- 1Department of Radiology, People's Hospital of Tongchuan City, Tongchuan, China
- 2Department of Ultrasound, People's Hospital of Tongchuan City, Tongchuan, China
- 3Department of Radiology, Zhongnan Hospital of Wuhan University, Wuhan, China
Hypertension with high homocysteine (Hcy, ≥10 μmol/L) is also known as H-type hypertension (HHT) and proposed as an independent risk factor for stroke and cognitive impairment. Although previous studies have established the relationships among hypertension, Hcy levels, and cognitive impairment, how they affect brain neuroanatomy remains unclear. Thus, we aimed to investigate whether and to what extent hypertension and high Hcy may affect gray matter volume in 52 middle-aged HHT patients and 51 demographically matched normotensive subjects. Voxel-based morphological analysis suggested that HHT patients experienced significant gray matter loss in the default network. The default network atrophy was significantly correlated with Hcy level and global cognitive function. These findings provide, to our knowledge, novel insights into how HHT affects brain gray matter morphology through blood pressure and Hcy.
Introduction
Hypertension is a well-known risk factor for stroke and cognitive impairment. In China, about 75% of primary hypertension is accompanied by high homocysteine (plasma Hcy ≥ 10 μmol/L) and is defined as H-type hypertension (HHT) (1). High Hcy is an independent risk factor for cerebrovascular events (2, 3) and cognitive impairment (4–7). Despite these prominent dual toxic effects, little is known about the neuroanatomical basis for cognitive impairment in HHT. Investigation upon the neuroanatomical basis may help to understand how dual cerebrovascular risk factors exacerbate the impact of brain morphological integrity, promoting a targeted prevention of risk factors and progression monitoring.
One potential neuroimaging biomarker that encodes cognitive impairment is integrity of the default mode network (DMN). The DMN was first proposed by Raichle et al. (8) and anatomically comprises the posterior cingulate/precuneus, bilateral inferior parietal lobule, lateral temporal, and hippocampus (9, 10). The DMN regions show less activity during goal-directed tasks and greater activity during resting state or several particular social cognitive tasks (9, 10). The DMN is considered to be a critical basis for advanced cognition of humans during development and evolution. Moreover, the DMN has been proposed as a neural biomarker for cognitive impairment in neuroimaging community. For example, DMN connectivity differentiates Alzheimer disease (11), mild cognitive impairment (12), mild traumatic injury (13, 14), and chemotherapy-related (15) cognitive impairment.
Previous studies on primary hypertension have shown evidence on the DMN. For example, primary hypertension is associated with increased coupling between the core and the dorsal medial subpart of the DMN during resting state (16) and abnormal spontaneous activity in DMN in a rodent model (17).
Etiologically, hypertension is a group of syndromes with high heterogeneity; each subgroup classification may represent different outcomes. Among the various types of hypertensions, HHT is the most common in China and has been suggested to predict more adverse consequences and outcomes (1). Essentially, HHT is a, at least, silent, cerebrovascular disease, often associated with higher silent infarcts, white matter hyperintensity (WMH), and gray matter loss (1, 18–24). These silent lesions represent physical dysconnectivity, compromised coordination between interconnected gray matter regions, and progressive atrophy itself. We know that cortical gray matter integrity is the neuroanatomical basis of large-scale brain networks that support human behaviors and cognition, and mounting evidence links gray matter atrophy to cognitive impairment (25–30). However, it is unclear that whether and to what extent HHT may affect the DMN gray matter morphometry.
Voxel-based morphometry (VBM) serves as a non-invasive, automatic framework for predicting brain disease and aging related model (31–34). Previously, it has been reported that hypertensive individuals experienced shrinkage of gray matter (35). Moreover, in dementia and mild cognitive impairment, the DMN appears to be the most impacted and has the ability to be a potential imaging biomarker (16, 36, 37). In both human and rodent studies, higher serum Hcy is associated with significant brain atrophy (38, 39); for more details, please see de Jager (40). Together, these evidences suggest that HHT is an adverse factor in the atrophy of DMN and cognitive performance and may interact with particular ways.
Here, we aimed to investigate whether HHT is associated with significant gray matter loss in the DMN and the relationships among the DMN atrophy, Hcy level, and global cognition. We hypothesized that HHT was associated with significant gray matter loss in the DMN, and Hcy level significantly mediated the relationship between DMN atrophy and global cognitive behavioral variables in patients with HHT.
Materials and Methods
Subjects
Fifty-two H-type hypertensive subjects (26 females and 26 males, mean age 53.20 ± 10.98 years) and 51 normotensive healthy adults (33 females and 18 males, mean age 50.84 ± 9.92 years) were enrolled in this study. Informed consent was obtained from all the participants, and the Ethical Committee for Human Research at the People's Hospital of Tongchuan City approved all the procedures in accordance with the Declaration of Helsinki.
All of subjects were screened and diagnosed by a physician. All the subjects received comprehensive neurological examination and blood and neurocognitive tests. The inclusion criteria for the HHT subjects were as follows: (a) 18–65 years; (b) right-handed; (c) conforming to diagnostic criteria for HHT, i.e., serum Hcy ≥ 10 μmol/L and clinical systolic pressure >140 mmHg and/or diastolic pressure >90 mmHg; (d) currently not receiving systemically antihypertensive treatment; and (e) no contraindications for magnetic resonance imaging (MRI) examination.
Exclusion criteria were as follows: (a) any previous cardiovascular or cerebrovascular event; (b) large-scale old cerebral infarction and cerebral hemorrhage; (c) major diseases, including psychiatric diseases, renal failure (serum creatinine level >176 mmol/L), liver damage (aspartate aminotransferase or alanine aminotransferase >40 IU/L), coronary heart disease, congestive heart failure, or history of malignant tumors; (d) structural abnormalities that could impair cognitive function; and (e) low education years (<6 years).
