Bioinformatic Prediction and Characterization of Proteins in Porphyra dentata by Shotgun Proteomics
- 1Key Laboratory of Aquatic Product Processing, Ministry of Agriculture and Rural Affairs, National R&D Center for Aquatic Product Processing, South China Sea Fisheries Research Institute, Chinese Academy of Fishery Sciences, Guangzhou, China
- 2College of Food Science and Bioengineering, Tianjin Agricultural University, Tianjin, China
- 3Co-innovation Center of Jiangsu Marine Bio-Industry Technology, Jiangsu Ocean University, Lianyungang, China
- 4Collaborative Innovation Center of Seafood Deep Processing, Dalian Polytechnic University, Dalian, China
Porphyra dentata is an edible red seaweed with high nutritional value. It is widely cultivated and consumed in East Asia and has vast economic benefits. Studies have found that P. dentata is rich in bioactive substances and is a potential natural resource. In this study, label-free shotgun proteomics was first applied to identify and characterize different harvest proteins in P. dentata. A total of 13,046 different peptides were identified and 419 co-expression target proteins were characterized. Bioinformatics was used to study protein characteristics, functional expression, and interaction of two important functional annotations, amino acid, and carbohydrate metabolism. Potential bioactive peptides, protein structure, and potential ligand conformations were predicted, and the results suggest that bioactive peptides may be utilized as high-quality active fermentation substances and potential targets for drug production. Our research integrated the global protein database, the first time bioinformatic analysis of the P. dentata proteome during different harvest periods, improves the information database construction and provides a framework for future research based on a comprehensive understanding.
Introduction
As an excellent source of bioactive substances, marine algae possess unique developmental potential owing to the diversity of natural products (1). Porphyra processing, utilization, and health promotion are representative of red algae research; they are mainly distributed in the intertidal and subtidal zones of the ocean. Moreover, they are large, ancient, and rich in protein, polyunsaturated fatty acids, β-carotene, polyphenols, and polysaccharides. They also possess antibacterial, anti-inflammatory, antioxidant, and anti-tumor properties (2–4). With the development of modern biochemistry and molecular biotechnology, Porphyra has been used to develop numerous resources, including drugs, food, cosmetics, bioactive products, etc (5). As a staple food and drug resource, Porphyra dentata (P. dentata) is widely cultivated in East Asia. The total production output of China, Japan, and South Korea has reached more than 95% of the world (6, 7). It also contains numerous amino acids and carbohydrates and is considered a high-quality food supplement and dietary source (8–10).
In P. dentata, amino acids and carbohydrate quality are the criteria for nutrient value of intensive processing. In previous studies, plants were subjected to abiotic stress during growth, and produce molecular metabolites with biological functions and health promotion characteristics. Amino acid and carbohydrate metabolism protein can be used as potential active health components. They are not only pivotal to the expression of structural composition and functional characteristics but also are important reactants of plant life activities (11–13). Porphyra proteins and their bioactive peptides play an important role in the metabolic function of organisms and human health. They have dietary and pharmaceutical activities and can promote the regulation of body function (14). Although correlations between structural and functional properties have not been fully determined, the use of bioinformatic characterizations and bioactive peptide databases such as APD3 and BIOPepDB can improve the analysis and exploration of the physical and chemical properties of bioactive peptides (15, 16). But the harvest period and growing cycle of P. dentata remain difficult and uncertain for development, utilization, and intensive processing (17).
Omics analysis is used to obtain unique nutrients and potential health promotion functions (18). Proteomics is a powerful approach used for protein identification and analysis in Porphyra (19, 20). In the label-free proteomics method, a mixture of protein samples was digested by proteases (trypsin) and quantitative information from peptides is obtained and analyzed by liquid chromatography-tandem mass spectrometry (LC-MS/MS). Then, the peptide sequences are loaded into a database program to search and identify matching proteins (21). Label-free proteomics is widely used to predict and identify unknown proteins and peptides with complete matching numbers. This method has high sensitivity and wide application and is suitable for analyzing algal research with small molecular weight proteins (22). In a recent study, the label-free proteomic analysis revealed specific changes in muscle proteins in pike eel (Muraenesox cinereus) under cold stress and freshness-related proteins in sea bass (Lateolabrax japonicus) filets, during cold storage; however, no reports are available on protein changes at different harvest periods in seaweeds (23, 24).
This is the first study employing label-free shotgun proteomics to comprehensively identify the proteome of P. dentata during different harvest periods. Moreover, different bioinformatics program repositories were used to characterize amino acid and carbohydrate metabolism protein in first and fifth harvest co-expression, and explored potential bioactive peptides, providing new understanding in the study of P. dentata.
Materials and Methods
Chemicals and Reagents
Iodoacetamide (IAM), and triethylammonium bicarbonate buffer (TEAB) were purchased from Sigma (St. Louis, MO, United States). Sodium dodecyl sulfate (SDS) and acetone were obtained from Sinopharm Chemical Reagent (Shanghai, China). Coomassie brilliant blue staining solution (Beyotime, China) and sequencing modified trypsin (mass spectrometry grade) were sourced from Promega (Madison, WI, United States). Oasis® HLB 96-Well Plate 30 μm and Oasis® MCX μ Elution Plate 30 μm were from Waters (Milford, MA, United States). Protein ladder, Pierce™ BCA (bicinchoninic acid) protein assay kit, Halt™ Protease inhibitor cocktail, NUPAGE™ 12% BT GEL 1.0MM 12W, Bond-Breaker TCEP solution [Tris(2-carboxyethyl) phosphine hydrochloride], Pierce quantitative colorimetric peptide assay were obtained from Thermo Fisher Scientific (United States). All other chemicals were reagent/analytical grade.
Porphyra dentata Samples
First, third, and fifth harvests of P. dentata were obtained from Shen’ao Bay (23.46°N, 117.09°E), Nan’ao island, Shantou City, Guangdong Province, China, in December 2020, with each harvest having two biological replicates. After collection, they were cleaned with sterilized water to remove sediment, appressorium, and other impurities, placed in sterile sealed tubes, and stored in a −80°C ultra-low temperature freezer after transportation by a liquid nitrogen container.
Total Protein Extraction and Sodium Dodecyl Sulfate-Polyacrylamide Gel Electrophoresis
The samples were ground in liquid nitrogen and BPP [borax, polyvinylpolypyrroli-done (PVPP), and phenol] mixed in a 1:10 ratio. The suspensions were centrifuged at 12,000 × g and 4°C for 20 min and the supernatants were collected. An equal volume of Tris-saturated phenol was added to each supernatant and each mixture was vortexed at 4°C for 10 min. The mixtures were centrifuged at 12,000 × g and 4°C for 20 min and the phenol phases were collected. An equal volume of BPP was added to each supernatant and the mixtures were vortexed at 4°C for 10 min. The solutions were centrifuged at 12,000 × g and 4°C for 20 min and the phenol phases were collected. Five times volumes of pre-cooled 0.1M ammonium acetate in methanol were added and the proteins were precipitated at −20°C overnight. The supernatants were discarded by centrifugation and the precipitates were washed twice with 90% (v/v) acetone. The supernatants were discarded by centrifugation and the precipitates were air-dried. The precipitates were re-suspended in lysis buffer [1% (v/v) SDS plus 8M urea] and sonicated on ice for 3 min. The lysates were centrifuged, and the supernatants were collected to determine the protein content in a BCA Protein Assay Kit (Thermo Fisher Scientific, United States).
The NuPAGE™ 12% BIS-Tris Protein Gels electrophoresis gel (Thermo Fisher Scientific, United States) was removed from the packaging and bottom seal and fixed in the electrophoresis tank. Sodium dodecyl sulfate-polyacrylamide gel electrophoresis (SDS-PAGE) electrophoresis buffer (1×) was added. The samples were prepared by mixing 4 μL of protein sample and 1 μL of sample loading buffer solution. After fully mixing, the samples were heated at 100°C for 3–5 min. After the protein was fully denatured, the samples were loaded, and the voltage was set to 200 V for 45 min. The results were observed and photographed by an automatic digital gel image analyzer.
Protein Digestion, Peptide Desalination, and Quantification
Protein digestion was performed according to the standard procedure. Briefly, for each sample tube containing 150 μg protein, appropriate TCEP was added to achieve a final concentration of 10 mol/L and the tubes were incubated at 37°C for 60 min. Appropriate IAM was added to the final concentration of 40 mM and reaction for 40 min in dark. Six volumes of cold acetone as added to the sample tube. The tube was inverted three times and incubated at −20°C until a precipitate formed, which was approximately 4 h. The acetone was removed by centrifugation at 10,000 g for 20 min and the precipitated protein was resuspended with 150 μL 100 mol/L TEAB buffer. To each sample tube, a 1:50 proportion ratio of sample to trypsin solution was added and incubated at 37°C overnight.
