- 1Department of Preventive Medicine, Institute of Biomedical Informatics, Cell Signal Transduction Laboratory, Bioinformatics Center, Henan Provincial Engineering Center for Tumor Molecular Medicine, School of Software, School of Basic Medical Sciences, Henan University, Kaifeng, China
- 2Department of Thoracic Surgery, The Affiliated Nanshi Hospital of Henan University, Nanyang, China
Evaluating the prognostic value of genes of interest in different populations of gastric cancer (GC) is difficult and time-consuming for basic and translational researchers even though many datasets are available in public dataset depositories. In the current study, we developed a robust web-based portal called OSgc (Online consensus Survival analysis of gastric cancer) that enables easy and swift verification of known and novel biomarker candidates in GC. OSgc is composed of gene expression profiling data and clinical follow-up information of 1,824 clinical GC cases, which are collected from 7 public independent datasets derived from Gene Expression Omnibus (GEO) and The Cancer Genome Atlas (TCGA). By OSgc, users input the official gene symbol and will promptly retrieve the Kaplan–Meier survival plot with hazard ratio (HR) and log rank p value on the output webpage, by which users could assess the prognostic value of interesting genes for GC patients. Five survival end points containing overall survival, progression-free survival, progression-free interval, relapse-free survival, and disease-free survival could be measured in OSgc. OSgc can greatly help cancer biologists and clinicians to explore the effect of gene expression on patient survival. OSgc is freely available without restrictions at http://bioinfo.henu.edu.cn/GC/GCList.jsp.
Introduction
Gastric cancer (GC) is the fourth leading factor of cancer mortality in the world. In 2020, GC occurred in 1,089,103 people and resulted in 768,793 deaths. Although there are many advances in treatment of GC, patients have poor prognosis and the 5-year survival rate is just 5%–20%. Prognostic biomarkers can assist clinicians in assessing the risk of clinical outcomes including cancer recurrence or disease progression in the future (1, 2). Molecular characteristics such as gene expression and somatic mutations have been reported to represent the primary source of prognostic biomarker (3, 4). A recent study showed that high SETD2 (SET domain-containing protein 2, also known as HYPB) expression was correlated with better prognosis for GC patients, and its overexpression in GC cell lines significantly inhibits cell proliferation, migration, and invasion (5). Moreover, lower MTBP (MDM2-binding protein) expression and HOXA5 (homeobox A5) expression were significantly associated with longer overall survival time in GC (6). However, these present retrospective cohort studies were limited to relatively small case series and further validation is required when these findings are going to be translated.
The expression profiling of gastric cancer has been performed using high-throughput technology such as microarrays and RNA sequencing. These data have been used to measure the association of mRNAs to clinical outcomes in GC patients, while a key step for biomarker development is the biomarker validation in multiple independent cohorts. Even though massive public datasets are available, multistep specialized analyses such as exploring repositories and acquiring and processing data make it difficult for most researchers. Previous studies have reported several good online prognosis tools including PROGgene (7), PRECOG (8), OncoLnc (9), GEPIA (10), KM plotter (11, 12), and ITTACA (13), which are available to explore expression changes of individual genes and their association with GC patients’ survival. However, these above tools are restricted regarding low number of clinical cases, lacking most updated data, or login/registering, or limited survival terms.
Hence, to aid and facilitate the evaluation and verification of prognostic biomarkers in independent cohorts, we developed OSgc, a free and easy-to-use web portal to perform the survival analysis in GC. OSgc is composed of 7 public datasets with available follow-up data for 1,824 GC cases from TCGA and GEO databases, and by which survival analysis can be completed in minutes.
Material and Methods
Data Collection
Data were collected by searching for keywords related to gastric cancer, clinical outcome, mRNA profiles, and ≥20 samples. The searches were performed in GEO (http://www.ncbi.nlm.nih.gov/geo) and TCGA (https://tcga-data.nci.nih.gov/tcga). TCGA data are level 3 RNA-Seq data.
Web Implementation
The OSgc web portal was set up as we previously developed with minor modification (14, 15). In brief, OSgc contains two main components: storage and data analysis (Figure 1). A Java implementation was used to realize OSgc. OSgc used the SQL Server database to provide the storage and management of the gene expression profiles and clinical data for GC and used the Browser/Server architecture network management system to manage the database. R packages including “survminer” and “ggplot2” were used to plot the Kaplan–Meier curves.