Data Collection
We used a standardized questionnaire to record demographic information, life style, and medical history for each participant. Medical history including cardiovascular factors (hypertension, hyperlipidemia, stroke, coronary artery disease), diabetes complications, medication use, and age of diabetes onset were recorded using a standardized questionnaire. Body mass index (BMI) was calculated as weight/height (kg/m2). Neurocognitive tests were administered to examine global cognition function with the Mini-Mental State Examination (MMSE) (41, 42). Neurocognitive tests and questionnaire were administered by a trained neuropsychologist and nurse staff. The diastolic and systolic blood pressures were measured by a trained staff after resting for at least 5 min in a seated position.
MRI Acquisition and Data Processing
Structural brain images, including 3D T1-weighted fast field echo (3D T1-FFE, repetition time = 8.29 ms, echo time = 3.81 ms, flip angle = 8°, slice thickness = 1 mm, 160 axial slices covering whole brain) high-resolution anatomical and T2-fluid-attenuated inversion recovery (FLAIR, repetition time = 8,000 ms, echo time = 140 ms, inversion time = 2,200 ms, flip angle = 90°, slice thickness = 1 mm, 160 axial slices covering whole brain) images, were acquired on a 1.5T MRI (Phillips, Achieva, Andover, MA, USA) scanner equipped with an eight-channel head coil.
T1 structural images were processed using the computational anatomy toolbox (CAT, version 12.6; http://www.neuro.uni-jena.de/cat/) implanted in SPM12 (www.fil.ion.ucl.ac.uk/spm). Briefly, T1 images were first visually inspected and manually reoriented to ensure image quality and whole brain covering and further segmented into gray matter, white matter, and cerebral spinal fluid, with the diffeomorphic anatomical registration through an exponentiated lie algebra (DARTEL) algorithm (31). The individual gray matter images were then spatially normalized into the Montreal Neurological Institute (MNI) standard space and modulated. The modulated and spatial normalized gray matter images were then smoothed with an isotropic Gaussian kernel of 8 mm full-width at half-maximum. The FLAIR images were used for manually calculating white matter hyperintensity (WMH) volume for each participant.
Two HHT subjects and one control were excluded from the analysis due to poor image quality or segment failure.
Statistical Analysis
Demographic and clinical variables, Hcy, and cognitive tests were analyzed using SPSS 16 (SPSS Inc., USA). Categorical variables (including gender, diabetes, obesity, smoking, drinking, etc.) were tested using the chi-square test, while the remaining variables were tested using independent two-sample t-tests. The significance was set at p < 0.05. Differences/associations with a p < 0.05 were considered to be statistically significant.
Mediation Analysis
Since prior research has suggested that Hcy level (X, independent variable) can predict cognitive functions (Y, dependent variable) (43), we considered to take the DMN gray matter volume as a mediator (M) and build a mediation model. The mediation analysis was conducted by using the Hayes Process macro (44) (http://www.afhayes.com/), which is an observed least-squares regressions path analysis modeling tool. We used a model 4, with the independent factor as Hcy level and dependent variable as the cognitive measures (i.e., the MMSE scores), and the proposed mediator was DMN gray matter size. Age, gender, and education were controlled as nuisance of no interest. Statistical significance was set at p < 0.05.
Results
Demographic and Clinical Data
Demographic and clinical data of all the participants are presented in Table 1. We excluded two HHT subjects and one control subject from data analysis due to poor image quality or segment failure; thus, a total of 100 subjects were finally included. The HHT subjects demonstrated an average Hcy of 21.79 ± 10.97 μmol/L compared with the normotensive controls (11.47 ± 7.65 μmol/L, p < 0.05). In addition, the HHT subjects demonstrated lower MMSE scores (p = 0.006) and higher but non-significant frequency of vascular risks, including diabetes mellitus, hyperlipidemia, and obesity (p > 0.05). However, the hypertensive subjects were generally comparable with normotensive controls on age, gender, and education (p > 0.05).
Voxel-Wise Gray Matter Volume
To investigate gray matter volume differences, we performed voxel-based morphological analysis. Our findings showed that HHT subjects exhibited smaller gray matter volume in several clusters in the DMN, including the left inferior parietal, right medial prefrontal, precuneus, and bilateral middle temporal gyri and additionally in the left lateral frontal, left precentral, and right superior occipital and left cerebellum crus regions [Table 2, Figure 1; voxel-wise p < 0.001 and family-wise error (FWE) cluster corrected at cluster-level p < 0.05].
