Predictive microbial feature analysis in patients with depression after acute ischemic stroke
- 1Department of Geriatrics, The Second Affiliated Hospital of Wenzhou Medical University, Wenzhou, Zhejiang, China
- 2Department of Preventive Medicine, School of Public Health and Management, Wenzhou Medical University, Wenzhou, Zhejiang, China
- 3Department of Neurology, The First Affiliated Hospital of Wenzhou Medical University, Wenzhou, Zhejiang, China
Introduction: Post-stroke depression (PSD) is the most common emotional problem following a stroke, which requires early diagnosis to improve the prognosis. Gut microbiota plays important role in the pathological mechanisms of acute ischemic stroke and influences the outcome of patients. However, the relationship between PSD and gut microbiota remains unknown. Here, we explored whether the microbial signatures of gut microbiota in the patients with stroke could be an appropriate predictor of PSD.
Methods: Fecal samples were collected from 232 acute ischemic stroke patients and determined by 16s rRNA sequencing. All patients then received 17-Hamilton Depression Rating Scale (HAMD-17) assessment 3 months after discharge, and were further divided into PSD group and non-PSD group. We analyzed the differences of gut microbiota between these groups. To identify gut microbial biomarkers, we then established microbial biomarker model.
Results: Our results showed that the composition of gut microbiota in the PSD patients differed significantly from that in non-PSD patients. The genus Streptococcus, Akkermansia, and Barnesiella were significantly increased in PSD patients compared to non-PSD, while the genus Escherichia-Shigella, Butyricicoccus, and Holdemanella were significantly decreased. Correlation analyses displayed that Akkermansia, Barnesiella, and Pyramidobacter were positively correlated with HAMD score, while Holdemanella was negatively correlated with HAMD score. The optimal microbial markers were determined, and the combination achieved an area under the curve (AUC) value of 0.705 to distinguish PSD from non-PSD.
Conclusions: Our findings suggest that PSD patients had distinct gut microbiota compared to non-PSD patients, and explore the potential of microbial markers, which might provide clinical decision-making in PSD.
1. Introduction
Post-stroke depression (PSD) is the most common emotional disorder after stroke (GBD 2015 Neurological Disorders Collaborator Group, 2017), which can increase the risk of mortality and stroke recurrence (Hackett et al., 2014; Cai et al., 2019). About 31% of acute ischemic stroke (AIS) patients may suffer from PSD within 5 years after stroke (Hackett and Pickles, 2014). A meta-analysis showed that delayed diagnosis and treatment of PSD may lead to stroke recurrence and suicide (Cai et al., 2019). Early recognition of patients who were prone to develop PSD was crucial, especially at clinical admission. Close monitoring and early intervention may prevent the adverse outcome (Maaijwee et al., 2016; Baccaro et al., 2019; Fantu et al., 2022; Ladwig et al., 2022). A series of studies evaluated the prediction potential of a variety of scoring systems for PSD, but none were robust enough. Thus, more efficient methods for prediction of PSD were needed.
Risk factors of PSD were multifactorial and complex, including the severity and location of the stroke, social support and personal factors such as gender, age and medical history (Elias et al., 2022; Li et al., 2022; Zhang et al., 2023). Recently, numerous studies revealed the potential association between changes in gut microbiota and prognosis after acute ischemic stroke (Tian et al., 2022; Wang et al., 2022). Previous studies showed that oral administration of antibiotics could reduce neurological impairment and the cerebral infarct volume, relieve cerebral edemas by altering the gut microbiota, while probiotic administration could ameliorate patients’ mood though the regulation of gut microbiota (Chen et al., 2019; Liu et al., 2020). Meanwhile, changes of gut microbiota could also be considered as a prognostic factor in acute ischemic stroke (Benakis and Liesz, 2022; Tian et al., 2022). In recent years, microbial marker severs as a non-invasive diagnosis tool for some diseases (Arnoldussen et al., 2017). Gut microbial marker had the potential to predict the development of AIS, and they may be an effective target tool to influence clinical outcome of AIS. Our previous study showed that the microbiota composition of patients with post stroke cognitive impairment (PSCI) was different from that of non-PSCI patients, characterized by an increase in the abundance of Proteobacteria (Ling et al., 2020a). Moreover, we also revealed that patients with post-stroke comorbid cognitive impairment and depression (PSCCID) had lower abundance of short-chain fatty acids (SCFAs) producing bacteria compared with non-PSCCID patients, and revealed that the abundance of Gammaproteobacteria and Enterobacteriaceae was negatively correlated with MoCA score (Ling et al., 2020b). These studies inspired the potential role of the microbiota in PSD progression. There was a correlation between gut microbiota and some complications of acute ischemic stroke, but the link between gut microbiota and PSD has not been well described.
To determine the prediction potential of gut microbiota for PSD outcome on admission, we performed a prospective study. In this study, we compared the difference between the gut microbiota of PSD patients and non-PSD patients, and further explored the “key microbial signatures” of gut microbiota of PSD patients, and searched for possible marker for the diagnosis of PSD patients. Further, through in-depth study on the relationship between the characteristics of gut microbiota and their clinical efficacy, we try to find potential biomarkers for clinical diagnosis, providing a new direction for the screening PSD patients on admission.
2. Materials and methods
2.1. Patients and sample collection
We enrolled 588 AIS patients at Second Affiliated Hospital of Wenzhou Medical University from September 2020 to July 2021. These patients came from Zhejiang Province with a long-term settlement. Patients with AIS diagnosed according to American Heart Association/American Stroke Association were included (Sacco et al., 2013). The inclusion criteria for this study were: ischemic stroke patients within 3 days after onset, aged 40–95 years, without special diets, such as vegetarianism, no communication deficit (e.g., aphasia and deafness). Patients following conditions were excluded: transient ischemic attack or lacunar infarction; depression, anxiety or other mental illnesses diagnosed or psycho-emotional drug used before the onset of stroke; accompanied with severe systemic diseases (renal failure, respiratory failure, and circulatory failure); medical histories of other central nervous system diseases; failure to complete psychological assessment; use antidepressants, antibiotics or probiotics within 3 months. Eighty-one AIS patients were excluded according to the exclusion criteria, 116 AIS patients failed to collect stool samples, 159 AIS patients lost follow-up, 232 AIS patients that could be analyzed (Supplementary Figure 1). The protocol was approved by the Medical Ethics Committee of the Second Affiliated Hospital of Wenzhou Medical University. All clinical features were recorded according to standard procedures. The peripheral venous blood of the participants was measured at admission. We collected stool samples from each AIS patients at admission.
