Regional Homogeneity Abnormalities and Its Correlation With Impulsivity in Male Abstinent Methamphetamine Dependent Individuals
- 1National Clinical Research Center for Mental Disorders, and Department of Psychiatry, The Second Xiangya Hospital of Central South University, Changsha, China
- 2Hunan Key Laboratory of Psychiatry and Mental Health, Changsha, China
- 3Department of Psychiatry, Hunan Brain Hospital (Hunan Second People’s Hospital), Changsha, China
- 4Department of Psychiatry, Sir Run Run Shaw Hospital, School of Medicine, Zhejiang University, Hangzhou, China
- 5Laboratory of Psychological Heath and Imaging, Shanghai Mental Health Center, Shanghai Jiao Tong University School of Medicine, Institute of Psychological and Behavioral Science, Shanghai Jiao Tong University, Shanghai, China
- 6School of Computer Science and Engineering, Central South University, Changsha, China
- 7Department of Psychological Sciences, College of Arts & Sciences, Texas Tech University, Lubbock, TX, United States
- 8Department of Psychiatry, Sanming City Taijiang Hospital, Sanming, China
Methamphetamine (MA) use affects the brain structure and function. However, no studies have investigated the relationship between changes in regional homogeneity (ReHo) and impulsivity in MA dependent individuals (MADs). The aim of this study was to investigate the changes of brain activity under resting state in MADs and their relationship to impulsivity using ReHo method. Functional magnetic resonance imaging (fMRI) was performed to collect data from 46 MADs and 44 healthy controls (HCs) under resting state. ReHo method was used to investigate the differences in average ReHo values between the two groups. The ReHo values abnormalities of the brain regions found in inter-group comparisons were extracted and correlated with impulsivity. Compared to the HCs, MADs showed significant increased ReHo values in the bilateral striatum, while the ReHo values of the bilateral precentral gyrus and the bilateral postcentral gyrus decreased significantly. The ReHo values of the left precentral gyrus were negatively correlated with the BIS-attention, BIS-motor, and BIS-nonplanning subscale scores, while the ReHo values of the postcentral gyrus were only negatively correlated with the BIS-motor subscale scores in MADs. The abnormal spontaneous brain activity in the resting state of MADs revealed in this study may further improve our understanding of the neuro-matrix of MADs impulse control dysfunction and may help us to explore the neuropathological mechanism of MADs related dysfunction and rehabilitation.
Introduction
Methamphetamine (MA), commonly known as “ice,” is an amphetamine-type stimulant (ATS) that is one of the most abused new drugs in the world (Shadloo et al., 2017). According to the 2019 China Drug Situation Report released in 2020, MA users in China accounted for 55.2 percent of its 2.148 million registered drug users, making it the largest drug user in the country by far, (China National Narcotic Control Commission, 2020). In addition to the high prevalence of MA use, the high recurrence rates of MA dependent individuals (MADs) exacerbate the problem, creating a huge public health burden worldwide (Jiang et al., 2021). Chronic use of MA has been associated with a variety of physical and mental health problems (e.g., cardiovascular disease, depression) (Schwarzbach et al., 2020; Jiang et al., 2021), daily dysfunction (e.g., impulsivity) (Moallem et al., 2018; Wang et al., 2020) and neurocognitive dysfunction (Basterfield et al., 2019; Mizoguchi and Yamada, 2019), contributing to a considerable global disease burden. Although increasing studies have been conducted on the treating MA use disorders, such as pharmacotherapy (Chan et al., 2019), psychotherapy (Harada et al., 2018), and repetitive transcranial magnetic stimulation (rTMS) (AshaRani et al., 2020), the effectiveness in reducing MA recurrence remains unsatisfactory.
A challenge for treating MA abuse is recurrence during abstinence (Li X. et al., 2019). Although many factors contribute to recurrence of MADs, a possible key predictor is impulsivity (Ahn et al., 2016; Vassileva and Conrod, 2019). Impulsivity is defined as a predisposition toward rapid, unplanned reactions to internal or external stimuli with diminished regard to their negative consequences to themselves or others (Ahn et al., 2016; Psederska et al., 2021). It is considered a key etiological factor in current conceptualizations of substance use disorder (SUD). Moreover, self-reported impulsivity is a strong predictor of poor treatment response (Fernie et al., 2010; Winhusen et al., 2013). Although MA dependence is associated with many neuropsychiatric and behavioral problems, impulsivity has been studied extensively because of its purported importance in initiation and escalation of drug use and the probability of recurrence (Schwartz et al., 2010).
One of the popular methods of investigating impulsivity in MA patients is through resting-state functional magnetic resonance imaging (rs-fMRI). Rs-fMRI is a powerful tool that measures brain activities by detecting changes in blood-oxygen-level-dependent (BOLD) signals in the resting brain (Park et al., 2019). It has been widely used recently because it does not require the involvement of any specific task in the scanner and could reveal the neural substrates of task-independent processes in diseases (Xie et al., 2021). A specific methodology in rs-fMRI is regional homogeneity (ReHo). ReHo evaluates signal synchronization by calculating the consistency of temporal variations of BOLD signals within local brain regions (Liu et al., 2019c), and has been used in many neuropsychiatric disorders (Li H. et al., 2019; Ma et al., 2019), but rarely in addiction (Liao et al., 2012; Qiu et al., 2013). Over the past few decades, several neuroimaging studies have assessed the relationship between abnormal brain function and abnormal behaviors (such as impulsivity) in MADs, but the results have been inconsistent. For instance, abstinent MADs showed less frontal activation during cognitive control compared to healthy controls (HCs) (Salo et al., 2013; Weafer et al., 2020), and showed less delay discounting activation than HCs in the bilateral precuneus, right caudate, anterior cingulate cortex (ACC), and dorsolateral prefrontal cortex (DLPFC) (Hoffman et al., 2008). In contrast, abstinence MADs have greater activation of MA-related cues in the ventral striatum and medial frontal cortex (Malcolm et al., 2016). Moreover, few functional magnetic resonance imaging (fMRI) studies have focused on the relationship between impulsivity and brain dysfunction.
In this study, we used the ReHo method to study the difference in spontaneous brain activity between MADs and HCs in the resting state. Based on our previous studies (Xie et al., 2021), we hypothesized that ReHo values in the resting state would differ in the relevant brain regions between MADs and HCs. We also hypothesized that differences in ReHo values might be related to impulsivity.
