- 1Guangdong Provincial Key Laboratory of Malignant Tumor Epigenetics and Gene Regulation, Sun Yat Sen Memorial Hospital, Sun Yat-Sen University, Guangzhou, China
- 2Department of Oral and Maxillofacial Surgery, Sun Yat Sen Memorial Hospital, Sun Yat-Sen University, Guangzhou, China
- 3RNA Biomedical Institute, Sun Yat Sen Memorial Hospital, Sun Yat-Sen University, Guangzhou, China
- 4Department of Hepatobiliary Surgery, Sun Yat Sen Memorial Hospital, Sun Yat-Sen University, Guangzhou, China
Purpose: The biological roles and clinical significance of RNA-binding proteins (RBPs) in oral squamous cell carcinoma (OSCC) are not fully understood. We investigated the prognostic value of RBPs in OSCC using several bioinformatic strategies.
Materials and Methods: OSCC data were obtained from a public online database, the Limma R package was used to identify differentially expressed RBPs, and functional enrichment analysis was performed to elucidate the biological functions of the above RBPs in OSCC. We performed protein-protein interaction (PPI) network and Cox regression analyses to extract prognosis-related hub RBPs. Next, we established and validated a prognostic model based on the hub RBPs using Cox regression and risk score analyses.
Results: We found that the differentially expressed RBPs were closely related to the defense response to viruses and multiple RNA processes. We identified 10 prognosis-related hub RBPs (ZC3H12D, OAS2, INTS10, ACO1, PCBP4, RNASE3, PTGES3L-AARSD1, RNASE13, DDX4, and PCF11) and effectively predicted the overall survival of OSCC patients. The area under the receiver operating characteristic (ROC) curve (AUC) of the risk score model was 0.781, suggesting that our model exhibited excellent prognostic performance. Finally, we built a nomogram integrating the 10 RBPs. The internal validation cohort results showed a reliable predictive capability of the nomogram for OSCC.
Conclusion: We established a novel 10-RBP-based model for OSCC that could enable precise individual treatment and follow-up management strategies in the future.
Introduction
Oral cancer, comprising a group of tumors located in the alveolar ridge, buccal cavity, mucosa, floor of the mouth, palate, tongue, and other parts of the oral cavity, accounts for an estimated 350,000 new cases and 170,000 deaths per year worldwide. Of these, oral squamous cell carcinoma (OSCC) accounts for more than 95% of oral tumors. A major portion of global OSCC cases are diagnosed in Asia (1). Despite developments in the therapeutic strategies of OSCC that have been achieved over the last few years, the long-term survival rates of OSCC patients are still extremely low. Additionally, precise targeted therapies remain limited, resulting in treatment failure due to individual genetic differences and epigenetic changes among OSCC patients (2–4). Thus, a systematic study to identify key biomarkers for diagnosis and effective targets for treatment of OSCC is urgently needed.
Nomograms are statistics-based tools that can effectively integrate different variables and are widely used to detect prognostic factors for various cancers (5, 6). Many models have been established to predict the outcomes of OSCC by calculating the risk associated with clinicopathologic characteristics, such as age, sex, smoking status, TNM staging, and therapies (7, 8). Although these studies have enhanced our understanding of OSCC, few have taken into account genetic features, and these studies are not sufficiently accurate and reliable, as the underlying molecular mechanisms of OSCC might be considerably complex (9).
RNA-binding proteins (RBPs) modulate the functions of RNA transcripts at multiple levels (10). Currently, 1,542 RBPs have been identified, and more than 800 mRNAs have been discovered in human cells (11, 12). These RBPs bind RNA through globular RNA-binding domains (RBDs) and affect the splicing, stability, or translation of their target RNAs (13, 14). Considering the importance of RBPs in many cellular processes, it is not surprising that defects in their functions affect several human diseases, including neurological disorders, muscular atrophy, and cancer (15). Many studies have indicated that the dysregulation of RBPs is related to the biological behaviors of various cancer cells. For instance, human antigen R (HuR) is widely overexpressed in cancer cells and involved in posttranscriptional gene regulation (16). HuR not only affects lncRNAs but also binds to protein-coding mRNAs, exerting both positive and negative influences on their stability (17). The abnormal expression of heterogeneous nuclear ribonucleoprotein K (hnRNPK) is frequently observed in cancers and might play an important role in hepatocellular carcinoma (HCC) by changing the localization of MALAT1 (18). Serine/arginine-rich splicing factor 1 (SRSF1) is an RBP that is upregulated in glioma and plays an important role by regulating splicing, RNA stability, and nuclear export (19). Insulin-like growth factor 2 mRNA-binding protein 1 (IGF2BP1) modulates oncogenic impact on regulating cell proliferation and apoptosis in HCC (20). Mitogen-activated protein kinase-activated protein kinase-2 (MAPKAPK2) regulates transcript stability and exerts important effects in head and neck squamous cell carcinoma (HNSCC) (21). In summary, these previous studies have shown that RBPs may play important roles in tumorigenesis and tumor progression. However, only a few RBPs have been studied recently, and some RBPs were revealed to have critical functions in cancers.
In this study, we integrated all relevant OSCC information from The Cancer Genome Atlas (TCGA) database to perform a novel systematic analysis. To reveal the potential functions and clinical significance of RBPs in the pathogenesis of OSCC, we screened multiple differentially expressed RBPs in OSCC. Some of them may provide potential biomarkers for OSCC patients’ prognosis prediction.
Materials and Methods
Dataset Collection and Identification of Differentially Expressed RBPs
The RNA sequencing data of 32 normal oral tissues and 331 OSCC tissues with corresponding clinical information were obtained from TCGA (https://portal.gdc.cancer.gov/). Approval of the institutional ethics committee was not necessary because the data were collected from publicly available online databases. To identify the differentially expressed genes between normal oral and OSCC tissue samples, all raw data were preprocessed using the Limma package. In addition, we also identified differentially expressed RBPs with a threshold of |log2-fold change (FC)| ≥1 and a false discovery rate (FDR) <0.05. All TCGA patients were randomly divided into a training cohort with N*p (p = 2/3) samples and an internal validation cohort with N*q (q = 1/3) samples by caret R package. To validate our results responsibly, we searched for external validation cohort from Sun Yat-sen Memorial Hospital (n=58). This study was approved by the institutional review board of Sun Yat-Sen Memorial Hospital, Sun Yat-Sen University.
KEGG Pathway and GO Enrichment Analyses
The biological functions of differentially expressed RBPs were systematically detected by Gene Ontology (GO) enrichment and Kyoto Encyclopedia of Genes and Genomes (KEGG) pathway analyses. The GO analysis included terms in the cellular component (CC), molecular function (MF), and biological process (BP) categories. All enrichment analyses were conducted by the WEB-based Gene Set Analysis Toolkit (WebGestalt, http://www.webgestalt.org/) (22). For both analyses, P values less than 0.05 and gene numbers greater than 5 were considered statistically significant.
PPI Network Construction and Module Screening
The protein-protein interactions (PPIs) among all differentially expressed RBPs were investigated using the Search Tool for the Retrieval of Interacting Genes/Proteins (STRING) database (http://www.string-db.org/) (23). Cytoscape 3.8.0 software was used to further visualize the PPI network. The Molecular Complex Detection (MCODE) plug-in was used to select key modules and genes in the PPI network with both MCODE scores and node count numbers greater than 5 (24). A P value <0.05 was considered to indicate a significant difference.
Prognosis-Related RBP Identification and Prognostic Model Construction
We performed univariate Cox regression analysis on the differentially expressed RBPs in the critical modules of the training dataset using the survival R package. Subsequently, we performed a log-rank test to extract prognosis-related RBPs. Based on the above preliminarily screened significant candidate RBPs, a multivariate Cox proportional hazards regression model was further performed, and the risk score (RS) was calculated to predict the prognostic outcomes of OSCC patients (25). The RS formula for each sample was as follows: RS=Expressiongene1×βgene1+ ··· + Expressiongenen×βgenen (where β is the regression coefficient derived from multivariate Cox regression). OSCC patients were divided into low-risk and high-risk groups according to the median RS analysis. The log-rank test was used to compare the difference in overall survival (OS) between the two groups. In addition, the survival ROC package was used to assess the prognostic capability of the above model (26). In addition, an internal validation cohort was used to test the predictive capability of the nomogram. Calibration curves were constructed using the rms R package to observe the accuracy of the prediction. A P value <0.05 indicated a significant difference.
Verification of Express Level and Prognostic Significance by Immunohistochemistry (IHC)
Fixed OSCC and adjacent normal tissues samples were sectioned at 4 µm thickness. The tissue samples were deparaffinized, subjected to antigen retrieval with sodium citrate, and incubated with 3% hydrogen peroxide for 15 min at room temperature. Then, they were blocked with goat serum for 15 min at 37°C and incubated with the indicated antibody at 4°C overnight. Subsequently, the secondary antibody was added and incubated for 1 h at room temperature. Finally, the sections were visualized with 3,3’-diaminobenzidine. The stained slides were scanned, and the images were then digitalized and analyzed using Image-Pro Plus 5.1 software.