The peptides were vacuum-dried and resuspended in 2% (v/v) acetonitrile and 0.1% (v/v) trifluoroacetic acid (TF). The samples were desalted with Sep-Pak and vacuum-dried. The peptide concentrations were determined using a Peptide Quantification Kit (Thermo Fisher Scientific, United States). Loading buffer was added to each tube to prepare the samples for mass spectrometry (MS) analysis. The concentration of each sample was 0.5 μg/μL.
Shotgun Liquid Chromatography-Tandem Mass Spectrometry Analysis
Peptides were dissolved using a mass spectrometry loading buffer and LC-MS/MS analysis was performed. The peptide samples were separated by the EASY-nLC 1200 liquid system, and the chromatographic column was a C18 column (75 μm × 25 cm, Thermo, United States). Mobile phase A was 2% acetonitrile and 0.1% formic acid, and mobile phase B was 80% acetonitrile and 0.1% formic acid. The separation gradient was 0–2 min and mobile phase B increased linearly from 0 to 6%; when the separation gradient was 2–105 min, mobile phase B linearly increased from 6 to 23%; when the separation gradient was 105–130 min, mobile phase B linearly increased from 23 to 29%; when the separation gradient was 130–147 min, mobile phase B linearly increased from 29 to 38%; when the separation gradient was 147–148 min, mobile phase B linearly increased from 38 to 48%; when the separation gradient was 148–149 min, mobile phase B linearly increased from 48 to 100%; when the separation gradient was 149–155 min, mobile phase B linearly was maintained at 100%. Using Q-Exactive HF-X (Thermo, United States) for mass spectrometry analysis, MS scanning range was 350–1 300 (m/z), acquisition mode was DDA, fragmentation mode was HCD, resolution of the primary mass spectrometer was 70,000, and resolution of secondary was 17,500. A total of four replicates (n = 4) were analyzed independently.
Sequence Database Searching
Using Proteome Discoverer™ Software 2.2 (Thermo Fisher Scientific, United States) (25), MS/MS spectra were searched against the database. The highest score for a given peptide mass (best match to that predicted in the database) was used to identify parent proteins. The parameters for protein searches were set as follows: tryptic digestion with up to two missed cleavages, carbamidomethylation of cysteine as a fixed modification, and oxidation of methionine and protein N-terminal acetylation as variable modifications. Peptide spectral matches were validated based on q-values at a 1% false discovery rate (FDR).
Bioinformatics Analysis
The gene ontology (GO) database1 and Kyoto Encyclopedia of Genes and Genomes (KEGG) pathway database2 were used to perform GO function annotations and participate in metabolic pathway analysis of proteins to obtain information about biological functions, biological processes involved, cell location, functional domain analysis using Pfam3 position (14).
Network Analysis and Sequence Similarities
Network analysis was performed by submitting the protein dataset to STRING (Search Tool for the Retrieval of Interacting Genes) software (version 11.5).4 This database is known and used to predict protein interactions. Proteins were represented with nodes and their interactions with continuous lines to represent direct physical interactions, while indirect interactions and functional information were presented by interrupted lines. Utilizing the string software, we minimized false positives as well as false negatives, tagged all interactions as “Medium-confidence” (<0.4), and eliminated them from the analysis. Proteins not recorded were removed from the database. Cluster networks were created using the MCL inflation algorithm, which is included in the STRING search tool and a value of three was selected for all the analyses (26).
Sequence similarities of identified amino acid and carbohydrate Porphyra proteins involved in metabolism were searched using the BLAST program.5 We utilized the NCBI Conserved Domain Search Service6 to discover nucleotide sequences, and Texmaker to draw sequence alignment diagrams (27).
Potential Bioactive Peptide Prediction
Potential bioactive peptides were performed in silico using the MS-Digest software, which is included in Protein Prospector (version 6.3.1)7 (28). Pepsin and trypsin enzymes are commonly chosen. ‘‘Per peptide’’ with a minimum of six residues and no missed cleavages, were selected as parameters. To evaluate the results, all digested peptides were ranked using the Peptide Ranker.8 This is a prediction of bioactive peptides tool based on a novel N-to-1 neural network (29). Any peptide predicted with an overtop score is labeled as bioactive for the objective protein. In addition, potential peptides were searched against CAMP9 to identify antibacterial properties and Toxin Pred10 was used to identify high toxic or non-toxic peptides (30, 31). Finally, the Swiss-Model serve11 was used to create 3D models and predict bioactive ligand mechanisms (32).
Results and Discussion
Identification of Porphyra dentata Proteome by Label-Free Proteomic Analysis
Based on LC-MS/MS and Proteome Discoverer analyses, a trypsin digestion search of protein extracts from P. dentata was performed. The repository stores 288,272 identification profiles from 13,046 different peptides and 2,187 different non-redundant annotated proteins with different sample repeats (n = 6). Results showed that the molecular weight of proteins was concentrated in the 1–21 and 21–41 k Da range, and were mostly small molecular proteins; most peptides’ length distribution was 10–15 bp. both the first and fifth harvest co-expression resulted in 419 non-redundant annotated proteins (n = 4). To further observe and compare protein extraction quality and characteristic distribution of the two harvests, whole protein extracts were separated by 12% SDS-PAGE electrophoresis, with two biological repetitions for each harvest period (Figure 1). The results showed that protein mass for each harvest was evenly distributed.
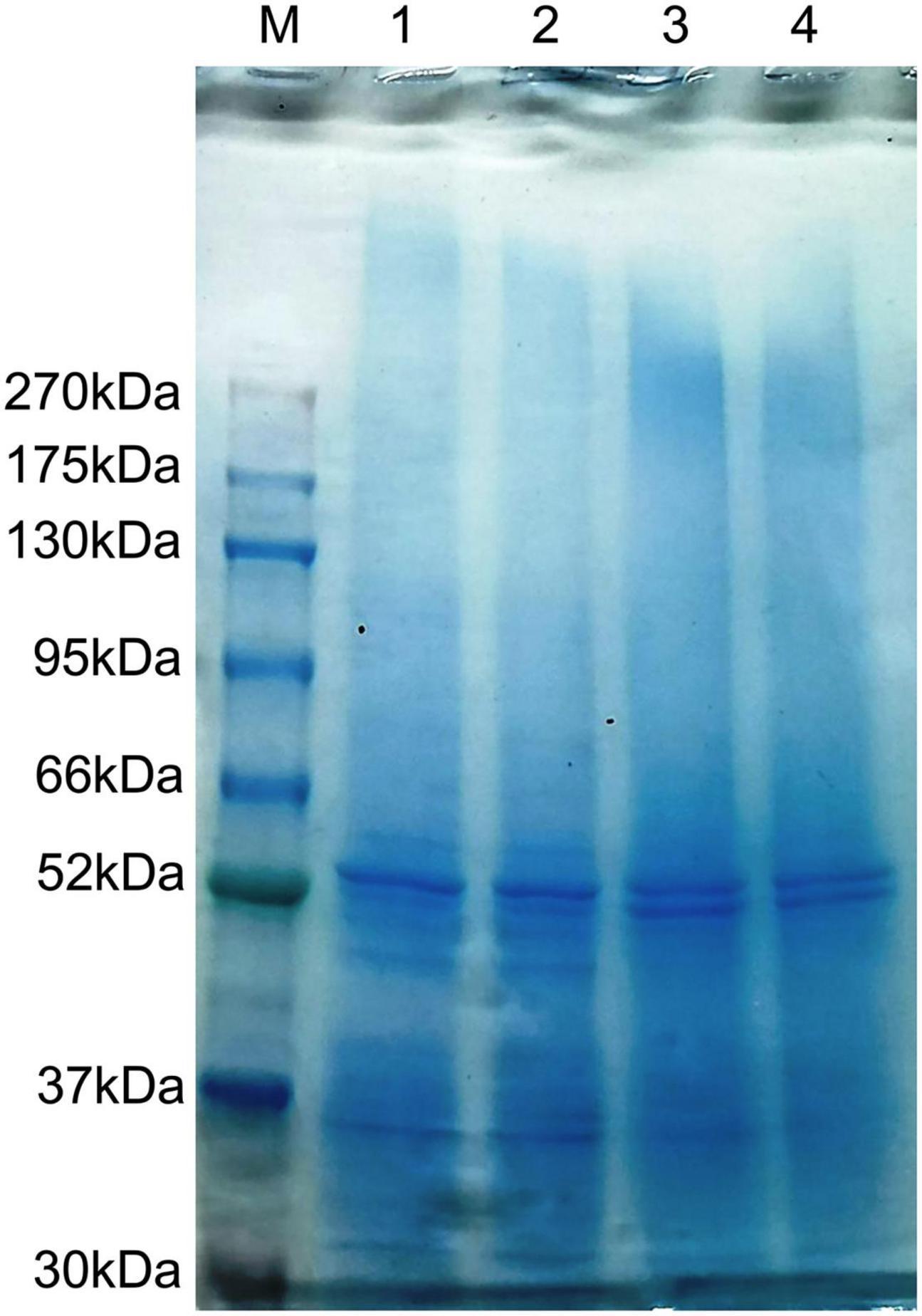
Figure 1. Sodium dodecyl sulfate-polyacrylamide gel electrophoresis 12% proteins of the Porphyra dentata samples (M, marker; 1–2, first harvest; 3–4, fifth harvest).
