oncology strategies, despite the limited time frame for drug-testing. As an example, Sivakumar et al. successfully used TSC to test the effect of IFN-γ and PD L1 blockage (47).
4.3.2 Immune-competent in-vivo models
The reconstruction of a proficient TME can be applied to in vivo models. In GEMMs, spontaneous neoplastic transformation in immunocompetent animals leads to the onset of tumors retaining TME (61, 142). However, the cross-reactivity between the murine and the human targets, especially when the tested agents need antigen presentation by human MHC class I, limits the use of GEMMs to test immunotherapy (143). Humanized animal models are promising platforms for the evaluation of immunotherapeutic strategies. Knock-in mice expressing key human genes regulating the cross-talk between cancer and immune system have been generated, including PD-1 (144), PDL1 (145), LAG3 (145), CTLA4 (77) and IL-15 (146). These platforms could be useful to not only to test drug sensitivity but also to study immune related toxicities associated with monoclonal antibodies specifically binding human targets, such as human PD1 or CTLA4. As an example, Du et al. used CTLA4 humanized mice to test anti-CTLA4 antibodies including ipilimumab, alone and in combination with an anti-PD1 (77). They observed correlation between the development of immune related toxicity and systemic T cell activation with an increased percentage of effector T memory lymphocytes. Humanized PDX models obtained with the engraftment of human immune cells into immunocompromised animals represent another possible approach to obtain animal models suitable for immunotherapy testing (78). This strategy is however burdened by multiple limitations (147). Firstly, the efficacy of the model is limited by the severity of the immunosuppression of the PDX hosts; models with impaired T-cells function but maintained innate immunity will reject human cells. Secondly, since the hematopoietic cells cannot be propagated across multiple animals, this approach requires sequential blood draws from the patients. Finally, the infusion of human hematopoietic cells results in graft versus host disease of PDXs, limiting the timeframe for observation. The transfer of hematopoietic cells is not the only option attempted to generate humanized PDX models. The injection of patient-specific TILs has demonstrated to mimic antitumor responses observed in patients (148). This technique has contributed to identify a dysfunctional subset of CD8+ cells as possible mechanism of resistance to PD 1 inhibitors in NSCLC (149). Ex-vivo expansion and subsequent re-infusion of TILs is not the only approach attempted in order to elicit antitumor activity. Yin et al. injected nanoparticle incorporating immunostimulants molecules to induce antitumor activity in endogenous TILs in mouse models. This strategy resulted in CD8+ cells expansion and reduction of regulatory T cells as well as in a delay of tumour growth (150).
4.4 Evaluating the impact of microbiome
The human microbiota, the set microorganisms populating human epithelial surfaces, influences the development of several pathologic conditions, including cancer (151). For patient-derived models, this has important conceptual implications: First - for some patient-derived models, the microbial ecology of the model (e.g. the experimental animal’s endogenous microbiota) may influence experimental outcomes and therefore must be accounted for in experimental design and analysis. For example, in murine models, vendor-specific microbiota (152), microbial metabolites (153) and microbiome-tumour neoantigen cross-reactivity (154) have all been implicated in immunotherapy responsiveness. Thus, ignorance of the composition or contribution of the microbiota to model outcomes may result in contradictory findings between investigators, or even within a research group based on variability in the composition of the microbiome between experimental replicates. Secondly - experimental manipulation of the microbiome in patient-derived models may identify novel (host or microbial) targets representing promising therapeutic avenues (155). Both 3D cultures and animal models have been used to mechanistically implicate the microbiome in cancer biology. Using intestinal PDOs, Kadosh et al. observed that the addition of the microbial metabolite gallic acid alters the effect of Trp53 gain of function mutations from tumor-suppressive to pro-oncogenic (156). In adenomatous polyposis coli (APC)-mutated mouse models, depletion of Streptococcus thermophilus plays a key role in colorectal cancer tumorigenesis (157). The impact of probiotic and high fiber diet on immunotherapy outcomes have been evaluated in several studies showing controversial results (158, 159). Spencer et al. observed an association between higher dietary fiber content and prolonged progression-free survival in melanoma patients receiving ICI while the use of probiotic shown a detrimental effect in the same population (160). To validate these results, they tested the influence of fiber and probiotics on anti-PD1 responses in patient-derived mouse models, confirming that a diet with lower fiber content and addition of a probiotic reduced cytotoxic TILs (160). Finally, mice with microbiome transplanted from human donors (with cancer) represent a type of patient-derived model themselves (161). Routy et al. compared mice with fecal microbiomes transplanted from human patients who responded to anti-PD1 antibody and those receiving fecal transplant from non-responders and found a greater density of intratumoral CD8+ T cells, upregulation of PD L1 and a lower presence of myeloid suppressive cells was in mice transplanted with responders’ stools, suggesting microbiota-induced “hot” TME (162). Critically, these observations have led to the development of novel, microbiome-targeting therapeutic strategies. Shi et al. evaluated the impact of combining a probiotic agent, Escherichia coli strain Nissle 1917, to an anti-Transforming Growth Factor, Beta (TGF-β) compound in mice transplanted with breast and hepatocellular carcinoma cells (163). They reported greater tumor growth inhibition and metastasis suppression in models receiving the probiotic. They also observed an increase in the proportion of intratumoral CD8+ T cells and greater numbers of mature dendritic cells in tumour-draining lymph nodes. Fecal transplants from treatment-responsive donors have been investigated in clinical trials assessing their potential to restore sensitivity to immunotherapy in refractory melanoma (164, 165). These data highlight the experimental importance and potential utility of microbiome-informed preclinical studies and the potential for patient-derived microbiome models for the investigation of cancer biology and therapeutic discovery (147).
5 Inclusion of patient-derived models in co-clinical trials
Patient-derived platforms have allowed investigators to perform drug testing in models originated from subjects simultaneously receiving therapy in clinical trials. These types of studies, known as co-clinical trials, use laboratory data to guide clinical development or treatment strategies, with the final goal of identifying predictive biomarkers and increasing the rate of success of experimental treatments (166). As an example, Kim et al. tested the fibroblast growth factor receptor (FGFR) inhibitor dovitinib in PDXs derived from squamous cell lung cancer patients treated with the same drug in a clinical study (167). They observed preservation of genomic and histologic profiles of parental tumours in PDXs. The responsiveness to dovitinib displayed by the models recapitulated the clinical outcomes observed in the patients. Gene expression profiling performed in the PDXs indicated upregulation of FGF3 and FGF19 in responders, representing a potential predictive biomarker. The Gustave Roussy MATCH-R project is another example of a co-clinical trial. This is a prospective study aimed at extensive characterization of tumours with acquired resistance to immunotherapeutic agents or targeted therapies. Generation of PDX models for 54 patients with a success rate of 33% and the highest probability of engraftment for urothelial bladder cancer (72.7%) was reported (168). Despite these models being helpful to provide further insights into the mechanism of drug resistance, the authors reported that the outcome data from drug testing were often not timely to guide clinical decision-making. Having a faster turnaround time and being easier to maintain, PDOs might be more suitable for co-clinical studies (169). Yao et al. used PDOs originated from patients with rectal cancer to test the efficacy of chemoradiation and compared it with clinical outcomes (170). The authors reported poor response to chemoradiation in 42/64 patients whose models were resistant, and a good response in 16/17 patients with matched responsive PDOs. They also tested the responsiveness to single components of the chemoradiation regiment (5-FU, irinotecan and radiation) and correlated it with clinical responses. Good clinical outcomes were observed in patients whose PDOs were sensitive to one, two or all the three agents. A good clinical response was reported in 3 patients with PDOs resistant to all three components of the chemoradiation regimen, tested separately. Interestingly, when these models were exposed to the combination, drug synergy was demonstrated in one of the PDOs indicating the potential utility of these models to explore combined treatments to overcome drug resistance. The reliability of PDOs in predicting responses to radiation and chemotherapy in patients with rectal cancer has been evaluated also by further co-clinical studies (171–173). Park et al. developed PDOs from 33 patients radiation for retal adenocarcinoma and confirmed the possibility to use these models to predict sensibility through a machine-learning algorithm (171). In another study, Ganesh et al. were able to generate 65 PDOs from 41 patients with newly diagnosed, metastatic or recurrent rectal cancer with a success rate of 77% (172). Interestingly, 43/65 PDOs were established from samples obtained after exposure to 1 or 2 lines of systemic therapy demonstrating the possibility to generate 3D cultures from pre-treated tumors. Hu et al. have shown that is possible to minimize the time for anticancer drug testing by using microwell arrays that enable to evaluate PDOs sensitivity to hundreds of different compounds at passage 0 (174). Aside from allowing a timely determination of anticancer activity the possibility to perform drug testing at an early stage might reduce the risk of phenotypic changes at later passages and the need of complex culture media with growth factors enrichment. Acoustic droplet printing (ADP) is another approach attempted to decrease the time necessary for PDOs generation (134, 175, 176). This technique enables the development of PDOs in 2 weeks, with preservation of autologous immune cells and integration of a microfluidic system for drug delivery (134). These characteristics make ADP-generated PDOs a promising platform for anticancer drug testing including immunotherapeutic agents. Another critical step to implement co-clinical trial is the definition of fast and standardized methods for the interpretation of antitumor activity in the pre-clinical models. Usually drug sensitivity is estimated using cell viability assays such as ATP-dependent luminescence, tetrazolium-based colorimetric techniques, or fluoresce-based assays (90). These techniques however results PDOs death impairing sequential assessments (177). Emergent technologies such as label-free light microscopy and positron-emission microscopy have been tested to evaluate the antitumor activity of investigational agents in 3D cultures (90, 178, 179). Light microscopy might provide a more precise measurement of antitumor activity because it allows the evaluation of cell viability at single organoid level (90). Moreover, this technique demonstrated the potential to detect not only cytotoxic but also cytostatic activity (90). With the use of positron-emission microscopy was possible to establish that PDOs retain metabolic characteristics of parental tumours, indicating that this technique can be used to monitor responses in the organoid cultures (178). Even when the data obtained from preclinical models are not suitable to guide treatment decisions the information provided by these platforms might be precious to unveil mechanisms underlying drug resistances and develop strategies to restore or increase responsiveness to treatments (180, 181). Moreover, this approach can be used to support the development of non-invasive techniques for the prediction of disease response. As an example, Roy et al. used patient derived PDXs of TNBC to identify [(18)F] fluorodeoxyglucose with positron emission tomography radiomic signatures of response to neoadjuvant chemotherapy (182). A list of co-clinical trials currently ongoing is reported in Table 1.