2.2. Demographic and clinical data
Demographic and clinical baseline data were collected, such as age, gender, marital status, education level, smoking and alcohol drinking, past disease history, education level, NIHSS scale, MMSE scale, baseline Barthel Index (BI), baseline blood pressure, baseline blood glucose and lipid levels, other lab results, imaging data, severity stratification, complications, and outcomes. The National Institute of Health Stroke Scale (NIHSS) was used to evaluate the degree of neurological impairment. The cognitive level was assessed by Minimum Mental State Examination (MMSE). PSD was diagnosed with a HAMD score ≥8 according to 17-item Hamilton Depression Scale (HAMD-17) for 3 months after AIS onset (Zimmerman et al., 2013).
2.3. Fecal sample collection and analysis
All patients provided fresh stool within 1 week of admission. Fecal samples were collected and immediately transferred to the laboratory. The 200 mg feces samples were placed into a 2 ml sterile centrifuge tube and labeled. All specimens were stored at −80°C within 30 min of preparation. DNA of fecal samples was extracted by DNA Kit (Omega Bio-tek, Norcross, GA, USA.) according to the manufacturer. The DNA concentration and purity were determined using NanoDrop 2000 UV-vis spectrophotometer (Thermo Scientific, Wilmington, DE, USA), and quantified by 1% agarose gel. After DNA extraction, the V3-V4 16S ribosomal RNA gene region was amplified with forward primer (5′-ACTCCTACGGGAGGCAGCAG-3′) and the reverse primer (5′-GGACTACHVGGGTWTCTAAT-3′). Sequencing was performed on the Illumina MiSeq platform (Illumina, San Diego, CA, USA). Raw fastq files were quality-filtered by Trimmomatic and merged by FLASH.
Chao1 and Shannon indexes were applied to analyze the alpha diversity of gut microbiota. A Wilcoxon rank sum test was performed to compare the alpha diversity of groups. Use the rarefaction curves to assess differences in richness between the two groups. The linear discriminant analysis (LDA) effect size (LEfSe) was performed using the Kruskal–Wallis test with LDA score of >2 used as thresholds. Differences in microbiota composition between groups were analyzed using Wilcoxon rank sum test. The Spearman correlation coefficients were calculated and visualized by heatmap. To determine the correlation between the microbial communities in the PSD and Non-PSD group, we constructed correlation network diagram inferred from the abundance of ASV with the threshold of 0.6. Spearman correlation was applied to determine the positive or negative relationship between the ASVs. Nodes were colored by phylum and size was related to the abundance of taxa. ROC curves and the AUC were used to confirm the specificity and sensitivity of the characteristic gut microbiota to the diagnosis of PSD.
2.4. Statistical analysis
Data were analyzed by SPSS 22.0 software. Categorical variables were presented as numbers with percentages, and continuous variables were shown as means with standard deviation (SD), or medians with interquartile range (IQR) according to the Kolmogorov-Smirnov normality test. Mann–Whitney U-test was used for continuous variables and the Chi-squared test was used for categorical variables. All variables showing a trend in association in univariate analysis (P < 0.10) were included in the multivariable model. The relative risk was expressed as the odds ratio (OR) with a 95% confidence interval (CI). P values < 0.05 was considered significant.
3. Results
3.1. Clinical characteristics of enrolled patients
There were 88 patients diagnosed with PSD (37.93%) after 3-month follow-up. The baseline characteristics of the patients in the two groups are shown in Table 1. In the PSD group, the mean age was 65.43 years old, 46.6% were female, and median education level 2 (2–2). The mean age was 64.57 years old in the non-PSD group, with 29.9% being female and with 2 (2–3) being education level. Univariate analysis showed that the differences between the two groups were gender, education level, diabetes mellitus, alcohol drinking, Barthel Index, FT3, baseline NHISS scores, baseline MMSE scores, and baseline PSQI scores (all P < 0.05). Multivariable logistic regression showed that lower MMSE score was an independent risk factors for PSD (P = 0.040, OR 0.840, 95% CI 0.711–0.992).
3.2. Diversity and distribution of gut microbiota in PSD and non-PSD groups
The alpha diversity of the bacterial community was assessed by Shannon and Chao1 indexes. There was no significant difference between PSD and non-PSD groups (P = 0.9029, 0.3832), indicating similarity in community richness and diversity between PSD and non-PSD patients (Figures 1A, B). The rarefaction curves (Figure 1C) showed that the richness of PSD group tended to be higher than that of the non-PSD group. The Venn diagram (Figure 1D) showed the common and unique ASVs detected in PSD and non-PSD groups. The number of shared ASVs was 1,839. The number of unique ASVs in PSD group was 1,300, while that in PSND group was 2,445. As shown in Supplementary Figure 2, the gut microbiota beta-diversity between PSD and non-PSD groups was different.