Through repository matching, we found less protein information and more species annotation, including proteins related to other red algae such as Pyropia yezoensis and Porphyra umbilicalis. In the repository, 77 labeled and unnamed protein products appeared and were annotated as Chondrus crispus, which has developmental similarities to P. dentata. In addition, there are 88 proteins that are not applicable (N/A). Further research should consider the limitations and characteristics of organisms sequencing, providing database updated accordingly. Supplementary Table 1 shows the complete annotated protein information. The raw data and analysis outputs (including all protein abundance) are publicly available at iProX data repository12 (Reference: PXD033319).
Functional Analysis: Gene Ontologies and Pathways Analysis
Go analysis was carried out on 419 annotated co-expression proteins of P. dentata; a total of 28 results were counted (Figure 2A). In the biological process, proteins are mainly enriched in metabolic (GO: 0008152, 94), cellular (GO: 0009987, 93), and single organizational pathways (Go: 0044699, 50). Cellular components consist of the cell (GO: 0005623, 70), cellular compartments (GO: 00044464, 68), and organelles (GO: 0043226, 49). Catalytic activity (GO:0003824, 103) and binding (GO:0005488, 69) are the main molecular function. The KEGG pathway showed that most identified proteins are involved in amino acid (17), carbohydrate (24), and energy metabolism (23). Identified proteins also participate in protein translation (23), folding, sorting, degradation, and genetic information processing (Figure 2B).
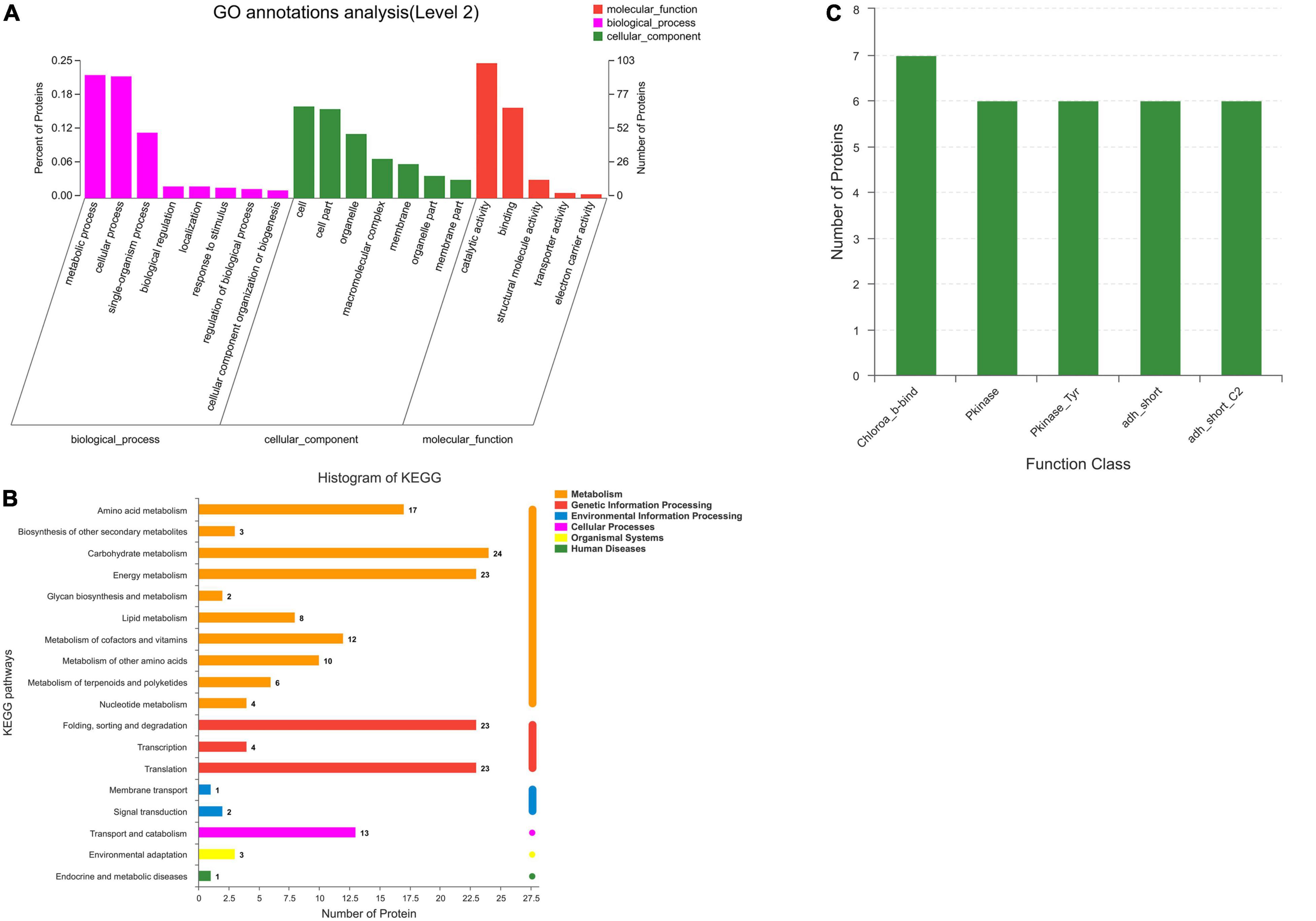
Figure 2. GO, KEGG pathway and function class analysis of Porphyra dentata. (A) Protein classes of Porphyra dentata by GO. (B) KEGG pathway analysis of Porphyra dentata. (C) Function class analysis of Porphyra dentata.
Moreover, the functional domain of P. dentata was studied and analyzed by Pfam software. Sequence functional regions in protein annotation were focused on chlorophyll A-B binding protein (Chloroa_b-bind); protein kinase domain (Pkinase); protein tyrosine kinase (Pkinase_Tyr); short-chain dehydrogenase (adh_short); enoyl-(Acyl carrier protein); reductase (adh_short_C2). Figure 2C shows the location number. Most of these domains are classified as proteases and may be related to quality differences in P. dentata.
Interactive Network Analysis and Sequence Similarities
Proteins involved in amino acid and carbohydrate metabolism from KEGG are summarized in Tables 1, 2. In STRING software network interaction analysis. P. dentata was not annotated in the software and not Auto-detect was not supported. According to the database search results, C. crispus was selected as a reference for the biological pattern analysis. Based on MCL clustering (MCL = 3), 32 nodes (proteins) and 37 edges (interactions) were obtained. The predicted network interaction was 10 and a significant increase in the number of edges may be attributed to increased protein expression and biological activity in P. dentata (Figure 3). Physical direct interactions are represented by continuous lines, while functional interactions are represented by discontinuous lines. In carbohydrate metabolism, have a protein indicated as N/A, no annotation information will be discussed temporarily.
The interaction analysis shows nine different sub-networks, with enriched color annotations focused on four aspects. Red stands for the metabolic process of histidine, isoleucine, and the alpha-amino acid biosynthetic process. Brown is rumen kinase activity. In addition, dark golden-red is described and mixed, including the poly metallic process, and serine-type exercise activity. Green is glutathione metabolism and carbon metabolism, and they participate in endoplasmic reticulum protein processing, induce synthesis of specific and differential proteins, stimulate unique biological activities, and induce the formation of active peptides in P. dentata.
Other molecular networks such as phosphoglycerate kinase, annotated in Cyan, are a major protein involved in the ATP production stage of glycolysis and concerted catalysis with pyruvate kinase. Our previous study found that ATP could significantly affect the quality of Porphyra while harvesting during photosynthesis. Thus, the overall quality of P. dentata results in protein and carbohydrate changes, as well as flavor differences (17). During the first and fifth harvest of P. dentata, this network is the first and most comprehensive interactomics map of interactions between amino acids and carbohydrate metabolism functional substances involved (Supplementary Table 2 for full-color annotation).
To further analyze the biological functions of annotated proteins, the Porphyra biological information resource library was examined, and annotated proteins were screened out as P. yezoensis. We compared proteins’ active functional domains identified from amino acid sequences. Results showed that the most abundant functional domains in the target protein were transketolase (A) and trehalose-6-phosphate synthase (B); functional domains, respectively, were 72–731 and 76–850. The first layer was homologous closest NeoPyropia yezoensis protein and the second layer was our target protein in Figure 4 (Other small domains protein figure in Supplementary Figure 1). Trehalose-6-phosphate synthase (T6P) is essential for carbohydrate synthesis in plants (33). Previous studies found that T6P promoted the growth process and biosynthesis of the final substances in plant starch and promoted growth recovery after abiotic stress (34). Our study confirmed that with the gradual extension of harvest time, excitation of specific proteins reduced the production of proteins involved in photoinhibition and ensured stable the photosynthesis cycle. P. dentata gradually darkened, thickened, increased carbohydrate content, and decreased protein content (17). In addition, T6P can increase fatty acid synthesis and accumulation in Porphyra tissues, which was consistent with our results (determination not published). The amino acid content of the fifth harvest was higher than the first harvest.