6 Conclusion and future perspectives
Patient-derived models are powerful tools with multiple applications in oncology. Their molecular characterization and incorporation in multiomic biomarker-driven studies is crucial to identify mechanisms of resistance to anticancer treatments and guide the development of effective therapeutic strategies. The Immune Resistance Interrogation Study (IRIS, NCT04243720) currently ongoing at our institution, NEO-R (NCT04504747) and PITCHER (NCT04714957) are some examples of prospective trials using this approach. High genomic and transcriptomic fidelity, preservation of tumor heterogeneity and presence of a proficient TME are some of the key factors that should be implemented to obtain results that can be translated into clinic. The availability of large PDO and PDX repositories combined with the development of machine learning techniques can partially bridge the molecular gap with original tumors and optimize drug testing in preclinical studies (92–95). Until today, tumor heterogeneity represented a main pitfall, jeopardizing the reliability of preclinical models in predicting drug sensitivity. Although there is a big caveat on the success and expansion of CTCs, there is a question of whether they could help overcome this limitation by capturing inter- and intratumoral heterogeneity. The use of 3D bioprinting techniques might enable the development of complex 3D cultures starting from CTCs, comprising proficient autologous immune cells and vascular system. Aside from overcoming tumour heterogeneity, the use of CTCs as primary source for the development of cancer models may offer further advantages. Due to its relatively low invasiveness, a liquid biopsy-based approach might be particularly useful to investigate mechanisms of acquired resistance through the comparison of CDXs obtained by sequential blood draws. Finally multiple strategies have been implemented to preserve a functional TME leading to the development of complex in-vivo, ex-vivo and in-vitro models. These platforms may enable a deeper understanding of the factors regulating the networking between cancer cells and immune system, such as microbiome and ECM. Moreover humanized in-vivo models and 3D cultures retaining functional immune effectors and ECM are promising tools to test not only immunotherapy but also novel therapeutic strategies targeting critical processes underlying cancer initiation and progression such as matrix deposition and remodeling. Further elements should be taken into consideration if the patient derived model is intended to guide treatment selection in the context of a co-clinical trial. The models are highly time-sensitive. Despite the molecular affinity with the parental tumors, mouse PDXs need months to be established; therefore, limiting their applicability in treatment decision making, as demonstrated by the MATCH-R study (168). Alternative models including 3D cell cultures, along with innovative techniques such as 3D bioprinting and microwell arrays could overcome these limitations and have higher molecular fidelity thanks to the limited number of passages between tissue collection and drug-testing.
Author contributions
SG: conceptualization and original draft preparation; BC, DC, and AS: supervision and manuscript review/editing. All authors have read and agreed to the published version of the manuscript.
Conflict of interest
BC: Research funding: Nubiyota, Sanofi. DC: Consulting/Advisory: AstraZeneca, Extract Sciences, Eisai, Gilead, GlaxoSmithKline, Inivata, Merck, Novartis, Pfizer and Roche. Research funding to institution: AstraZeneca, Gilead, GlaxoSmithKline, Inivata, Merck, Pfizer and Roche. Patent (US62/675,228) for methods of treating cancers characterized by a high expression level of spindle and kinetochore associated complex subunit 3 (ska3) gene. AS: Consultant for (Advisory Board): Merck (compensated), Bristol-Myers Squibb (compensated), Novartis (compensated), Oncorus (compensated), Janssen (compensated). Grant/Research support from (Clinical Trials): Novartis, Bristol-Myers Squibb, Symphogen AstraZeneca/Medimmune, Merck, Surface Oncology, Northern Biologics, Janssen Oncology/Johnson & Johnson, Roche, Regeneron, Alkermes, Array Biopharma/Pfizer, GSK.
The remaining author declares that the research was conducted in the absence of any commercial or financial relationships that could be construed as a potential conflict of interest.
Publisher’s note
All claims expressed in this article are solely those of the authors and do not necessarily represent those of their affiliated organizations, or those of the publisher, the editors and the reviewers. Any product that may be evaluated in this article, or claim that may be made by its manufacturer, is not guaranteed or endorsed by the publisher.
Abbreviations
ADP, Acoustic droplet printing; BC, breast cancer; BRAF, B-rapidly accelerated fibro sarcoma; CAR-T, chimeric antigen receptor T-cell; CCN, CancerCellNet; CDX, cell line derived tumor xenograft; CRC, colorectal cancer; CRISPR-Cas9, clustered regularly interspaced short palindromic repeats/associated protein; CTC, circulating tumor cell; GEMM, Genetically engineered mouse model; ECM, extracellular matrix; EGFR, epidermal growth factor receptor; HER-2, epidermal growth factor receptor 2; HNSCC, head and neck squamous cell cancer; ICI, immune checkpoint inhibitors; IFN-γ, interferon gamma; MHC, major histocompatibility complex; NSCLC, non-small cell lung cancer; NK, natural killer; NOD/SCID, non-obese diabetic/severe combined immunodeficient; OS, overall survival; PD-1, protein death 1; PD-L1, protein death 1ligand; PDO, patient-derived organoid; PDX, patient derived xenograft; TCR, T cell receptor; TEC, tumour explant cultures; TIL, tumor infiltrating lymphocyte; TME, tumor microenvronment; TNBC, triple negative breast cancer; TSC, tumour slice cultures.