Results
Identification of Differentially Expressed RBPs in OSCC
Figure 1 shows the workflow of this study. RNA expression data and corresponding clinical information were downloaded from TCGA. In total, 331 OSCC samples and 32 normal oral samples were analyzed. The Limma R software package was used to preprocess these data and to identify differentially expressed RBPs (11). A total of 1,542 RBPs were analyzed in this study, and 257 met our inclusion criteria (P<0.05, |log2FC)| >1.0), including 121 downregulated and 136 upregulated RBPs (Figure 2).
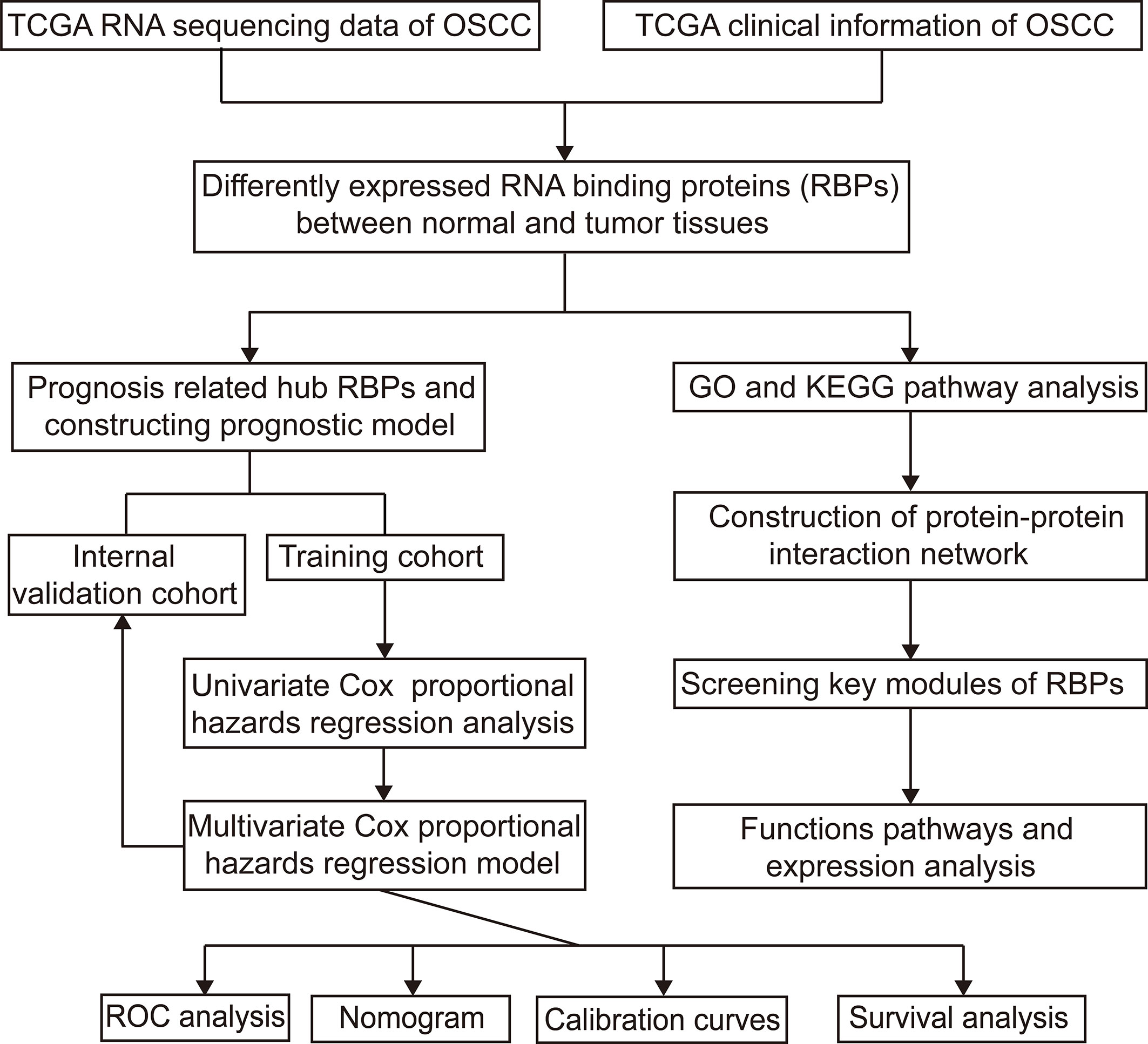
Figure 1 Study flowchart for analyzing RBPs in OSCC. OSCC, oral squamous cell carcinoma; RBPs, RNA binding proteins.
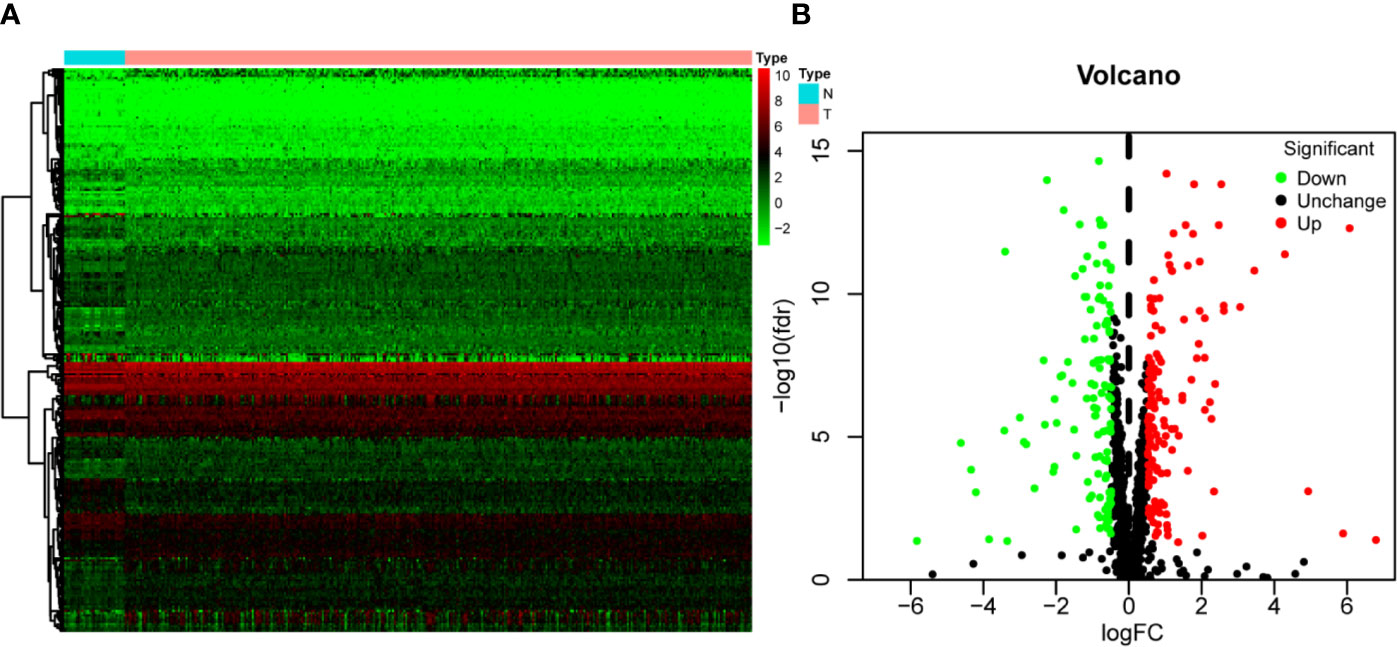
Figure 2 The differentially expressed RBPs in OSCC. (A) Hierarchical clustering of OSCC tissues and normal tissues by differentially expressed RBPs. The upper horizontal axis represents samples, and the left vertical axis represents clusters of RBPs. Red represents upregulated RBPs, and green represents downregulated RBPs. (B) Volcano plot of differentially expressed RBPs. The red dots represent upregulated RBPs, and the green dots represent downregulated RBPs. FC, Fold Change; fdr, false discovery rate.
Functional Enrichment Analysis of the Differentially Expressed RBPs
The identified RBPs were divided into two groups, the upregulation group and the downregulation group, to explore their potential functions and mechanisms. The upregulated RBPs were significantly enriched in defense response to viruses and regulation of mRNA metabolic processes by GO and pathway analyses (Supplementary Table 1 and Figure 3A), while the downregulated RBPs were considerably enriched in mRNA processing, RNA splicing, and RNA catabolic processes (Supplementary Table 1 and Figure 3B). Additionally, the KEGG pathway analysis results showed that the upregulated RBPs were enriched in the RNA transport, spliceosome, and mRNA surveillance pathways (Supplementary Table 1 and Figure 3C), while the downregulated RBPs were mainly enriched in the RNA transport, ribosome, and mRNA surveillance pathways (Supplementary Table 1 and Figure 3D).