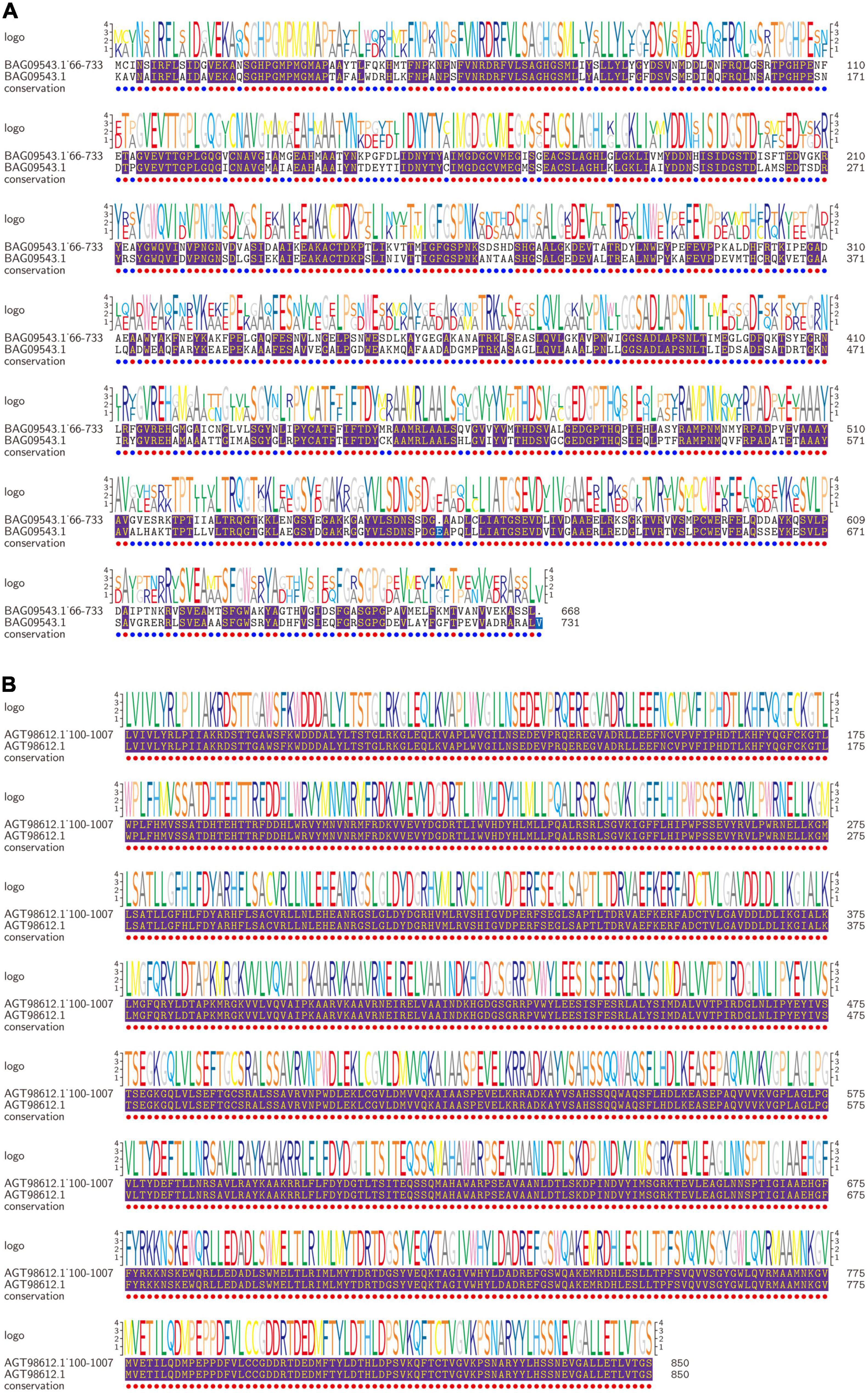
Figure 4. Proteins amino acid residues of predicted motifs comparison. The first layer is NeoPyropia protein, and second layer is target protein. The purple part is sequence homology (A: Transketolase; B: Trehalose-6-phosphate synthase).
Prediction of Bioactive Peptides
Bioactive peptides are specific protein fragments that positively affect and regulate human functions and their characteristics are determined by amino acid composition. They can be released and become active to function as enzymes and improve both human immunity sources and the quality of functional food to provide nourishment (35, 36). Proteins were hydrolyzed with pepsin and trypsin in the MS digest in silico program, predicted peptides for each enzymatic hydrolysis are shown in Supplementary Table 3 (pepsin and trypsin). Pepsin cleavage sites P1 and P1′, primarily cleave proteins on Phe, Tyr, Trp, and Leu residues (37). A total of 1,271 and 2,191 different polypeptides were released from proteins involved in amino acid and carbohydrate metabolism (5–31 amino acid residues).
We utilized Peptide Ranker to sequence hydrolyzed peptides for each protein and select the peptide with the greatest biological activity. Referring to the Pan method (14), peptides with the highest Peptide Ranker score are considered potential bioactive peptides for the target protein (Table 3 is amino acid metabolism, Table 4 is carbohydrate metabolism). Based on the analysis, we found that the Alanine transaminase protein cannot be characterized by Amino acid metabolism. The putrescine amino propyltransferase protein predicted high peptide scores but was toxic. Finally, an unrecorded protein was characterized in carbohydrate metabolism and the presumed active protein was unique to P. dentata, which is not listed in the table.
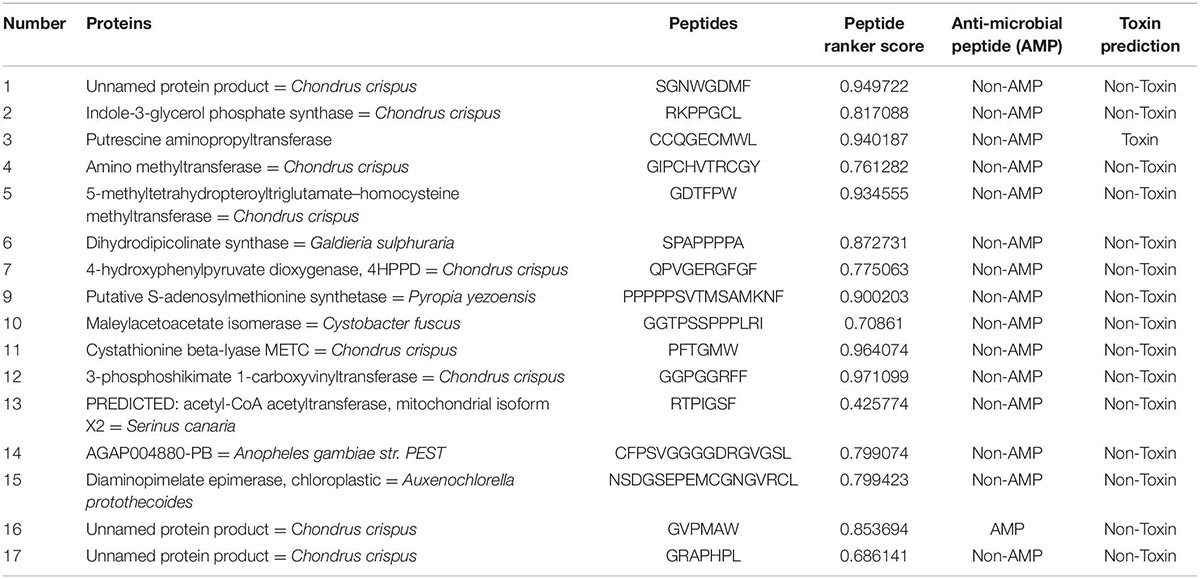
Table 3. Selected potential bioactive peptides of the amino acid metabolism proteome predicted by in silico digestions with pepsin.