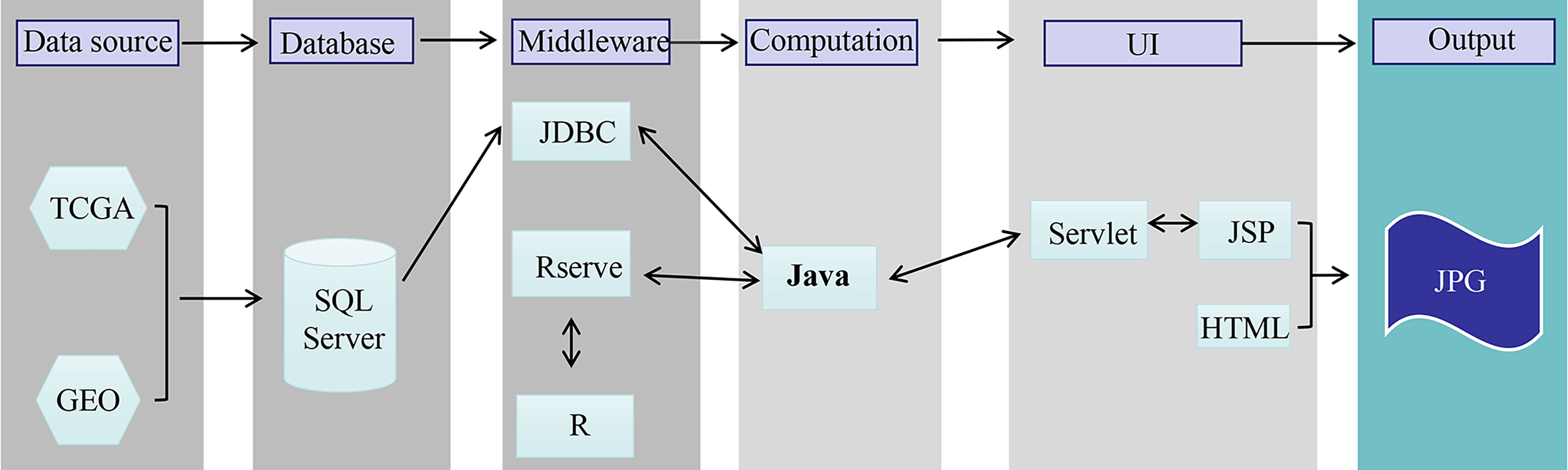
Figure 1 The flowchart of OSgc establishment. GEO, Gene Expression Omnibus; TCGA, The Cancer Genome Atlas.
Searching Previously Reported Prognostic Biomarkers
A PubMed search was performed to find gastric cancer’s prognostic biomarkers using keywords “gastric cancer,” “stomach cancer,” “adenocarcinoma,” “GC,” “survival,” and “gene expression.” Only studies published in English were encompassed. Eligibility criteria also contained the investigation of the biomarker in >30 patients while biomarkers described only in experimental models or meta-analysis or bioinformatics were excluded.
Statistical Analysis
The relationship between clinic-pathological factors and clinical survival outcomes was analyzed by GraphPad Prism 8. R package “survival” was used to perform Cox regression analysis to calculate hazard ratios (HR) and 95% confidence intervals (95% CIs). HR with their corresponding 95% CIs was assessed to explore the prognostic significance of gene of interest in gastric cancer. If a HR is >1 and the 95% CI did not cross 1, the typed gene will show a worse prognosis in the high gene expression group. If a HR is <1 and the 95% CI did not cross 1, it suggests a better prognosis of typed gene in the high gene expression group. Prognostic value was assessed by KM plot analysis and log-rank test. p value < 0.05 is regarded as statistically significant.
Results
Clinical Information of GC Datasets Used in OSgc
To our knowledge, OSgc provides the largest compilation of expression profiling datasets related to clinical outcomes, comprising 7 datasets and 1,824 clinical GC cases. The median age of these patients is 67 years, and the ratio of male to female is 2.2:1. 1,392 patients have OS, 776 patients have DFS, 420 patients have PFS, and 337 patients have RFS. A summary of above GC cohorts is shown in Table 1.
To explore the relationship between clinical characteristics and outcomes, we performed Kaplan–Meier plots for GC patients stratified by TNM stage and gender in datasets used for OSgc (Figure 2). In these patients, TNM stage was significantly related with OS (p < 0.0001) and DFS (p < 0.0001), as we knew (22). Nevertheless, gender showed no significant association with OS (p = 0.4939) and DFS (p = 0.7764).
Web Interface
As shown in Figure 3, OSgc could be easily used by typing only the gene symbol and selecting the individual/combined cohort, stage, gender, grade, and age on the input webpage. After then, “Kaplan–Meier plot” is clicked, and the survival outcome plots will be shown up on the output webpage in less than a minute (might vary if advanced plots are selected). Furthermore, users can easily obtain KM plots for multiple genes (with maximum 5 genes).