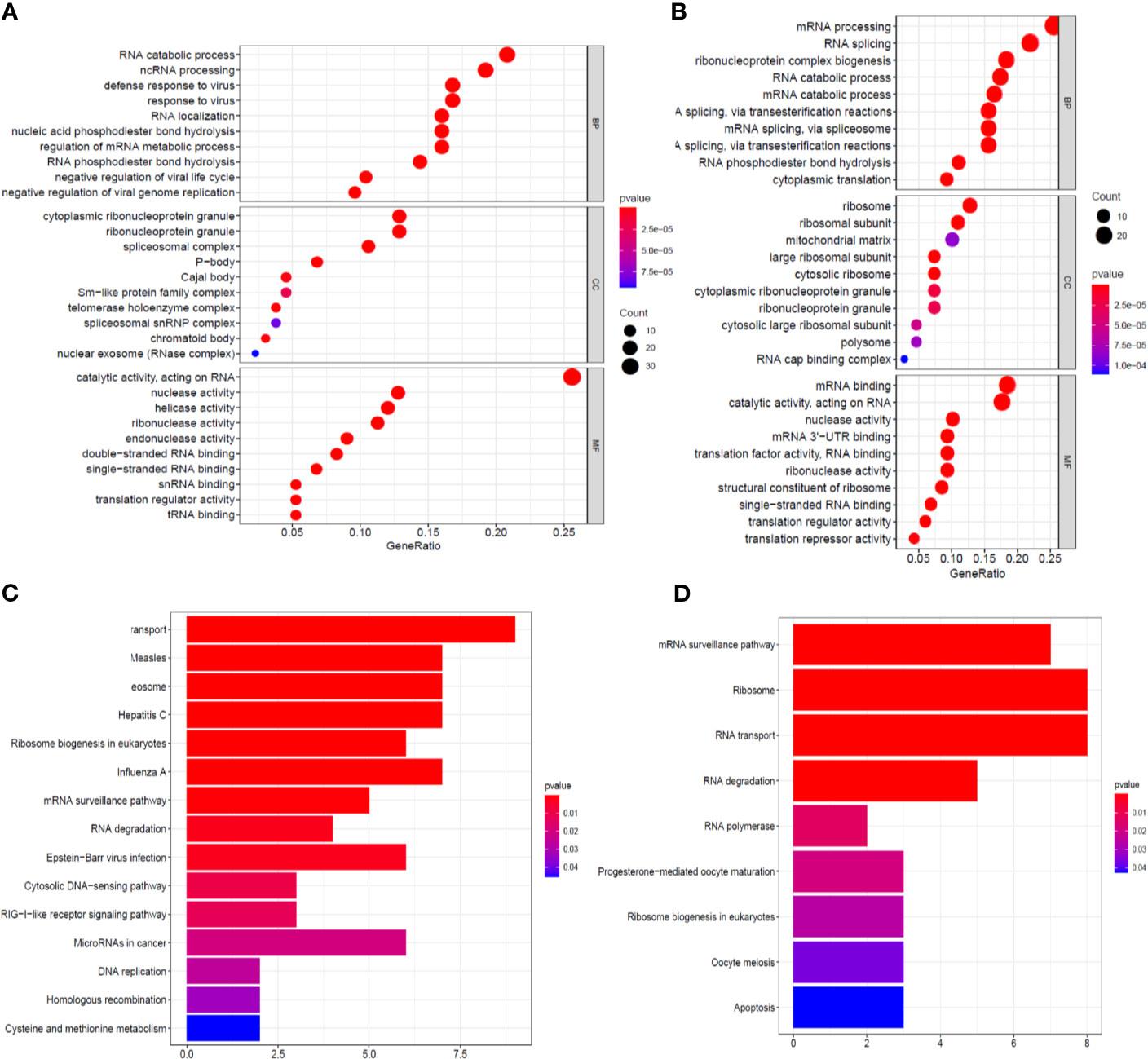
Figure 3 GO enrichment and KEGG pathway analyses of aberrantly expressed RBPs. Biological process, cellular components, and molecular function enrichment for up-expressed RBPs (A) and down-expressed RBPs (B); KEGG pathway analysis for up-expressed RBPs (C) and down-expressed RBPs (D).
PPI Network Construction and Key Module Screening
To better understand the potential roles of these identified RBPs in OSCC, we constructed a PPI network using the STRING database and Cytoscape software. This PPI network consists of 228 nodes and 1,384 edges in total (Figure 4A). We further built the coexpression network using the MCODE plug-in and identified the key modules (Figure 4B). Of these, the first essential module, module 1, contained 49 nodes and 316 edges; the second module, module 2, consisted of 8 nodes and 28 edges; module 3 consisted of 13 nodes and 43 edges; module 4 consisted of 6 nodes and 12 edges; the next module consisted of 4 nodes and 6 edges; and the last module consisted of 16 nodes and 29 edges (Supplementary Figures 1A–F). The functional analysis showed that the RBPs in these key modules were enriched in mitochondrial translation, mitochondrial gene expression, and cellular protein complex disassembly (Supplementary Table 1).
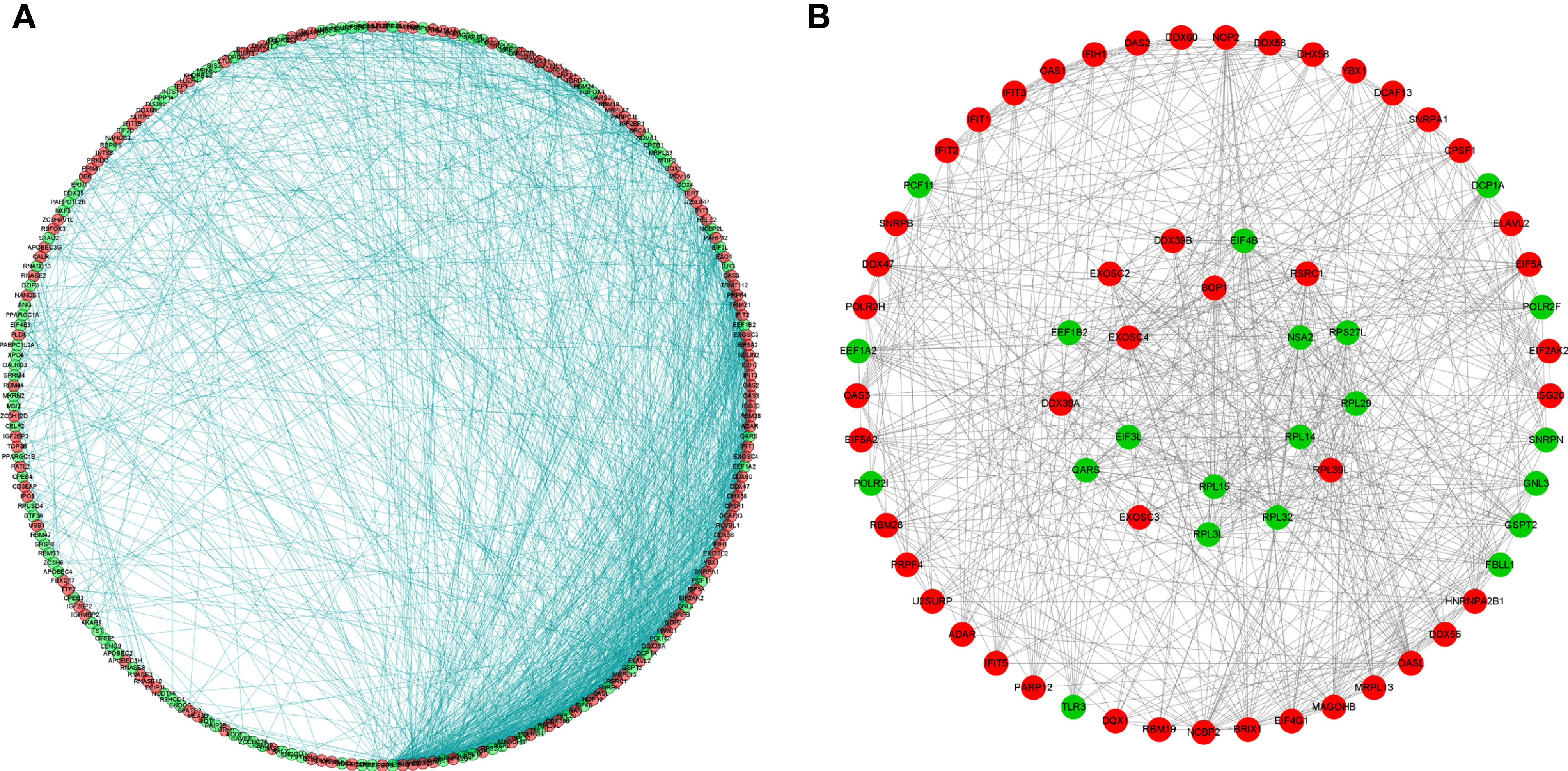
Figure 4 Protein-protein interaction network and modules analysis. (A) Protein-protein interaction network of differentially expressed RBPs; (B) Significant modules from PPI network. Green circles: down-expressed RBPs; red circles: up-expressed RBPs.