Materials and Methods
Participants
One hundred Han male participants (50 MADs and 50 HCs, aged 18 – 45, completion of at least 6 years of formal education; fluency in Chinese; right-handed) were enrolled in this study. Data for 3 MADs (2 had contraindications to MRI and 1 had abnormal scan) and 6 HCs (3 were lost to follow-up, 1 had contraindication to MRI, and 2 had abnormal scans) were excluded. Meanwhile, one MADs with maximal head motion exceeding 2 mm or rotations over 2° was excluded from further analysis. A total of 46 MADs and 44 HCs were included in the final analysis. MADs were recruited from the Kangda Voluntary Drug Rehabilitation Centers in Changsha, Hunan Province, while drug-free HCs were recruited via local community advertisements. All the MADs were diagnosed with MA use disorders per DSM-5 by two trained senior psychiatrists using the Structured Clinical Interview (SCID) (First et al., 2002). All the MADs were required not to use any psychoactive substances other than tobacco, including alcohol, at least 48 h before the MRI scan. In order to reduce the effects of different stages of abstinence on brain cognition, MADs were assessed 3 months after abstinence, during which time MADs used drugs. Participants were excluded if they met any of following criteria: (1) had any general medical condition or neurological disorders that could confound brain function; (2) had a history of severe head injury with skull fracture or loss of consciousness of more than 10 min; (3) had any current or previous psychiatric disorder or family history of psychiatric disorder; and (4) had contraindications for MRI (including implanted metallic devices or ferromagnetic material or claustrophobia).
Clinical Assessment
All the participants completed the following self-report scales; all the instruments have good reliability and validity.
General Information
Basic demographic information included age, height, weight, education, marital status, employment, and income. We also reported the drinking, smoking, and betel use status in the two groups.
Impulsivity
The level of impulsivity was measured using the Barratt Impulsivity Scale 11 Edition (BIS-11), which is the most extensive self-report scale for this purpose (Subramanian et al., 2020). The Chinese version of BIS-11 was used to measure the cognitive impulsiveness, motor impulsiveness and non-planning impulsiveness of MADs. Items 4, 5, 13, 14, 15, 16, 17, 19, 20, 21, and 26 were reverse scored (Yao et al., 2007). The whole scale consists of 30 items, using a 5-point Likert scale for each item with a higher total score indicating stronger impulsivity (Patton et al., 1995).
Magnetic Resonance Imaging Data Acquisition
Magnetic resonance imaging data of all participants were acquired in the resting condition using a 3.0T MRI scanner (Siemens Skyra, Munich) equipped with a 16-channel head coil at the Magnetic Resonance Imaging Center of Hunan Children’s Hospital, Changsha, China. None of the participants were taking any medications on the day of the MRI scan. Participants were instructed to remain awake and still in supine position with eyes closed. During the scanning, foam pads and earplugs were used to restrain head motion and to attenuate noise. Anatomical T1-weighted MRI data were acquired using a 3D magnetization preparing rapid acquisition gradient echo sequence with the following parameters: repetition time (TR) = 2,530 ms, echo time (TE) = 2.98 ms, flip angle = 7°, field of view = 256 × 256 mm, slice thickness = 1 mm, slice gap = 0 mm, voxel size = 1 × 1 × 1 mm3, number of slices = 176, and scanning time = 363 s. Functional images were obtained using a gradient echo-planar imaging (EPI) sequence with the following parameters: TR = 2,000 ms, TE = 30 ms, flip angle = 78°, field of view = 224 × 224 mm, slice thickness = 3.5 mm, slice gap = 0.7 mm, voxel size = 3.5 × 3.5 × 3.5 mm3, number of slice = 33, and scanning time = 488 s.
Data Preprocessing
Functional MRI data were preprocessed according to standard procedures with the Data Processing Assistant for Resting-State fMRI (DPARSF, version 4.1) (Chao-Gan and Yu-Feng, 2010),1 running in MATLAB (version R2013b, The MathWorks, Inc., Natick, MA, United States) (Yan et al., 2016). Functional MRI data preprocessing consisted of the following steps: (1) remove the first 10 time points in case of unstable signal quality, (2) perform slice-timing adjustment, (3) perform realignment, excluding subjects with maximal head motion exceeding 2 mm or rotations over 2°, (4) remove the mean framewise displacement (FD) > 0.2 mm, (5) conduct spatial normalization to the EPI template of Montreal Neurological Institute (MNI) space by resampling to 3 mm × 3 mm × 3 mm, (6) remove linear detrending, (7) temporal band-pass filtering (0.01 – 0.1 Hz), (8) smooth at 8 mm full width at half maximum (FWHM), and (9) nuisance signals were regressed out, including Friston 24 head motion parameters, global signal, white matter signal, and cerebrospinal fluid signal.
Regional Homogeneity Calculation
ReHo calculation was performed with the REST2 software. In short, this was achieved by calculating Kendall’s coefficient of concordance (KCC) of time series of a given voxel with those of its nearest 26 neighbors on a voxel-by-voxel basis (Zang et al., 2004). The KCC value was calculated to this voxel, and a separate KCC map was obtained for each participant. For standardization purpose, the individual ReHo maps were divided by their own global mean KCC within the whole-brain mask. The individual ReHo maps were then spatially smoothed with an 8 mm FWHM Gaussian kernel to reduce noise and residual differences in gyral anatomy.
Statistical Analysis
All statistical analyses were performed using R version 3.5.3. Before statistical analysis, normality, and variance homogeneity were tested. Demographic and clinical data are compared between groups using the Chi-square test, Mann–Whitney U test, or Student’s t-test, When appropriate. Several one-way analyses of covariances (ANCOVAs) were used to analyze group differences in ReHo values among the pre-defined regions of interest (ROIs). Covariances used were smoking, drinking, and betel use. Bonferroni-correction was used at this stage to reduce the number of type-I errors. ANOVAs were also used to analyze group differences in impulsivity. Significant ReHo results were subsequently used as individual predictors in linear regression for impulsivity. Scores were mean centered before running linear regressions as interaction terms needed to be computed.
Results
Demographics and Clinical Characteristics
MADs were significantly older than HCs with lower weight and higher education levels (Table 1). More importantly, MADs smoked more (95.70%) than HCs (45.50%, p < 0.001), and had longer smoking duration (12.80 ± 4.85 years) than HCs (7.42 ± 6.73, p = 0.001). Although there were no significant differences in drinking (p = 0.052), MADs drank for a longer time (3.89 ± 5.44 years) compared to HCs (1.27 ± 3.01, p = 0.006). Finally, MADs also use more betel (67.40%) compared to HCs (40.90%, p = 0.021) and used betel for a longer duration (9.02 ± 5.17 years) as compared to HCs (3.78 ± 2.16, p < 0.001).