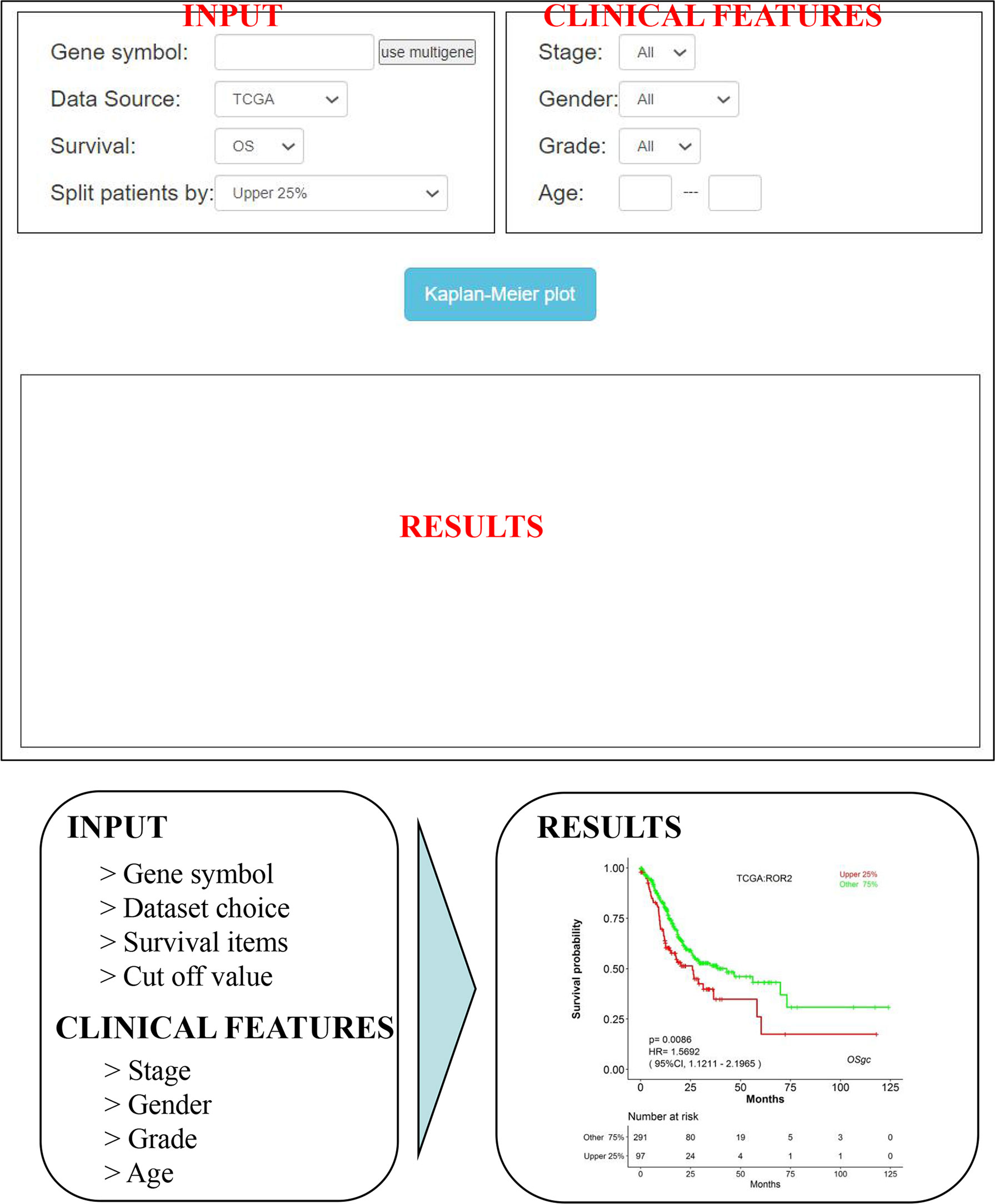
Figure 3 An overview of OSgc application. OSgc application mainly contains three sections including input, clinical features, and results.
Validation and Application
To assess the capabilities of OSgc in the evaluation of prognostic biomarkers, we collected 20 previously published GC prognostic biomarkers through PubMed search (shown in Table 2). These published prognostic biomarkers include 16 unfavorable and 4 favorable prognostic biomarkers which have been verified by tissue-based immunohistochemistry (IHC), Western blot, and RT-PCR in literatures. To test these published prognostic biomarkers in OSgc, OS or DFS was selected as survival term in the combined datasets in OSgc. Combined datasets mean that each cohort was divided separately into strata by selecting the proper cutoff value, which are then put together for survival analysis. The hazard ratio and p value in original studies and OSgc are given in Table 2. The test results showed that the prognostic values of 17 genes line up with previous reports, while 3 genes did not reach significance in OSgc.
Comprehensive Analysis of the Prognosis Significance of E2Fs by OSgc
Increasing evidence has indicated that E2Fs, a family of critical transcription factors that regulate cell cycle progression and other cellular processes, are aberrantly expressed and involved in the tumor progression in various malignant tumors (41, 42). Recently, four research groups had systematically studied the expression patterns and prognostic values of eight E2Fs in patients with breast cancer (BC) (43), ovarian cancer (OC) (44), hepatocellular carcinoma (HCC) (45), and lung carcinoma (46) by investigating a series of databases. For example, Huang et al. had shown that high expression of individual E2Fs was related with poor prognosis in HCC patients (45). However, the expression and prognostic significance of each E2F in gastric cancer have not yet been elucidated. Herein, we can easily explore the prognostic significance for all E2F members in GC by using web tool OSgc. The results showed that the higher transcriptional levels of both E2F2 and E2F8 were associated with better overall survival in gastric cancer patients (Figure 4). However, other E2Fs members were not significantly correlated with OS of GC patients (data not shown).