References
1. Luo J, Solimini NL, Elledge SJ. Principles of cancer therapy: oncogene and non-oncogene addiction. Cell (2009) 136(5):823–37. doi: 10.1016/j.cell.2009.02.024
2. Hanahan D, Weinberg RA. Hallmarks of cancer: the next generation. Cell (2011) 144(5):646–74. doi: 10.1016/j.cell.2011.02.013
3. Dienstmann R, Rodon J, Barretina J, Tabernero J. Genomic medicine frontier in human solid tumors: prospects and challenges. J Clin Oncol (2013) 31(15):1874–84. doi: 10.1200/JCO.2012.45.2268
4. Li J, Kwok HF. Current Strategies for Treating NSCLC: From Biological Mechanisms to Clinical Treatment. Cancers (Basel) (2020). doi: 10.3390/cancers12061587
5. Loibl S, Gianni L. HER2-positive breast cancer. Lancet (2017) 389(10087):2415–29. doi: 10.1016/S0140-6736(16)32417-5
6. El Dika I, Ilson DH. Current and future therapies for targeting HER2 mutations in gastrointestinal cancer. Expert Rev Anticancer Ther (2018) 18(11):1085–92. doi: 10.1080/14737140.2018.1510324
8. Velcheti V, Schalper K. Basic Overview of Current Immunotherapy Approaches in Cancer. Am Soc Clin Oncol Educ Book (2016) 35:298–308. doi: 10.14694/EDBK_15657210.1200/EDBK_156572
9. Feins S, Kong W, Williams EF, Milone MC, Fraietta JA. An introduction to chimeric antigen receptor (CAR) T-cell immunotherapy for human cancer. Am J Hematol (2019) 94(S1):S3–9. doi: 10.1002/ajh.25418
10. Gong J, Chehrazi-Raffle A, Reddi S, Salgia R. Development of PD-1 and PD-L1 inhibitors as a form of cancer immunotherapy: a comprehensive review of registration trials and future considerations. J Immunother Cancer (2018) 6(1):8. doi: 10.1186/s40425-018-0316-z
11. Sharma P, Hu-Lieskovan S, Wargo JA, Ribas A. Primary, Adaptive, and Acquired Resistance to Cancer Immunotherapy. Cell (2017) 168(4):707–23. doi: 10.1016/j.cell.2017.01.017
12. Hu X, Zhang Z. Understanding the Genetic Mechanisms of Cancer Drug Resistance Using Genomic Approaches. Trends Genet (2016) 32(2):127–37. doi: 10.1016/j.tig.2015.11.003
13. Bedard PL, AR H, MJ R, Siu LL. Tumour heterogeneity in the clinic. Nature (2013) 501(7467):355–64. doi: 10.1038/nature12627
14. Sarmento-Ribeiro AB, Scorilas A, Goncalves AC, Efferth T, Trougakos IP. The emergence of drug resistance to targeted cancer therapies: Clinical evidence. Drug Resist Update (2019) 47:100646. doi: 10.1016/j.drup.2019.100646
15. Tentler JJ, Tan AC, Weekes CD, Jimeno A, Leong S, Pitts TM, et al. Patient-derived tumour xenografts as models for oncology drug development. Nat Rev Clin Oncol (2012) 9(6):338–50. doi: 10.1038/nrclinonc.2012.61
16. Weeber F, Ooft SN, Dijkstra KK, Voest EE. Tumor Organoids as a Pre-clinical Cancer Model for Drug Discovery. Cell Chem Biol (2017) 24(9):1092–100. doi: 10.1016/j.chembiol.2017.06.012
17. Aparicio S, Hidalgo M, Kung AL. Examining the utility of patient-derived xenograft mouse models. Nat Rev Cancer (2015) 15(5):311–6. doi: 10.1038/nrc3944
18. Izumchenko E, Meir J, Bedi A, Wysocki PT, Hoque MO, Sidransky D. Patient-derived xenografts as tools in pharmaceutical development. Clin Pharmacol Ther (2016) 99(6):612–21. doi: 10.1002/cpt.354
19. Cescon D, Siu LL. Cancer Clinical Trials: The Rear-View Mirror and the Crystal Ball. Cell (2017) 168(4):575–78. doi: 10.1016/j.cell.2017.01.027
20. Gillet JP, Varma S, Gottesman MM. The clinical relevance of cancer cell lines. J Natl Cancer Inst (2013) 105(7):452–8. doi: 10.1093/jnci/djt007
21. Goodspeed A, Heiser LM, Gray JW, Costello JC. Tumor-Derived Cell Lines as Molecular Models of Cancer Pharmacogenomics. Mol Cancer Res (2016) 14(1):3–13. doi: 10.1158/1541-7786.MCR-15-0189
22. DiMasi JA, Reichert JM, Feldman L, Malins A. Clinical approval success rates for investigational cancer drugs. Clin Pharmacol Ther (2013) 94(3):329–35. doi: 10.1038/clpt.2013.117
23. Gao D, Chen Y. Organoid development in cancer genome discovery. Curr Opin Genet Dev (2015) 30:42–8. doi: 10.1016/j.gde.2015.02.007
24. Sharma SV, Haber DA, Settleman J. Cell line-based platforms to evaluate the therapeutic efficacy of candidate anticancer agents. Nat Rev Cancer (2010) 10(4):241–53. doi: 10.1038/nrc2820
25. Costa EC, Moreira AF, de Melo-Diogo D, Gaspar VM, Carvalho MP, Correia IJ. 3D tumor spheroids: an overview on the tools and techniques used for their analysis. Biotechnol Adv (2016) 34(8):1427– 41. doi: 10.1016/j.biotechadv.2016.11.002
26. Clevers H. Modeling Development and Disease with Organoids. Cell (2016) 165(7):1586–97. doi: 10.1016/j.cell.2016.05.082
27. Zanoni M, Cortesi M, Zamagni A, Arienti C, Pignatta S, Tesei A. Modeling neoplastic disease with spheroids and organoids. J Hematol Oncol (2020) 13(1):97. doi: 10.1186/s13045-020-00931-0
28. Minchinton AI, Tannock IF. Drug penetration in solid tumours. Nat Rev Cancer (2006) 6(8):583–92. doi: 10.1038/nrc1893
29. Carragher N, Piccinini F, Tesei A, Trask OJ Jr., Bickle M, Horvath P. Concerns, challenges and promises of high-content analysis of 3D cellular models. Nat Rev Drug Discovery (2018) 17(8):606. doi: 10.1038/nrd.2018.99
30. Gunti S, Hoke ATK, Vu KP, London NR Jr.. Organoid and Spheroid Tumor Models: Techniques and Applications. Cancers (Basel) (2021). doi: 10.3390/cancers13040874
31. Tuveson D, Clevers H. Cancer modeling meets human organoid technology. Science (2019) 364(6444):952–55. doi: 10.1126/science.aaw6985
32. Boj SF, Hwang CI, Baker LA, Chio II, DD E, Corbo V, et al. Organoid models of human and mouse ductal pancreatic cancer. Cell (2015) 160(1-2):324–38. doi: 10.1016/j.cell.2014.12.021
33. Gao D, Vela I, Sboner A, Iaquinta PJ, Karthaus WR, Gopalan A, et al. Organoid cultures derived from patients with advanced prostate cancer. Cell (2014) 159(1):176–87. doi: 10.1016/j.cell.2014.08.016
34. Sachs N, de Ligt J, Kopper O, Gogola E, Bounova G, Weeber F, et al. A Living Biobank of Breast Cancer Organoids Captures Disease Heterogeneity. Cell (2018) 172(1-2):373–86 e10. doi: 10.1016/j.cell.2017.11.010
35. van de Wetering M, Francies HE, Francis JM, Bounova G, Iorio F, Pronk A, et al. Prospective derivation of a living organoid biobank of colorectal cancer patients. Cell (2015) 161(4):933–45. doi: 10.1016/j.cell.2015.03.053
36. Broutier L, Mastrogiovanni G, Verstegen MM, Francies HE, Gavarro LM, Bradshaw CR, et al. Human primary liver cancer-derived organoid cultures for disease modeling and drug screening. Nat Med (2017) 23(12):1424–35. doi: 10.1038/nm.4438
37. Lee SH, Hu W, Matulay JT, Silva MV, Owczarek TB, Kim K, et al. Tumor Evolution and Drug Response in Patient-Derived Organoid Models of Bladder Cancer. Cell (2018) 173(2):515–28.e17. doi: 10.1016/j.cell.2018.03.017