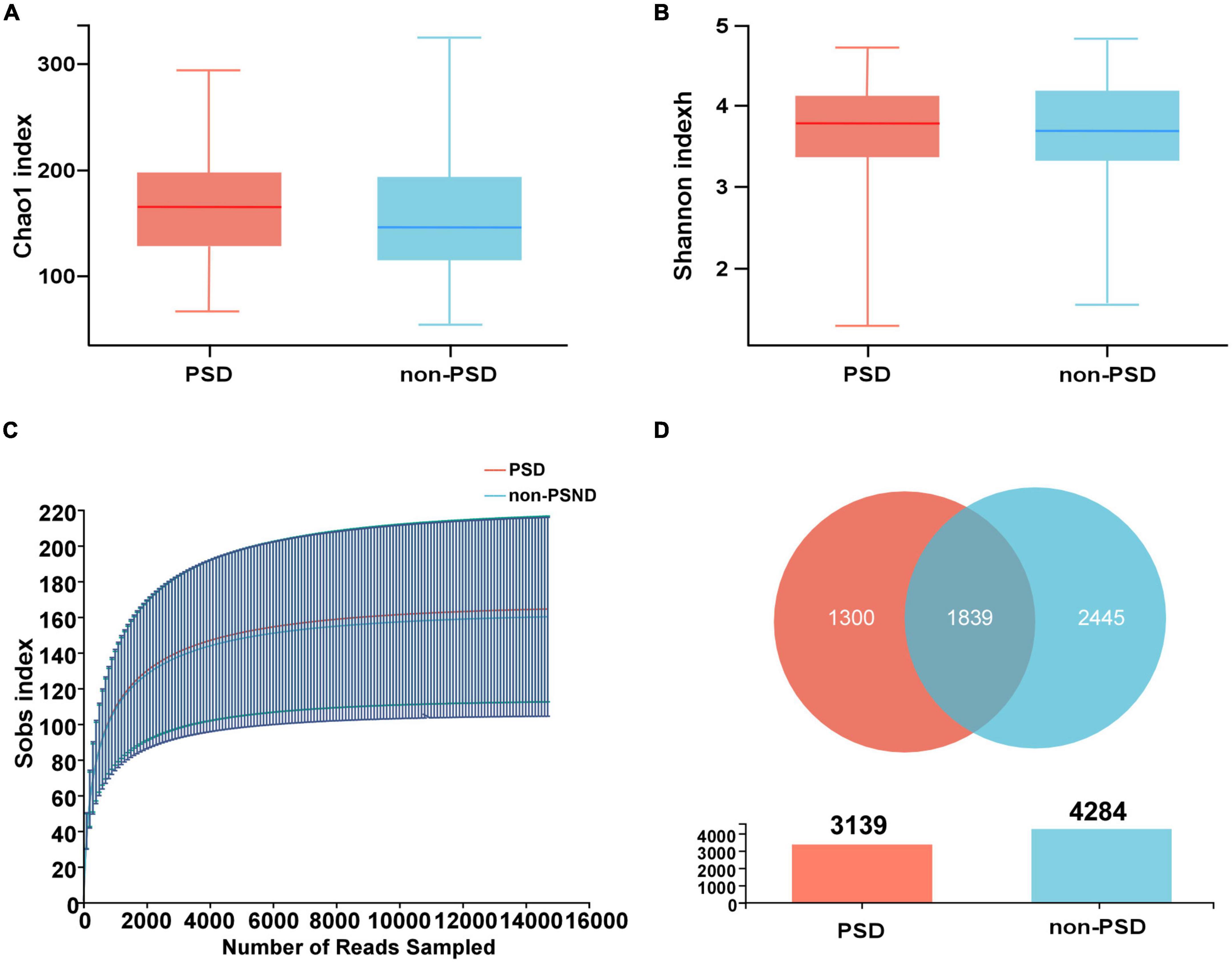
Figure 1. Distribution of gut microbiota in PSD and non-PSD groups. (A) Chao1 indexes of gut microbiota α diversity in PSD and non-PSD groups. (B) Shannon indexes of gut microbiota α diversity in PSD and non-PSD groups. (C) A refraction curve demonstrating richness. (D) Venn diagram of gene numbers in PSD and non-PSD groups.
Taxonomic profiles at different levels of two groups were presented in Figure 2. phylum Firmicutes, Bacteroidota, Proteobacteria, and Actinobacteria were the predominant in two groups (Figure 2A). Taxonomic classification at the family level showed that most of the intestinal bacteria detected fell into Lachnospiraceae, Ruminococcaceae, Bacteroidaceae, Enterobacteriaceae, Streptococcaceae, Lactobacillaceae, Bifidobacteriaceae, Veillonellaceae, Peptostreptococcaceae, Prevotellaceae, and Oscillospiraceae, which occupied >80% of the total microbiota in PSD group (Figure 2B). Compared with the non-PSD group, PSD group showed lower relative abundances of Enterobacteriaceae and higher relative abundance of Bacteroidacea and Streptococcaceae. At the genera level (Figure 2C), the top five enriched genera were Bacteroides, Blautia, Escherichia-Shigella, Streptococcus, and Lactobacillus. PSD group showed lower relative abundances of Escherichia-Shigella and higher relative abundance of Bacteroides and Streptococcus compared with the non-PSD group. Co-occurrence clustering analysis of the top 200 genera of relative abundance were presented in Figure 3. The absolute value of coefficient was set as more than 0.6. The network of PSD contained a total of 45 nodes and 50 edges with the average degree of 2.22 and average clustering of 0.16. The network of non-PSD contained a total of 27 nodes and 42 edges with the average degree of 3.03 and average clustering of 0.21. The results showed that the microbial networks in PSD were sparser than in non-PSD. The clustering coefficient of gut microbiota network in PSD group was lower than that in non-PSD group, indicating that the compactness of the network was reduced (from 6 to 5).
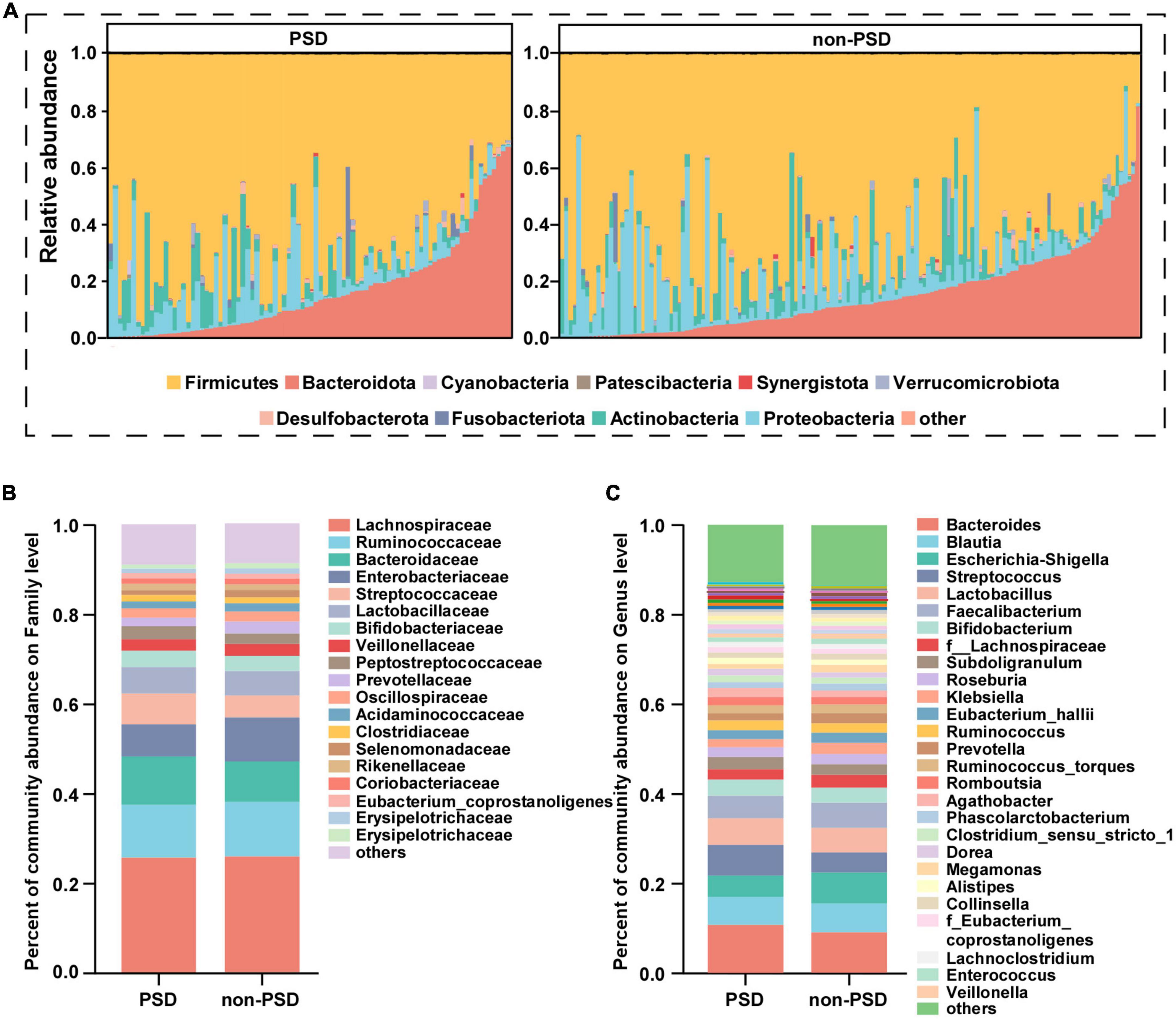
Figure 2. Composition of gut microbiota between PSD and non-PSD groups. (A) Predominant bacteria at the phylum level, each bar represents one sample. Relative abundances of bacteria between groups at the family level (B) and genus (C) level.