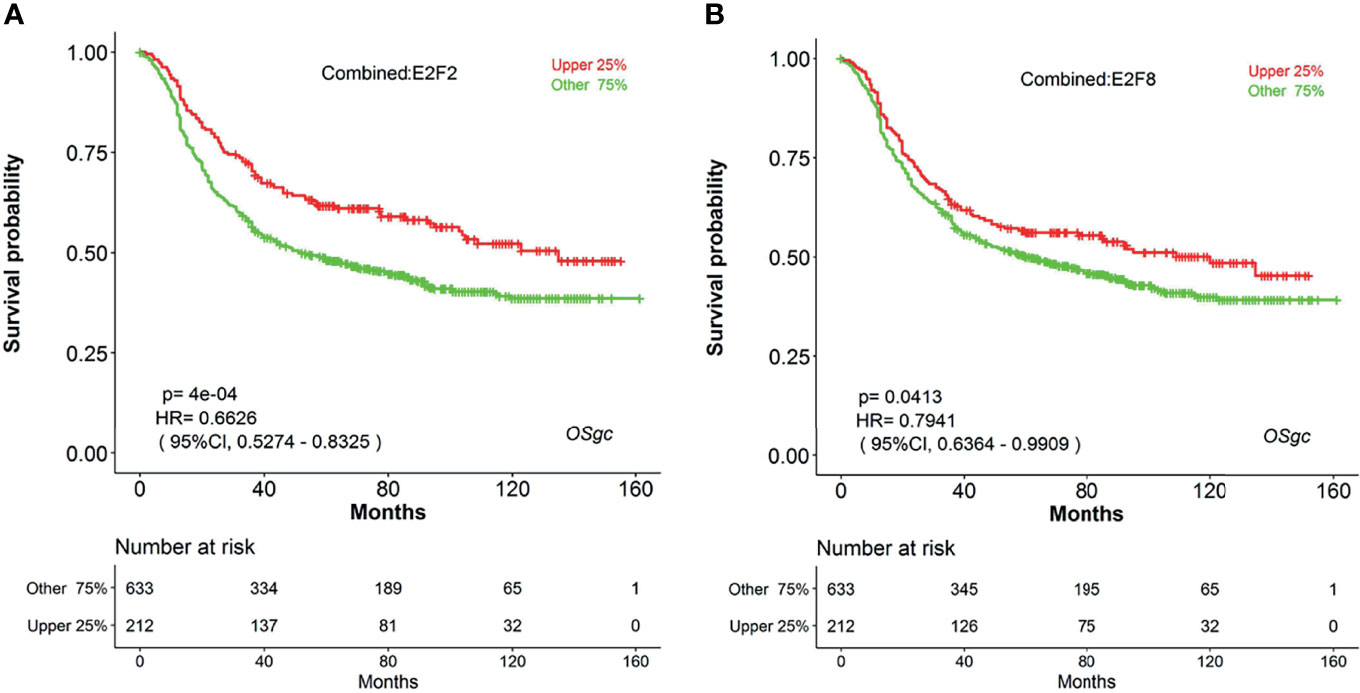
Figure 4 The prognostic value of E2F2 and E2F8 in GC patients (OS in OSgc). (A) KM survival plot for E2F2 suggests that its high expression (red) indicates favorable prognosis (GC patients were separated by the quarter of gene expression level); (B) KM survival plot for E2F8 suggests that its high expression (red) indicates favorable prognosis (GC patients were separated by the quarter of gene expression level); E2F2, E2F transcription factor 2; E2F8, E2F transcription factor 8; GC, gastric cancer; OS, overall survival.
Discussion
Prognostic biomarkers are an important supplement to traditional clinical and histopathological features, for example, tumor size and lymph node metastasis, which cannot completely predict the prognosis of patients with cancer. The development of biomarkers by genomic, transcriptomic, and proteomic methods holds the promise of “individualized medicine,” bringing a new ground-breaking point to disease diagnosis, classification, and prognosis. The gene expression profiling datasets in TCGA and GEO are of great value in deepening our understanding of the underlying molecular mechanisms involved in GC, as well as in the identification of novel diagnostic and prognostic biomarkers (47, 48). For the maximum utilization of GEO and TCGA data resources, it is very necessary to provide a web-based portal that allows clinicians and cancer biologists (regardless of having bioinformatics background or not) to easily access, analyze, and visualize the data. To identify genes related with patient survival is one possible way to prioritize genes with oncogenic or tumor-suppressor properties for further study. The user-friendly web portal of OSgc promotes the gene identification for survival associations in GC. Compared with published survival web tools such as OncoLnc (9), GEPIA (10), and KM plotter (11, 12), GEPIA and KM plotters are good in performing an extensive survey of prognosis in general cancer types; however, it has limited cases of GC (GEPIA contains 375 patients from TCGA; KM plotter includes 1,440 cases from TCGA and GEO). OncoLnc only containing TCGA data was developed to assess the prognostic significance of non-coding genes. More importantly, OSgc has integrated seven GC cohorts and incorporated the clinical covariates to provide more informative survival plots to the researchers.