Identification of Prognosis-Related RBPs
A total of 20 prognosis-associated candidate hub RBPs were identified by univariate Cox regression analysis (Supplementary Table 2). We further analyzed the candidate RBPs by multivariate Cox regression to determine their significance for prognosis. Ten hub RBPs—ZC3H12D, OAS2, INTS10, ACO1, PCBP4, RNASE3, PTGES3L-AARSD1, RNASE13, DDX4, and PCF11—were identified as independent predictors of OSCC (Supplementary Table 3). According to the expression profiles of 10 RBPs, cluster analysis was performed to analyze the 331 OSCC samples from the entire TCGA cohort, and two subtypes were determined, which demonstrates associated heterogeneity can be effectively identified by 10 RBPs model (Supplementary Figure 2A). Subsequently, the result of principal component analysis (PCA) indicates that 10 RBPs can distinguish OSCC patients in training cohort, validation cohort, and entire TCGA cohort (Supplementary Figures 2B–D).
Prognosis-Related Genetic RS Model Construction and Validation
The 10 hub RBPs were used to build a prognostic model. To assess the predictive ability of this model, we further carried out an RS analysis in the training cohort. The RS of each patient was calculated according to the following formula: RS=ZC3H12D*-1.505+OAS2*-0.006+INTS10*-0.135+ACO1*0.108+PCBP4*-0.122+RNASE3*2.757+PTGES3L-AARSD1*2.827+RNASE13*-11.340+DDX4*8.283+PCF11*-0.204. A total of 221 OSCC patients were divided into low-risk and high-risk groups according to the median RS. The results showed that patients in the low-risk group had significantly longer OS times than those in the high-risk group (Figure 5A). Time-dependent ROC curve analysis was further conducted to estimate the prognostic ability of the identified RBPs. The area under the ROC curve (AUC) of the RS model was 0.781 (Figure 5B), which suggested that our model has high prognostic accuracy. The expression heat map of the 10 prognostic RBPs is illustrated in Figure 5C, and the RS and survival status for the low- and high-risk groups are displayed in Figure 5D. Moreover, the results from our internal validation cohort, using the same formula used to assess the predictive capability of the 10-gene prognostic model, showed that patients with lower RSs had better OS (Figures 6A–D). These results indicated that the prognostic model has significant predictive capability.
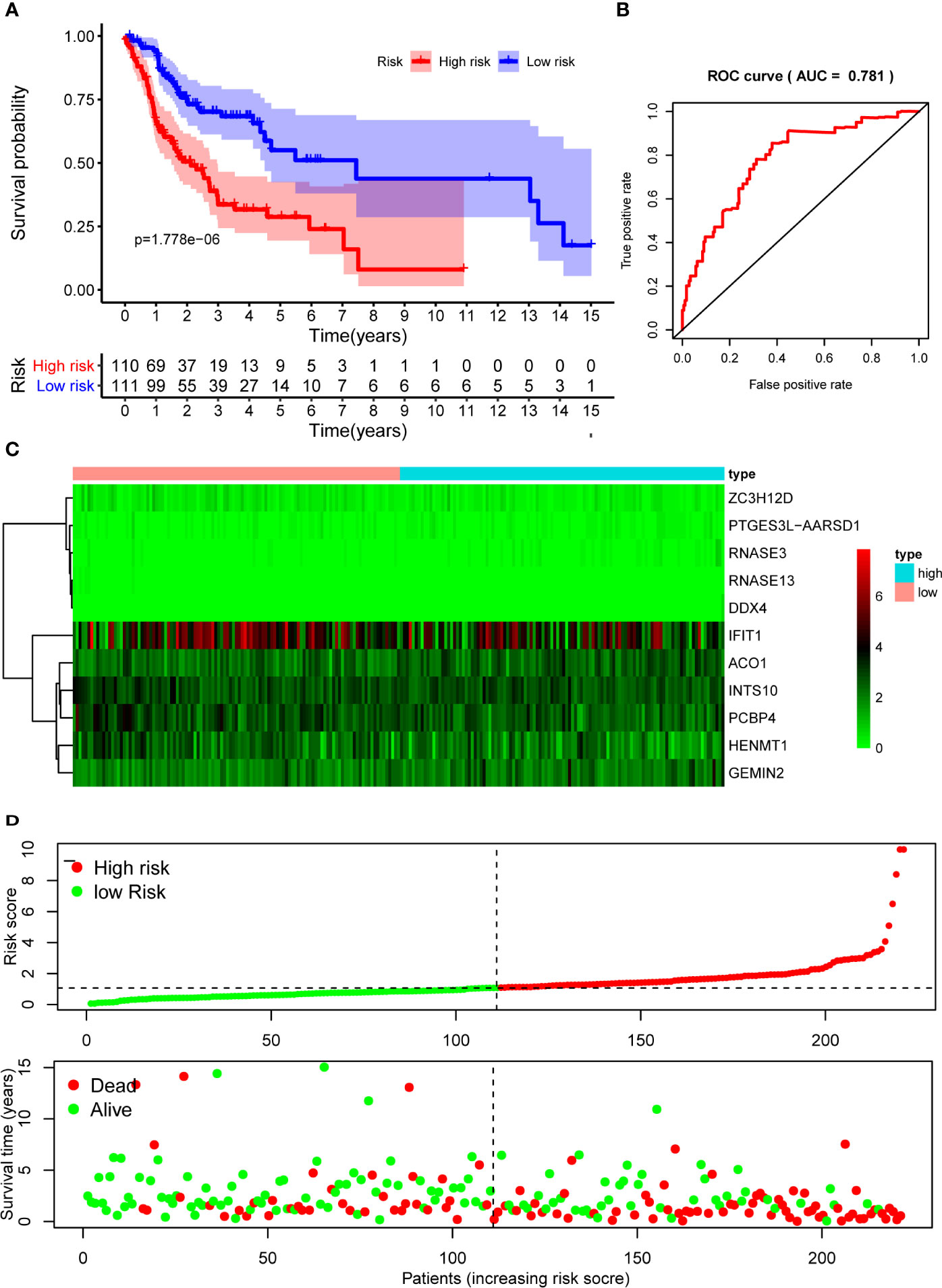
Figure 5 Risk score analysis of 10-gene prognostic model in the training cohort. (A) Survival curves for OS in low- and high-risk subgroups; (B) ROC curves for predicting OS based on risk score; (C) Expression heat map of 10 prognostic RBPs in low- and high-risk subgroups; (D) Risk score distribution and survival status for low- and high-risk subgroups. OS, overall survival; ROC, receiver operating characteristic.
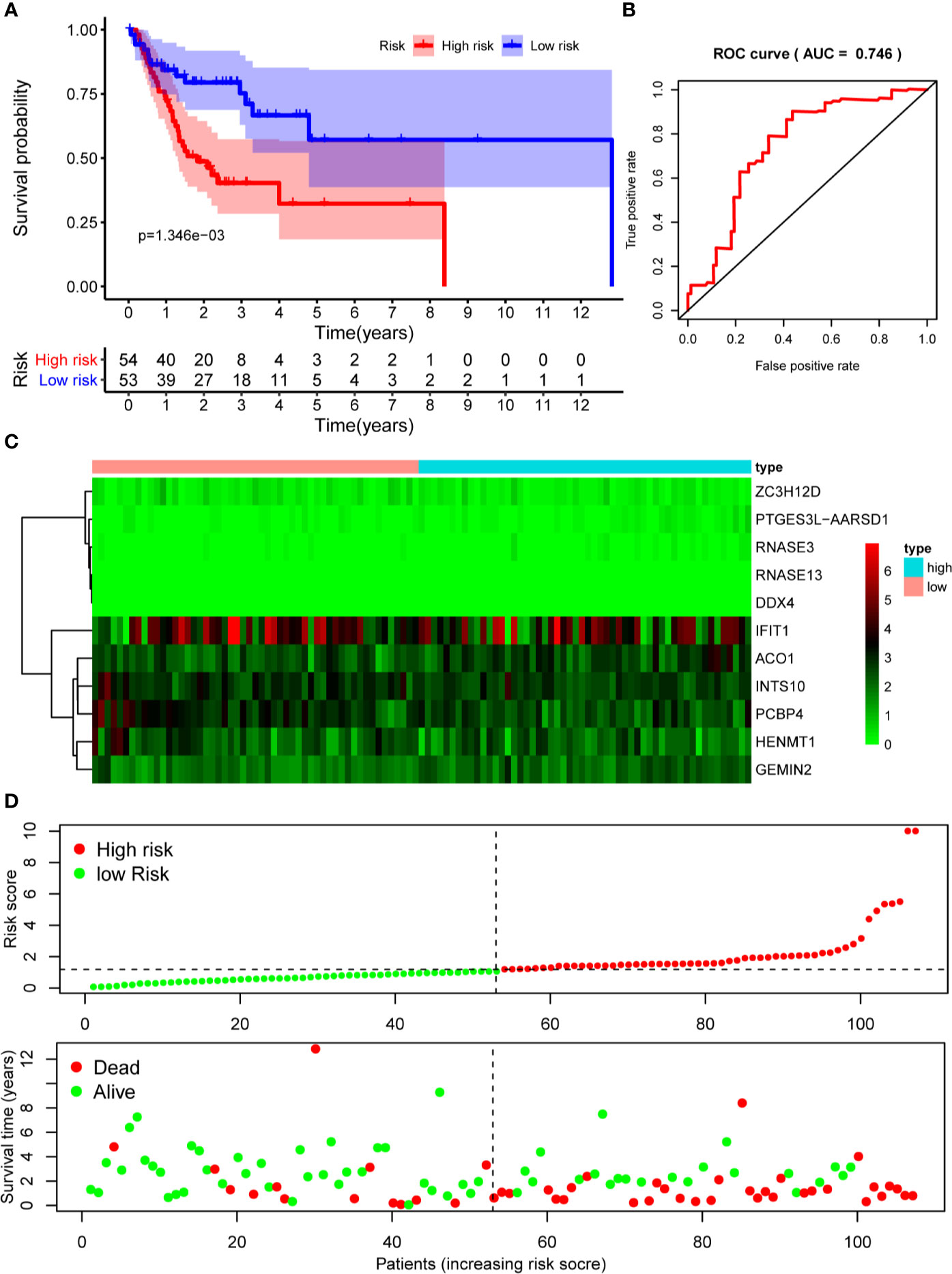
Figure 6 Risk score analysis of 10-gene prognostic model in the internal validation cohort. (A) Survival curves for OS in low- and high-risk subgroups; (B) ROC curves for predicting OS based on risk score; (C) Expression heat map of 10 prognostic RBPs in low- and high-risk subgroups; (D) Risk score distribution and survival status for low- and high-risk subgroups. OS, overall survival; ROC, receiver operating characteristic.