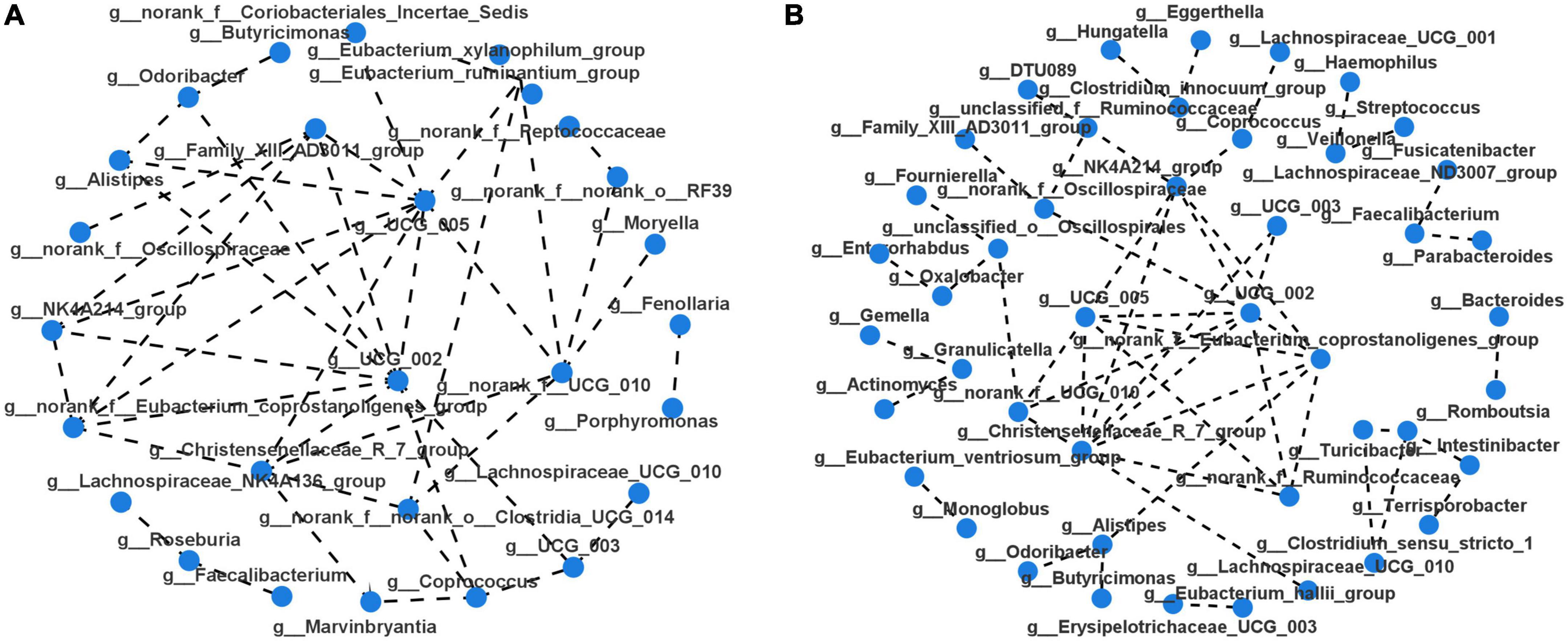
Figure 3. Microbiota correlation network of PSD and non-PSD groups. Each circular node represents a genus. The connecting lines between the two samples represent their Spearman index correlation. (A) Correlation network of non-PSD. (B) Correlation network of PSD.
3.3. Differential signatures of gut microbiota in PSD and non-PSD groups
A LEfSe analysis was used to screen the differential biomarkers of the gut microbiota to explore the specific bacteria associated with PSD. The taxonomic cladogram showed the dominant species of gut microbiota in the two groups from phylum level to genus level (Figure 4A). We marked 31 distinguishing taxa with differential abundances between two groups by LDA scores above 2.0 (Figure 4B). Compared with the non-PSD group, the abundance of phylum Verrucomicrobiota (P = 0.048) and Cyanobacteria (P = 0.011) in PSD group is higher, and the abundance of phylum Synergistota (P = 0.014) is lower (Figure 5A, all P < 0.05). Compared with the non-PSD group, the abundance of family Akkermansiaceae (P = 0.035), Barnesiellaceae (P = 0.0016), Norank_o_Chloroplast (P = 0.035) and Staphylococaceae (P = 0.0015) in PSD group is higher, and the abundance of family Butyricicoccaceae (P = 0.0099) and Synergistaceae (P = 0.014) is lower (Figure 5B). At the genus level, PSD exhibited a lower relative abundance of Escherichia-Shigella (P = 0.043), unclassified_f_Lachnospiraceae (P = 0.039), Butyricicoccus (P = 0.012), and Holdemanella (P = 0.025), and a higher relative abundance of Streptococcus (P = 0.042), Akkermansia (P = 0.035), and Barnesiella (P = 0.0043) (Figure 5C).