We have demonstrated here the usefulness of OSgc using 20 previously published prognostic biomarkers as examples for rapid survival analysis. 85% (17 of 20) of these reported prognostic biomarkers were confirmed to be prognostically significant in OSgc. The three genes without statistical significance in OSgc could be due to several factors including different detecting methods (the analysis method for the HOXBP and SMYD3 prognosis study was IHC) and starting material (the material for SETD2 prognosis study was paraffin-embedded FFPE tissues). Moreover, we used OSgc to quickly systematically analyze the potential prognostic values of E2F family members and found that both E2F2 and E2F8 are favorable prognostic biomarkers for GC patients. Previous studies indicated that E2F2 exhibited as a tumor suppressor in epithelial tissues (49) or Myc-induced T cell lymphomagenesis (50), and overexpression of E2F2 inhibited the progression of these tumors. E2F8 is also a critical tumor suppressor for postnatal liver development (51). Compared with other web tools, OSgc is a free tool with the largest number of gastric cancers to perform the survival analysis based on gene expression. In the future, we will check whether the new standard datasets (including gene expression profile and clinical survival information) from different databases (such as TCGA, GEO and ArrayExpress) come out every 3 months, then we will incorporate these new gene expression profiles as well as additional utilities which are suggested by the users.
All in all, OSgc is a free and easy-to-use web portal to assess the performance of potential prognostic biomarkers for gastric cancer.
Data Availability Statement
The original contributions presented in the study are included in the article/supplementary material. Further inquiries can be directed to the corresponding authors.
Author Contributions
XG, XL: study concept and design. LX, QW, ZY: acquisition of data. QW, LX, YH, HL, LZ: analysis and interpretation of data. LX, QW, ZY, XG, XL: draft of the manuscript. XG, LX, QW, ZY, XL: critical revision of the manuscript for intellectual content. All authors contributed to the article and approved the submitted version.
Funding
This work was supported by the National Natural Science Foundation of China (No. U2004136), supporting program for Central Plain Young Top Talents (ZYQR201912176), Kaifeng Science and technology development Plan (2103005) program from the Academy for Advanced Interdisciplinary Studies of Henan University (Y21008L).
Conflict of Interest
The authors declare that the research was conducted in the absence of any commercial or financial relationships that could be construed as a potential conflict of interest.
Publisher’s Note
All claims expressed in this article are solely those of the authors and do not necessarily represent those of their affiliated organizations, or those of the publisher, the editors and the reviewers. Any product that may be evaluated in this article, or claim that may be made by its manufacturer, is not guaranteed or endorsed by the publisher.
Abbreviations
GC, gastric cancer; GEO, Gene Expression Omnibus; TCGA, The Cancer Genome Atlas; SETD2, SET domain-containing protein 2; BC, breast cancer; OC, ovarian cancer; HCC, hepatocellular carcinoma; LUCA, lung carcinoma.