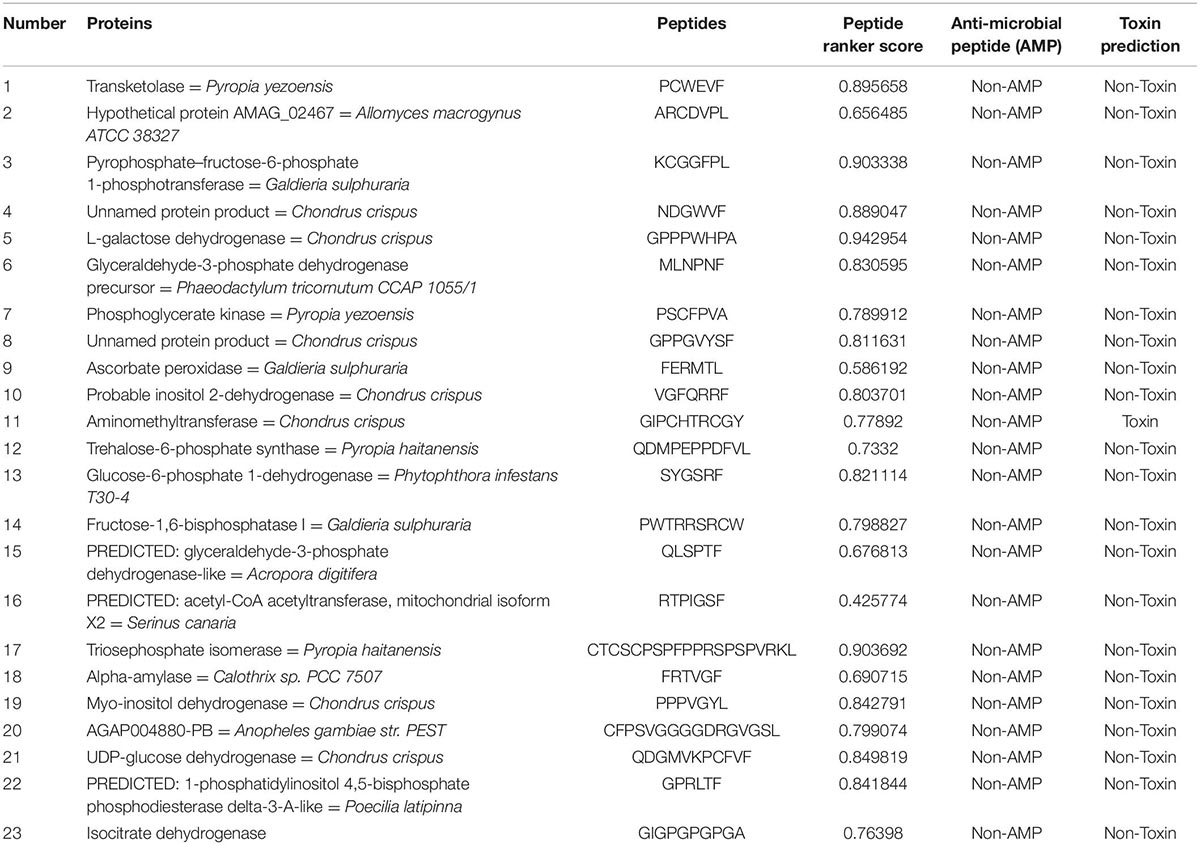
Table 4. Selected potential bioactive peptides of the carbohydrate metabolism proteome predicted by in silico digestions with pepsin.
During trypsin digestion, it was found that Lys and Arg residues at the P1 position of the target protein were preferentially cleaved; however, the P1’ site was involved in the cleavage of pro (14). In total, 1,046 protein peptides were involved in amino acid metabolism and 1,870 protein peptides involved in carbohydrate metabolism were predicted (5–40 amino acid residues). Results from the Peptide Ranker prediction server showed that the activity of 3–phosphokinase–1–carboxyvinyltransferase protein was the highest, with an increased number of peptide toxicity predictions in carbohydrate metabolism proteins and no predicted peptides with antibacterial activity in trypsin digest (Table 5, Amino acid metabolism; Table 6, Carbohydrate metabolism).
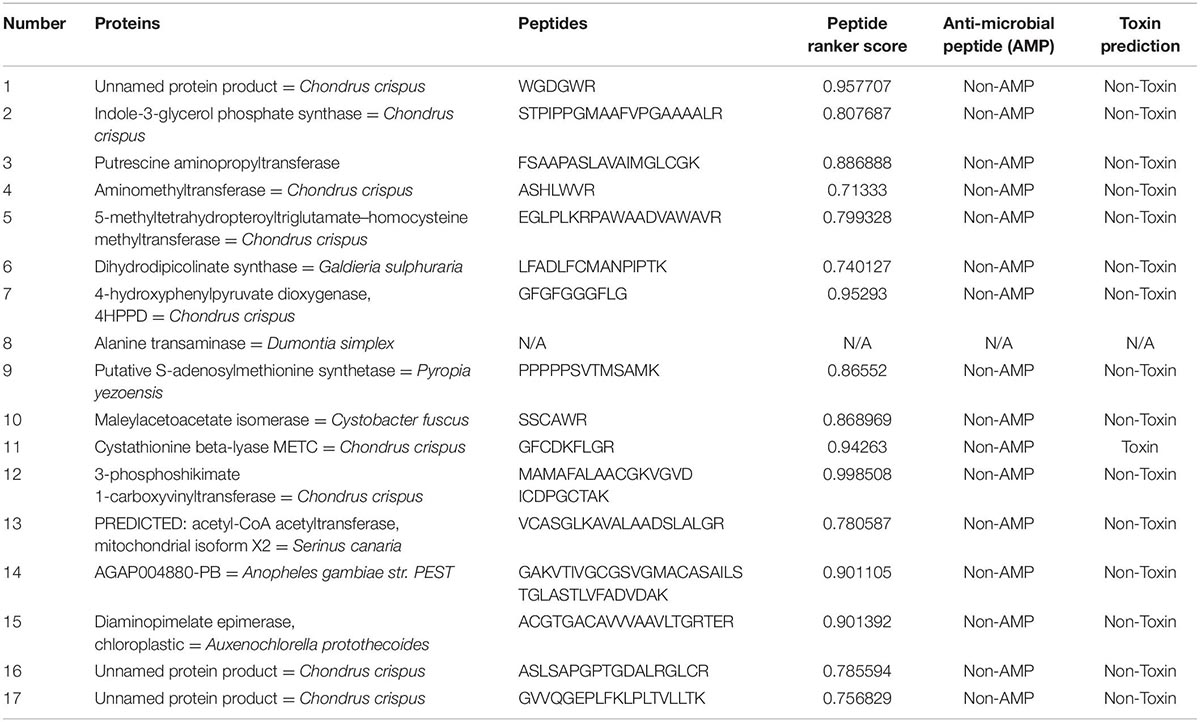
Table 5. Selected potential bioactive peptides of the amino acid metabolism proteome predicted by in silico digestions with trypsin.
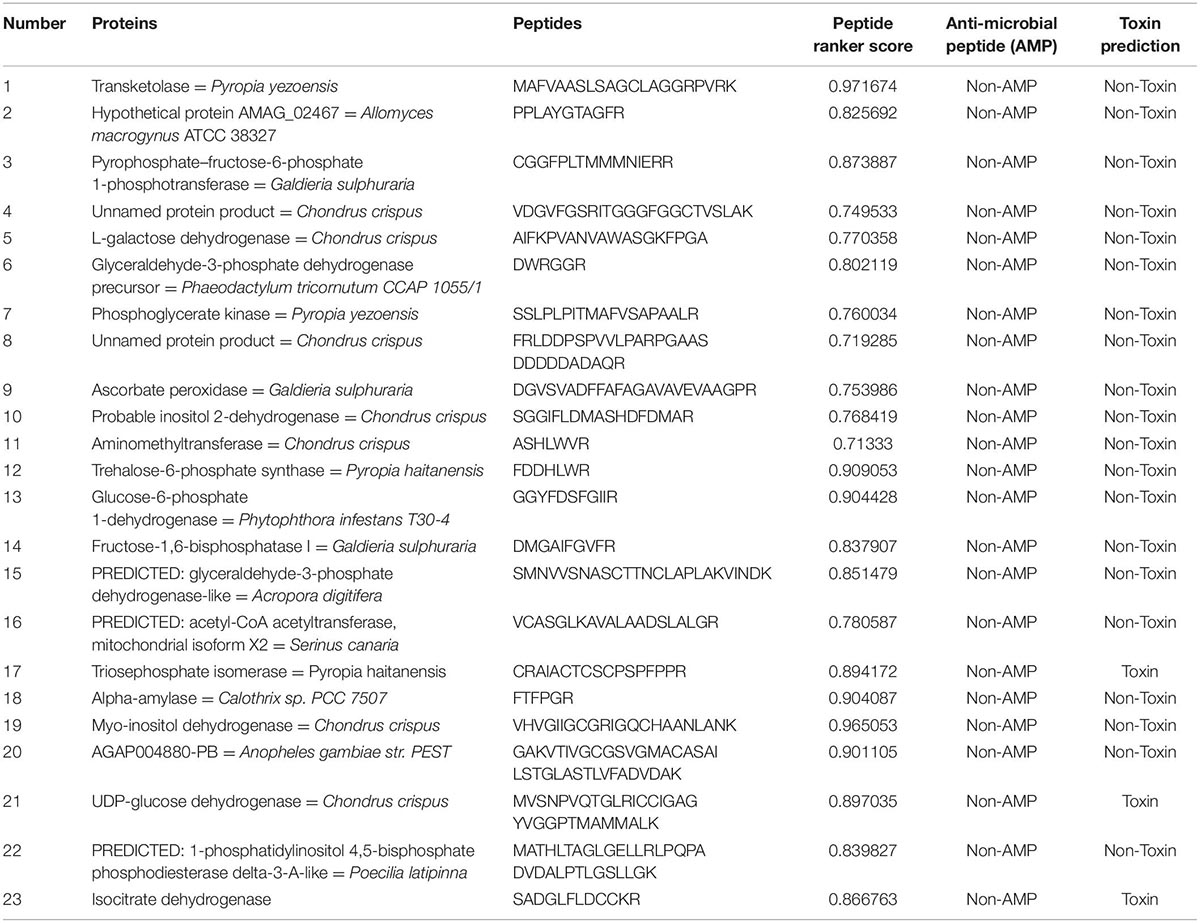
Table 6. Selected potential bioactive peptides of the carbohydrate metabolism proteome predicted by in silico digestions with trypsin.