Prognostic Value of Different Clinical Parameters
To assess the prognostic significance of different clinical features, we performed a univariate Cox regression analysis of OSCC patients in the training set and internal validation set. The results showed that stage, grade, age, and RS were correlated with the OS of OSCC patients in both cohorts (P<0.05) (Figures 7A, B), whereas only stage and RS were independent prognostic factors for OS in the multiple regression analysis of both cohorts (P<0.05) (Figures 7C, D).
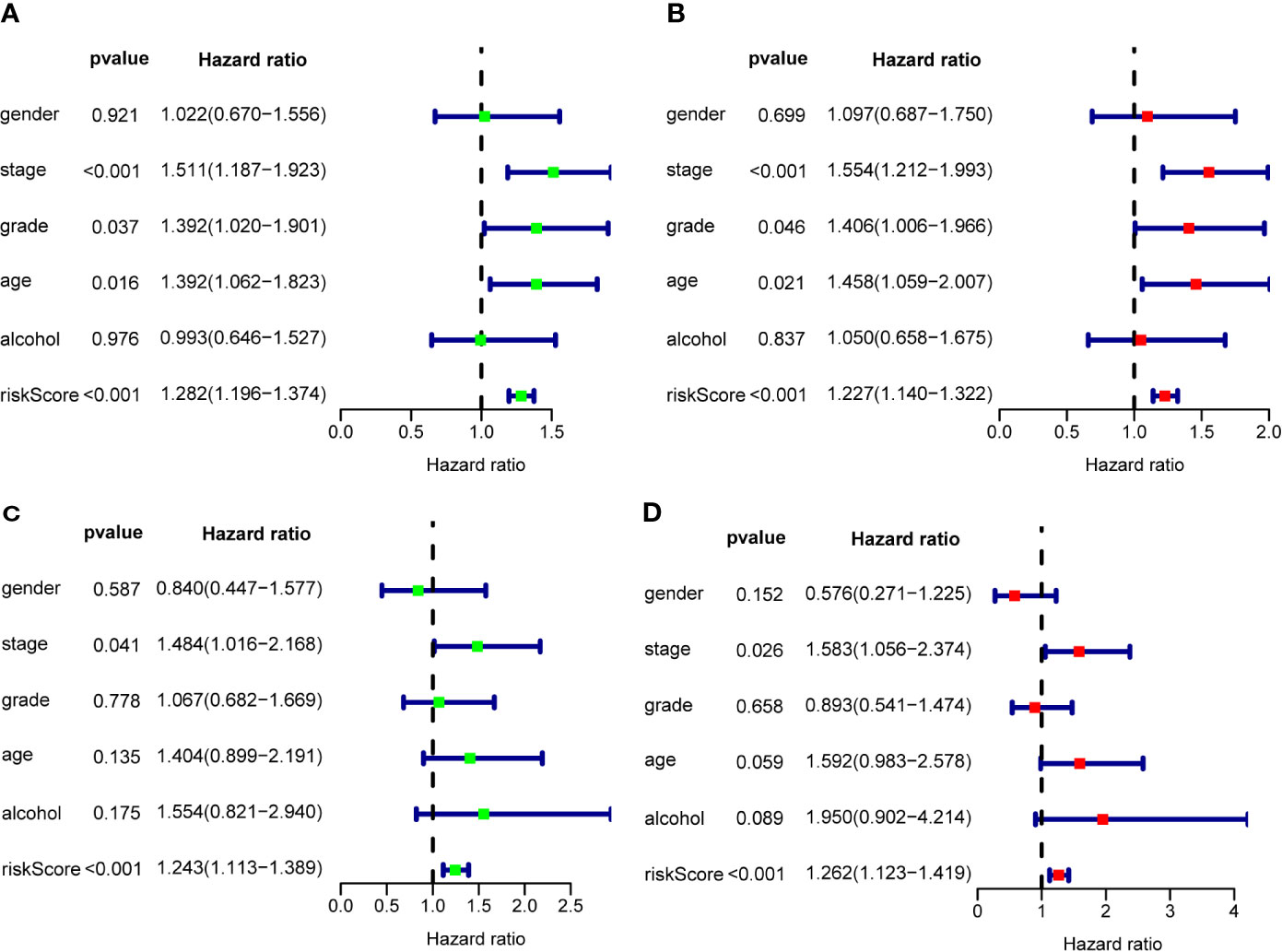
Figure 7 The prognostic value of different clinical parameters. Univariate Cox regression analysis and forest plots of the HR and 95% CIs in the training cohort (A) and internal validation cohort (C); Multivariate Cox regression analysis and forest plots of the HR and 95% CIs in the training cohort (B) and internal validation cohort (D).
Development and Validation of a Nomogram Based on the Hub RBPs
Based on the multivariate analysis results, a nomogram was established to predict the 1-, 2-, and 3-year OS (Figure 8A). We list the points for each independent prognostic factor in the nomogram and summed them to obtain the total point value, which was drawn on the bottom scale. We plotted the calibration curves, which indicated no apparent deviation from the ideal line, indicating our model might be effectively used to predict outcomes and survival rates of OSCC patients (Figures 8B, C).