Behavioral Results
There were no significant differences between MADs and HCs on BIS-attention at F(1,85) = 0.11, p = 0.74, BIS-nonplanning at F(1,85) = 0.29, p = 0.59, or BIS-motor at F(1,85) = 1.58, p = 0.21.
Neuroimaging Results
Regional Homogeneity
There were significant differences between MADs and HCs on ReHo values of the left caudate at F(1,85) = 14.79, p = 0.017, right caudate at F(1,85) = 30.00, p < 0.001, left postcentral gyrus at F(1,85) = 31.96, p < 0.001, left precentral gyrus at F(1,85) = 39.21, p = 0.0087, right postcentral gyrus at F(1,85) = 16.73, p < 0.001, and right precentral gyrus at F(1,85) = 17.06, p = 0.0089 after Bonferroni correction. See Figure 1 for more details.
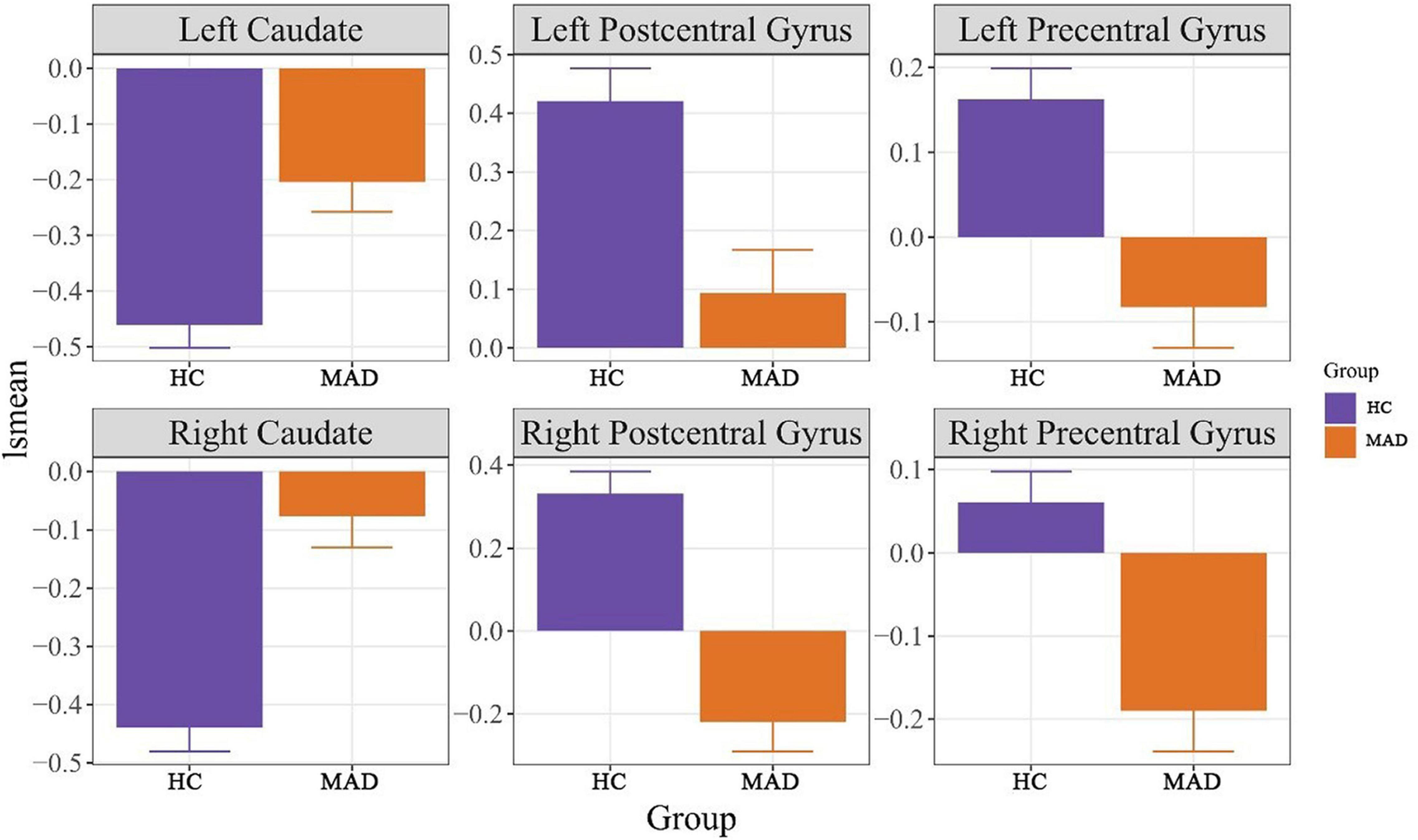
Figure 1. It displays the least square means (lsmeans) among HC and MAD for the significant regions of interest. HC, healthy control, MAD, methamphetamine dependent individual.
Regression Analysis
Regression analysis of predicting BIS from ReHo values revealed a significant model for left postcentral gyrus for predicting BIS-motor at F(3,86) = 4.72, p = 0.0043. Although there was a Group × ReHo interaction where higher left postcentral gyrus ReHo values in the MAD group was associated with lower BIS-motor scores, it only approached significance (B = −3.74, p = 0.074). There was also a significant model for ReHo values of the left precentral gyrus at F(3,86) = 4.32, p = 0.0069. Although there was a Group × ReHo interaction where higher left precentral gyrus ReHo values in the MAD group was associated with lower BIS-nonplanning scores, it only approached significance (B = −5.35, p = 0.061). ReHo values of the left precentral gyrus predicted BIS-motor scores at F(3,86) = 4.32, p = 0.001. There was a Group × ReHo interaction where higher left precentral gyrus ReHo values in the MAD group was associated with lower BIS-motor scores (B = −6.75, p = 0.034). ReHo values of the left precentral gyrus also predicted BIS-attention scores at F(3,86) = 3.03, p = 0.033. There was a Group × ReHo interaction where higher left precentral gyrus ReHo values in the MAD group was associated with lower BIS-attention scores (B = −8.29, p = 0.017). There were no other significant models of ReHo values predicting BIS scores. See Figure 2 for more details.