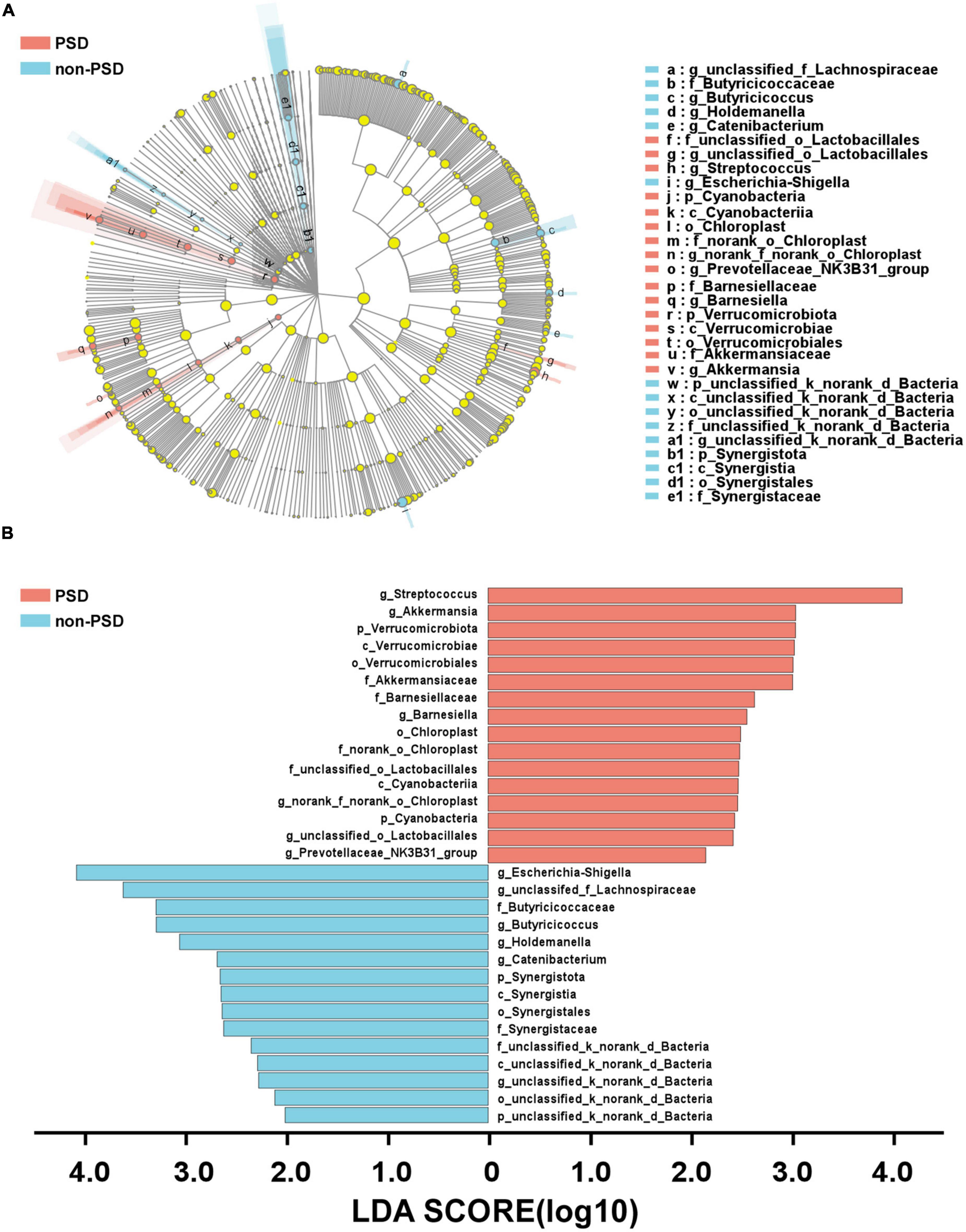
Figure 4. Differential bacteria of gut microbiota between PSD and non-PSD groups. (A) Annotated branch diagram of the different bacteria between PSD and non-PSD patients. (B) LEfSe analysis showing the most differentially abundant taxa between PSD and non-PSD groups. Red and green bars represented species with relatively higher abundance in PSD and non-PSD patients, respectively. bacterial taxa are shown with a LDA score >2.0.
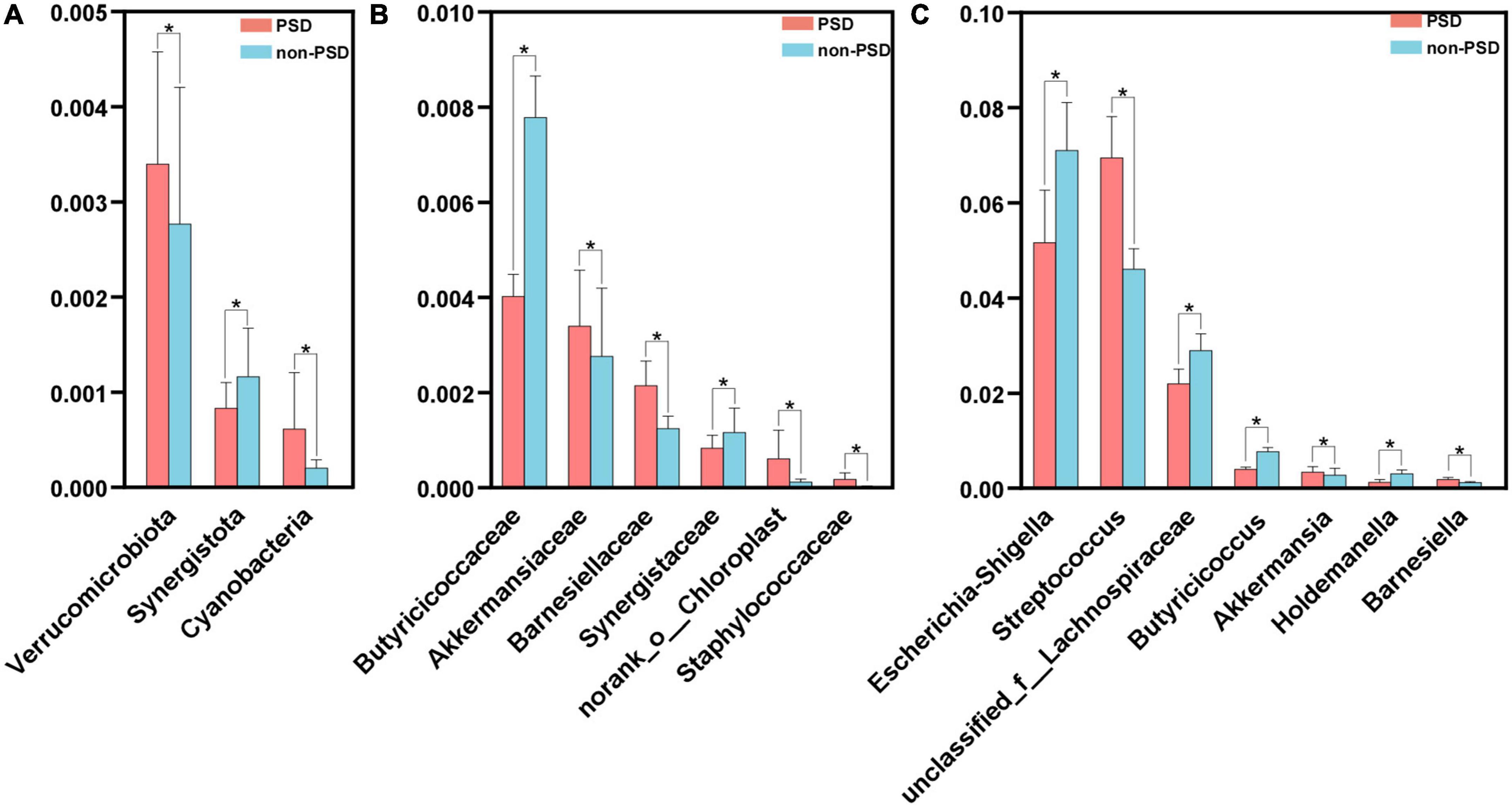
Figure 5. Comparison of the significant bacteria in PSD and non-PSD groups. The relative abundances of the significant bacteria at the phylum level (A), the family (B) and the genus level (C) in PSD patients compared with non-PSD patients. *P < 0.05.