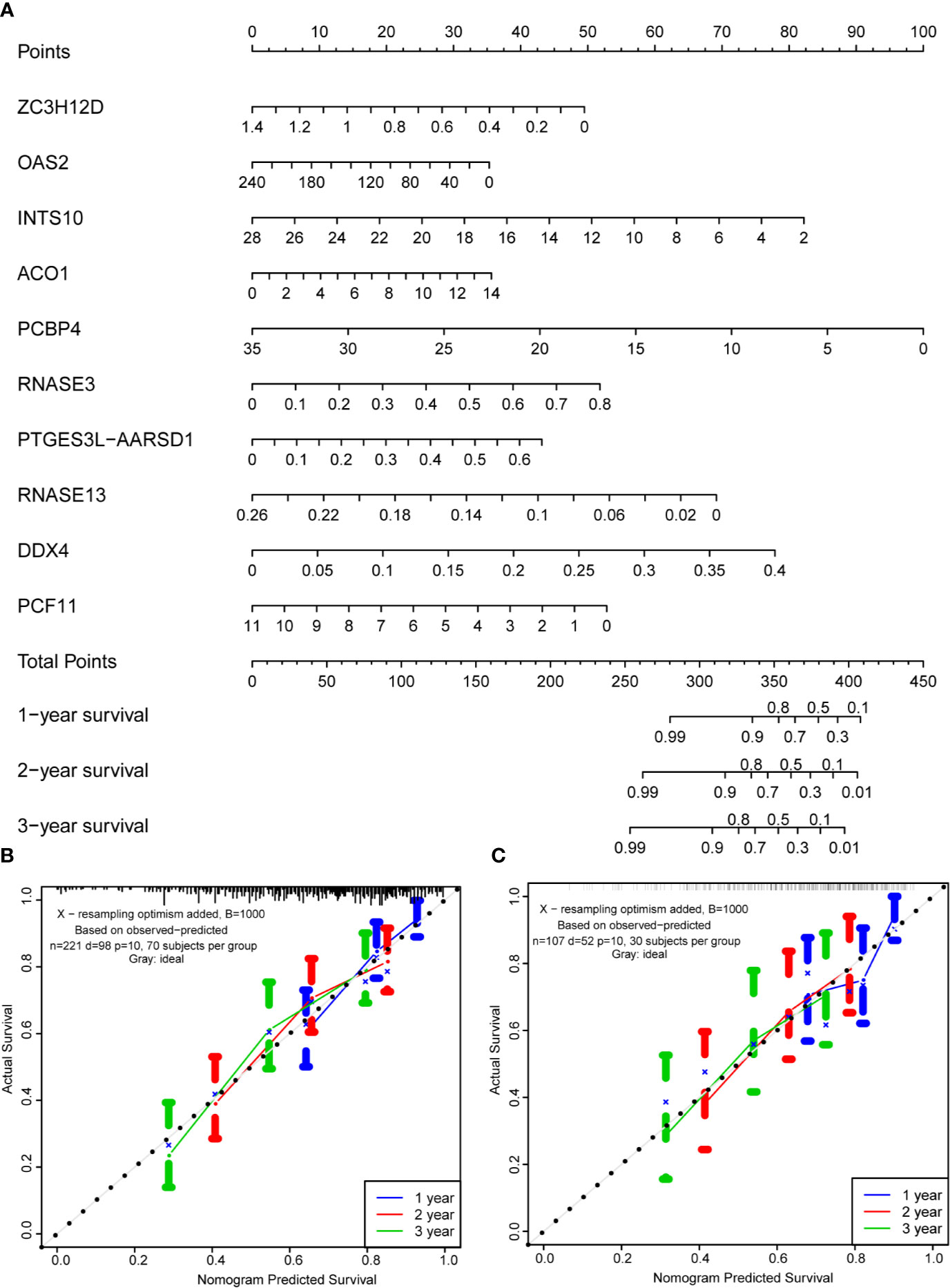
Figure 8 Prognostic nomogram based on hub RBPs and calibration plots. (A) Nomogram for predicting the 1-, 2-, and 3-year OS in the training cohort. All the points identified on the top scale for each factor were added to generate a total score. The total points projected on the bottom scale were used to determine the probabilities of 1-, 2-, and 3-year OS for each patient. Nomograms for predicting the 1-, 2-, and 3-year OS probabilities of OSCC patients in the training cohort (B) and internal validation cohort (C).
Next, the protein expression pattern of 10 RBPs in the prognosis model were analyzed in the tissues of OSCC patients from Sun Yat-Sen Memorial Hospital, Sun Yat-Sen University. The results demonstrated that the expression of the ZC3H12D, OAS2, INTS10, PCBP4, RNASE13, PCF11 protein were low expressed in OSCC tissues than that in normal tissues. ACO1, DDX4, PTGES3L-AARSD1, RNASE3 were high expressed in OSCC tissues than that in normal tissues (Supplementary Figure 3).
Discussion
The survival rate of OSCC patients remains low because there are limited prognostic factors that can provide effective targeted therapies and precisely predict outcomes (9). Previous studies have demonstrated that RBPs, which regulate oncogene expression at the posttranscriptional level, significantly affect tumorigenesis and progression, indicating that RBPs are potential cancer biomarkers (27, 28). In fact, several RBPs have been identified to predict OS in cancer. Zhao et al. identified a novel extra-ribosomal role of RPS3 as a potential therapeutic target in HCC via the posttranscriptional regulation of SIRT1 expression (29). Soni et al. highlighted that MK2, as the master regulator of RBPs, plays an important role in the regulation of transcript stability and tumor progression, as well as the possibility of the use of MK2 as a therapeutic target in tumor management (30). However, the role of RBPs in OSCC is not well understood, even though studies have focused on the functions of RBPs in head and neck carcinoma. Berggen et al. revealed that MK2 could be a potential prognostic biomarker for head and neck cancer and that MK2 pathway activation can mediate radiation resistance in HNSCC (31). Jiang et al. indicated that LINC00460 is a promising candidate prognostic predictor for HNSCC because it facilitates the entry of an RBP called PRDX1 into the nucleus and promotes the epithelial-mesenchymal transition (EMT) in HNSCC cells (32). However, most of these studies analyzed the functions of single RBPs, while prognostic markers including several RBPs should be collectively investigated (33).
In the present study, we identified dysregulated RBPs in OSCC based on TCGA database and systematically analyzed the related biological pathways of these RBPs. We further constructed a PPI network to identify the key modules that may have significant impact on the prognosis of OSCC. Subsequently, we performed Cox regression analysis and identified 10 prognosis-related RBPs. Moreover, we conducted RS analysis of this 10-gene prognostic model in the training and validation cohorts to explore the potential roles of these 10 hub RBPs. The model exhibited good predictive capability in both cohorts. Finally, we built and validated a nomogram to predict OSCC prognosis based on these hub RBPs. Our findings may provide novel insights into the prognosis of OSCC patients.
The biological function and pathway enrichment analyses showed that the downregulated RBPs were markedly enriched in the mRNA processing, RNA splicing, RNA catabolic process, RNA transport, ribosome, and mRNA surveillance pathways. Upregulated RBPs were significantly enriched in the defense response to viruses, response to viruses, regulation of mRNA metabolic process, RNA transport, RNA degradation, spliceosome, and mRNA surveillance pathways. Many studies have revealed the role of metabolism, RNA degradation, and RNA transport in OSCC (34–36). Human papillomavirus (HPV) is closely associated with OSCC (37). HPV infection significantly increases the number of binding sites of RBPs and subsequently upregulates a group of oncogenic genes by absorbing RBPs (38). A previous study showed that RBPs can regulate mRNA stability or translational efficiency via ribosomes and are involved in various diseases (39). These reports indicated that RBPs may affect the biological behavior of OSCC by interacting with components related to HPV or regulating the multiple biological processes of OSCC cells.
We identified 10 hub RBPs by constructing a PPI network and performing Cox regression analysis, inducing ZC3H12D, OAS2, INTS10, ACO1, PCBP4, RNASE3, PTGES3L-AARSD1, RNASE13, DDX4, and PCF11. Most of these RBPs are associated with tumorigenesis and cancer progression, including OAS2 (40), INTS10 (41), ACO1 (42), PCBP4 (43), DDX4 (44), and PCF11 (45). Of these, OAS2, an interferon (IFN)-induced antiviral enzyme, has been reported as an OSCC-related gene. Similarly, Wang et al. analyzed three microarray datasets and found that OAS2 expression was closely related to the survival rate of patients with oral cancer (46), which was consistent with our results. Interestingly, the IFN system is a central and powerful host first-line antiviral defense strategy that is inhibited by HPV16 in HNSCC (47). A substantial OAS2 level can activate the IFN signaling pathway and in turn produce a T-cell response that is effective in clearing HPV infection (48). Thus, OAS2 may serve as a key regulator and may provide rational insight into immunotherapy for HPV-associated OSCC. However, the detailed mechanisms underlying these hub RBPs in OSCC are still unexplored, and further in-depth studies to discover their potential roles would be valuable.
Subsequently, we established a risk model including the 10 hub RBPs. The ROC analysis revealed that these 10 genes had a good diagnostic capability to identify OSCC patients with a poor prognosis. Next, a prognostic nomogram was built to predict OSCC patients’ survival. Finally, calibration curves were plotted to detect the prognostic value of the 10 RBP coding genes, and the results indicated that the nomogram-predicted outcomes were consistent with the actual survival rates.
To sum up, all the evidence suggested that our prognostic model including 10 RBPs has a certain value for the treatment decisions of OSCC patients. Nonetheless, there are still several limitations in our study. The main one is the lack of clinical data. Survival analysis included only basic clinical factors which are obtained from TCGA database. However, as we have known, other factors, such as the HPV status of patients, may affect the reliability of the Cox regression analysis. In addition, the results of functional enrichment analysis in this study have suggested that some RBPs may affect the outcome of OSCC patients through interactions with virus. If we have more clinical information, we were able to have a better understanding of combinations between RBPs and HPV infection. Another limitation lies in the expression of all genes from TCGA cohort was detected in an OSCC tissue sample from one patient. But OSCC is an extremely heterogeneous tumor at both genetic and molecular levels. In the future, clinical trials are needed. More analysis in multiple OSCC specimens from one patient should be applied to confirm our results. Besides, cooperation with other hospitals is also needed to enlarge the clinical sample size for model validation.
Conclusion
We systematically explored the prognostic value of differentially expressed RBPs by performing a series of bioinformatics analyses on OSCC. A prognostic model of the 10 hub RBPs was constructed and further validated. Our results were used to establish a novel prognostic model for OSCC and will contribute new candidate markers to aid in therapeutic decision-making in the future.
Author’s Note
This manuscript has been released as a preprint at ResearchSquare.