In Peptide Ranker analysis, peptides with scores higher than 0.9 were considered to have more biological potential activity (38). Peptide 3–phosphokinase–1–carboxyvinyltransferase’s in amino acid metabolism ranker scores reached 0.971099 and 0.998508. A previous study confirmed and identified a potential drug target in Brucella millitensis (16M simulated protein screening library) for the drug treatment of brucellosis (39). Therefore, we identified a potential pepsin/trypsin digest of peptides (GGPGGRFF, MAMAFALAACGKVGVDICDPGCTAK) that may be used as a natural plant preparation in antibacterial research to improve the health and stability of animal-derived foods and drugs.
The predictive peptide scores for Cystathionine beta-lyase MetC are 0.964074 and 0.94263. It is the key factor of methionine synthesis and can be used as a growth regulator or for flavor preparation in food production (40). In terms of lactobacillus fermentation products, volatile sulfur compounds (VSCs) are important substances that help achieve the desired flavor of fermented foods. Cystathionine beta lyase MetC can stimulate cysteine synthesis and improve amino acid metabolism. Presently, Cystathione beta lyase MetC can be inoculated and is preserved as the dominant strain in cheese production (41, 42). In the future, the natural bioactive peptide from P. dentata can be applied to the fermentation of food products, such as yogurt, cheese, and bacon. It can also be used to stimulate diversified applications and development advantages in seafood. Unfortunately, trypsin digestion causes toxicity; therefore, the production and application of this peptide need to be further studied.
In protein structure, the ligand is a signaling molecule and matches specific receptor protein sites on the walls of an organism’s somatic cell. When the ligand binds to the specific protein site, it will change the physical morphology of the protein, and a change in the shape of the receptor protein may activate or inhibit another biological mechanism, which is related to this specific interaction. Protein-ligand interactions are crucial to the creation of new drugs for the treatment of diseases and the development of bioactive substances (43). Here, we used 3D-model constructs to predict the protein structure of 3-phoshikimate-1-carboxyvinyltransferase and cystathionine beta lyase MetC. Predicted peptides are located in the protein structure. The target protein was predicted by Swiss-model. 3-phoshikimate-1-carboxyvinyltransferase protein had the highest matching degree of 1.02-angstrom resolution crystal structure with 3–phosphoshikimate–1–carboxyvinyltransfer from Vibrio cholerae in complex with shikimate-3-phosphate (partially photolyzed) and glyphosate (SMTL ID: 3nvs.1.A). The GMQE score was 0.51 and Seq Identity was 54.85% (Figure 5A). The monomer is similar to 1 × glyphosate and 1 × shikimate-3-phosphate. In P. dentata, a total of 14 residues within 4 Å, correlated with 3nvs.1.A. less A119. The ligand co-expression site of S3P.1 and the lack of site SKM2 are shown in Figures 5B,C. We hypothesized that 3–phosphoshikimate–1–carboxyvinyltransferase protein lacks ligands, but its structural similarity is low. The unique functional domain structure can cooperate with the S3P.1 site to stimulate the creation and expression of biological activity, resulting in a better therapeutic effect.
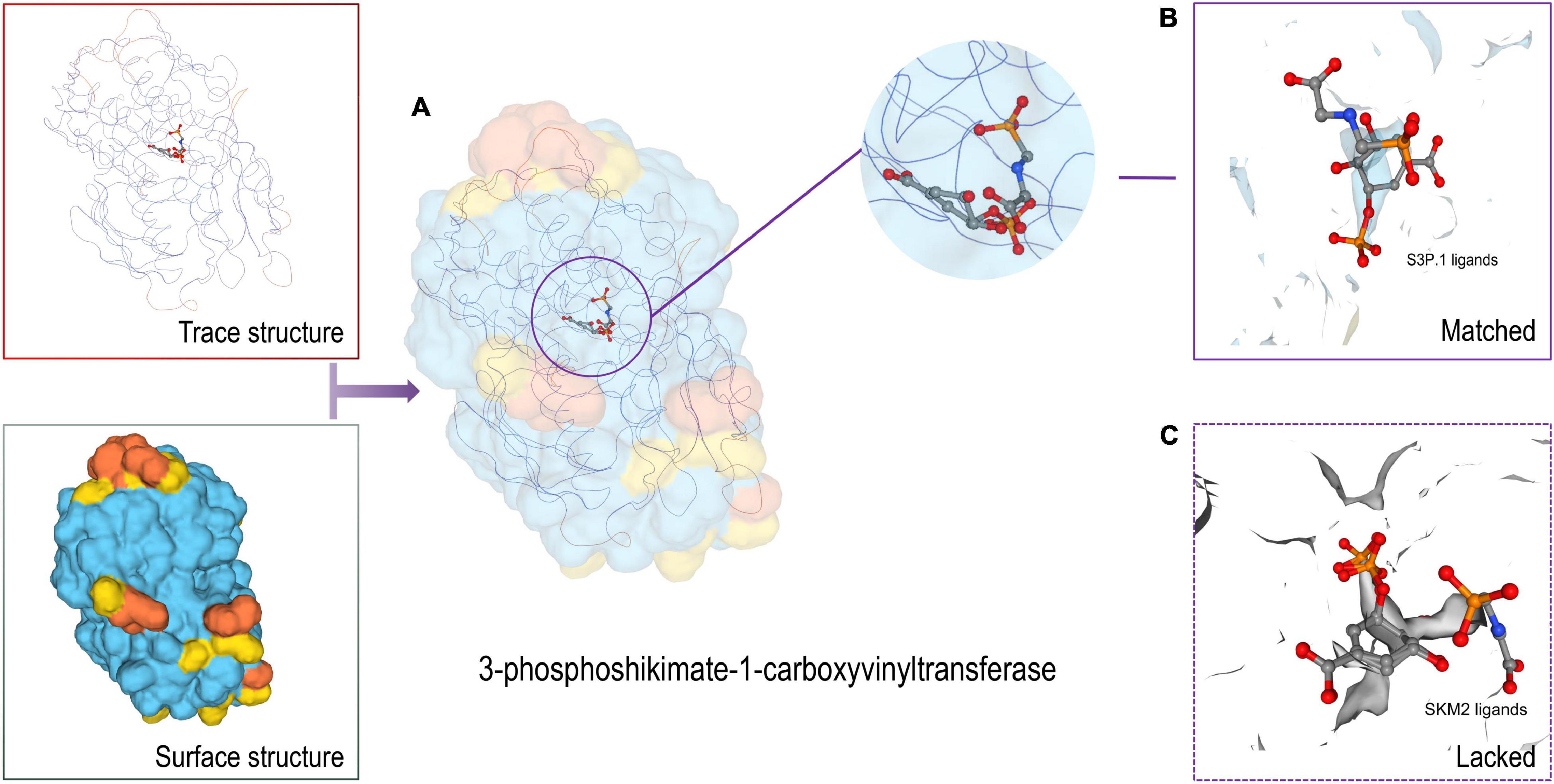
Figure 5. 3D model structure of protein and ligands (A: 3-phosphoshikimate-1-carboxyvinyltransferase; B: S3P.1 ligands; C: SKM2 ligands).
Compared with 3–phosphoshikimate–1–carboxyvinyltransferase, the tertiary structure of Cystathionine beta-lyase METC was obtained by sequence alignment, and the P113S Mutant of E. coli Cystathionine beta-lyase MetC structure (STML ID: 4ITG.1a) was used as a template. The GMQE score was 0.68 and the seq identity was 40.26% (Figure 6). Our previous determination confirmed that amino acid content increased with gradually prolonging the harvest (determination not published), and palate fullness in P. dentata. Unfortunately, no ligand was found in the predicted protein, suggesting that bioactive sites were stored in the protein as a whole. This research focuses on the production of diversified bioactive peptides, enriches sustainable development in the seaweed industry, and highlights the developmental potential of P. dentata an edible seaweed source and bioactive compound.
Others Bioactive Peptides Expectation
In conclusion, active peptide hydrolyzed with trypsin scores better than pepsin in carbohydrate metabolism. In pepsin-predicted peptides, acetyl-CoA acetyltransferase, mitochondrial isoform X2, and Ascorbate peroxidase protein ranker scores are lower than 0.6, with no biological significance (38). It has been reported that most of the active peptides in Porphyra are released by pepsin, but peptides hydrolyzed by trypsin in Porphyra haitanensis have better anti-inflammatory and hypoglycemic activities, which are similar to our prediction results. Carbohydrates can be fully digested, and this approach can be applied to potential drugs and biological additives (44).