38. Nanki Y, Chiyoda T, Hirasawa A, Ookubo A, Itoh M, Ueno M, et al. Patient-derived ovarian cancer organoids capture the genomic profiles of primary tumours applicable for drug sensitivity and resistance testing. Sci Rep (2020) 10(1):12581. doi: 10.1038/s41598-020-69488-9
39. Wilson BE, Gorrini C, Cescon DW. Breast cancer immune microenvironment: from pre-clinical models to clinical therapies. Breast Cancer Res Treat (2021). doi: 10.1007/s10549-021-06431-0
40. Driehuis E, Kretzschmar K, Clevers H. Establishment of patient-derived cancer organoids for drug- screening applications. Nat Protoc (2020) 15(10):3380–409. doi: 10.1038/s41596-020-0379-4
41. Drost J, van Boxtel R, Blokzijl F, Mizutani T, Sasaki N, Sasselli V, et al. Use of CRISPR-modified human stem cell organoids to study the origin of mutational signatures in cancer. Science (2017) 358(6360):234–38. doi: 10.1126/science.aao3130
42. Drost J, Clevers H. Organoids in cancer research. Nat Rev Cancer (2018) 18(7):407–18. doi: 10.1038/s41568-018-0007-6
43. Powley IR, Patel M, Miles G, Pringle H, Howells L, Thomas A, et al. Patient-derived explants (PDEs) as a powerful preclinical platform for anti-cancer drug and biomarker discovery. Br J Cancer (2020) 122(6):735–44. doi: 10.1038/s41416-019-0672-6
44. Vaira V, Fedele G, Pyne S, Fasoli E, Zadra G, Bailey D, et al. Preclinical model of organotypic culture for pharmacodynamic profiling of human tumors. Proc Natl Acad Sci U.S.A. (2010) 107(18):8352–6. doi: 10.1073/pnas.0907676107
45. Misra S, Moro CF, Del Chiaro M, Pouso S, Sebestyen A, Lohr M, et al. Ex vivo organotypic culture system of precision-cut slices of human pancreatic ductal adenocarcinoma. Sci Rep (2019) 9(1):2133. doi: 10.1038/s41598-019-38603-w
46. Salas A, Lopez J, Reyes R, Evora C, de Oca FM, Baez D, et al. Organotypic culture as a research and preclinical model to study uterine leiomyomas. Sci Rep (2020) 10(1):5212. doi: 10.1038/s41598-020-62158-w
47. Sivakumar R, Chan M, Shin JS, Nishida-Aoki N, Kenerson HL, Elemento O, et al. Organotypic tumor slice cultures provide a versatile platform for immuno-oncology and drug discovery. Oncoimmunology (2019) 8(12):e1670019. doi: 10.1080/2162402X.2019.1670019
48. Kenerson HL, Sullivan KM, Seo YD, Stadeli KM, Ussakli C, Yan X, et al. Tumor slice culture as a biologic surrogate of human cancer. Ann Transl Med (2020) 8(4):114. doi: 10.21037/atm.2019.12.88
49. Weitz JR, Tiriac H, Hurtado de Mendoza T, Wascher A, Lowy AM. Using Organotypic Tissue Slices to Investigate the Microenvironment of Pancreatic Cancer: Pharmacotyping and Beyond. Cancers (Basel) (2021). doi: 10.3390/cancers13194991
50. Gerlach MM, Merz F, Wichmann G, Kubick C, Wittekind C, Lordick F, et al. Slice cultures from head and neck squamous cell carcinoma: a novel test system for drug susceptibility and mechanisms of resistance. Br J Cancer (2014) 110(2):479–88. doi: 10.1038/bjc.2013.700
51. Lim CY, Chang JH, Lee WS, Lee KM, Yoon YC, Kim J, et al. Organotypic slice cultures of pancreatic ductal adenocarcinoma preserve the tumor microenvironment and provide a platform for drug response. Pancreatology (2018) 18(8):913–27. doi: 10.1016/j.pan.2018.09.009
52. Klinghammer K, Walther W, Hoffmann J. Choosing wisely - Preclinical test models in the era of precision medicine. Cancer Treat Rev (2017) 55:36–45. doi: 10.1016/j.ctrv.2017.02.009
53. Centenera MM, Hickey TE, Jindal S, Ryan NK, Ravindranathan P, Mohammed H, et al. A patient- derived explant (PDE) model of hormone-dependent cancer. Mol Oncol (2018) 12(9):1608–22. doi: 10.1002/1878-0261.12354
54. Majumder B, Baraneedharan U, Thiyagarajan S, Radhakrishnan P, Narasimhan H, Dhandapani M, et al. Predicting clinical response to anticancer drugs using an ex vivo platform that captures tumour heterogeneity. Nat Commun (2015) 6:6169. doi: 10.1038/ncomms7169
55. Ghosh S, Prasad M, Kundu K, Cohen L, Yegodayev KM, Zorea J, et al. Tumor Tissue Explant Culture of Patient-Derived Xenograft as Potential Prioritization Tool for Targeted Therapy. Front Oncol (2019) 9:17. doi: 10.3389/fonc.2019.00017
56. Dorrigiv D, Simeone K, Communal L, Kendall-Dupont J, St-Georges-Robillard A, Peant B, et al. Microdissected Tissue vs Tissue Slices-A Comparative Study of Tumor Explant Models Cultured On-Chip and Off-Chip. Cancers (Basel) (2021). doi: 10.3390/cancers13164208
57. Kersten K, de Visser KE, van Miltenburg MH, Jonkers J. Genetically engineered mouse models in oncology research and cancer medicine. EMBO Mol Med (2017) 9(2):137–53. doi: 10.15252/emmm.201606857
58. Sinn E, Muller W, Pattengale P, Tepler I, Wallace R, Leder P. Coexpression of MMTV/v-Ha-ras and MMTV/c-myc genes in transgenic mice: synergistic action of oncogenes. vivo. Cell (1987) 49(4):465–75. doi: 10.1016/0092-8674(87)90449-1
59. Heyer J, Kwong LN, Lowe SW, Chin L. Non-germline genetically engineered mouse models for translational cancer research. Nat Rev Cancer (2010) 10(7):470–80. doi: 10.1038/nrc2877
60. Day CP, Merlino G, Van Dyke T. Preclinical mouse cancer models: a maze of opportunities and challenges. Cell (2015) 163(1):39–53. doi: 10.1016/j.cell.2015.08.068
61. Zitvogel L, Pitt JM, Daillere R, Smyth MJ, Kroemer G. Mouse models in oncoimmunology. Nat Rev Cancer (2016) 16(12):759–73. doi: 10.1038/nrc.2016.91
62. Gargiulo G. Next-Generation in vivo Modeling of Human Cancers. Front Oncol (2018) 8:429. doi: 10.3389/fonc.2018.00429
63. Hidalgo M, Amant F, Biankin AV, Budinska E, Byrne AT, Caldas C, et al. Patient-derived xenograft models: an emerging platform for translational cancer research. Cancer Discovery (2014) 4(9):998–1013. doi: 10.1158/2159-8290.CD-14-0001
64. Okada S, Vaeteewoottacharn K, Kariya R. Application of Highly Immunocompromised Mice for the Establishment of Patient-Derived Xenograft (PDX) Models. Cells (2019). doi: 10.3390/cells8080889
65. Murayama T, Gotoh N. Patient-Derived Xenograft Models of Breast Cancer and Their Application. Cells (2019). doi: 10.3390/cells8060621
66. Namekawa T, Ikeda K, Horie-Inoue K, Inoue S. Application of Prostate Cancer Models for Preclinical Study: Advantages and Limitations of Cell Lines, Patient-Derived Xenografts, and Three- Dimensional Culture of Patient-Derived Cells. Cells (2019). doi: 10.3390/cells8010074
67. Hoffman RM. Patient-derived orthotopic xenografts: better mimic of metastasis than subcutaneous xenografts. Nat Rev Cancer (2015) 15(8):451–2. doi: 10.1038/nrc3972
68. Fazio M, Ablain J, Chuan Y, Langenau DM, Zon LI. Zebrafish patient avatars in cancer biology and precision cancer therapy. Nat Rev Cancer (2020) 20(5):263–73. doi: 10.1038/s41568-020-0252-3