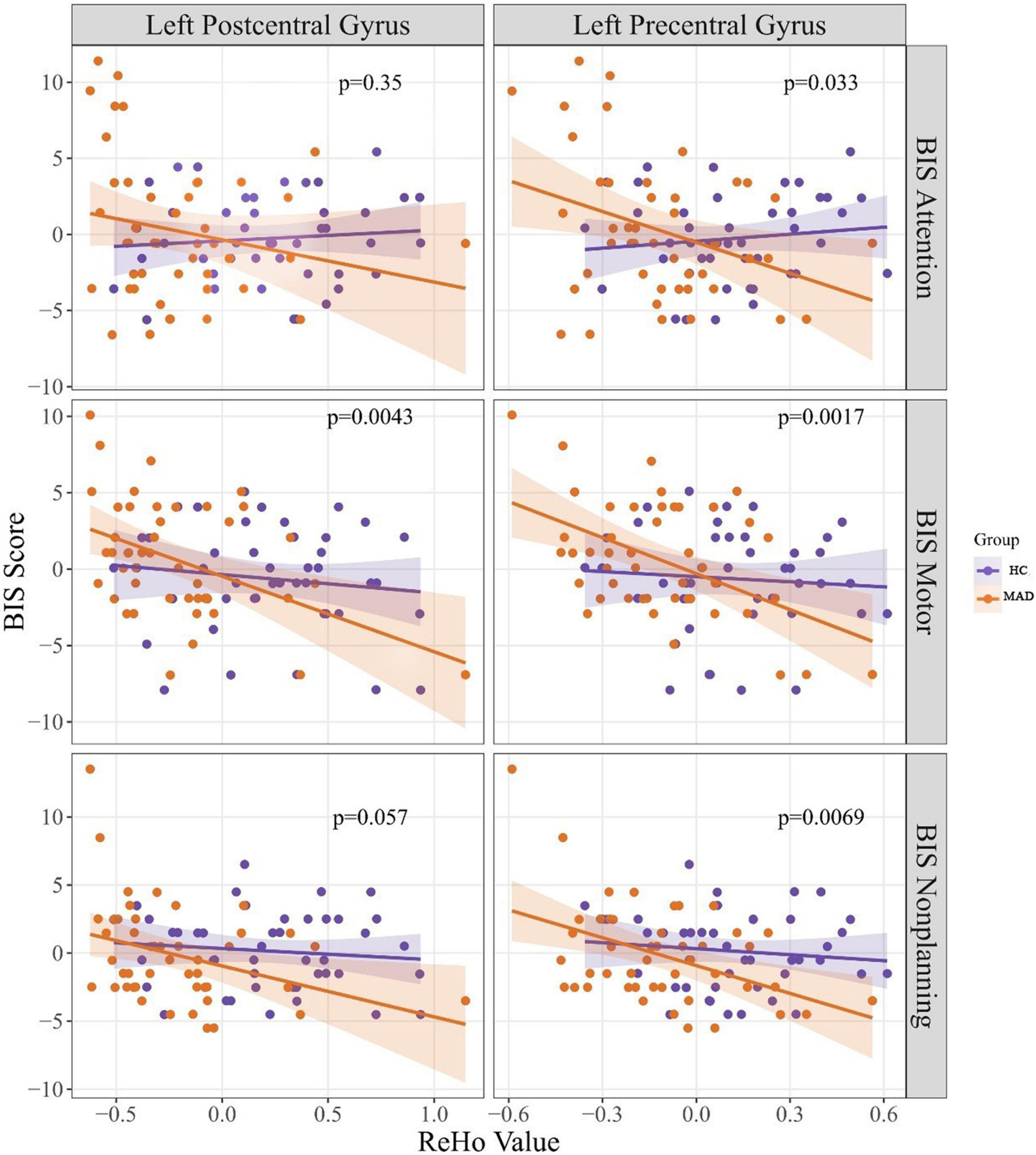
Figure 2. It presents the correlation between BIS scores and ReHo values. The p-values presented in this figure were p-values of the regression model. BIS, Barratt Impulsivity Scale, ReHo, regional homogeneity, HC, healthy control, and MAD, methamphetamine dependent individual.
Discussion
To our knowledge, this is the first study to explore the relationship between ReHo values of local spontaneous brain activity and impulsivity in MADs. Compared with HCs, MADs showed significantly increased ReHo values in bilateral caudate, and decreased ReHo values in the bilateral postcentral gyrus, and the bilateral precentral gyrus. We further found that the ReHo values of the left precentral gyrus were negatively correlated with the BIS-attention, BIS-motor, and BIS-nonplanning subscale scores, while the ReHo values of the left postcentral gyrus were only negatively correlated with the BIS-motor subscale scores in MADs.
Compared to one of our previous studies, this study focused on the relationship between the abnormal ReHo values of the brain ROIs in MADs and the impulsivity of MADs (Xie et al., 2021). Moreover, most previous studies have focused on exploring the relationship between low-frequency fluctuation (ALFF) (Wang et al., 2013; Liu Y. et al., 2020), fractional amplitude of low frequency fluctuation (fALFF) (Chu et al., 2014; Wang et al., 2017) or structural MRI (Huang et al., 2020; Meade et al., 2020) and clinical variables, rather than ReHo. Furthermore, some studies on ReHo have focused on other addicts (Huang et al., 2020; Meade et al., 2020) and psychiatric patients (Liu P. et al., 2020; Shan et al., 2021), but not on MADs.
The striatum is a continuous mass structure composed of the caudate and putamen (Lerner et al., 2012), which can directly participate in rewards, movement control, regulation, and decision-making, especially action selection and initiation (de la Fuente-Fernández et al., 2002; Liu et al., 2019a). In this study, the increase in the bilateral striatum ReHo of MADs indicates the importance of their spontaneous neural activity in resting brain of MADs. This is consistent with our previous findings that ReHo was increased in the bilateral striatum of MADs compared to HCs (Xie et al., 2021; Yang et al., 2021). To date, only a few rs-fMRI studies have used ReHo to explore spontaneous brain activity changes and synchronization in individuals with SUD. A comparative study in recurring heroin addicts found that ReHo in the right caudate of recurred heroin addicts increased (Chang et al., 2016). It should be noted that compared with HCs, the ReHo of the left dorsal striatum was reduced in codeine-containing cough syrups (CCS) dependent individuals, and was negatively correlated with the BIS-11 total scores and attentional impulsivity score (Qiu et al., 2013). The reason why our results were inconsistent with this study may be mainly due to the heterogeneity of clinical samples (i.e., different addictive substances, different data collection status, and different sample sizes). Thus, the different mechanisms underlying MA and codeine use may result in representation in the brain. In addition, the ReHo values may change during withdrawal. Using fALFF, it was found that the activity of spontaneous neurons in the caudate of smokers was enhanced (Feng et al., 2016). Meanwhile, structural MRI studies showed a significant increase in gray matter volume in the putamen and caudate nuclei of cocaine users compared to HCs (Ersche et al., 2011). Although the methodologies were different, these findings provide strong support for the theory that the bilateral striatum is a key region in addiction disorders, and our findings support this view.