3.4. Correlation analysis of gut microbiota composition and clinical indicators
As shown in Figure 6, the correlations between differential species and diverse clinical indexes were estimated by the Spearman correlation analysis. The clinical indexes were mainly involving demographic characteristics (educational level, diabetes mellitus, alcohol drinking and Barthel index), laboratory examinations (FT3) and psychiatric outcomes evaluation (NIHSS and MMSE). Akkermansia (P < 0.05), Barnesiella (P < 0.05), and Pyramidobacter (P < 0.01) were positively related with HAMD, while Holdemanella (P < 0.01) and norank_f_norank_o_Chloroplast (P < 0.01) negatively connected with HAMD. MMSE score was positively associated with Butyricicoccus (P < 0.05), while negatively associated with Pyramidobacter (P < 0.001).
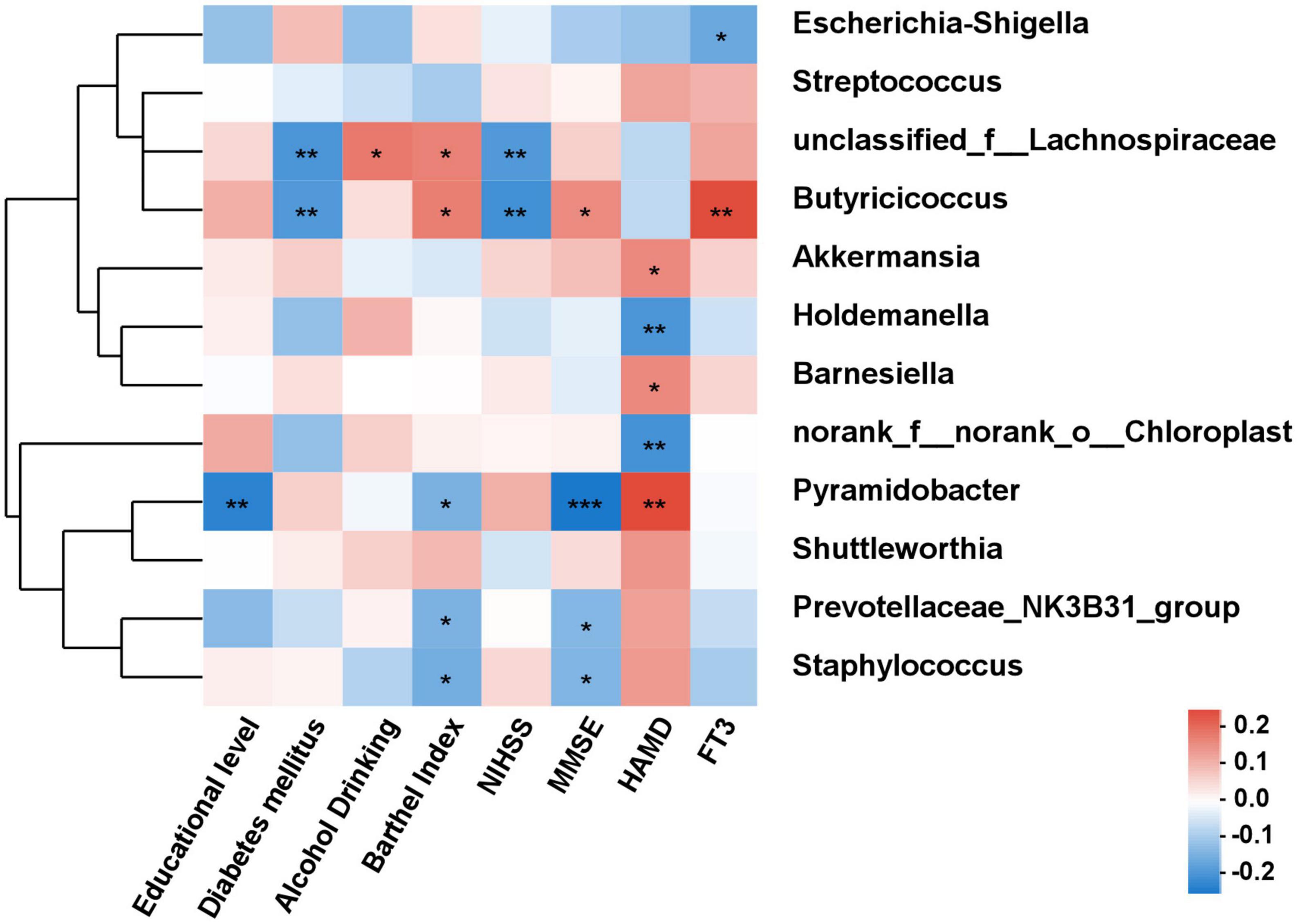
Figure 6. The correlation analysis between the differential bacteria and clinical indexes. Spearman correlations between gut microbiota and clinical indicators. Red and blue indicate positive and negative correlations, respectively. *P < 0.05, **P < 0.01, ***P < 0.001.