References
1. Goossens N, Nakagawa S, Sun X, Hoshida Y. Cancer Biomarker Discovery and Validation. Transl Cancer Res (2015) 4:256–69. doi: 10.3978/j.issn.2218-676X.2015.06.04
2. Dang Y, Guo Y, Ma X, Chao X, Wang F, Cai L, et al. Systemic Analysis of the Expression and Prognostic Significance of PAKs in Breast Cancer. Genomics (2020) 112(3):2433–44. doi: 10.1016/j.ygeno.2020.01.016
3. Xie L, Dang Y, Guo J, Sun X, Xie T, Zhang L, et al. High KRT8 Expression Independently Predicts Poor Prognosis for Lung Adenocarcinoma Patients. Genes (2019) 10:36. doi: 10.3390/genes10010036
4. Zhao G, Liu C, Wen X, Luan G, Xie L, Guo X. The Translational Values of TRIM Family in Pan-Cancers: From Functions and Mechanisms to Clinics. Pharmacol Ther (2021) 227:107881. doi: 10.1016/j.pharmthera.2021.107881
5. Chen Z, Raghoonundun C, Chen W, Zhang Y, Tang W, Fan X, et al. SETD2 Indicates Favourable Prognosis in Gastric Cancer and Suppresses Cancer Cell Proliferation, Migration, and Invasion. Biochem Biophys Res Commun (2018) 498(3):579–85. S0006291X18304960. doi: 10.1016/j.bbrc.2018.03.022
6. Wang W, Chen Z, Jin J, Long Z, Liu X, Cai H, et al. MDM2 Binding Protein as a Predictor of Metastasis and a Novel Prognostic Biomarker in Patients With Gastric Cancer. Oncol Lett (2017) 14:6409–16. doi: 10.3892/ol.2017.7031
7. Goswami CP, Nakshatri H. PROGgeneV2: Enhancements on the Existing Database. BMC Cancer (2014) 14:970. doi: 10.1186/1471-2407-14-970
8. Gentles AJ, Newman AM, Liu CL, Bratman SV, Feng W, Kim D, et al. The Prognostic Landscape of Genes and Infiltrating Immune Cells Across Human Cancers. Nat Med (2015) 21:938–45. doi: 10.1038/nm.3909
9. Anaya J. OncoLnc: Linking TCGA Survival Data to mRNAs, miRNAs, and lncRNAs. PeerJ Comput Sci (2016) 2:e67. doi: 10.7717/peerj-cs.67
10. Tang Z, Li C, Kang B, Gao G, Li C, Zhang Z. GEPIA: A Web Server for Cancer and Normal Gene Expression Profiling and Interactive Analyses. Nucleic Acids Res (2017) 45(W1):W98–W102. doi: 10.1093/nar/gkx247
11. Szász AM, Lánczky A, Nagy Á, Förster S, Hark K, Green JE, et al. Cross-Validation of Survival Associated Biomarkers in Gastric Cancer Using Transcriptomic Data of 1,065 Patients. Oncotarget (2016) 7:49322–33. doi: 10.18632/oncotarget.10337
12. Győrffy B, Surowiak P, Budczies J, Lánczky A. Online Survival Analysis Software to Assess the Prognostic Value of Biomarkers Using Transcriptomic Data in Non-Small-Cell Lung Cancer. PloS One (2013) 8:e82241. doi: 10.1371/journal.pone.0082241
13. Elfilali A, Lair S, Verbeke C, Rosa PL, Radvanyi F, Barillot E. ITTACA: A New Database for Integrated Tumor Transcriptome Array and Clinical Data Analysis. Nucleic Acids Res (2006) 34:D613–6. doi: 10.1093/nar/gkj022
14. Xie L, Wang Q, Dang Y, Ge L, Sun X, Li N, et al. OSkirc: A Web Tool for Identifying Prognostic Biomarkers in Kidney Renal Clear Cell Carcinoma. Future Oncol (London England) (2019) 15:3103–10. doi: 10.2217/fon-2019-0296
15. Xie L, Wang Q, Nan F, Ge L, Dang Y, Sun X, et al. OSacc: Gene Expression-Based Survival Analysis Web Tool For Adrenocortical Carcinoma. Cancer Manag Res (2019) 11:9145–52. doi: 10.2147/CMAR.S215586
16. Förster S, Gretschel S, Jöns T, Yashiro M, Kemmner W. THBS4, a Novel Stromal Molecule of Diffuse-Type Gastric Adenocarcinomas, Identified by Transcriptome-Wide Expression Profiling. Mod Pathol (2011) 24:1390. doi: 10.1038/modpathol.2011.99
17. Lee J, Sohn I, Do I-G, Kim K-M, Park SH, Park JO, et al. Nanostring-Based Multigene Assay to Predict Recurrence for Gastric Cancer Patients After Surgery. PloS One (2014) 9:e90133. doi: 10.1371/journal.pone.0090133
18. Soldner F, Laganière J, Cheng AW, Hockemeyer D, Gao Q, Alagappan R, et al. Generation of Isogenic Pluripotent Stem Cells Differing Exclusively at Two Early Onset Parkinson Point Mutations. Cell (2011) 146:318–31. doi: 10.1016/j.cell.2011.06.019