69. Yang HW, Kutok JL, Lee NH, Piao HY, Fletcher CD, Kanki JP, et al. Targeted expression of human MYCN selectively causes pancreatic neuroendocrine tumors in transgenic zebrafish. Cancer Res (2004) 64(20):7256–62. doi: 10.1158/0008-5472.CAN-04-0931
70. Patton EE, Widlund HR, Kutok JL, Kopani KR, Amatruda JF, Murphey RD, et al. BRAF mutations are sufficient to promote nevi formation and cooperate with p53 in the genesis of melanoma. Curr Biol (2005) 15(3):249–54. doi: 10.1016/j.cub.2005.01.031
71. Topczewska JM, Postovit LM, Margaryan NV, Sam A, Hess AR, Wheaton WW, et al. Embryonic and tumorigenic pathways converge via Nodal signaling: role in melanoma aggressiveness. Nat Med (2006) 12(8):925–32. doi: 10.1038/nm1448
72. Ablain J, Xu M, Rothschild H, Jordan RC, Mito JK, Daniels BH, et al. Human tumor genomics and zebrafish modeling identify SPRED1 loss as a driver of mucosal melanoma. Science (2018) 362(6418):1055–60. doi: 10.1126/science.aau6509
73. Yan C, Brunson DC, Tang Q, Do D, Iftimia NA, Moore JC, et al. Visualizing Engrafted Human Cancer and Therapy Responses in Immunodeficient Zebrafish. Cell (2019) 177(7):1903–14 e14. doi: 10.1016/j.cell.2019.04.004
74. Lin J, Zhang W, Zhao JJ, Kwart AH, Yang C, Ma D, et al. A clinically relevant in vivo zebrafish model of human multiple myeloma to study preclinical therapeutic efficacy. Blood (2016) 128(2):249–52. doi: 10.1182/blood-2016-03-704460
75. Miserocchi G, Spadazzi C, Calpona S, De Rosa F, Usai A, De Vita A, et al. Precision Medicine in Head and Neck Cancers: Genomic and Preclinical Approaches. J Pers Med (2022). doi: 10.3390/jpm12060854
76. De Vita A, Recine F, Miserocchi G, Pieri F, Spadazzi C, Cocchi C, et al. The potential role of the extracellular matrix in the activity of trabectedin in UPS and L-sarcoma: evidences from a patient-derived primary culture case series in tridimensional and zebrafish models. J Exp Clin Cancer Res (2021) 40(1):165. doi: 10.1186/s13046-021-01963-1
77. Du X, Liu M, Su J, Zhang P, Tang F, Ye P, et al. Uncoupling therapeutic from immunotherapy-related adverse effects for safer and effective anti-CTLA-4 antibodies in CTLA4 humanized mice. Cell Res (2018) 28(4):433–47. doi: 10.1038/s41422-018-0012-z
78. Mhaidly R, Verhoeyen E. Humanized Mice Are Precious Tools for Preclinical Evaluation of CAR T and CAR NK Cell Therapies. Cancers (Basel) (2020). doi: 10.3390/cancers12071915
79. deCarvalho AC, Kim H, Poisson LM, Winn ME, Mueller C, Cherba D, et al. Discordant inheritance of chromosomal and extrachromosomal DNA elements contributes to dynamic disease evolution in glioblastoma. Nat Genet (2018) 50(5):708–17. doi: 10.1038/s41588-018-0105-0
80. Villafranca-Magdalena B, Masferrer-Ferragutcasas C, Lopez-Gil C, Coll-de la Rubia E, Rebull M, Parra G, et al. Genomic Validation of Endometrial Cancer Patient-Derived Xenograft Models as a Preclinical Tool. Int J Mol Sci (2022). doi: 10.3390/ijms23116266
81. Vanni S, De Vita A, Gurrieri L, Fausti V, Miserocchi G, Spadazzi C, et al. Myxofibrosarcoma landscape: diagnostic pitfalls, clinical management and future perspectives. Ther Adv Med Oncol (2022) 14:17588359221093973. doi: 10.1177/17588359221093973
82. Ben-David U, Ha G, Tseng YY, Greenwald NF, Oh C, Shih J, et al. Patient-derived xenografts undergo mouse-specific tumor evolution. Nat Genet (2017) 49(11):1567–75. doi: 10.1038/ng.3967
83. Shi J, Li Y, Jia R, Fan X. The fidelity of cancer cells in PDX models: Characteristics, mechanism and clinical significance. Int J Cancer (2020) 146(8):2078–88. doi: 10.1002/ijc.32662
84. Kim M, Mun H, Sung CO, Cho EJ, Jeon HJ, Chun SM, et al. Patient-derived lung cancer organoids as in vitro cancer models for therapeutic screening. Nat Commun (2019) 10(1):3991. doi: 10.1038/s41467-019-11867-6
85. Vlachogiannis G, Hedayat S, Vatsiou A, Jamin Y, Fernandez-Mateos J, Khan K, et al. Patient-derived organoids model treatment response of metastatic gastrointestinal cancers. Science (2018) 359(6378):920–26. doi: 10.1126/science.aao2774
86. Pasch CA, Favreau PF, Yueh AE, Babiarz CP, Gillette AA, Sharick JT, et al. Patient-Derived Cancer Organoid Cultures to Predict Sensitivity to Chemotherapy and Radiation. Clin Cancer Res (2019) 25(17):5376–87. doi: 10.1158/1078-0432.CCR-18-3590
87. de Witte CJ, Espejo Valle-Inclan J, Hami N, Lohmussaar K, Kopper O, Vreuls CPH, et al. Patient- Derived Ovarian Cancer Organoids Mimic Clinical Response and Exhibit Heterogeneous Inter- and Intrapatient Drug Responses. Cell Rep (2020) 31(11):107762. doi: 10.1016/j.celrep.2020.107762
88. Saito Y, Muramatsu T, Kanai Y, Ojima H, Sukeda A, Hiraoka N, et al. Establishment of Patient-Derived Organoids and Drug Screening for Biliary Tract Carcinoma. Cell Rep (2019) 27(4):1265–76 e4. doi: 10.1016/j.celrep.2019.03.088
89. Vande Voorde J, Ackermann T, Pfetzer N, Sumpton D, Mackay G, Kalna G, et al. Improving the metabolic fidelity of cancer models with a physiological cell culture medium. Sci Adv (2019). doi: 10.1126/sciadv.aau7314
90. Larsen BM, Kannan M, Langer LF, Leibowitz BD, Bentaieb A, Cancino A, et al. A pan-cancer organoid platform for precision medicine. Cell Rep (2021) 36(4):109429. doi: 10.1016/j.celrep.2021.109429
91. Peng D, Gleyzer R, Tai WH, Kumar P, Bian Q, Isaacs B, et al. Evaluating the transcriptional fidelity of cancer models. Genome Med (2021) 13(1):73. doi: 10.1186/s13073-021-00888-w
92. Conte N, Mason JC, Halmagyi C, Neuhauser S, Mosaku A, Yordanova G, et al. PDX Finder: A portal for patient-derived tumor xenograft model discovery. Nucleic Acids Res (2019). doi: 10.1093/nar/gky984
93. Dudova Z, Conte N, Mason J, Stuchlik D, Pesa R, Halmagyi C, et al. The EurOPDX Data Portal: an open platform for patient-derived cancer xenograft data sharing and visualization. BMC Genomics (2022) 23(1):156. doi: 10.1186/s12864-022-08367-1
94. Adam G, Rampasek L, Safikhani Z, Smirnov P, Haibe-Kains B, Goldenberg A. Machine learning approaches to drug response prediction: challenges and recent progress. NPJ Precis Oncol (2020) 4:19. doi: 10.1038/s41698-020-0122-1
95. Mourragui SMC, Loog M, Vis DJ, Moore K, Manjon AG, van de Wiel MA, et al. Predicting patient response with models trained on cell lines and patient-derived xenografts by nonlinear transfer learning. Proc Natl Acad Sci U.S.A. (2021). doi: 10.1073/pnas.2106682118
96. Nguyen LC, Naulaerts S, Bruna A, Ghislat G, Ballester PJ. Predicting Cancer Drug Response In Vivo by Learning an Optimal Feature Selection of Tumour Molecular Profiles. Biomedicines (2021) 9(10). doi: 10.3390/biomedicines9101319
97. Gohil SH, Iorgulescu JB, Braun DA, Keskin DB, Livak KJ. Applying high-dimensional single-cell technologies to the analysis of cancer immunotherapy. Nat Rev Clin Oncol (2021) 18(4):244–56. doi: 10.1038/s41571-020-00449-x