3.5. Diagnostic potential of PSD based on gut microbial markers
To further explore the value of microbial signatures in the prediction of PSD, this study picked out genera Akkermansia and Holdemanella which were closely related to HAMD with relatively high abundance, and the top seven bacteria. Then, receiver operating characteristic (ROC) curve analyses were carried out according to the relative abundance of the top seven bacteria. As shown in Figure 7, Akkermansia, Holdemanella and the combination of seven bacteria in the distinguishing between PSD and non-PSD had AUC of 0.563, 0.565, and 0.705, respectively (95% CI: 0.486–0.640;95% CI: 0.491–0.640;95% CI: 0.634–0.771), suggesting that the characteristic bacteria might be a well candidate for the diagnosis of PSD.
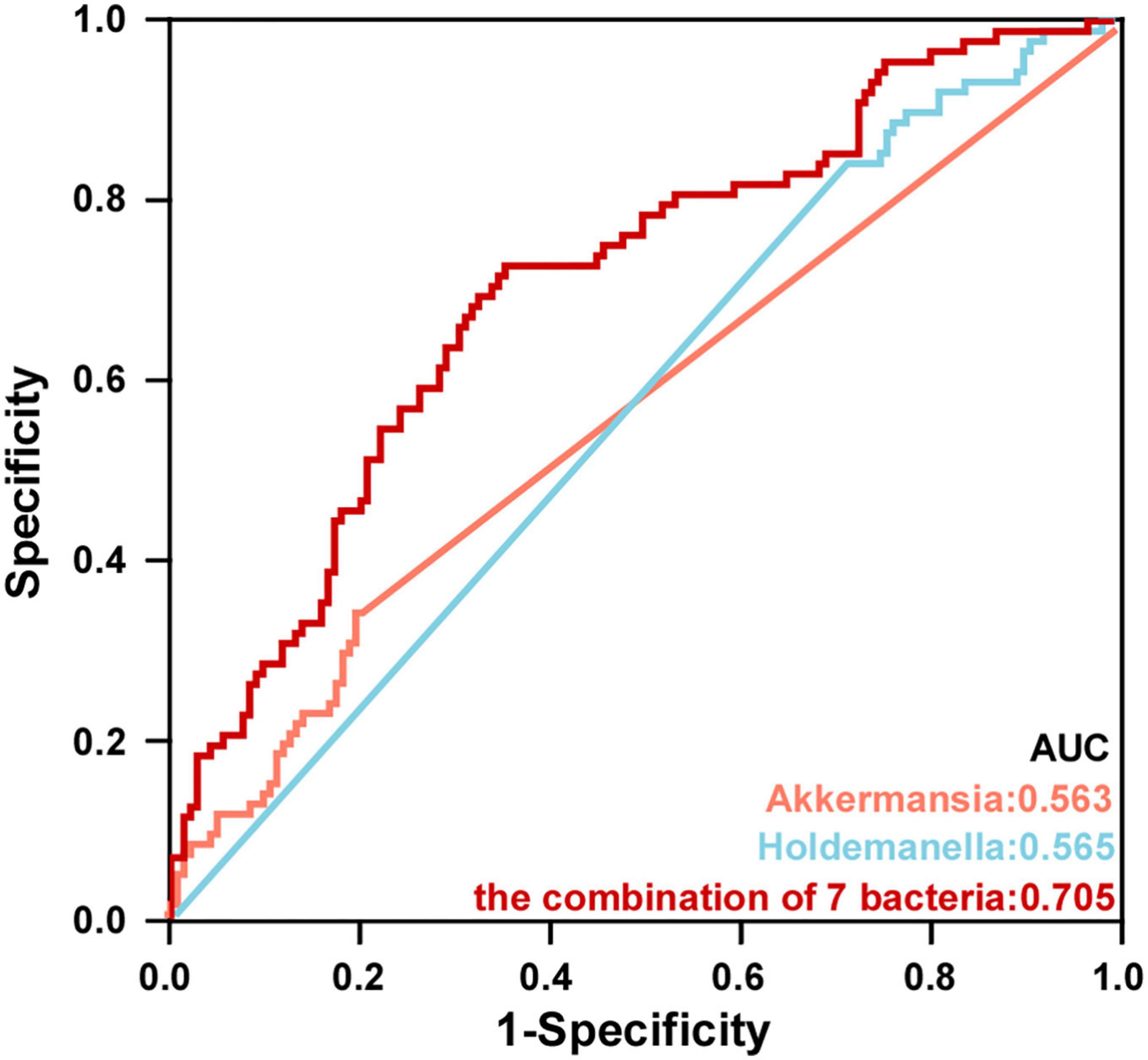
Figure 7. Potential microbiota biomarkers distinguished PSD group from non-PSD group. ROC curve showed the ability to differentiate PSD group from non-PSD group based on specific bacteria.
4. Discussion
The pathogenesis of PSD was usually affected by many factors, including gut microbiota. Our analysis revealed a significant difference in gut microbiota composition between PSD and non-PSD groups. Then, we proposed that bacterial biomarkers, such as diversity, abundance feature and specific bacteria, might be useful to identify patients who were likely to develop PSD.
In this study, gender, education level, diabetes mellitus, alcohol drinking, Barthel Index, FT3 differ significantly in PSD and non-PSD, which may be attributed to the occurrence of PSD compared with non-PSD. Many researchers have investigated a series of scoring systems from PSD prediction (Pan et al., 2022; Yi et al., 2022). Prospective studies assessed the predictive power of HAMD score for PSD, which were commonly used in clinical (Kang et al., 2013). There were still flaws in this method. Besides, HAMD score was usually assessed 3 months after the stroke discharge, which is to the disadvantage of early clinical judgment of PSD. However, more relevant data should be included to improve the early recognition of patients.