It is worth noting that the ReHo values of the bilateral postcentral gyrus and the bilateral precentral gyrus were decreased in MADs. It is well known that precentral gyrus, as the primary motor cortex, is involved in somatosensory activity, while postcentral gyrus, as the primary sensory cortex, is involved in the initiation and regulation of spontaneous movement (Javed et al., 2021). However, their relevance to addiction should not be underestimated. Studies have shown that the sensory and motor cortex is involved in various stages of drug addiction (Yalachkov et al., 2010). Meanwhile, the activation of sensory and motor cortex induced by drug-related cues can predict relapse (Kosten et al., 2006). Previous studies have also shown that the ReHo values of MA-associated psychosis (MAP) decreases in the left postcentral gyrus (Yang et al., 2021). Moreover, studies of nicotine addicts have shown a significant increase in the ReHo values of paracentral lobule after 2 weeks of abstinence (Mo et al., 2018). More interestingly, the study further found that the ReHo values of the left precentral gyrus were negatively correlated with the BIS-attention, BIS-motor, and BIS-nonplanning subscales scores, while the ReHo values of the left postcentral gyrus were only negatively correlated with the BIS-motor subscale scores in MADs. It is not difficult to know from the results that the negative correlation with impulsivity is mainly concentrated in the left precentral gyrus, rather than the left postcentral gyrus, which is consistent with the previous research results. For example, a meta-analysis of cue-reactivity in behavioral addictions found increased neural activation in the precentral gyrus (Starcke et al., 2018). Similar results also exist in individual with SUD (such as cannabis, alcohol) (Cheng et al., 2014; Fede et al., 2020). The precentral gyrus is one of the four most important gyrus in the prefrontal cortex. The prefrontal cortex is known to be the higher-order association center of the brain as it is responsible for decision making, regulating impulsivity-related disorders such as drug addiction, reasoning, personality expression, maintaining social appropriateness, and other complex cognitive behaviors (Liu et al., 2019b; El-Baba and Schury, 2021). This may be one of the reasons why the precentral gyrus is more associated with impulsivity than the postcentral gyrus.
We observed that the ReHo values of the left precentral gyrus were negatively correlated with BIS-attention, BIS-motor, and BIS-nonplanning subscales scores in MADs. This may indicate that reduced ReHo values in the left precentral gyrus are more closely associated with impulsivity in MADs than in HCs. Also, as presented in this study, ReHo values of the left postcentral gyrus only negatively correlated with BIS-motor subscale scores in MADs. Different brain regions are thought to be preferentially involved in complementary aspects of cognitive control to produce appropriate behavior (Crunelle et al., 2014). The left precentral gyrus may be particularly involved in cognitive control of MAD behavior, behavioral aspect of impulsivity, and temporal impulsivity, while the left postcentral gyrus may only be particularly involved in the behavioral aspect of impulsivity. In addition, no correlation was observed between left precentral gyrus of HCs and BIS subscales scores in the current study. This may indicate that changes in the ReHo values of the left precentral gyrus cannot be a predictor of impulsivity in HCs. Therefore, the reduced ReHo values in the left precentral gyrus might indicate dysfunction in this region in MADs.
While our study provided new insights on the role of ReHo in the left precentral gyrus on impulsive behaviors, some limitations of our study need to be addressed. Firstly, the study was cross-sectional, and causal relationships between variables could not be rigorously evaluated. Therefore, longitudinal studies are needed to help address these issues in the future. Secondly, the study recruited only male MADs, and the results are not representative of brain changes in women. As previous studies have shown that gender is an influential factor of brain dysfunction in MADs, further study on female MADs is needed. Finally, as with all ReHo analyses, it is still debated what higher or lower ReHo represents in terms of biologic functions, or their interpretability toward behavioral change. As ReHo only measures local connectivity, and the brain consists of connected networks, other analyses are needed to supplement ReHo results. Furthermore, this study did not evaluate other factors related to the ReHo of MADs, such as chronic stress.
Conclusion
In conclusion, this study found abnormal ReHo values of MADs in the bilateral striatum, the bilateral precentral gyrus, and the bilateral postcentral gyrus. These brain changes might have to do with self-control and external perception. We also observed that decreased ReHo values in the left precentral gyrus and the left postcentral gyrus were associated with impulsivity. These findings may help to illustrate the pathophysiological mechanism of MADs, particularly in impulsivity, and provide a basis for the urgent formulation of good clinical treatment and prevention strategies to prevent recurrence.
Data Availability Statement
The data analyzed in this study is subject to the following licenses/restrictions: All data in the current study was stored in the PI’s affiliation, and is available from the corresponding authors on reasonable request and with completion of data user agreement. Requests to access these datasets should be directed to TL, liutieqiao123@csu.edu.cn.
Ethics Statement
The studies involving human participants were reviewed and approved by the Ethics Committee of the Second Xiangya Hospital, Central South University (No. S095, 2013). The patients/participants provided their written informed consent to participate in this study.
Author Contributions
TL and YL designed and supervised the study. YZ, YH, ML, YfW, and YyW collected the data. YZ, WY, QjW, and JX analyzed and interpreted the data. YZ and QjW drafted the manuscript. HR, XW, WY, YM, QxW, DY, LW, and TL revised the manuscript. All co-authors revised and approved the final version to be published.
Funding
This study was supported by the National Key R&D Program of China (2017YFC1310400 to TL), the provincial Natural Science Foundation of Hunan (2020JJ4795 to TL and 2018JJ2221 to DY), the Health and Family Planning Commission of Hunan Province Project (B20180484 to YZ and B20180123 to DY), and the Science and Technology Bureau, Changsha Project (kq2004106 to YZ).
Conflict of Interest
The authors declare that the research was conducted in the absence of any commercial or financial relationships that could be construed as a potential conflict of interest.
Publisher’s Note
All claims expressed in this article are solely those of the authors and do not necessarily represent those of their affiliated organizations, or those of the publisher, the editors and the reviewers. Any product that may be evaluated in this article, or claim that may be made by its manufacturer, is not guaranteed or endorsed by the publisher.
Acknowledgments
We acknowledged all the staff at Kangda Voluntary Drug Rehabilitation Center for their assistance in data collection, and we would like to express our sincere thanks to all the participants in this work.