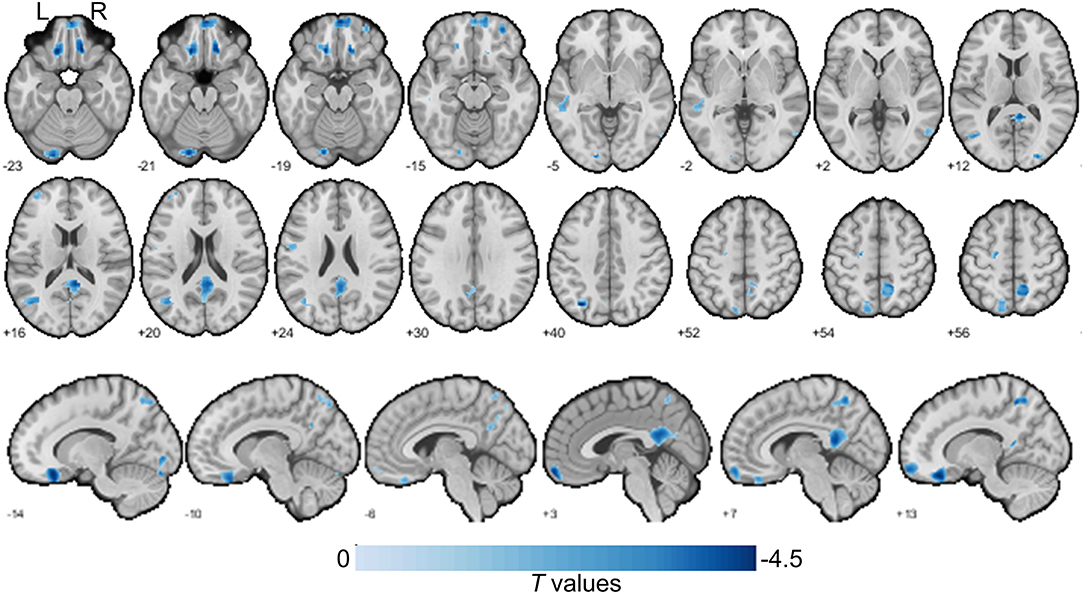
Figure 1. Gray matter size difference between the H-type hypertensive and normotensive subjects. The axial slices are labeled with Z coordinates and sagittal slices with Y coordinates. The results were reported with voxel-wise p < 0.001 and family-wise error (FWE) cluster corrected at cluster-level p < 0.05. A negative colorbar indicates gray matter losses in the H-type hypertensive subjects. Regions were automatically reported and labeled using BSPMVIEW (http://www.bobspunt.com/software/bspmview) and automated anatomical labeling atlas 3 (https://www.gin.cnrs.fr/en/tools/aal/). L, left; R, right.
Brain Size and White Matter Lesion Burden
The HHT subjects showed larger intracranial volume (p = 0.042) and cerebrospinal fluid volumes (p = 0.049), and higher WMH burden (p = 0.015), but comparable gray matter and white matter sizes (Table 3).
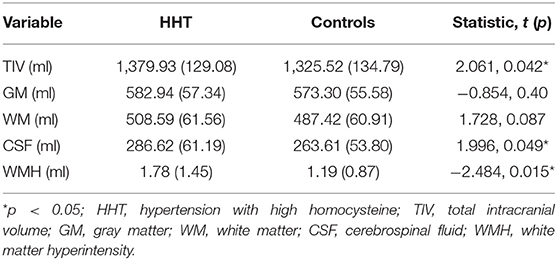
Table 3. Gross segment volumes and white matter hyperintensity burden in the two groups included in this study.
Association Analyses
To determine the relationships between brain (WMH and gray matter volume) and behavioral and physiological parameters, we conducted linear correlation analyses. Results showed that gray matter in the DMN and thalamus was negatively correlated with WMH but positively correlated with MMSE scores. In other words, the DMN gray matter size predicted white matter lesion load and general cognition, although there was no significant relationship between WMH and MMSE.
We also found that increased systolic blood pressure was associated with decreased gray matter size within the thalamus and DMN (Figures 2, 3). We also determined correlations between the whole brain (excluding the significant clusters) voxel-wise gray matter volume and blood pressure (BP), MMSE, Hcy, and WMH loads (Figure 3).
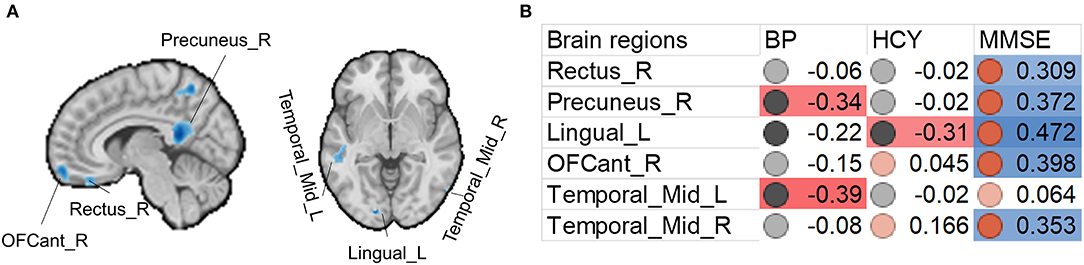
Figure 2. Associations between gray matter size within the significant clusters and clinical (blood pressure), physiological (Hcy level), and behavioral (MMSE scores) parameters in H-type hypertensive subjects. (A) Among the significant clusters, brain regions showing at least one significant correlation (B) are marked out. (B) Heatmap showing Pearson's correlation coefficients between gray matter size within the significant clusters and clinical (blood pressure), physiological (Hcy level), and behavioral (MMSE scores) parameters in H-type hypertensive subjects. Significant correlations are marked with warm (positive) or cold (negative) colors, and non-significant correlations are marked with white color. BP, blood pressure based measure, here hypertension grades 1–3; HCY, homocysteine; MMSE, Mini-Mental State Examination.
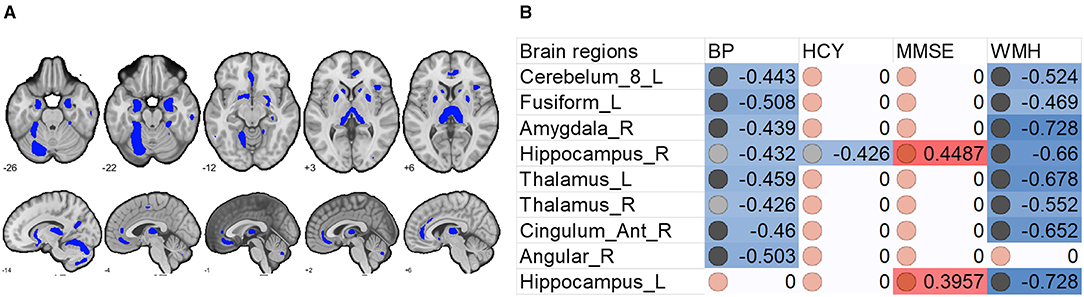
Figure 3. Associations between whole brain gray matter size (excluding the significant clusters) and clinical (blood pressure), physiological (Hcy level), and behavioral (MMSE scores) parameters, and lesion (WMH load) in H-type hypertensive subjects. (A) Excluding the significant clusters, brain regions showing at least one significant correlation (B) are marked out. (B) Heatmap showing Pearson's correlation coefficients between gray matter size within the significant clusters and clinical (blood pressure), physiological (Hcy level), and behavioral (MMSE scores) parameters, and lesion (WMH load) in H-type hypertensive subjects. Significant correlations are marked with warm (positive) or cold (negative) colors, and non-significant correlations are marked with white color. BP, blood pressure based measure, here hypertension grades 1–3; Hcy, homocysteine; MMSE, Mini-Mental State Examination; WMH, white matter hyperintensity.