Transketolase is an important glycolytic synthase in the pentose phosphate pathway and is involved in oxidative stress processes in cells. Clinical studies confirmed that Transketolase can affect the production of NAPDH, inhibition of oxidative stress, and promote the growth of cancer cells (45). Our data results show that the peptide obtained by trypsin (MAFVAASLSAGCLAGGRPVRK) has a score of 0.971674 and is not classified as an anti-microbial. It can be used as a potential anti-inflammatory and targeted biological regulatory peptide in the treatment of cancer cells or used in the molecular synthesis of antioxidants for cancer research.
Myo-inositol dehydrogenase is the first step in catalyzing the catabolism of inositol. It is closely related to NAD, a homologous biological substance that can be used in the production of medicine and food. VHVGIIGCGRIGQCHAANLANK ranker score is 0.965053. Not only is it used for cheese fermentation, but it has become the dominant strain to improve the activity of fermentation substrates and is also a potential drug for treating Alzheimer’s disease (46, 47).
Finally, the Collection of Anti-Microbial Peptides (CAMP) database showed that only one unnamed protein product [C. Crispus (GVPMAW)] was determined with a potential anti-microbial peptide. Further studies are required because the benefits of P. dentata are yet to be elucidated.
For P. dentata, a synthetic bioactivity analysis is required to discuss and apply potential bioactive peptides. The bioinformatics method provides a faster and lower cost potential prediction method to screen and locate potential targets and provides a macro-analysis and demonstration for development.
Conclusion
In this study, Label-free shotgun proteomics was used for the first time to identify protein changes and characterization of different harvest periods of P. dentata. A total of 13,046 different peptides were identified and 419 co-expression target proteins were obtained. Bioinformatics is used for research, including GO, KEGG, and STRING network interaction analysis. Results showed that metabolic process, cell, and catalytic activity were enrichment in GO and Amino acid metabolism, Carbohydrate metabolism, Energy metabolism, Genetic Folding, sorting, and degradation, and Translation in KEGG most prominent. Squido Jumbo network diagrams of amino acid metabolism and carbohydrate metabolism containing 32 protein interaction nodes were constructed, this is the first time to analyze interaction functional annotation proteins of P. dentata during the first and fifth harvest period and compared with the Porphyra resource library to enrich the expression of information. In addition, pepsin and trypsin were used for different digestion hydrolysis, predicting potential bioactive peptides. The results show that bioactive peptides can be high-quality fermentation active substances and drug activity, are potential targets for production. By combining proteomic results and bioinformatics analysis, we comprehensive understanding of functional changes in different harvest periods of P. dentata, providing a potential development direction for application as a source of food and biomedical compounds.
Data Availability Statement
The original contributions presented in the study are included in the article/Supplementary Material, further inquiries can be directed to the corresponding author.
Author Contributions
MY, LM, and YZ wrote the first draft of the manuscript. XY, LL, and SC wrote sections of the manuscript. BQ, YW, CL, and SY organized data and contributed to visualization. YZ conceptualized the idea. All authors contributed to manuscript revision, read, and approved the submitted version.
Funding
We were grateful for the financial support from the National Key R&D Program of China (2019YFD0901905), the China Agriculture Research System of MOF and MARA (CARS-50), Central Public-interest Scientific Institution Basal Research Fund, CAFS (2020TD73), and Central Public-interest Scientific Institution Basal Research Fund, South China Sea Fisheries Research Institute, CAFS (2021SD07).
Conflict of Interest
The authors declare that the research was conducted in the absence of any commercial or financial relationships that could be construed as a potential conflict of interest.
Publisher’s Note
All claims expressed in this article are solely those of the authors and do not necessarily represent those of their affiliated organizations, or those of the publisher, the editors and the reviewers. Any product that may be evaluated in this article, or claim that may be made by its manufacturer, is not guaranteed or endorsed by the publisher.
Supplementary Material
The Supplementary Material for this article can be found online at: https://www.frontiersin.org/articles/10.3389/fnut.2022.924524/full#supplementary-material
Footnotes
- ^ http://geneontology.org/
- ^ http://www.genome.jp/kegg
- ^ http://pfam.xfam.org/
- ^ http://stringdb.org/
- ^ http://blast.ncbi.nlm.nih.gov/
- ^ https://www.ncbi.nlm.nih.gov/Structure/cdd/wrpsb.cgi
- ^ http://prospector.ucsf.edu/prospector/mshome.htm
- ^ http://distilldeep.ucd.ie/PeptideRanker/
- ^ http://www.bicnirrh.res.in/antimicrobial/
- ^ https://webs.iiitd.edu.in/raghava/toxinpred/multi_submit.php
- ^ http://swissmodel.expasy.org
- ^ https://www.iprox.cn
References
1. Blunt JW, Copp BR, Keyzers RA, Munroa MH, Prinsepd MR. Natural product reports. Nat Prod Rep. (2016) 33:382–431. doi: 10.1039/c6np00124f
2. Aziz E, Batool R, Khan MU, Rauf A, Akhtar W, Heydari M, et al. An overview on red algae bioactive compounds and their pharmaceutical applications. J Complement Integr Med. (2020) 17:20190203. doi: 10.1515/jcim-2019-0203
3. Li Y, Huo Y, Wang F, Wang C, Zhu Q, Wang Y, et al. Improved antioxidant and immunomodulatory activities of enzymatically degraded Porphyra haitanensis polysaccharides. J Food Biochem. (2020) 44:e13189. doi: 10.1111/jfbc.13189
4. Qiu Y, Jiang H, Fu L, Ci F, Mao X. Porphyran and oligo-porphyran originating from red algae Porphyra: preparation, biological activities, and potential applications. Food Chem. (2021) 349:129209. doi: 10.1016/j.foodchem.2021.129209
5. Bito T, Fei T, Watanabe F. Bioactive compounds of edible purple laver Porphyra sp. (Nori). J Agric Food Chem. (2017) 65:10685–92. doi: 10.1021/acs.jafc.7b0468844
6. Niwa K, Iga H, Sato T. Potential of Neoporphyra kitoi (Bangiales, Rhodophyta) as a candidate species for marine crops with high temperature tolerance. Aquaculture. (2022) 548:737650. doi: 10.1016/j.aquaculture.2021.737650
7. Chopin T, Tacon AGJ. Importance of seaweeds and extractive species in global aquaculture production. Rev Fish Sci Aquac. (2021) 29:139–48. doi: 10.1080/23308249.2020.1810626
8. Kazłowska K, Hsu T, Hou C, Yang W, Tsai GJ. Anti-inflammatory properties of phenolic compounds and crude extract from Porphyra dentata. J Ethnopharmacol. (2010) 128:123–30. doi: 10.1016/j.jep.2009.12.037
9. Aung T, Kim SJ, Eun JB. A hybrid RSM-ANN-GA approach on optimisation of extraction conditions for bioactive component-rich laver (Porphyra dentata) extract. Food Chem. (2022) 366:130689. doi: 10.1016/j.foodchem.2021.130689
10. Aung T, Eun JB. Impact of time and temperature on the physicochemical, microbiological, and nutraceutical properties of laver kombucha (Porphyra dentata) during fermentation. LWT. (2022) 154:112643. doi: 10.1016/j.lwt.2021.112643
11. Garcia C, Guerin M, Souidi K, Remize F. Lactic fermented fruit or vegetable juices: past, present and future. Beverages. (2020) 6:8. doi: 10.3390/beverages6010008
12. Du Y, Lu R, Xia J. Impacts of global environmental change drivers on non-structural carbohydrates in terrestrial plants. Funct Ecol. (2020) 34:1525–36. doi: 10.1111/1365-2435.13577
13. Batista-Silva W, Heinemann B, Rugen N, Nunes-Nesi A, Araújo WL, Braun HP, et al. The role of amino acid metabolism during abiotic stress release. Plant Cell Environ. (2019) 42:1630–44. doi: 10.1111/pce.13518
14. Pan C, Ma J, Tao F, Ji C, Zhao Y, Chen S, et al. Novel insight into the antioxidant proteins derived from laver (Porphyra haitanensis) by proteomics analysis and protein based bioinformatics. Food Biosci. (2021) 42:101134. doi: 10.1016/j.fbio.2021.101134