19. Qian Z, Zhu G, Tang L, Wang M, Zhang L, Fu J, et al. Whole Genome Gene Copy Number Profiling of Gastric Cancer Identifies PAK1 and KRAS Gene Amplification as Therapy Targets. Genes Chromosomes Cancer (2014) 53:883–94. doi: 10.1002/gcc.22196
20. Cristescu R, Lee J, Nebozhyn M, Kim K-M, Ting JC, Wong SS, et al. Molecular Analysis of Gastric Cancer Identifies Subtypes Associated With Distinct Clinical Outcomes. Nat Med (2015) 21:449–56. doi: 10.1038/nm.3850
21. Cheong JH, Yang HK, Kim H, Kim WH, Kim YW, Kook MC, et al. Predictive Test for Chemotherapy Response in Resectable Gastric Cancer: A Multi-Cohort, Retrospective Analysis. Lancet Oncol (2018) 19:629. doi: 10.1016/S1470-2045(18)30108-6
22. Gravalos C, Jimeno A. HER2 in Gastric Cancer: A New Prognostic Factor and a Novel Therapeutic Target. Ann Oncol (2008) 19:1523–9. doi: 10.1093/annonc/mdn169
23. Wu Y, Zhou T, Tang Q, Xiao J. HOXA5 Inhibits Tumor Growth of Gastric Cancer Under the Regulation of microRNA-196a. Gene (2019) 681:62–8. doi: 10.1016/j.gene.2018.09.051
24. Li L, Fu LQ, Wang HJ, Wang YY. CAP2 is a Valuable Biomarker for Diagnosis and Prognostic in Patients With Gastric Cancer. Pathol Oncol Res (2018) 26(1):1–7. doi: 10.1007/s12253-018-0450-4
25. Wang X, Qinge H, Xinling Z. LAMA4 Expression is Activated by Zinc Finger E?box?binding Homeobox?1 and Independently Predicts Poor Overall Survival in Gastric Cancer. Oncol Rep (2018) 40(3):1725–33. doi: 10.3892/or.2018.6564
26. He XY, Zhao J, Chen ZQ, Jin R, Liu CY. High Expression of Retinoic Acid Induced 14 (RAI14) in Gastric Cancer and Its Prognostic Value. Med Sci Monit (2018) 24:2244. doi: 10.12659/MSM.910133
27. Zhang Z, She J, Yang J, Bu X, Ji G, Zhu S, et al. NDRG4 in Gastric Cancer Determines Tumor Cell Proliferation and Clinical Outcome. Mol Carcinog (2018) 57(6):762–71. doi: 10.1002/mc.22798
28. Tsutomu S, Takashi O, Naoto Y, Takanobu Y, Shinichi H, Norio Y, et al. Clinical Significance of SPARC Gene Expression in Patients With Gastric Cancer. J Surg Oncol (2013) 108:364–8. doi: 10.1002/jso.23425
29. Sha S, Gu Y, Xu B, Hu H, Yang Y, Kong X, et al. Decreased Expression of HOXB9 is Related to Poor Overall Survival in Patients With Gastric Carcinoma. Dig Liver Dis (2013) 45:422–9. doi: 10.1016/j.dld.2012.12.004
30. Florou D, Fragoulis EG, Scorilas A, Papadopoulos IN. L-Dopa Decarboxylase (DDC) Constitutes an Emerging Biomarker in;Predicting Patients' Survival With Stomach Adenocarcinomas. J Cancer Res Clin Oncol (2013) 139:297–306. doi: 10.1007/s00432-012-1326-8
31. Qiuhua D, Haihong Y, Yongping L, Yuan Q, Xia G, Ping H, et al. Prognostic Value of ERCC1 mRNA Expression in non-Small Cell Lung Cancer, Breast Cancer, and Gastric Cancer in Patients From Southern China. Int J Clin Exp Pathol (2014) 7:8312–21.
32. Liu Y, Deng J, Luo X, Pan Y, Zhang L, Zhang R, et al. Overexpression of SMYD3 was Associated With Increased STAT3 Activation in Gastric Cancer. Med Oncol (2015) 32:1–11. doi: 10.1007/s12032-014-0404-y
33. Liu L, Yang Z, Zhang W, Yan B, Gu Q, Jiao J, et al. Decreased Expression of IGFBP7 was a Poor Prognosis Predictor for Gastric Cancer Patients. Tumor Biol (2014) 35:8875–81. doi: 10.1007/s13277-014-2160-1
34. De la Peña S, Sampieri CL, Ochoa-Lara M, León-Córdoba K, Remes-Troche JM. Expression of the Matrix Metalloproteases 2, 14, 24, and 25 and Tissue Inhibitor 3 as Potential Molecular Markers in Advanced Human Gastric Cancer. Dis Markers (2014) 2014:285906. doi: 10.1155/2014/285906
35. Hisashi N, Koshi M, Tohru U, Barnard GF, Masaichi O, Kosei H, et al. Clinicopathologic and Biological Significance of Kallikrein 6 Overexpression in Human Gastric Cancer. Clin Cancer Res (2005) 11:6800–6. doi: 10.1158/1078-0432.CCR-05-0943
36. Chen C, Wang X, Huang X, Yong H, Shen J, Tang Q, et al. Nicotinamide N-Methyltransferase: A Potential Biomarker for Worse Prognosis in Gastric Carcinoma. Am J Cancer Res (2016) 6:649.