Mediation Analyses
To investigate whether the gray matter loss significantly affected the association between Hcy and cognitive performance, we used a mediation model to answer this issue. Unfortunately, we did not find any mediation effect of gray matter volume on the reported significant clusters.
Discussion
In this study, we investigated gray matter atrophy underlying HHT and the associations with cognitive performance and plasma Hcy, while considering multiple vascular variables. We found that HHT patients showed significant DMN atrophy, which can reflect Hcy level, global cognitive function (MMSE), and WMH load. HHT patients demonstrated parallelized gray matter atrophy and global cognitive decline, suggesting that both hypertension and high Hcy aggravate the neuroanatomical and cognitive toxic effects.
Consistent with our hypothesis and previous published reports (16, 35), we found significant gray matter loss in the DMN and sensorimotor regions in the HHT subjects. The DMN has been well-characterized in advanced cognitive functions, including autobiographical memory, social cognition, memory consolidation, and reasoning; for more details, please see Buckner and DiNicola (9) and Raichle (10). Brain regions that constitute the DMN include the inferior parietal lobule that is associated with spatial orientation, the medial prefrontal cortex that is linked to reasoning, precuneus that is linked to social cognition, and middle temporal region that is involved in multimodal sensory processing and social cognition. These components are crucial to advanced cognitive functions (43), have been linked to cognitive impairment in Alzheimer's disease (37, 45), and also generally align to the findings from rodents and other animals (39, 46) in HHT. Anatomically, lateral temporal regions receive anterior circulation whose arteries emerge from the Sylvian fissure, thus a potential focus that is targeted by the hypertension-induced hypoperfusion. Also, these key DMN regions are a marker for cognitive impairment and heavily hit regions of altered hemodynamics. Thus, the DMN atrophy reported here is consistent but beyond the previous published reports on sole or mixed primary hypertension.
Apart from the DMN, we also identified gray matter loss in the left lateral prefrontal, left precentral, right occipital, and cerebellum regions. These regions are mainly involved in sensory and motor processing. For example, the left inferior frontal region is a classical Broca's area, responsible for speech and other higher motor functions, and is also a vulnerable foci for stroke and other cerebrovascular events (47). The precentral region is involved in the somatomotor system and associated with physical fitness and well-being (48, 49). The occipital is also largely a primary sensory cortex and involved in visuospatial processing. Visuospatial deficit is also supposed as a biomarker of Alzheimer's disease (50).
Beyond the gray matter atrophy, we also found significant associations. We first report the associations between significant atrophic clusters with blood pressure, Hcy, and MMSE scores. Gray matter volume in the precuneus and left middle temporal gyrus was negatively correlated with blood pressure; gray matter volume in the left lingual cortex was negatively correlated with plasma Hcy. Nevertheless, we found that the MMSE scores were correlated with almost all the significant clusters, thereby suggesting the role of these regions in global cognition. This finding is in line with many studies investigating the brain anatomical correlates with global cognition (25–30). The precuneus is a core of the DMN, and this result is also in line with other published reports on vascular cognitive impairment (51–53). The middle temporal gyrus is a vulnerable locus attacked by the vascular pathologies. For example, in patients with carotid stenosis, the same region is the most impacted (51–53).
The finding that HHT showed higher WMH load and correlated with lower gray matter is generally consistent with previous reports. For example, previous cohort studies have reported positive associations between elevated Hcy and WMH and silent infarction (54–56) but inconsistent findings on whether these factors act as mediators (54). However, in healthy aging and some major brain diseases, WMH load tends to associate with regional gray matter atrophy (57, 58).
Hcy and cognitive impairment together promote brain atrophy, especially when they cross a threshold level (40). Therefore, we were also interested in investigating whether there is a relationship between high Hcy and cognitive decline mediated by gray matter atrophy in HHT subjects. Unfortunately, due to the lack of a significant correlation between Hcy and global cognition, our mediation model could not confirm this hypothesis. One possibility is that Hcy is a non-specific measure, especially when it is associated with non-specific global cognitive measures such as MMSE. Another possibility, as mentioned at the beginning of this paragraph, is that Hcy needs to reach a certain threshold level to establish a parallel relationship with cognitive impairment, which makes this problem more complicated. In addition, HHT, as a kind of vascular cognitive impairment, may need more sensitive tests focused on specific cognitive domains (59–63). We also found slightly elevated Hcy in the controls, suggesting independent risk factor in this population. Although this does not necessarily provide any indications, it suggests a possibility that there may be regional and dietary predisposition in this population, which may also be the reason for the high incidence of HHT (64). Nevertheless, investigations on this topic are still lacking. However, HHT subjects demonstrated far higher Hcy; this may become highly unfavorable.
We found that the HHT group had larger intracranial and cerebrospinal fluid volumes. One reason is that the HHT group had a higher proportion of males, although there was no statistical difference in the gender ratio; thus, we included gender as a nuisance covariate in all the analyses. At the same time, patients with hypertension have more WMHs, which is consistent with clinical observation and previous published report (24), thereby suggesting that HHT is accompanied by more white matter damage burden than the normotensive controls.