Data Availability Statement
The datasets presented in this study can be found in online repositories. The names of the repository/repositories and accession number(s) can be found in the article/Supplementary Material.
Ethics Statement
For TCGA database, approval of the institutional ethics committee was not necessary because the data were collected from publicly available online databases. For external validation cohort, all procedures were approved by the institutional ethics committee of Sun Yat-Sen Memorial Hospital, and informed consent was obtained from all patients.
Author Contributions
Conception and design: YLu and YYa. Development of methodology: YLu and YYa. Analysis and interpretation of data (e.g., statistical analysis, bioinformatics analysis): YLu, YYa, BL, ML, and YLi. Writing, review, and/or revision of the manuscript: YLu, YYa, BL, ML, YLi, YYe, WC, JJ, JL, and SC. Administrative, technical, or material support (i.e., reporting or organizing data): YLu, BL, ML, YLi, JL, JJ, and SC. Study supervision: JL, JJ, and SC. All authors contributed to the article and approved the submitted version.
Funding
This work was supported by the National Natural Science Foundation of China (NSFC; 81900959 to YLu, 81802700 to YLi, 82072990 and 81872194 to JL).
Conflict of Interest
The authors declare that the research was conducted in the absence of any commercial or financial relationships that could be construed as a potential conflict of interest.
The reviewer WW declared a shared affiliation, with no collaboration, with the authors to the handling editor at the time of review.
Publisher’s Note
All claims expressed in this article are solely those of the authors and do not necessarily represent those of their affiliated organizations, or those of the publisher, the editors and the reviewers. Any product that may be evaluated in this article, or claim that may be made by its manufacturer, is not guaranteed or endorsed by the publisher.
Supplementary Material
The Supplementary Material for this article can be found online at: https://www.frontiersin.org/articles/10.3389/fonc.2021.592614/full#supplementary-material
Supplementary Figure 1 | Modules analysis of protein-protein interaction (PPI) network. (A) module 1 from PPI network; (B) module 2 from PPI network; (C) module 3 from PPI network; (D) module 4 from PPI network; (E) module 5 from PPI network; (F) module 6 from PPI network. Green circles: down-expressed RBPs; red circles: up-expressed RBPs. Related to Figure 4.
Supplementary Figure 2 | Cluster analysis based on 10 RBPs. (A) Cluster analysis indicated that 331 OSCC samples in TCGA can be divided into two groups. Principal component analysis was performed on the basis of cluster analysis in training cohort (B), validation cohort (C), and entire TCGA cohort (D).
Supplementary Figure 3 | Immunohistochemistry results in the prognosis-related 10 RBPs. Scale bar: 50 μm.
References
1. Bray F, Ferlay J, Soerjomataram I, Siegel RL, Torre LA, Jemal A. Global Cancer Statistics 2018: GLOBOCAN Estimates of Incidence and Mortality Worldwide for 36 Cancers in 185 Countries. CA Cancer J Clin (2018) 68(6):394–424. doi: 10.3322/caac.21492
2. Colevas AD, Yom SS, Pfister DG, Spencer S, Adelstein D, Adkins D, et al. NCCN Guidelines Insights: Head and Neck Cancers, Version 1.2018. J Natl Compr Canc Netw (2018) 16(5):479–90. doi: 10.6004/jnccn.2018.0026
3. Argiris A, Karamouzis MV, Raben D, Ferris RL. Head and Neck Cancer. Lancet (2008) 371(9625):1695–709. doi: 10.1016/S0140-6736(08)60728-X
4. Liu X, Zhao X, Gou C. Identification of Novel Methylated DNA Marker ZNF569 for Head and Neck Squamous Cell Carcinoma. J Cancer (2019) 10(10):2250–60. doi: 10.7150/jca.31156
5. Wang K, Li L, Fu L, Yuan Y, Dai H, Zhu T, et al. Integrated Bioinformatics Analysis the Function of RNA Binding Proteins (RBPs) and Their Prognostic Value in Breast Cancer. Front Pharmacol (2019) 10:140. doi: 10.3389/fphar.2019.00140
6. Li W, Li X, Gao LN, You CG. Integrated Analysis of the Functions and Prognostic Values of RNA Binding Proteins in Lung Squamous Cell Carcinoma. Front Genet (2020) 11:185. doi: 10.3389/fgene.2020.00185
7. Wang F, Zhang H, Wen J, Zhou J, Liu Y, Cheng B, et al. Nomograms Forecasting Long-Term Overall and Cancer-Specific Survival of Patients With Oral Squamous Cell Carcinoma. Cancer Med (2018) 7(4):943–52. doi: 10.1002/cam4.1216
8. Chen F, Lin L, Liu F, Yan L, Qiu Y, Wang J, et al. Three Prognostic Indexes as Predictors of Response to Adjuvant Chemoradiotherapy in Patients With Oral Squamous Cell Carcinoma After Radical Surgery: A Large-Scale Prospective Study. Head Neck (2019) 41(2):301–8. doi: 10.1002/hed.25495
9. Mattavelli D, Lombardi D, Missale F, Calza S, Battocchio S, Paderno A, et al. Prognostic Nomograms in Oral Squamous Cell Carcinoma: The Negative Impact of Low Neutrophil to Lymphocyte Ratio. Front Oncol (2019) 9:339. doi: 10.3389/fonc.2019.00339
10. Nahalka J. The Role of the Protein-RNA Recognition Code in Neurodegeneration. Cell Mol Life Sci (2019) 76(11):2043–58. doi: 10.1007/s00018-019-03096-3
11. Gerstberger S, Hafner M, Tuschl T. A Census of Human RNA-Binding Proteins. Nat Rev Genet (2014) 15(12):829–45. doi: 10.1038/nrg3813
12. Kechavarzi B, Janga SC. Dissecting the Expression Landscape of RNA-Binding Proteins in Human Cancers. Genome Biol (2014) 15(1):R14. doi: 10.1186/gb-2014-15-1-r14
13. Masuda K, Kuwano Y. Diverse Roles of RNA-Binding Proteins in Cancer Traits and Their Implications in Gastrointestinal Cancers. Wiley Interdiscip Rev RNA (2019) 10(3):e1520. doi: 10.1002/wrna.1520
14. Lunde BM, Moore C, Varani G. RNA-Binding Proteins: Modular Design for Efficient Function. Nat Rev Mol Cell Biol (2007) 8(6):479–90. doi: 10.1038/nrm2178
15. Castello A, Fischer B, Hentze MW, Preiss T. RNA-Binding Proteins in Mendelian Disease. Trends Genet (2013) 29(5):318–27. doi: 10.1016/j.tig.2013.01.004
16. Wang E, Aifantis I. RNA Splicing and Cancer [Published Online Ahead of Print, 2020 May 17]. Trends Cancer (2020) S2405-8033(20):30141–2. doi: 10.1016/j.trecan.2020.04.011
17. Hu YP, Jin YP, Wu XS, Yang Y, Li YS, Li HF, et al. LncRNA-HGBC Stabilized by HuR Promotes Gallbladder Cancer Progression by Regulating miR-502-3p/SET/AKT Axis. Mol Cancer (2019) 18(1):167. doi: 10.1186/s12943-019-1097-9
18. Nguyen TM, Kabotyanski EB, Reineke LC, Shao J, Xiong F, Lee JH, et al. The SINEB1 Element in the Long Non-Coding RNA Malat1 is Necessary for TDP-43 Proteostasis. Nucleic Acids Res (2020) 48(5):2621–42. doi: 10.1093/nar/gkz1176
19. Zhou X, Li X, Yu L, Wang R, Hua D, Shi C, et al. The RNA-Binding Protein SRSF1 Is a Key Cell Cycle Regulator via Stabilizing NEAT1 in Glioma. Int J Biochem Cell Biol (2019) 113:75–86. doi: 10.1016/j.biocel.2019.06.003
20. Gutschner T, Hämmerle M, Pazaitis N, Bley NM, Fiskin E, Uckelmann H, et al. Insulin-Like Growth Factor 2 mRNA-Binding Protein 1 (IGF2BP1) is an Important Protumorigenic Factor in Hepatocellular Carcinoma. Hepatology (2014) 59(5):1900–11. doi: 10.1002/hep.26997
21. Soni S, Saroch MK, Chander B, Tirpude NV, Padwad YS. MAPKAPK2 Plays a Crucial Role in the Progression of Head and Neck Squamous Cell Carcinoma by Regulating Transcript Stability. J Exp Clin Cancer Res (2019) 38(1):175. doi: 10.1186/s13046-019-1167-2