98. Touat M, Li YY, Boynton AN, Spurr LF, Iorgulescu JB, Bohrson CL, et al. Mechanisms and therapeutic implications of hypermutation in gliomas. Nature (2020) 580(7804):517–23. doi: 10.1038/s41586-020-2209-9
99. Cristobal A, van den Toorn HWP, van de Wetering M, Clevers H, Heck AJR, Mohammed S. Personalized Proteome Profiles of Healthy and Tumor Human Colon Organoids Reveal Both Individual Diversity and Basic Features of Colorectal Cancer. Cell Rep (2017) 18(1):263–74. doi: 10.1016/j.celrep.2016.12.016
100. Li H, Dai W, Xia X, Wang R, Zhao J, Han L, et al. Modeling tumor development and metastasis using paired organoids derived from patients with colorectal cancer liver metastases. J Hematol Oncol (2020) 13(1):119. doi: 10.1186/s13045-020-00957-4
101. Allaway RJ, Fischer DA, de Abreu FB, Gardner TB, Gordon SR, Barth RJ, et al. Genomic characterization of patient-derived xenograft models established from fine needle aspirate biopsies of a primary pancreatic ductal adenocarcinoma and from patient-matched metastatic sites. Oncotarget (2016) 7(13):17087–102. doi: 10.18632/oncotarget.7718
102. Zhang Z, Shiratsuchi H, Lin J, Chen G, Reddy RM, Azizi E, et al. Expansion of CTCs from early stage lung cancer patients using a microfluidic co-culture model. Oncotarget (2014) 5(23):12383–97. doi: 10.18632/oncotarget.2592
103. Mout L, van Dessel LF, Kraan J, de Jong AC, Neves RPL, Erkens-Schulze S, et al. Generating human prostate cancer organoids from leukapheresis enriched circulating tumour cells. Eur J Cancer (2021) 150:179–89. doi: 10.1016/j.ejca.2021.03.023
104. Hodgkinson CL, Morrow CJ, Li Y, Metcalf RL, Rothwell DG, Trapani F, et al. Tumorigenicity and genetic profiling of circulating tumor cells in small-cell lung cancer. Nat Med (2014) 20(8):897–903. doi: 10.1038/nm.3600
105. Pantel K, Speicher MR. The biology of circulating tumor cells. Oncogene (2016) 35(10):1216–24. doi: 10.1038/onc.2015.192
106. Li X, Larsson P, Ljuslinder I, Ohlund D, Myte R, Lofgren-Burstrom A, et al. Ex Vivo Organoid Cultures Reveal the Importance of the Tumor Microenvironment for Maintenance of Colorectal Cancer Stem Cells. Cancers (Basel) (2020). doi: 10.3390/cancers12040923
107. Lamichhane SP, Arya N, Kohler E, Xiang S, Christensen J, Shastri VP. Recapitulating epithelial tumor microenvironment in vitro using three dimensional tri-culture of human epithelial, endothelial, and mesenchymal cells. BMC Cancer (2016) 16:581. doi: 10.1186/s12885-016-2634-1
108. Gronholm M, Feodoroff M, Antignani G, Martins B, Hamdan F, Cerullo V. Patient-derived organoids for precision cancer immunotherapy. Cancer Res (2021). doi: 10.1158/0008-5472.CAN-20-4026
109. Hirt C, Papadimitropoulos A, Mele V, Muraro MG, Mengus C, Iezzi G, et al. "In vitro" 3D models of tumor-immune system interaction. Adv Drug Delivery Rev (2014) 79-80:145–54. doi: 10.1016/j.addr.2014.05.003
110. Feder-Mengus C, Ghosh S, Reschner A, Martin I, Spagnoli GC. New dimensions in tumor immunology: what does 3D culture reveal? Trends Mol Med (2008) 14(8):333–40. doi: 10.1016/j.molmed.2008.06.001
111. Courau T, Bonnereau J, Chicoteau J, Bottois H, Remark R, Assante Miranda L, et al. Cocultures of human colorectal tumor spheroids with immune cells reveal the therapeutic potential of MICA/B and NKG2A targeting for cancer treatment. J Immunother Cancer (2019) 7(1):74. doi: 10.1186/s40425-019-0553-9
112. Dijkstra KK, Cattaneo CM, Weeber F, Chalabi M, van de Haar J, Fanchi LF, et al. Generation of Tumor-Reactive T Cells by Co-culture of Peripheral Blood Lymphocytes and Tumor Organoids. Cell (2018) 174(6):1586–98 e12. doi: 10.1016/j.cell.2018.07.009
113. Tsai S, McOlash L, Palen K, Johnson B, Duris C, Yang Q, et al. Development of primary human pancreatic cancer organoids, matched stromal and immune cells and 3D tumor microenvironment models. BMC Cancer (2018) 18(1):335. doi: 10.1186/s12885-018-4238-4
114. Walker C, Mojares E, Del Rio Hernandez A. Role of Extracellular Matrix in Development and Cancer Progression. Int J Mol Sci (2018). doi: 10.3390/ijms19103028
115. Cox TR. The matrix in cancer. Nat Rev Cancer (2021) 21(4):217–38. doi: 10.1038/s41568-020-00329-7
116. Li D, Nie W, Chen L, McCoul D, Liu D, Zhang X, et al. Self-Assembled Hydroxyapatite-Graphene Scaffold for Photothermal Cancer Therapy and Bone Regeneration. J BioMed Nanotechnol (2018) 14(12):2003–17. doi: 10.1166/jbn.2018.2646
117. Habanjar O, Diab-Assaf M, Caldefie-Chezet F, Delort L. 3D Cell Culture Systems: Tumor Application, Advantages, and Disadvantages. Int J Mol Sci (2021) 22(22). doi: 10.3390/ijms222212200
118. Le MN, Xu K, Wang Z, Beverung S, Steward RL, Florczyk SJ. Evaluation of the effect of 3D porous Chitosan-alginate scaffold stiffness on breast cancer proliferation and migration. J BioMed Mater Res A (2021) 109(10):1990–2000. doi: 10.1002/jbm.a.37191
119. Tan ML, Shao P, Friedhuber AM, van Moorst M, Elahy M, Indumathy S, et al. The potential role of free chitosan in bone trauma and bone cancer management. Biomaterials (2014) 35(27):7828–38. doi: 10.1016/j.biomaterials.2014.05.087
120. Shi X, Cheng Y, Wang J, Chen H, Wang X, Li X, et al. 3D printed intelligent scaffold prevents recurrence and distal metastasis of breast cancer. Theranostics (2020) 10(23):10652–64. doi: 10.7150/thno.47933
121. Hamdi DH, Barbieri S, Chevalier F, Groetz JE, Legendre F, Demoor M, et al. In vitro engineering of human 3D chondrosarcoma: a preclinical model relevant for investigations of radiation quality impact. BMC Cancer (2015) 15:579. doi: 10.1186/s12885-015-1590-5
122. Unnikrishnan K, Thomas LV, Ram Kumar RM. Advancement of Scaffold-Based 3D Cellular Models in Cancer Tissue Engineering: An Update. Front Oncol (2021) 11:733652. doi: 10.3389/fonc.2021.733652
123. Neal JT, Li X, Zhu J, Giangarra V, Grzeskowiak CL, Ju J, et al. Organoid Modeling of the Tumor Immune Microenvironment. Cell (2018) 175(7):1972–88 e16. doi: 10.1016/j.cell.2018.11.021
124. Zumwalde NA, Haag JD, Sharma D, Mirrielees JA, Wilke LG, Gould MN, et al. Analysis of Immune Cells from Human Mammary Ductal Epithelial Organoids Reveals Vdelta2+ T Cells That Efficiently Target Breast Carcinoma Cells in the Presence of Bisphosphonate. Cancer Prev Res (Phila) (2016) 9(4):305–16. doi: 10.1158/1940-6207.CAPR-15-0370-T
125. Li X, Nadauld L, Ootani A, Corney DC, Pai RK, Gevaert O, et al. Oncogenic transformation of diverse gastrointestinal tissues in primary organoid culture. Nat Med (2014) 20(7):769–77. doi: 10.1038/nm.3585