Although we have reported some novel findings, several limitations of our study cannot be neglected. First, we could not build the casual relationship between Hcy and hypertension, which might affect the statistical model; nonetheless the current findings suggest dual adverse effects from HHT. Further study may validate the casual relationship by considering more variables, including WMH load, blood pressure classification, vitamins, and other risk factors. Second, factors affecting gray matter atrophy also include hemodynamic changes (65) and lacunar infarction (66–68). Although these two aspects are still poorly characterized, they may contribute to brain atrophy to varying degrees. Future research should include these factors as explanatory variables and promote a more comprehensive model. Third, we only measured global cognition for each subject; this could hamper the ability to classify between-group differences. Increasing evidence suggests that vascular cognitive impairment is more likely to affect processing speed and semantic/recall memory, which is different from the pattern of global cognitive impairment caused by Alzheimer's disease (60, 69–71). Therefore, we need to further include domain-specific tests, such as the Digit Symbol (72) and the Rey Auditory Verbal Learning Tests (73), to accurately characterize the affected cognitive domains that are most sensitive to HHT and its underlying neuroanatomical and physiological basis.
Conclusion
In conclusion, this study reports that HHT is associated with default network atrophy and correlated with WMH, Hcy level, and MMSE score. These results provide, to our knowledge, the first insights into how HHT affects brain gray matter morphology and how these three risk factors interact with one another.
Data Availability Statement
The original contributions presented in the study are included in the article/supplementary material, further inquiries can be directed to the corresponding author.
Ethics Statement
The studies involving human participants were reviewed and approved by the Ethical Committee for Human Research at the People's Hospital of Tongchuan City. The patients/participants provided their written informed consent to participate in this study.
Author Contributions
YK collected data and wrote the draft. XL wrote the draft. LC, YL, and LJ collected data and analyzed data. LG analyzed data and proofread the manuscript. LR conceived and revised the manuscript. All authors contributed to the article and approved the submitted version.
Funding
This work was supported by the Natural Science Foundation of China (Grant No. 82001799).
Conflict of Interest
The authors declare that the research was conducted in the absence of any commercial or financial relationships that could be construed as a potential conflict of interest.
Publisher's Note
All claims expressed in this article are solely those of the authors and do not necessarily represent those of their affiliated organizations, or those of the publisher, the editors and the reviewers. Any product that may be evaluated in this article, or claim that may be made by its manufacturer, is not guaranteed or endorsed by the publisher.
Acknowledgments
We thank all the subjects for their active participation and cooperation. We also thank Dr. Yawen Ren (Clinical Laboratory Director), Xiaoyan Yan (Division of Chronic Disease), Baiqiang Yang (Medical Department), Xiaoping He (General Medicine Section), and Liping Cheng (Branch Training Section) of People's Hospital of Tongchuan City for their kind help in subject recruitment and data collection.
References
1. Lu Z, Li J, Li X, Ding M, Mao C, Zhu X, et al. Hypertension with hyperhomocysteinemia increases the risk of early cognitive impairment after first-ever ischemic stroke. Eur Neurol. (2019) 82:75–85. doi: 10.1159/000504704
2. Hassan A, Hunt BJ, O'Ullivan M, Bell R, D'Ouza R, Jeffery S, et al. Homocysteine is a risk factor for cerebral small vessel disease, acting via endothelial dysfunction. Brain. (2004) 127:212–9. doi: 10.1093/brain/awh023
3. Ostrakhovitch EA, Tabibzadeh S. Homocysteine and age-associated disorders. Ageing Res Rev. (2019) 49:144–64. doi: 10.1016/j.arr.2018.10.010
4. Garcia A, Zanibbi K. Homocysteine and cognitive function in elderly people. CMAJ. (2004) 171:897–904. doi: 10.1503/cmaj.1031586
5. Price BR, Wilcock DM, Weekman EM. Hyperhomocysteinemia as a risk factor for vascular contributions to cognitive impairment and dementia. Front Aging Neurosci. (2018) 10:350. doi: 10.3389/fnagi.2018.00350
6. Sala I, Sánchez-Saudinós MB, Molina-Porcel L, Lázaro E, Gich I, Clarimón J, et al. Homocysteine and cognitive impairment. Dement Geriatr Cogn Disord. (2008) 26:506–12. doi: 10.1159/000173710
7. Zhou S, Chen J, Cheng L, Fan K, Xu M, Ren W, et al. Age-dependent association between elevated homocysteine and cognitive impairment in a post-stroke population: a prospective study. Front Nutr. (2021) 8:691837. doi: 10.3389/fnut.2021.691837
8. Raichle ME, MacLeod AM, Snyder AZ, Powers WJ, Gusnard DA, Shulman GL. A default mode of brain function. Proc Nat Acad Sci USA. (2001) 98:676–82. doi: 10.1073/pnas.98.2.676