Footnotes
References
Ahn, W. Y., Ramesh, D., Moeller, F. G., and Vassileva, J. (2016). Utility of machine-learning approaches to identify behavioral markers for substance use disorders: impulsivity dimensions as predictors of current cocaine dependence. Front. Psychiatry 7:34. doi: 10.3389/fpsyt.2016.00034
AshaRani, P. V., Hombali, A., Seow, E., Ong, W. J., Tan, J. H., and Subramaniam, M. (2020). Non-pharmacological interventions for methamphetamine use disorder: a systematic review. Drug Alcohol Depend. 212:108060. doi: 10.1016/j.drugalcdep.2020.108060
Basterfield, C., Hester, R., and Bowden, S. C. (2019). A meta-analysis of the relationship between abstinence and neuropsychological functioning in methamphetamine use disorder. Neuropsychology 33, 739–753. doi: 10.1037/neu0000552
Chan, B., Freeman, M., Kondo, K., Ayers, C., Montgomery, J., Paynter, R., et al. (2019). Pharmacotherapy for methamphetamine/amphetamine use disorder-a systematic review and meta-analysis. Addiction 114, 2122–2136. doi: 10.1111/add.14755
Chang, H., Li, W., Li, Q., Chen, J., Zhu, J., Ye, J., et al. (2016). Regional homogeneity changes between heroin relapse and non-relapse patients under methadone maintenance treatment: a resting-state fMRI study. BMC Neurol. 16:145. doi: 10.1186/s12883-016-0659-3
Chao-Gan, Y., and Yu-Feng, Z. (2010). DPARSF: a MATLAB toolbox for “pipeline” data analysis of resting-state fMRI. Front. Syst. Neurosci. 4:13. doi: 10.3389/fnsys.2010.00013
Cheng, H., Skosnik, P. D., Pruce, B. J., Brumbaugh, M. S., Vollmer, J. M., Fridberg, D. J., et al. (2014). Resting state functional magnetic resonance imaging reveals distinct brain activity in heavy cannabis users - a multi-voxel pattern analysis. J. Psychopharmacol. 28, 1030–1040. doi: 10.1177/0269881114550354
China National Narcotic Control Commission (2020). Drug Situation in China (2019). Available online at: http://www.nncc626.com/2020-06/25/c_1210675877.htm (accessed on 25 June 2020).
Chu, S., Xiao, D., Wang, S., Peng, P., Xie, T., He, Y., et al. (2014). Spontaneous brain activity in chronic smokers revealed by fractional amplitude of low frequency fluctuation analysis: a resting state functional magnetic resonance imaging study. Chin. Med. J. (Engl) 127, 1504–1509.
Crunelle, C. L., Kaag, A. M., van Wingen, G., van den Munkhof, H. E., Homberg, J. R., Reneman, L., et al. (2014). Reduced frontal brain volume in non-treatment-seeking cocaine-dependent individuals: exploring the role of impulsivity, depression, and smoking. Front. Hum. Neurosci. 8:7. doi: 10.3389/fnhum.2014.00007
de la Fuente-Fernández, R., Phillips, A. G., Zamburlini, M., Sossi, V., Calne, D. B., Ruth, T. J., et al. (2002). Dopamine release in human ventral striatum and expectation of reward. Behav. Brain Res. 136, 359–363. doi: 10.1016/s0166-4328(02)00130-4
El-Baba, R. M., and Schury, M. P. (2021). Neuroanatomy, Frontal Cortex [Online]. Treasure Island, FL: StatPearls Publishing.
Ersche, K. D., Barnes, A., Jones, P. S., Morein-Zamir, S., Robbins, T. W., and Bullmore, E. T. (2011). Abnormal structure of frontostriatal brain systems is associated with aspects of impulsivity and compulsivity in cocaine dependence. Brain 134(Pt 7), 2013–2024. doi: 10.1093/brain/awr138
Fede, S. J., Abrahao, K. P., Cortes, C. R., Grodin, E. N., Schwandt, M. L., George, D. T., et al. (2020). Alcohol effects on globus pallidus connectivity: role of impulsivity and binge drinking. PLoS One 15:e0224906. doi: 10.1371/journal.pone.0224906
Feng, D., Yuan, K., Li, Y., Cai, C., Yin, J., Bi, Y., et al. (2016). Intra-regional and inter-regional abnormalities and cognitive control deficits in young adult smokers. Brain Imaging Behav. 10, 506–516. doi: 10.1007/s11682-015-9427-z
Fernie, G., Cole, J. C., Goudie, A. J., and Field, M. (2010). Risk-taking but not response inhibition or delay discounting predict alcohol consumption in social drinkers. Drug Alcohol Depend. 112, 54–61. doi: 10.1016/j.drugalcdep.2010.05.011
First, M. B., Spitzer, R. L., Gibbon, M., and Williams, J. (2002). Structured Clinical Interview for DSM-IV-TR Axis I Disorders, Research Version, Patient Edition With Psychotic Screen (SCID-I/P W/PSY SCREEN). New York, NY: New York State Psychiatric Institute.
Harada, T., Tsutomi, H., Mori, R., and Wilson, D. B. (2018). Cognitive-behavioural treatment for amphetamine-type stimulants (ATS)-use disorders. Cochrane Database Syst. Rev. 12:Cd011315. doi: 10.1002/14651858.CD011315.pub2
Hoffman, W. F., Schwartz, D. L., Huckans, M. S., McFarland, B. H., Meiri, G., Stevens, A. A., et al. (2008). Cortical activation during delay discounting in abstinent methamphetamine dependent individuals. Psychopharmacology (Berl) 201, 183–193. doi: 10.1007/s00213-008-1261-1
Huang, S., Dai, Y., Zhang, C., Yang, C., Huang, Q., Hao, W., et al. (2020). Higher impulsivity and lower grey matter volume in the bilateral prefrontal cortex in long-term abstinent individuals with severe methamphetamine use disorder. Drug Alcohol Depend. 212, 108040. doi: 10.1016/j.drugalcdep.2020.108040
Javed, K., Reddy, V., and Lui, F. (2021). Neuroanatomy, Cerebral Cortex [Online]. Treasure Island, FL: StatPearls Publishing.