126. Wakamatsu T, Ogawa H, Yoshida K, Matsuoka Y, Shizuma K, Imura Y, et al. Establishment of Organoids From Human Epithelioid Sarcoma With the Air-Liquid Interface Organoid Cultures. Front Oncol (2022) 12:893592. doi: 10.3389/fonc.2022.893592
127. Ootani A, Li X, Sangiorgi E, Ho QT, Ueno H, Toda S, et al. Sustained in vitro intestinal epithelial culture within a Wnt-dependent stem cell niche. Nat Med (2009) 15(6):701–6. doi: 10.1038/nm.1951
128. Jacob F, Salinas RD, Zhang DY, Nguyen PTT, Schnoll JG, Wong SZH, et al. A Patient-Derived Glioblastoma Organoid Model and Biobank Recapitulates Inter- and Intra-tumoral Heterogeneity. Cell (2020) 180(1):188–204 e22. doi: 10.1016/j.cell.2019.11.036
129. Park SE, Georgescu A, Huh D. Organoids-on-a-chip. Science (2019) 364(6444):960–65. doi: 10.1126/science.aaw7894
130. Shirure VS, Bi Y, Curtis MB, Lezia A, Goedegebuure MM, Goedegebuure SP, et al. Tumor-on-a-chip platform to investigate progression and drug sensitivity in cell lines and patient-derived organoids. Lab Chip (2018) 18(23):3687–702. doi: 10.1039/c8lc00596f
131. Dornhof J, Kieninger J, Muralidharan H, Maurer J, Urban GA, Weltin A. Microfluidic organ-on-chip system for multi-analyte monitoring of metabolites in 3D cell cultures. Lab Chip (2022) 22(2):225–39. doi: 10.1039/d1lc00689d
132. Paolillo M, Comincini S, Schinelli S. In Vitro Glioblastoma Models: A Journey into the Third Dimension. Cancers (Basel) (2021). doi: 10.3390/cancers13102449
133. Augustine R, Kalva SN, Ahmad R, Zahid AA, Hasan S, Nayeem A, et al. 3D Bioprinted cancer models: Revolutionizing personalized cancer therapy. Transl Oncol (2021) 14(4):101015. doi: 10.1016/j.tranon.2021.101015
134. Gong Z, Huang L, Tang X, Chen K, Wu Z, Zhang L, et al. Acoustic Droplet Printing Tumor Organoids for Modeling Bladder Tumor Immune Microenvironment within a Week. Adv Healthc Mater (2021) 10(22):e2101312. doi: 10.1002/adhm.202101312
135. Quinn CH, Beierle AM, Hutchins SC, Marayati R, Bownes LV, Stewart JE, et al. Targeting High-Risk Neuroblastoma Patient-Derived Xenografts with Oncolytic Virotherapy. Cancers (Basel) (2022). doi: 10.3390/cancers14030762
136. Wu D, Wang Z, Li J, Song Y, Perez MEM, Wang Z, et al. A 3D-Bioprinted Multiple Myeloma Model. Adv Healthc Mater 2021:e2100884. doi: 10.1002/adhm.202100884
137. Jeong YM, Bang C, Park M, Shin S, Yun S, Kim CM, et al. 3D-Printed Collagen Scaffolds Promote Maintenance of Cryopreserved Patients-Derived Melanoma Explants. Cells (2021). doi: 10.3390/cells10030589
138. Tang M, Tiwari SK, Agrawal K, Tan M, Dang J, Tam T, et al. Rapid 3D Bioprinting of Glioblastoma Model Mimicking Native Biophysical Heterogeneity. Small (2021) 17(15):e2006050. doi: 10.1002/smll.202006050
139. Xie F, Sun L, Pang Y, Xu G, Jin B, Xu H, et al. Three-dimensional bio-printing of primary human hepatocellular carcinoma for personalized medicine. Biomaterials (2021) 265:120416. doi: 10.1016/j.biomaterials.2020.120416
140. Chadwick M, Yang C, Liu L, Gamboa CM, Jara K, Lee H, et al. Rapid Processing and Drug Evaluation in Glioblastoma Patient-Derived Organoid Models with 4D Bioprinted Arrays. iScience (2020) 23(8):101365. doi: 10.1016/j.isci.2020.101365
141. Mao S, He J, Zhao Y, Liu T, Xie F, Yang H, et al. Bioprinting of patient-derived in vitro intrahepatic cholangiocarcinoma tumor model: establishment, evaluation and anti-cancer drug testing. Biofabrication (2020) 12(4):045014. doi: 10.1088/1758-5090/aba0c3
142. Ireson CR, Alavijeh MS, Palmer AM, Fowler ER, Jones HJ. The role of mouse tumour models in the discovery and development of anticancer drugs. Br J Cancer (2019) 121(2):101–08. doi: 10.1038/s41416-019-0495-5
143. Pajot A, Michel ML, Fazilleau N, Pancre V, Auriault C, Ojcius DM, et al. A mouse model of human adaptive immune functions: HLA-A2.1-/HLA-DR1-transgenic H-2 class I-/class II-knockout mice. Eur J Immunol (2004) 34(11):3060–9. doi: 10.1002/eji.200425463
144. DeAngelis N, Ferrante C, Powers G, Sendecki J, Mattson B, Pizutti D, et al. Discovery and pharmacological characterization of cetrelimab (JNJ-63723283), an anti-programmed cell death protein-1 (PD-1) antibody, in human cancer models. Cancer Chemother Pharmacol (2022) 89(4):515–27. doi: 10.1007/s00280-022-04415-5
145. Jiang H, Ni H, Zhang P, Guo X, Wu M, Shen H, et al. PD-L1/LAG-3 bispecific antibody enhances tumor-specific immunity. Oncoimmunology (2021) 10(1):1943180. doi: 10.1080/2162402X.2021.1943180
146. Herndler-Brandstetter D, Shan L, Yao Y, Stecher C, Plajer V, Lietzenmayer M, et al. Humanized mouse model supports development, function, and tissue residency of human natural killer cells. Proc Natl Acad Sci U.S.A. (2017) 114(45):E9626–E34. doi: 10.1073/pnas.1705301114
147. Olson B, Li Y, Lin Y, Liu ET, Patnaik A. Mouse Models for Cancer Immunotherapy Research. Cancer Discovery (2018) 8(11):1358–65. doi: 10.1158/2159-8290.CD-18-0044
148. Jespersen H, Lindberg MF, Donia M, Soderberg EMV, Andersen R, Keller U, et al. Clinical responses to adoptive T-cell transfer can be modeled in an autologous immune-humanized mouse model. Nat Commun (2017) 8(1):707. doi: 10.1038/s41467-017-00786-z
149. Sanmamed MF, Nie X, Desai SS, Villaroel-Espindola F, Badri T, Zhao D, et al. . doi: 10.1158/2159-8290.CD-20-0962
150. Yin Q, Yu W, Grzeskowiak CL, Li J, Huang H, Guo J, et al. Nanoparticle-enabled innate immune stimulation activates endogenous tumor-infiltrating T cells with broad antigen specificities. Proc Natl Acad Sci U.S.A. (2021). doi: 10.1073/pnas.2016168118
151. Elinav E, Garrett WS, Trinchieri G, Wargo J. The cancer microbiome. Nat Rev Cancer (2019) 19(7):371–76. doi: 10.1038/s41568-019-0155-3
152. Sivan A, Corrales L, Hubert N, Williams JB, Aquino-Michaels K, Earley ZM, et al. Commensal Bifidobacterium promotes antitumor immunity and facilitates anti-PD-L1 efficacy. Science (2015) 350(6264):1084–9. doi: 10.1126/science.aac4255
153. Mager LF, Burkhard R, Pett N, Cooke NCA, Brown K, Ramay H, et al. Microbiome-derived inosine modulates response to checkpoint inhibitor immunotherapy. Science (2020) 369(6510):1481–89. doi: 10.1126/science.abc3421
154. Fluckiger A, Daillere R, Sassi M, Sixt BS, Liu P, Loos F, et al. Cross-reactivity between tumor MHC class I-restricted antigens and an enterococcal bacteriophage. Science (2020) 369(6506):936–42. doi: 10.1126/science.aax0701
156. Kadosh E, Snir-Alkalay I, Venkatachalam A, May S, Lasry A, Elyada E, et al. The gut microbiome switches mutant p53 from tumour-suppressive to oncogenic. Nature (2020) 586(7827):133–38. doi: 10.1038/s41586-020-2541-0
157. Li Q, Hu W, Liu WX, Zhao LY, Huang D, Liu XD, et al. Streptococcus thermophilus Inhibits Colorectal Tumorigenesis Through Secreting beta-Galactosidase. Gastroenterology (2021) 160(4):1179–93 e14. doi: 10.1053/j.gastro.2020.09.003