9. Buckner RL, DiNicola LM. The brain's default network: updated anatomy, physiology and evolving insights. Nat Rev Neurosci. (2019) 20:593–608. doi: 10.1038/s41583-019-0212-7
10. Raichle ME. The brain's default mode network. Annu Rev Neurosci. (2015) 38:433–47. doi: 10.1146/annurev-neuro-071013-014030
11. Greicius MD, Srivastava G, Reiss AL, Menon V. Default-mode network activity distinguishes Alzheimer's disease from healthy aging: evidence from functional MRI. Proc Nat Acad Sci USA. (2004) 101:4637–42. doi: 10.1073/pnas.0308627101
12. Bai F, Zhang Z, Yu H, Shi Y, Yuan Y, Zhu W, et al. Default-mode network activity distinguishes amnestic type mild cognitive impairment from healthy aging: a combined structural and resting-state functional MRI study. Neurosci Lett. (2008) 438:111–5. doi: 10.1016/j.neulet.2008.04.021
13. Sharp DJ, Beckmann CF, Greenwood R, Kinnunen KM, Bonnelle V, De Boissezon X, et al. Default mode network functional and structural connectivity after traumatic brain injury. Brain. (2011) 134:2233–47. doi: 10.1093/brain/awr175
14. Zhou Y, Milham MP, Lui YW, Miles L, Reaume J, Sodickson DK, et al. Default-mode network disruption in mild traumatic brain injury. Radiology. (2012) 265:882–92. doi: 10.1148/radiol.12120748
15. Kesler SR, Wefel JS, Hosseini SH, Cheung M, Watson CL, Hoeft F. Default mode network connectivity distinguishes chemotherapy-treated breast cancer survivors from controls. Proc Nat Acad Sci USA. (2013) 110:11600–5. doi: 10.1073/pnas.1214551110
16. Gu Y, Liu R, Qin R, Chen X, Zou J, Jiang Y, et al. Characteristic changes in the default mode network in hypertensive patients with cognitive impairment. Hypertension Res. (2019) 42:530–40. doi: 10.1038/s41440-018-0176-4
17. Huang S, Wu Y, Peng S, Peng H, Huang T, Ho K, et al. Inter-strain differences in default mode network: a resting state fmri study on spontaneously hypertensive rat and Wistar Kyoto rat. Sci Rep. (2016) 6:21697. doi: 10.1038/srep21697
18. Ji Y, Li X, Teng Z, Li X, Jin W, Lv PY. Homocysteine is associated with the development of cerebral small vessel disease: retrospective analyses from neuroimaging and cognitive outcomes. J Stroke Cerebrovasc Dis. (2020) 29:105393. doi: 10.1016/j.jstrokecerebrovasdis.2020.105393
19. Dufouil C, Alpérovitch A, Ducros V, Tzourio C. Homocysteine, white matter hyperintensities, and cognition in healthy elderly people. Ann Neurol. (2003) 53:214–21. doi: 10.1002/ana.10440
20. Feng L, Isaac V, Sim S, Ng T, Krishnan KRR, Chee MW. Associations between elevated homocysteine, cognitive impairment, and reduced white matter volume in healthy old adults. Am J Geriatric Psychiatry. (2013) 21:164–72. doi: 10.1016/j.jagp.2012.10.017
21. Glodzik L, Rusinek H, Tsui W, Pirraglia E, Kim H, Deshpande A, et al. Different relationship between systolic blood pressure and cerebral perfusion in subjects with and without hypertension. Hypertension. (2019) 73:197–205. doi: 10.1161/HYPERTENSIONAHA.118.11233
22. Lee KO, Woo M, Chung D, Choi J, Kim N, Kim O, et al. Differential impact of plasma homocysteine levels on the periventricular and subcortical white matter hyperintensities on the brain. Front Neurol. (2019) 10:1174. doi: 10.3389/fneur.2019.01174
23. Li T, Liu X, Diao S, Kong Y, Duan X, Yang S, et al. H-type hypertension is a risk factor for cerebral small-vessel disease. BioMed Res Int. (2020) 2020:6498903. doi: 10.1155/2020/6498903
24. Li X, Liang Y, Chen Y, Zhang J, Wei D, Chen K, et al. Disrupted frontoparietal network mediates white matter structure dysfunction associated with cognitive decline in hypertension patients. J Neurosci. (2015) 35:10015–24. doi: 10.1523/JNEUROSCI.5113-14.2015
25. Fein G, Di Sclafani V, Tanabe J, Cardenas V, Weiner MW, Jagust WJ, et al. Hippocampal and cortical atrophy predict dementia in subcortical ischemic vascular disease. Neurology. (2000) 55:1626–35. doi: 10.1212/WNL.55.11.1626
26. Han Y, Lui S, Kuang W, Lang Q, Zou L, Jia J. Anatomical and functional deficits in patients with amnestic mild cognitive impairment. PLoS ONE. (2012) 7:e28664. doi: 10.1371/journal.pone.0028664
27. Jack CR, Shiung MM, Weigand SDO, Brien PC, Gunter JL, Boeve BF, et al. Brain atrophy rates predict subsequent clinical conversion in normal elderly and amnestic MCI. Neurology. (2005) 65:1227–31. doi: 10.1212/01.wnl.0000180958.22678.91
28. Li J, Gao L, Wen Z, Zhang J, Wang P, Tu N, et al. Structural covariance of gray matter volume in HIV vertically infected adolescents. Sci Rep. (2018) 8:1182. doi: 10.1038/s41598-018-19290-5
29. Mok V, Wong KK, Xiong Y, Wong A, Schmidt R, Chu W, et al. Cortical and frontal atrophy are associated with cognitive impairment in age-related confluent white-matter lesion. J Neurol Neurosurg Psychiatry. (2011) 82:52–7. doi: 10.1136/jnnp.2009.201665
30. van de Mortel LA, Thomas RM, van Wingen GA, Alzheimer's Disease Neuroimaging Initiative. Grey matter loss at different stages of cognitive decline: a role for the thalamus in developing Alzheimer's disease. J Alzheimer's Dis. (2021) 83:705–20. doi: 10.3233/JAD-210173