Jiang, P., Sun, J., Zhou, X., Lu, L., Li, L., Huang, X., et al. (2021). Functional connectivity abnormalities underlying mood disturbances in male abstinent methamphetamine abusers. Hum. Brain Mapp. 42, 3366–3378. doi: 10.1002/hbm.25439
Kosten, T. R., Scanley, B. E., Tucker, K. A., Oliveto, A., Prince, C., Sinha, R., et al. (2006). Cue-induced brain activity changes and relapse in cocaine-dependent patients. Neuropsychopharmacology 31, 644–650. doi: 10.1038/sj.npp.1300851
Lerner, A., Bagic, A., Simmons, J. M., Mari, Z., Bonne, O., Xu, B., et al. (2012). Widespread abnormality of the γ-aminobutyric acid-ergic system in Tourette syndrome. Brain 135(Pt 6), 1926–1936. doi: 10.1093/brain/aws104
Li, H., Guo, W., Liu, F., Chen, J., Su, Q., Zhang, Z., et al. (2019). Enhanced baseline activity in the left ventromedial putamen predicts individual treatment response in drug-naive, first-episode schizophrenia: results from two independent study samples. EBioMedicine 46, 248–255. doi: 10.1016/j.ebiom.2019.07.022
Li, X., Davis, I. R., Lofaro, O. M., Zhang, J., Cimbro, R., and Rubio, F. J. (2019). Distinct gene alterations between Fos-expressing striatal and thalamic neurons after withdrawal from methamphetamine self-administration. Brain Behav. 9:e01378. doi: 10.1002/brb3.1378
Liao, Y., Tang, J., Fornito, A., Liu, T., Chen, X., Chen, H., et al. (2012). Alterations in regional homogeneity of resting-state brain activity in ketamine addicts. Neurosci. Lett. 522, 36–40. doi: 10.1016/j.neulet.2012.06.009
Liu, J., Tao, J., Liu, W., Huang, J., Xue, X., Li, M., et al. (2019a). Different modulation effects of Tai Chi Chuan and Baduanjin on resting-state functional connectivity of the default mode network in older adults. Soc. Cogn. Affect. Neurosci. 14, 217–224. doi: 10.1093/scan/nsz001
Liu, J., Wu, R., Johnson, B., Vu, J., Bass, C., and Li, J. X. (2019b). The claustrum-prefrontal cortex pathway regulates impulsive-like behavior. J. Neurosci. 39, 10071–10080. doi: 10.1523/jneurosci.1005-19.2019
Liu, P., Li, Q., Zhang, A., Liu, Z., Sun, N., Yang, C., et al. (2020). Similar and different regional homogeneity changes between bipolar disorder and unipolar depression: a resting-state fMRI study. Neuropsychiatr. Dis. Treat. 16, 1087–1093. doi: 10.2147/ndt.S249489
Liu, Y., Li, M., Chen, H., Wei, X., Hu, G., Yu, S., et al. (2019c). Alterations of regional homogeneity in Parkinson’s disease patients with freezing of gait: a resting-state fMRI study. Front. Aging Neurosci. 11:276. doi: 10.3389/fnagi.2019.00276
Liu, Y., Zhu, J., Li, Q., Wang, Y., Li, Y., Chen, J., et al. (2020). Differences in the amplitude of low-frequency fluctuation between methamphetamine and heroin use disorder individuals: a resting-state fMRI study. Brain Behav. 10:e01703. doi: 10.1002/brb3.1703
Ma, X., Zheng, W., Li, C., Li, Z., Tang, J., Yuan, L., et al. (2019). Decreased regional homogeneity and increased functional connectivity of default network correlated with neurocognitive deficits in subjects with genetic high-risk for schizophrenia: a resting-state fMRI study. Psychiatry Res. 281:112603. doi: 10.1016/j.psychres.2019.112603
Malcolm, R., Myrick, H., Li, X., Henderson, S., Brady, K. T., George, M. S., et al. (2016). Regional brain activity in abstinent methamphetamine dependent males following cue exposure. J. Drug Abuse 2:16. doi: 10.21767/2471-853x.100016
Meade, C. S., Bell, R. P., Towe, S. L., and Hall, S. A. (2020). Cocaine-related alterations in fronto-parietal gray matter volume correlate with trait and behavioral impulsivity. Drug Alcohol Depend. 206:107757. doi: 10.1016/j.drugalcdep.2019.107757
Mizoguchi, H., and Yamada, K. (2019). Methamphetamine use causes cognitive impairment and altered decision-making. Neurochem. Int. 124, 106–113. doi: 10.1016/j.neuint.2018.12.019
Mo, S., Feng, S., and Chen, H. (2018). [Research on the rest functional magnetic resonance imaging before and after smoking cessation]. Sheng Wu Yi Xue Gong Cheng Xue Za Zhi 35, 87–91. doi: 10.7507/1001-5515.201609026
Moallem, N. R., Courtney, K. E., and Ray, L. A. (2018). The relationship between impulsivity and methamphetamine use severity in a community sample. Drug Alcohol Depend. 187, 1–7. doi: 10.1016/j.drugalcdep.2018.01.034
Park, B. Y., Shim, W. M., James, O., and Park, H. (2019). Possible links between the lag structure in visual cortex and visual streams using fMRI. Sci. Rep. 9:4283. doi: 10.1038/s41598-019-40728-x
Patton, J. H., Stanford, M. S., and Barratt, E. S. (1995). Factor structure of the Barratt impulsiveness scale. J. Clin. Psychol. 51, 768–774. doi: 10.1002/1097-4679(199511)51:6<768::aid-jclp2270510607<3.0.co;2-1
Psederska, E., Thomson, N. D., Bozgunov, K., Nedelchev, D., Vasilev, G., and Vassileva, J. (2021). Effects of psychopathy on neurocognitive domains of impulsivity in abstinent opiate and stimulant users. Front. Psychiatry 12:660810. doi: 10.3389/fpsyt.2021.660810
Qiu, Y., Lv, X., Su, H., Jiang, G., Tian, J., Zhuo, F., et al. (2013). Reduced regional homogeneity in bilateral frontostriatal system relates to higher impulsivity behavior in codeine-containing cough syrups dependent individuals. PLoS One 8:e78738. doi: 10.1371/journal.pone.0078738
Salo, R., Fassbender, C., Buonocore, M. H., and Ursu, S. (2013). Behavioral regulation in methamphetamine abusers: an fMRI study. Psychiatry Res. 211, 234–238. doi: 10.1016/j.pscychresns.2012.10.003
Schwartz, D. L., Mitchell, A. D., Lahna, D. L., Luber, H. S., Huckans, M. S., Mitchell, S. H., et al. (2010). Global and local morphometric differences in recently abstinent methamphetamine-dependent individuals. Neuroimage 50, 1392–1401. doi: 10.1016/j.neuroimage.2010.01.056
Schwarzbach, V., Lenk, K., and Laufs, U. (2020). Methamphetamine-related cardiovascular diseases. ESC Heart Fail. 7, 407–414. doi: 10.1002/ehf2.12572
Shadloo, B., Amin-Esmaeili, M., Haft-Baradaran, M., Noroozi, A., Ghorban-Jahromi, R., and Rahimi-Movaghar, A. (2017). Use of amphetamine-type stimulants in the Islamic Republic of Iran, 2004-2015: a review. East Mediterr. Health J. 23, 245–256. doi: 10.26719/2017.23.3.245
Shan, X., Liao, R., Ou, Y., Pan, P., Ding, Y., Liu, F., et al. (2021). Increased regional homogeneity modulated by metacognitive training predicts therapeutic efficacy in patients with schizophrenia. Eur. Arch. Psychiatry Clin. Neurosci. 271, 783–798. doi: 10.1007/s00406-020-01119-w
Starcke, K., Antons, S., Trotzke, P., and Brand, M. (2018). Cue-reactivity in behavioral addictions: a meta-analysis and methodological considerations. J. Behav. Addict. 7, 227–238. doi: 10.1556/2006.7.2018.39
Subramanian, K., Menon, V., Sarkar, S., Chandrasekaran, V., and Selvakumar, N. (2020). Study of risk factors associated with suicide attempt in patients with bipolar disorder type I. J. Neurosci. Rural Pract. 11:291.