158. Soldati L, Di Renzo L, Jirillo E, Ascierto PA, Marincola FM, De Lorenzo A. The influence of diet on anti-cancer immune responsiveness. J Transl Med (2018) 16(1):75. doi: 10.1186/s12967-018-1448-0
159. Zhao S, Peralta RM, Avina-Ochoa N, Delgoffe GM, Kaech SM. Metabolic regulation of T cells in the tumor microenvironment by nutrient availability and diet. Semin Immunol (2021) 52:101485. doi: 10.1016/j.smim.2021.101485
160. Spencer CN, McQuade JL, Gopalakrishnan V, McCulloch JA, Vetizou M, Cogdill AP, et al. Dietary fiber and probiotics influence the gut microbiome and melanoma immunotherapy response. Science (2021) 374(6575):1632–40. doi: 10.1126/science.aaz7015
161. Singh RP, Bashir H, Kumar R. Emerging role of microbiota in immunomodulation and cancer immunotherapy. Semin Cancer Biol (2021) 70:37–52. doi: 10.1016/j.semcancer.2020.06.008
162. Routy B, Le Chatelier E, Derosa L, Duong CPM, Alou MT, Daillere R, et al. Gut microbiome influences efficacy of PD-1-based immunotherapy against epithelial tumors. Science (2018) 359(6371):91–7. doi: 10.1126/science.aan3706
163. Shi L, Sheng J, Wang M, Luo H, Zhu J, Zhang B, et al. Combination Therapy of TGF-beta Blockade and Commensal-derived Probiotics Provides Enhanced Antitumor Immune Response and Tumor Suppression. Theranostics (2019) 9(14):4115–29. doi: 10.7150/thno.35131
164. Baruch EN, Youngster I, Ben-Betzalel G, Ortenberg R, Lahat A, Katz L, et al. Fecal microbiota transplant promotes response in immunotherapy-refractory melanoma patients. Science (2021) 371(6529):602–09. doi: 10.1126/science.abb5920
165. Davar D, Dzutsev AK, McCulloch JA, Rodrigues RR, Chauvin JM, Morrison RM, et al. Fecal microbiota transplant overcomes resistance to anti-PD-1 therapy in melanoma patients. Science (2021) 371(6529):595–602. doi: 10.1126/science.abf3363
166. Clohessy JG, Pandolfi PP. Mouse hospital and co-clinical trial project–from bench to bedside. Nat Rev Clin Oncol (2015) 12(8):491–8. doi: 10.1038/nrclinonc.2015.62
167. Kim HR, Kang HN, Shim HS, Kim EY, Kim J, Kim DJ, et al. Co-clinical trials demonstrate predictive biomarkers for dovitinib, an FGFR inhibitor, in lung squamous cell carcinoma. Ann Oncol (2017) 28(6):1250–59. doi: 10.1093/annonc/mdx098
168. Recondo G, Mahjoubi L, Maillard A, Loriot Y, Bigot L, Facchinetti F, et al. Feasibility and first reports of the MATCH-R repeated biopsy trial at Gustave Roussy. NPJ Precis Oncol (2020) 4:27. doi: 10.1038/s41698-020-00130-7
169. Wensink GE, Elias SG, Mullenders J, Koopman M, Boj SF, Kranenburg OW, et al. Patient-derived organoids as a predictive biomarker for treatment response in cancer patients. NPJ Precis Oncol (2021) 5(1):30. doi: 10.1038/s41698-021-00168-1
170. Yao Y, Xu X, Yang L, Zhu J, Wan J, Shen L, et al. Patient-Derived Organoids Predict Chemoradiation Responses of Locally Advanced Rectal Cancer. Cell Stem Cell (2020) 26(1):17–26 e6. doi: 10.1016/j.stem.2019.10.010
171. Park M, Kwon J, Kong J, Moon SM, Cho S, Yang KY, et al. A Patient-Derived Organoid-Based Radiosensitivity Model for the Prediction of Radiation Responses in Patients with Rectal Cancer. Cancers (Basel) (2021). doi: 10.3390/cancers13153760
172. Ganesh K, Wu C, O'Rourke KP, Szeglin BC, Zheng Y, Sauve CG, et al. A rectal cancer organoid platform to study individual responses to chemoradiation. Nat Med (2019) 25(10):1607–14. doi: 10.1038/s41591-019-0584-2
173. Narasimhan V, Wright JA, Churchill M, Wang T, Rosati R, Lannagan TRM, et al. Medium-throughput Drug Screening of Patient-derived Organoids from Colorectal Peritoneal Metastases to Direct Personalized Therapy. Clin Cancer Res (2020) 26(14):3662–70. doi: 10.1158/1078-0432.CCR-20-0073
174. Hu Y, Sui X, Song F, Li Y, Li K, Chen Z, et al. Lung cancer organoids analyzed on microwell arrays predict drug responses of patients within a week. Nat Commun (2021) 12(1):2581. doi: 10.1038/s41467-021-22676-1
175. Wood LD, Ewald AJ. Organoids in cancer research: a review for pathologist-scientists. J Pathol (2021) 254(4):395–404. doi: 10.1002/path.5684
176. Chen H, Du L, Li J, Wu Z, Gong Z, Xia Y, et al. Modeling cancer metastasis using acoustically bio- printed patient-derived 3D tumor microtissues. J Mater Chem B (2022). doi: 10.1039/d1tb02789a
177. Spiller ER, Ung N, Kim S, Patsch K, Lau R, Strelez C, et al. Imaging-Based Machine Learning Analysis of Patient-Derived Tumor Organoid Drug Response. Front Oncol (2021) 11:771173. doi: 10.3389/fonc.2021.771173
178. Khan S, Shin JH, Ferri V, Cheng N, Noel JE, Kuo C, et al. High-resolution positron emission microscopy of patient-derived tumor organoids. Nat Commun (2021) 12(1):5883. doi: 10.1038/s41467-021-26081-6
179. Liu Z, Zhang P, Ji H, Long Y, Jing B, Wan L, et al. A mini-panel PET scanner-based microfluidic radiobioassay system allowing high-throughput imaging of real-time cellular pharmacokinetics. Lab Chip (2020) 20(6):1110–23. doi: 10.1039/c9lc01066a
180. Echeverria GV, Ge Z, Seth S, Zhang X, Jeter-Jones S, Zhou X, et al. Resistance to neoadjuvant chemotherapy in triple-negative breast cancer mediated by a reversible drug-tolerant state. Sci Transl Med (2019) 11(488). doi: 10.1126/scitranslmed.aav0936
181. Kim HR, Kang HN, Yun MR, Ju KY, Choi JW, Jung DM, et al. Mouse-human co-clinical trials demonstrate superior anti-tumour effects of buparlisib (BKM120) and cetuximab combination in squamous cell carcinoma of head and neck. Br J Cancer (2020) 123(12):1720–29. doi: 10.1038/s41416-020-01074-2
Keywords: patient-derived model, PDO, drug-testing, co-clinical trial, PDX, cancer
Citation: Genta S, Coburn B, Cescon DW and Spreafico A (2022) Patient-derived cancer models: Valuable platforms for anticancer drug testing. Front. Oncol. 12:976065. doi: 10.3389/fonc.2022.976065
Received: 23 June 2022; Accepted: 12 July 2022;
Published: 12 August 2022.
Edited by:
Sara Lovisa, Humanitas University, ItalyReviewed by:
Julienne Leigh Carstens, University of Alabama at Birmingham, United StatesAlessandro De Vita, Scientific Institute of Romagna for the Study and Treatment of Tumors (IRCCS), Italy
Copyright © 2022 Genta, Coburn, Cescon and Spreafico. This is an open-access article distributed under the terms of the Creative Commons Attribution License (CC BY). The use, distribution or reproduction in other forums is permitted, provided the original author(s) and the copyright owner(s) are credited and that the original publication in this journal is cited, in accordance with accepted academic practice. No use, distribution or reproduction is permitted which does not comply with these terms.
*Correspondence: Anna Spreafico, anna.spreafico@uhn.ca