31. Ashburner J. A fast diffeomorphic image registration algorithm. Neuroimage. (2007) 38:95–113. doi: 10.1016/j.neuroimage.2007.07.007
32. Ashburner J, Friston KJ. Why voxel-based morphometry should be used. Neuroimage. (2001) 14:1238–43. doi: 10.1006/nimg.2001.0961
33. Jónsson BA, Bjornsdottir G, Thorgeirsson TE, Ellingsen LM, Walters GB, Gudbjartsson DF, et al. Brain age prediction using deep learning uncovers associated sequence variants. Nat Commun. (2019) 10:5409. doi: 10.1038/s41467-019-13163-9
34. Mechelli A, Price CJ, Friston KJ, Ashburner J. Voxel-based morphometry of the human brain: methods and applications. Curr Med Imaging. (2005) 1:105–13. doi: 10.2174/1573405054038726
35. Schaare HL, Mueller K, Babayan A, Erbey M, Gaebler M, Kumral D, et al. Higher blood pressure is associated with lower regional grey matter density in healthy, young adults. Autonomic Neurosci. (2015) 192:97. doi: 10.1016/j.autneu.2015.07.138
36. Kesler SR. Default mode network as a potential biomarker of chemotherapy-related brain injury. Neurobiol Aging. (2014) 35:S11–9. doi: 10.1016/j.neurobiolaging.2014.03.036
37. Lee E, Yoo K, Lee Y, Chung J, Lim J, Yoon B, et al. Default mode network functional connectivity in early and late mild cognitive impairment. Alzheimer Dis Assoc Disord. (2016) 30:289–96. doi: 10.1097/WAD.0000000000000143
38. Madsen SK, Rajagopalan P, Joshi SH, Toga AW, Thompson PM, Alzheimer's Disease Neuroimaging Initiative (ADNI). Higher homocysteine associated with thinner cortical gray matter in 803 participants from the Alzheimer's Disease Neuroimaging Initiative. Neurobiol Aging. (2015) 36:S203–10. doi: 10.1016/j.neurobiolaging.2014.01.154
39. Matt,é C, Mussulini BHM, Dos Santos TM, Soares FM, Simão F, Matt,é A., et al. Hyperhomocysteinemia reduces glutamate uptake in parietal cortex of rats. Int J Dev Neurosci. (2010) 28:183–7. doi: 10.1016/j.ijdevneu.2009.11.004
40. de Jager CA. Critical levels of brain atrophy associated with homocysteine and cognitive decline. Neurobiol Aging. (2014) 35:S35–S9. doi: 10.1016/j.neurobiolaging.2014.03.040
41. Cockrell JR, Folstein MF. Mini-mental state examination. In: Abou-Saleh MT, Katona CLE, Anand KA, editors. Principles and Practice of Geriatric Psychiatry. Chichester; West Sussex: John Wiley & Sons, Ltd. (2002). p. 140-1. doi: 10.1002/0470846410.ch27(ii)
42. Tombaugh TN, McIntyre NJ. The mini-mental state examination: a comprehensive review. J Am Geriatr Soc. (1992) 40:922–35. doi: 10.1111/j.1532-5415.1992.tb01992.x
43. Nelson ME, Andel R, Nedelska Z, Martinkova J, Cechova K, Markova H, et al. The association between homocysteine and memory in older adults. J Alzheimer's Dis. (2021) 81:413–26. doi: 10.3233/JAD-201558
44. Hayes AF. Introduction to Mediation, Moderation, and Conditional Process Analysis: A Regression-Based Approach (2nd ed.). New York, NY: Guilford Press (2018).
45. Glodzik L, Mosconi L, Tsui W, de Santi S, Zinkowski R, Pirraglia E, et al. Alzheimer's disease markers, hypertension, and gray matter damage in normal elderly. Neurobiol Aging. (2012) 33:1215–27. doi: 10.1016/j.neurobiolaging.2011.02.012
46. Willette AA, Gallagher C, Bendlin BB, McLaren DG, Kastman EK, Canu E, et al. Homocysteine, neural atrophy, and the effect of caloric restriction in rhesus monkeys. Neurobiol Aging. (2012) 33:670–80. doi: 10.1016/j.neurobiolaging.2010.06.003
47. Nishitani N, Schurmann M, Amunts K, Hari R. Broca's region: from action to language. Physiology. (2005) 20:60–9. doi: 10.1152/physiol.00043.2004
48. Belcher BR, Zink J, Azad A, Campbell CE, Chakravartti SP, Herting MM. The roles of physical activity, exercise, and fitness in promoting resilience during adolescence: effects on mental well-being and brain development. Biol Psychiatry. (2020) 6:225–37. doi: 10.1016/j.bpsc.2020.08.005
49. Wei G, Xu T, Fan F, Dong H, Jiang L, Li H, et al. Can Taichi reshape the brain? A brain morphometry study. PLoS ONE. (2013) 8:e61038. doi: 10.1371/journal.pone.0061038
50. Mandal PK, Joshi J, Saharan S. Visuospatial perception: an emerging biomarker for Alzheimer's disease. J Alzheimer's Dis. (2012) 31:S117–35. doi: 10.3233/JAD-2012-120901
51. Chen L, Song J, Cheng R, Wang K, Liu X, He M, et al. Cortical thinning in the medial temporal lobe and precuneus is related to cognitive deficits in patients with subcortical ischemic vascular disease. Front Aging Neurosci. (2020) 12:536. doi: 10.3389/fnagi.2020.614833
52. Wang R, Liu N, Tao Y, Gong X, Zheng J, Yang C, et al. The application of rs-fMRI in vascular cognitive impairment. Front Neurol. (2020) 11:951. doi: 10.3389/fneur.2020.00951
53. Yi L, Wang J, Jia L, Zhao Z, Lu J, Li K, et al. Structural and functional changes in subcortical vascular mild cognitive impairment: a combined voxel-based morphometry and resting-state fMRI study. PLoS One. (2012) 7:e44758. doi: 10.1371/journal.pone.0044758
54. Censori B, Partziguian T, Manara O, Poloni M. Plasma homocysteine and severe white matter disease. Neurol Sci. (2007) 28:259–63. doi: 10.1007/s10072-007-0832-y