Vassileva, J., and Conrod, P. J. (2019). Impulsivities and addictions: a multidimensional integrative framework informing assessment and interventions for substance use disorders. Philos. Trans. R. Soc. Lond. B Biol. Sci. 374:20180137. doi: 10.1098/rstb.2018.0137
Wang, C., Shen, Z., Huang, P., Yu, H., Qian, W., Guan, X., et al. (2017). Altered spontaneous brain activity in chronic smokers revealed by fractional ramplitude of low-frequency fluctuation analysis: a preliminary study. Sci. Rep. 7:328. doi: 10.1038/s41598-017-00463-7
Wang, Y., Zhu, J., Li, Q., Li, W., Wu, N., Zheng, Y., et al. (2013). Altered fronto-striatal and fronto-cerebellar circuits in heroin-dependent individuals: a resting-state FMRI study. PLoS One 8:e58098. doi: 10.1371/journal.pone.0058098
Wang, Y., Zuo, J., Hao, W., Shen, H., Zhang, X., Deng, Q., et al. (2020). Quality of life in patients with methamphetamine use disorder: relationship to impulsivity and drug use characteristics. Front. Psychiatry 11:579302. doi: 10.3389/fpsyt.2020.579302
Weafer, J., Van Hedger, K., Keedy, S. K., Nwaokolo, N., and de Wit, H. (2020). Methamphetamine acutely alters frontostriatal resting state functional connectivity in healthy young adults. Addict. Biol. 25:e12775. doi: 10.1111/adb.12775
Winhusen, T., Lewis, D., Adinoff, B., Brigham, G., Kropp, F., Donovan, D. M., et al. (2013). Impulsivity is associated with treatment non-completion in cocaine- and methamphetamine-dependent patients but differs in nature as a function of stimulant-dependence diagnosis. J. Subst. Abuse Treat. 44, 541–547. doi: 10.1016/j.jsat.2012.12.005
Xie, A., Wu, Q., Yang, W. F. Z., Qi, C., Liao, Y., Wang, X., et al. (2021). Altered patterns of fractional amplitude of low-frequency fluctuation and regional homogeneity in abstinent methamphetamine-dependent users. Sci. Rep. 11:7705. doi: 10.1038/s41598-021-87185-z
Yalachkov, Y., Kaiser, J., and Naumer, M. J. (2010). Sensory and motor aspects of addiction. Behav. Brain Res. 207, 215–222. doi: 10.1016/j.bbr.2009.09.015
Yan, C. G., Wang, X. D., Zuo, X. N., and Zang, Y. F. (2016). DPABI: data processing & analysis for (resting-state) brain imaging. Neuroinformatics 14, 339–351. doi: 10.1007/s12021-016-9299-4
Yang, M., Jia, X., Zhou, H., Ren, P., Deng, H., Kong, Z., et al. (2021). Brain dysfunction of methamphetamine-associated psychosis in resting state: approaching schizophrenia and critical role of right superior temporal deficit. Addict. Biol. 26:e13044. doi: 10.1111/adb.13044
Yao, S., Yang, H., Zhu, X., Auerbach, R. P., Abela, J. R., Pulleyblank, R. W., et al. (2007). An examination of the psychometric properties of the Chinese version of the Barratt Impulsiveness Scale, 11th version in a sample of Chinese adolescents. Percept. Mot. Skills 104(3 Pt 2), 1169–1182. doi: 10.2466/pms.104.4.1169-1182
Keywords: methamphetamine, impulsivity, regional homogeneity, resting state, functional magnetic resonance image
Citation: Zhou Y, Wang Q, Ren H, Wang X, Liao Y, Yang Z, Hao Y, Wang Y, Li M, Ma Y, Wu Q, Wang Y, Yang D, Xin J, Yang WFZ, Wang L and Liu T (2022) Regional Homogeneity Abnormalities and Its Correlation With Impulsivity in Male Abstinent Methamphetamine Dependent Individuals. Front. Mol. Neurosci. 14:810726. doi: 10.3389/fnmol.2021.810726
Received: 07 November 2021; Accepted: 27 December 2021;
Published: 20 January 2022.
Edited by:
Jianfeng Liu, Texas A&M University, United StatesReviewed by:
Yixiao Luo, Hunan Normal University, ChinaJiajia Zhu, First Affiliated Hospital of Anhui Medical University, China
Xiang Yang Zhang, Institute of Psychology, Chinese Academy of Sciences (CAS), China
Copyright © 2022 Zhou, Wang, Ren, Wang, Liao, Yang, Hao, Wang, Li, Ma, Wu, Wang, Yang, Xin, Yang, Wang and Liu. This is an open-access article distributed under the terms of the Creative Commons Attribution License (CC BY). The use, distribution or reproduction in other forums is permitted, provided the original author(s) and the copyright owner(s) are credited and that the original publication in this journal is cited, in accordance with accepted academic practice. No use, distribution or reproduction is permitted which does not comply with these terms.
*Correspondence: Winson Fu Zun Yang, winson.yang@ttu.edu; Long Wang, 742978062@qq.com; Tieqiao Liu, liutieqiao123@csu.edu.cn
†These authors have contributed equally to this work