22. Liao Y, Wang J, Jaehnig EJ, Shi Z, Zhang B. WebGestalt 2019: Gene Set Analysis Toolkit With Revamped UIs and APIs. Nucleic Acids Res (2019) 47(W1):W199–205. doi: 10.1093/nar/gkz401
23. Szklarczyk D, Gable AL, Lyon D, Junge A, Wuder S, Simonovic M, et al. STRING V11: Protein-Protein Association Networks With Increased Coverage, Supporting Functional Discovery in Genome-Wide Experimental Datasets. Nucleic Acids Res (2019) 47(D1):D607–13. doi: 10.1093/nar/gky1131
24. Bader GD, Hogue CW. An Automated Method for Finding Molecular Complexes in Large Protein Interaction Networks. BMC Bioinf (2003) 4:2. doi: 10.1186/1471-2105-4-2
25. Jiang Y, Li T, Liang X, Hu Y, Huang L, Liao Z, et al. Association of Adjuvant Chemotherapy With Survival in Patients With Stage II or III Gastric Cancer. JAMA Surg (2017) 152(7):e171087. doi: 10.1001/jamasurg.2017.1087
26. Heagerty PJ, Lumley T, Pepe MS. Time-Dependent ROC Curves for Censored Survival Data and a Diagnostic Marker. Biometrics (2000) 56(2):337–44. doi: 10.1111/j.0006-341X.2000.00337.x
27. Wurth L, Gebauer F. RNA-Binding Proteins, Multifaceted Translationalregulators in Cancer. Biochim Biophys Acta (2015) 1849:881–6. doi: 10.1016/j.bbagrm.2014.10.001
28. Pereira B, Billaud M, Almeida R. RNA-Binding Proteins in Cancer:Old Players and New Actors. Trends Cancer (2017) 3:506–28. doi: 10.1016/j.trecan.2017.05.003
29. Zhao L, Cao J, Hu K, Wang P, Li G, He X, et al. RNA-Binding Protein RPS3 Contributes to Hepatocarcinogenesis by Post-Transcriptionally Up-Regulating SIRT1. Nucleic Acids Res (2019) 47(4):2011–28. doi: 10.1093/nar/gky1209
30. Soni S, Anand P, Padwad YS. MAPKAPK2: The Master Regulator of RNA-Binding Proteins Modulates Transcript Stability and Tumor Progression. J Exp Clin Cancer Res (2019) 38(1):121. doi: 10.1186/s13046-019-1115-1
31. Berggren K, Cruz SR, Hixon MD, Cowan A, Ozbun MA, Keysar S, et al. Inhibition of MK2 Decreases Inflammatory Cytokine Production and Tumor Volumes in HPV-Positive and HPV-Negative Models of Head and Neck Squamous Cell Carcinoma. Int J Radiat Oncol Biol Phys (2018) 100:1372–3. doi: 10.1016/j.ijrobp.2017.12.169
32. Jiang Y, Cao W, Wu K, Qin X, Wang X, Li Y, et al. LncRNA LINC00460 Promotes EMT in Head and Neck Squamous Cell Carcinoma by Facilitating Peroxiredoxin-1 Into the Nucleus. J Exp Clin Cancer Res (2019) 38(1):365. doi: 10.1186/s13046-019-1364-z
33. Li W, Gao LN, Song PP, You CG. Development and Validation of a RNA Binding Protein-Associated Prognostic Model for Lung Adenocarcinoma. Aging (Albany NY) (2020) 12(4):3558–73. doi: 10.18632/aging.102828
34. Hu Q, Peng J, Chen X, Li H, Song M, Cheng B, et al. Obesity and Genes Related to Lipid Metabolism Predict Poor Survival in Oral Squamous Cell Carcinoma. Oral Oncol (2019) 89:14–22. doi: 10.1016/j.oraloncology.2018.12.006
35. Liu S, Shi L, Yang X, Wang T, Dong C, Guo W, et al. Nuclear Survivin Promoted by Acetylation Is Associated With the Aggressive Phenotype of Oral Squamous Cell Carcinoma. Cell Cycle (2017) 16(9):894–902. doi: 10.1080/15384101.2017.1310352
36. Li M, Gao F, Yu X, Zhao Q, Zhou L, Liu W, et al. Promotion of Ubiquitination-Dependent Survivin Destruction Contributes to Xanthohumol-Mediated Tumor Suppression and Overcomes Radioresistance in Human Oral Squamous Cell Carcinoma. J Exp Clin Cancer Res (2020) 39(1):88. doi: 10.1186/s13046-020-01593-z
37. Luo X, Donnelly CR, Gong W, Heath BR, Hao Y, Donnelly LA, et al. HPV16 Drives Cancer Immune Escape via NLRX1-Mediated Degradation of STING. J Clin Invest (2020) 130(4):1635–52. doi: 10.1172/JCI129497
38. Wu Y, Chen H, Chen Y, Qu L, Zhang E, Wang Z, et al. HPV Shapes Tumor Transcriptome by Globally Modifying the Pool of RNA Binding Protein-Binding Motif. Aging (Albany NY) (2019) 11(8):2430–46. doi: 10.18632/aging.101927
39. Uchida Y, Chiba T, Kurimoto R, Asahara H. Post-Transcriptional Regulation of Inflammation by RNA-Binding Proteins via Cis-Elements of mRNAs. J Biochem (2019) 166(5):375–82. doi: 10.1093/jb/mvz067
40. Zhang Y, Yu C. Prognostic Characterization of OAS1/OAS2/OAS3/OASL in Breast Cancer. BMC Cancer (2020) 20(1):575. doi: 10.1186/s12885-020-07034-6
41. Li Y, Si L, Zhai Y, Hu Y, Hu Z, Bei J, et al. Genome-Wide Association Study Identifies 8p21.3 Associated With Persistent Hepatitis B Virus Infection Among Chinese. Nat Commun (2016) 7:11664. doi: 10.1038/ncomms11664
42. Gonzalez-Sanchez L, Cobos-Fernandez MA, Lopez-Nieva P, Villa-Morales M, Stamatakis K, Cuezva JM, et al. Exploiting the Passenger ACO1-Deficiency Arising From 9p21 Deletions to Kill T-Cell Lymphoblastic Neoplasia Cells. Carcinogenesis (2020) 41(8):1113–22. doi: 10.1093/carcin/bgz185
43. Pio R, Zudaire I, Pino I, Castaño Z, Zabalegui N, Vicent S, et al. Alpha CP-4, Encoded by a Putative Tumor Suppressor Gene at 3p21, But Not its Alternative Splice Variant Alpha CP-4a, Is Underexpressed in Lung Cancer. Cancer Res (2004) 64(12):4171–9. doi: 10.1158/0008-5472.CAN-03-2982
44. Schudrowitz N, Takagi S, Wessel GM, Yajima M. Germline Factor DDX4 Functions in Blood-Derived Cancer Cell Phenotypes. Cancer Sci (2017) 108(8):1612–9. doi: 10.1111/cas.13299
45. Ogorodnikov A, Levin M, Tattikota S, Tokalov S, Hoque M, Scherzinger D, et al. Transcriptome 3’end Organization by PCF11 Links Alternative Polyadenylation to Formation and Neuronal Differentiation of Neuroblastoma. Nat Commun (2018) 9(1):5331. doi: 10.1038/s41467-018-07580-5
46. Wang J, Wang Y, Kong F, Han R, Song W, Chen D, et al. Identification of a Six-Gene Prognostic Signature for Oral Squamous Cell Carcinoma. J Cell Physiol (2020) 235(3):3056–68. doi: 10.1002/jcp.29210
47. Tan YS, Sansanaphongpricha K, Xie Y, Donnelly CR, Luo X, Heath BR, et al. Mitigating SOX2-Potentiated Immune Escape of Head and NeckSquamous Cell Carcinoma With a STING-Inducing Nanosatellite Vaccine. Clin Cancer Res (2018) 24(17):4242–55. doi: 10.1158/1078-0432.CCR-17-2807
Keywords: oral squamous cell carcinoma, RNA-binding proteins, prognostic model, bioinformatic tools, nomogram
Citation: Lu Y, Yan Y, Li B, Liu M, Liang Y, Ye Y, Cheng W, Li J, Jiao J and Chang S (2021) A Novel Prognostic Model for Oral Squamous Cell Carcinoma: The Functions and Prognostic Values of RNA-Binding Proteins. Front. Oncol. 11:592614. doi: 10.3389/fonc.2021.592614
Received: 07 August 2020; Accepted: 08 June 2021;
Published: 30 July 2021.
Edited by:
Lei Jin, The University of Newcastle, AustraliaReviewed by:
Wenyu Wang, Sun Yat-Sen University, ChinaQihan Dong, The University of Sydney, Australia
Copyright © 2021 Lu, Yan, Li, Liu, Liang, Ye, Cheng, Li, Jiao and Chang. This is an open-access article distributed under the terms of the Creative Commons Attribution License (CC BY). The use, distribution or reproduction in other forums is permitted, provided the original author(s) and the copyright owner(s) are credited and that the original publication in this journal is cited, in accordance with accepted academic practice. No use, distribution or reproduction is permitted which does not comply with these terms.
*Correspondence: Shaohai Chang, Changshaoh@126.com; Jinsong Li, lijinsong1967@163.com; Jiuyang Jiao, 395481252@qq.com
†These authors have contributed equally to this work