55. Vermeer SE, Van Dijk EJ, Koudstaal PJ, Oudkerk M, Hofman A, Clarke R, et al. Homocysteine, silent brain infarcts, and white matter lesions: The Rotterdam Scan Study. Ann Neurol. (2002) 51:285–9. doi: 10.1002/ana.10111
56. Wright CB, Paik MC, Brown TR, Stabler SP, Allen RH, Sacco RL, et al. Total homocysteine is associated with white matter hyperintensity volume: the Northern Manhattan Study. Stroke. (2005) 36:1207–11. doi: 10.1161/01.STR.0000165923.02318.22
57. Arvanitakis Z, Fleischman DA, Arfanakis K, Leurgans SE, Barnes LL, Bennett DA. Association of white matter hyperintensities and gray matter volume with cognition in older individuals without cognitive impairment. Brain Struct Funct. (2016) 221:2135–46. doi: 10.1007/s00429-015-1034-7
58. Wen W, Sachdev PS, Chen X, Anstey K. Gray matter reduction is correlated with white matter hyperintensity volume: a voxel-based morphometric study in a large epidemiological sample. Neuroimage. (2006) 29:1031–9. doi: 10.1016/j.neuroimage.2005.08.057
59. de Jager CA, Hogervorst E, Combrinck M, Budge MM. Sensitivity and specificity of neuropsychological tests for mild cognitive impairment, vascular cognitive impairment and Alzheimer's disease. Psychol Med. (2003) 33:1039–50. doi: 10.1017/S0033291703008031
60. Dichgans M, Leys D. Vascular cognitive impairment. Circ Res. (2017) 120:573–91. doi: 10.1161/CIRCRESAHA.116.308426
61. Ghafar MZAA, Miptah HN, O'Caoimh R. Cognitive screening instruments to identify vascular cognitive impairment: a systematic review. Int J Geriatr Psychiatry. (2019) 34:1114–27. doi: 10.1002/gps.5136
62. Nyenhuis DL, Gorelick PB, Geenen EJ, Smith CA, Gencheva E, Freels S, et al. The pattern of neuropsychological deficits in vascular cognitive impairment-no dementia (vascular CIND). Clin Neuropsychol. (2004) 18:41–9. doi: 10.1080/13854040490507145
63. Van Der Flier WM, Skoog I, Schneider JA, Pantoni L, Mok V, Chen CL, et al. Vascular cognitive impairment. Nat Rev Disease Primers. (2018) 4:1–16. doi: 10.1038/nrdp.2018.3
64. Huo Y, Li J, Qin X, Huang Y, Wang X, Gottesman RF, et al. Efficacy of folic acid therapy in primary prevention of stroke among adults with hypertension in China: the CSPPT randomized clinical trial. JAMA. (2015) 313:1325–35. doi: 10.1001/jama.2015.2274
65. Lattanzi S, Carbonari L, Pagliariccio G, Bartolini M, Cagnetti C, Viticchi G, et al. Neurocognitive functioning and cerebrovascular reactivity after carotid endarterectomy. Neurology. (2018) 90:e307–15. doi: 10.1212/WNL.0000000000004862
66. Blanco-Rojas L, Arboix A, Canovas D, Grau-Olivares M, Morera JCO, Parra O. Cognitive profile in patients with a first-ever lacunar infarct with and without silent lacunes: a comparative study. BMC Neurol. (2013) 13:1–7. doi: 10.1186/1471-2377-13-203
67. Grau-Olivares M, Arboix A, Junqué C, Arenaza-Urquijo EM, Rovira M, Bartrés-Faz D. Progressive gray matter atrophy in lacunar patients with vascular mild cognitive impairment. Cerebrovasc Dis. (2010) 30:157–66. doi: 10.1159/000316059
68. Nam K, Kwon H, Jeong H, Park J, Kwon H, Jeong S. Serum homocysteine level is related to cerebral small vessel disease in a healthy population. Neurology. (2019) 92:e317–25. doi: 10.1212/WNL.0000000000006816
69. Gao L, Ruan Z, Xiao Y, Xu H. Surface-based cortical morphometry, white matter hyperintensity, and multidomain cognitive performance in asymptomatic carotid stenosis. Neuroscience. (2021) 467:16–27. doi: 10.1016/j.neuroscience.2021.05.013
70. Gao L, Wang T, Qian T, Xiao F, Bai L, Zhang J, et al. Severe asymptomatic carotid stenosis is associated with robust reductions in homotopic functional connectivity. Neuroimage Clin. (2019) 24:102101. doi: 10.1016/j.nicl.2019.102101
71. Gao L, Xiao Y, Xu H. Gray matter asymmetry in asymptomatic carotid stenosis. Hum Brain Mapp. (2021) 1–12. doi: 10.1002/hbm.25645
72. Strauss E, Sherman EMS, Spreen O. A Compendium of Neuropsychological Tests: Administration, Norms and Commentary. New York, NY: Oxford University Press (2006).
Keywords: hypertension, homocysteine, VBM, default mode network, cognitive impairment
Citation: Kong Y, Li X, Chang L, Liu Y, Jia L, Gao L and Ren L (2021) Hypertension With High Homocysteine Is Associated With Default Network Gray Matter Loss. Front. Neurol. 12:740819. doi: 10.3389/fneur.2021.740819
Received: 13 July 2021; Accepted: 24 August 2021;
Published: 28 September 2021.
Edited by:
Yuzhen Xu, Tongji University, ChinaReviewed by:
Anastasia Bougea, National and Kapodistrian University of Athens, GreeceNafisa M. Jadavji, Midwestern University, United States
Copyright © 2021 Kong, Li, Chang, Liu, Jia, Gao and Ren. This is an open-access article distributed under the terms of the Creative Commons Attribution License (CC BY). The use, distribution or reproduction in other forums is permitted, provided the original author(s) and the copyright owner(s) are credited and that the original publication in this journal is cited, in accordance with accepted academic practice. No use, distribution or reproduction is permitted which does not comply with these terms.
*Correspondence: Lijuan Ren, 1027452890@qq.com