37. Zhang MJ, Zhang CZ, Du WJ, Yang XZ, Chen ZP. ATAD2 is Overexpressed in Gastric Cancer and Serves as an Independent Poor Prognostic Biomarker. Clin Transl Oncol (2015) 18:1–6. doi: 10.1007/s12094-015-1430-8
38. Hu M, Li K, Maskey N, Xu Z, Yu F, Peng CW, et al. Overexpression of the Chemokine Receptor CXCR3 and its Correlation With Favorable Prognosis in Gastric Cancer. Hum Pathol (2015) 46(12):1872–80. doi: 10.1016/j.humpath.2015.08.004
39. Liu H, Liu Y, Kong F, Xin W, Li X, Liang H, et al. Elevated Levels of SET and MYND Domain-Containing Protein 3 Are Correlated With Overexpression of Transforming Growth Factor-β1 in Gastric Cancer. J Am Coll Surg (2015) 221:579–90. doi: 10.1016/j.jamcollsurg.2015.02.023
40. Wang YY, Ye ZZ. High-Level Expression of S100A4 Correlates With Lymph Node Metastasis and Poor Prognosis in Patients With Gastric Cancer. Ann Surg Oncol (2010) 17:89–97. doi: 10.1245/s10434-009-0722-z
41. Trimarchi JM, Lees JA. Sibling Rivalry in the E2F Family. Nat Rev Mol Cell Biol (2002) 3:11–20. doi: 10.1038/nrm714
42. Hui-Zi C, Shih-Yin T, Gustavo L. Emerging Roles of E2Fs in Cancer: An Exit From Cell Cycle Control. Nat Rev Cancer (2009) 9:785. doi: 10.1038/nrc2696
43. Li Y, Huang J, Yang D, Xiang S, Sun J, Li H, et al. Expression Patterns of E2F Transcription Factors and Their Potential Prognostic Roles in Breast Cancer. Oncol Lett (2018) 15:9216–30. doi: 10.3892/ol.2018.8514
44. Zhou Q, Zhang F, He Z, Zuo M-Z. E2F2/5/8 Serve as Potential Prognostic Biomarkers and Targets for Human Ovarian Cancer. Front Oncol (2019) 9:161. doi: 10.3389/fonc.2019.00161
45. Huang Y-L, Ning G, Chen L-B, Lian Y-F, Gu Y-R, Wang J-L, et al. Promising Diagnostic and Prognostic Value of E2Fs in Human Hepatocellular Carcinoma. Cancer Manag Res (2019) 11:1725. doi: 10.2147/CMAR.S182001
46. Sun C-C, Zhou Q, Hu W, Li S-J, Zhang F, Chen Z-L, et al. Transcriptional E2F1/2/5/8 as Potential Targets and Transcriptional E2F3/6/7 as New Biomarkers for the Prognosis of Human Lung Carcinoma. Aging (Albany NY) (2018) 10:973. doi: 10.18632/aging.101441
47. Liu J, Liu Z, Zhang X, Gong T, Yao D. Bioinformatic Exploration of OLFML2B Overexpression in Gastric Cancer Base on Multiple Analyzing Tools. BMC Cancer (2019) 19:227. doi: 10.1186/s12885-019-5406-x
48. Sun H, Ni Sj, Ye M, Weng W, Zhang Q, Zhang M, et al. Hedgehog Interacting Protein 1 is a Prognostic Marker and Suppresses Cell Metastasis in Gastric Cancer. J Cancer (2018) 9:4642. doi: 10.7150/jca.27686
49. Pusapati RV, Weaks RL, Rounbehler RJ, McArthur MJ, Johnson DG. E2F2 Suppresses Myc-Induced Proliferation and Tumorigenesis. Mol Carcinog (2010) 49:152–6. doi: 10.1002/mc.20584
50. Guimond M. Specific Tumor Suppressor Function for E2F2 in Myc-Induced T Cell Lymphomagenesis. Proc Natl Acad Sci U S A (2007) 104:15400–5. doi: 10.1073/pnas.0706307104
Keywords: gastric cancer, gene expression, GEO, OSgc, prognostic biomarker, survival analysis, TCGA
Citation: Xie L, Wang Q, Yan Z, Han Y, Ma X, Li H, Zhang L, Li X and Guo X (2022) OSgc: A Web Portal to Assess the Performance of Prognostic Biomarkers in Gastric Cancer. Front. Oncol. 12:856988. doi: 10.3389/fonc.2022.856988
Received: 19 January 2022; Accepted: 16 February 2022;
Published: 11 March 2022.
Edited by:
Meng Zhou, Wenzhou Medical University, ChinaReviewed by:
Zhihui Liu, Stanford University, United StatesShoukai Kang, University of Washington, United States
Tao Wan, University of Nebraska System, United States
Long-Qi Chen, Sichuan University, China
Copyright © 2022 Xie, Wang, Yan, Han, Ma, Li, Zhang, Li and Guo. This is an open-access article distributed under the terms of the Creative Commons Attribution License (CC BY). The use, distribution or reproduction in other forums is permitted, provided the original author(s) and the copyright owner(s) are credited and that the original publication in this journal is cited, in accordance with accepted academic practice. No use, distribution or reproduction is permitted which does not comply with these terms.
*Correspondence: Xiangqian Guo, xqguo@henu.edu.cn; Xianzhe Li, nslxz2007@163.com
†These authors share first authorship