15. Wang G, Li X, Wang Z. APD3: the antimicrobial peptide database as a tool for research and education. Nucleic Acids Res. (2016) 44:1087–93. doi: 10.1093/nar/gkv1278
16. Li Q, Zhang C, Chen H, Xue J, Guo X, Liang M, et al. BioPepDB: an integrated data platform for food-derived bioactive peptides. Int J Food Sci Nutr. (2018) 69:963–8. doi: 10.1080/09637486.2018.1446916
17. Yang M, Ma L, Yang X, Li L, Chen S, Qi B, et al. Photosynthetic protein-based edible quality formation in various Porphyra dentata harvests determined by label-free proteomics analysis. Cells. (2022) 11:1136. doi: 10.3390/cells11071136
18. Oh S, Kim J, Kim H, Son S, Choe E. Composition and antioxidant activity of dried laver, dolgim. Korean J Food Technol. (2013) 45:403–8. doi: 10.9721/KJFST.2013.45.4.403
19. Xu Y, Chen C, Ji D, Hang N, Xie C. Proteomic profile analysis of Pyropia haitanensis in response to high-temperature stress. J Appl Phycol. (2014) 26:607–18. doi: 10.1007/s10811-013-0066-8
20. Lee HJ, Choi J. Isolation and characterization of a high-growth-rate strain in Pyropia yezoensis induced by ethyl methane sulfonate. J Appl Phycol. (2018) 30:2513–22. doi: 10.1007/s10811-018-1426-1
21. Dupree EJ, Jayathirtha M, Yorkey H, Mihasan M, Petre BA, Darie CC. A critical review of bottom-up proteomics: the good, the bad, and the future of this field. Proteomes. (2020) 8:14. doi: 10.3390/proteomes8030014
22. Nahnsen S, Bielow C, Reinert K, Kohlbacher O. Tools for label-free peptide quantification. Mol Cell Proteomics. (2013) 12:549–56. doi: 10.1074/mcp.R112.025163
23. Xiang Y, Sun C, Zhao Y, Li L, Yang X, Wu Y, et al. Label-free proteomic analysis reveals freshness-related proteins in sea bass (Lateolabrax japonicus) fillets stored on ice. LWT. (2022) 155:112885. doi: 10.1016/j.lwt.2021.112885
24. Yuan P, Chen X, Benjakul S, Sun J, Zhang B. Label-free based proteomics revealed the specific changes of muscle proteins in pike eel (Muraenesox cinereus) under cold stress. Food Chem X. (2022) 14:100275. doi: 10.1016/j.fochx.2022.100275
25. Shi Y, Wang X, Huang A. Proteomic analysis and food-grade enzymes of Moringa oleifer Lam. a Lam. flower. Int J Biol Macromol. (2018) 115:883–90. doi: 10.1016/j.ijbiomac.2018.04.109
26. Damian S, Annika LG, Katerina CN, David L, Rebecca K, Sampo P, et al. The STRING database in 2021: customizable protein–protein networks, and functional characterization of user-uploaded gene/measurement sets. Nucleic Acids Res. (2021) 49:D605–12. doi: 10.1093/nar/gkaa1074
27. McGinnis S, Madden TL. BLAST: at the core of a powerful and diverse set of sequence analysis tools. Nucleic Acids Res. (2004) 32:W20–5. doi: 10.1093/nar/gkh435
28. Binz PA, Shofstahl J, Vizcaíno JA, Barsnes H, Chalkley RJ, Menschaert G, et al. Proteomics standards initiative extended FASTA format. J Proteome Res. (2019) 18:2686–92. doi: 10.1021/acs.jproteome.9b00064
29. Coscueta ER, Batista P, Gomes JEG, da Silva R, Pintado MM. Screening of novel bioactive peptides from goat casein: in silico to in vitro validation. Int J Mol Sci. (2022) 23:2439. doi: 10.3390/ijms23052439
30. Waghu FH, Barai RS, Gurung P, Idicula-Thomas S. CAMPR3: a database on sequences, structures and signatures of antimicrobial peptides. Nucleic Acids Res. (2016) 44:D1094–7. doi: 10.1093/nar/gkv1051
31. Kaushik V. In slico identification of epitope-based peptide vaccine for Nipah virus. Int J Pept Res Ther. (2020) 26:1147–53. doi: 10.1007/s10989-019-09917-0
32. Waterhouse A, Bertoni M, Bienert S, Studer G, Tauriello G, Gumienny R, et al. SWISS-MODEL: homology modelling of protein structures and complexes. Nucleic Acids Res. (2018) 46:W296–303. doi: 10.1093/nar/gky427
33. Paul MJ, Watson A, Griffiths CA. Trehalose 6-phosphate signalling and impact on crop yield. Biochem Soc Trans. (2020) 48:2127–37. doi: 10.1042/BST20200286
34. Zhai Z, Keereetaweep J, Liu H, Feil R, Lunn JE, Shanklin J. Expression of a bacterial trehalose-6-phosphate synthase otsA increases oil accumulation in plant seeds and vegetative tissues. Front Plant Sci. (2021) 12:656962. doi: 10.3389/fpls.2021.656962
35. Sánchez A, Vázquez A. Bioactive peptides: a review. Food Qual Saf. (2017) 1:29–46. doi: 10.1093/fqsafe/fyx006
36. Fazilah NF, Ariff AB, Khayat ME, Rios-Solis L, Halim M. Influence of probiotics, prebiotics, synbiotics and bioactive phytochemicals on the formulation of functional yogurt. J Funct Foods. (2018) 48:387–99. doi: 10.1016/j.jff.2018.07.039
37. Murtaza MA, Anees-Ur-Rehman M, Hafiz I, Ameer K, Celik OF. Effects of probiotic adjuncts on physicochemical properties, organic acids content, and proteolysis in cheese prepared from buffalo milk. J Food Process Preserv. (2022) 46:e16385. doi: 10.1111/jfpp.16385
38. Carrera M, Ezquerra-Brauer JM, Aubourg SP. Characterization of the jumbo squid (Dosidicus gigas) skin by-product by shotgun proteomics and protein-based bioinformatics. Mar Drugs. (2020) 18:31. doi: 10.3390/md18010031
39. Pradeepkiran JA, Munikumar M, Hema K, Natarajan P, Sainath SB. Chapter 4-Molecular docking and dynamics simulations of novel drug targets. In: Pradeepkiran JA, Sainath SB editors. Brucella Melitensis. Cambridge, MA: Academic Press (2021). p. 79–131. doi: 10.1016/B978-0-323-85681-2.00005-7
40. Alexander H, Rouco M, Haley ST, Dyhrman ST. Transcriptional response of Emiliania huxleyi under changing nutrient environments in the North Pacific Subtropical Gyre. Environ Microbiol. (2020) 22:1847–60. doi: 10.1111/1462-2920.14942
41. Wüthrich D, Wenzel C, Bavan T, Bruggmann R, Berthoud H, Irmler S. Transcriptional regulation of cysteine and methionine metabolism in Lactobacillus paracasei FAM18149. Front Microbiol. (2018) 9:1261. doi: 10.3389/fmicb.2018.01261
42. Schmitz-Esser S, Dzieciol M, Nischler E, Schornsteiner E, Bereuter O, Mann E, et al. Abundance and potential contribution of Gram-negative cheese rind bacteria from Austrian artisanal hard cheeses. Int J Food Microbiol. (2018) 266:96–103. doi: 10.1016/j.ijfoodmicro.2017.11.013
43. Iqbal D, Rehman MT, Bin Dukhyil A, Rizvi SMD, Al Ajmi MF, Alshehri BM, et al. High-throughput screening and molecular dynamics simulation of natural product-like compounds against Alzheimer’s disease through multitarget approach. Pharmaceuticals. (2021) 14:937. doi: 10.3390/ph14090937
44. Sharma P, Jha AB, Dubey RS, Pessarakli M. Reactive oxygen species, oxidative damage, and antioxidative defense mechanism in plants under stressful conditions. J Bot. (2012) 2012:1–26. doi: 10.1155/2012/217037
45. Xu IMJ, Lai RKH, Lin SH, Tse APW, Chiu DKC, Koh HY, et al. Transketolase counteracts oxidative stress to drive cancer development. Proc Natl Acad Sci USA. (2016) 113:725–34. doi: 10.1073/pnas.1508779113
46. Aamudalapalli HB, Bertwistle D, Palmer DR, Sanders DA. myo-Inositol dehydrogenase and scyllo-inositol dehydrogenase from Lactobacillus casei BL23 bind their substrates in very different orientations. Biochim Biophys Acta Proteins Proteom. (2018) 1866:1115–24. doi: 10.1016/j.bbapap.2018.08.011
Keywords: Porphyra dentata, proteomics, amino acid metabolism, carbohydrate metabolism, bioactive peptides
Citation: Yang M, Ma L, Yang X, Li L, Chen S, Qi B, Wang Y, Li C, Yang S and Zhao Y (2022) Bioinformatic Prediction and Characterization of Proteins in Porphyra dentata by Shotgun Proteomics. Front. Nutr. 9:924524. doi: 10.3389/fnut.2022.924524
Received: 20 April 2022; Accepted: 30 May 2022;
Published: 07 July 2022.
Edited by:
Joaquina Pinheiro, Politécnico de Leiria, PortugalReviewed by:
Bhakti Tanna, Central Salt and Marine Chemicals Research Institute (CSIR), IndiaChaogang Wang, Shenzhen University, China
Copyright © 2022 Yang, Ma, Yang, Li, Chen, Qi, Wang, Li, Yang and Zhao. This is an open-access article distributed under the terms of the Creative Commons Attribution License (CC BY). The use, distribution or reproduction in other forums is permitted, provided the original author(s) and the copyright owner(s) are credited and that the original publication in this journal is cited, in accordance with accepted academic practice. No use, distribution or reproduction is permitted which does not comply with these terms.
*Correspondence: Yongqiang Zhao, zhaoyq@scsfri.ac.cn
†These authors have contributed equally to this work