A large amount of evidence showed that the balance of gut microbiota was disrupted in patients with various brain diseases, such as Parkinson’s disease, Alzheimer’s disease, Wilson’s disease, depression, etc. (Bravo et al., 2011; Dinan and Cryan, 2013; Ait-Belgnaoui et al., 2014; Kennedy et al., 2017). Moreover, several clinical studies have reported that patients with AIS exhibit gut dysbiosis and, in turn, changes in the gut microbiota affected neuroinflammatory and functional outcome after brain injury (Singh et al., 2016). Patients with PSCI also had significantly different gut microbiota at multiple taxonomic levels, compared to AIS patients without cognitive impairment. Further study showed that fecal microbiota transplantation from patients to stroke mice was performed to examine the causal relationship between the gut microbiota and PSCI. These results indicated close contact between gut microbiota and complications after a stroke (Benakis et al., 2016). This study analyzed the characteristics of gut microbiota in patients with PSD. Although there was no significant difference in α diversity of PSD group compared with non-PSD group, but profound alteration in gut microbial structure were discovered in PSD patients. In this study, compared with the non-PSD group, the microbial composition of PSD group was characterized by a decrease in the abundance of the genus Escherichia-Shigella, Butyricicoccus, and Holdemanella as well as an increase in the abundance of the genus Streptococcus, Akkermansia, and Barnesiella. Moreover, three bacteria Akkermansia, Barnesiella, and Pyramidobacter were positively correlated with HAMD score, while Holdemanella was negatively correlated with HAMD score, which might predict PSD patients. Akkermansia is generally considered to be beneficial to human physiology (Kong et al., 2016), however, its changes are inconsistent in many brain diseases. The unpredictable restraint stress potentiated rotenone-induced effects in the colon including increased Akkermansia (Dodiya et al., 2018). Studies have shown that the abundance of Akkermansia in brain diseases including multiple sclerosis (Cantarel et al., 2015; Berer et al., 2017), PD (Heintz-Buschart et al., 2018), and AD patients (Li et al., 2019) were increased. Our previous studies demonstrated that the abundance of Akkermansia in the sepsis-induced cognitive impairment mice (Liu et al., 2021) and AD model mice (Sun et al., 2019) were significantly increased. This contrasts with former studies, where a decrease in the genus Akkermansia in PD (Aho et al., 2019) and APP/PS1 mouse model (Harach et al., 2017) were reported. The effect of Akkermansia was inconsistent, which has both beneficial effect and negative effect (Desai et al., 2016; Kong et al., 2016). Akkermansia was involved in immune regulation, which possessed both regulatory and pro-inflammatory properties (Derrien et al., 2004). Akkermansia can induce inflammatory responses, neurotoxicity, and blood-brain barrier disruption in the microenvironment of gliomas through its ability to degrade the intestinal mucosal layer (Patrizz et al., 2020), could aggravate inflammation during infection (Ganesh et al., 2013). Streptococcus has been shown to exhibit pro-inflammatory responses. In this study, the genus Streptococcus was significantly increased in PSD patients. A case-control study that genera Streptococcus were enriched in the depression school-aged children compared with healthy controls (Ling et al., 2022). It was reported that genus Streptococcus were significantly increased in the major depressive disorder and bipolar disorder with current major depressive episode groups compared with health participants (Rong et al., 2019). These were characterized by increased relative abundances of taxa that include strains with inflammatory properties, suggesting a potential mediator of inflammation between the gut microbiota and PSD. In addition to exploring the underlying pathogens, this study also evaluated the decreased abundance bacteria in PSD patients, including Escherichia-Shigella, Butyricicoccus, and Holdemanella. Therefore, bacteria alteration with the increase of opportunistic pathogens and the decrease of beneficial bacteria may be a risk factor for PSD. Moreover, a diagnostic model for PSD was established using seven abundant bacteria, and the AUC area exhibited satisfactory predictive performance. This finding suggested that PSD patients experienced more differences in gut microbial structure related to depressive symptoms, and characteristic bacteria can be used as diagnostic biomarkers for PSD.
There are several limitations to the present study. First, gut microbiota stool samples were collected at a single time point of admission. Future research needs longitudinal design to monitor the diversity and community composition of gut microbiota at different time points, so as to better understand the dynamic changes of microbiota in PSD patients. Second, although we tried to consider the influence of environmental factors (region, diet, educational background) on the depressive state and microbial population of AIS patients, there was still a lack of further detailed and comprehensive information (such as socioeconomic status, social support, seasonal factors, etc.). More detailed dietary and lifestyle factors information of the participants need provided to assess whether and how dietary habits affect the microbial composition of- PSD patients. The participants adopted different diet and style, which may lead to bacterial variations. Lastly, the sample size was limited, thus these conclusions may require careful interpretation. Large-scale multicenter and cross-regional studies are necessary to estimate the role of gut microbiota in predicting the diagnosis of PSD.
In conclusion, this study revealed that gut microbiome of PSD patients has changed, which is a predictor of PSD. Escherichia-Shigella, Butyricicoccus, Akkermansia, and Barnesiella were microbial biomarkers for PSD, which was worthy of further study on clinical application. Our findings may help to early predict PSD and provide information for clinical decision-making of patients.
Data availability statement
The datasets presented in this study can be found in online repositories. The names of the repository/repositories and accession number(s) can be found below: https://www.ncbi.nlm.nih.gov/, PRJNA905676.
Ethics statement
This study was approved by Ethics Committee of the Second Affiliated Hospital of Wenzhou Medical University. The patients/participants provided their written informed consent to participate in this study.
Author contributions
JL, JS, and QT: conception or design of the work. SY, HX, YW, NS, QC, YZ, QG, and JZ: data collection and analysis. All authors contributed to the article and approved the submitted version.
Funding
This work was supported by Clinical Medical Research Project of Zhejiang Medical Association (2022ZYC-D10).
Conflict of interest
The authors declare that the research was conducted in the absence of any commercial or financial relationships that could be construed as a potential conflict of interest.
Publisher’s note
All claims expressed in this article are solely those of the authors and do not necessarily represent those of their affiliated organizations, or those of the publisher, the editors and the reviewers. Any product that may be evaluated in this article, or claim that may be made by its manufacturer, is not guaranteed or endorsed by the publisher.
Supplementary material
The Supplementary Material for this article can be found online at: https://www.frontiersin.org/articles/10.3389/fnagi.2023.1116065/full#supplementary-material
Supplementary Figure 1 | Flowchart of patients included in the study.
Supplementary Figure 2 | Composition of gut microbiota beta-diversity between PSD and non-PSD groups. Beta-diversity illustrating the grouping patterns of PSD and non-PSD groups by principal coordinate analysis.
References
Aho, V., Pereira, P., Voutilainen, S., Paulin, L., Pekkonen, E., Auvinen, P., et al. (2019). Gut microbiota in Parkinson’s disease: Temporal stability and relations to disease progression. EBioMedicine 44, 691–707. doi: 10.1016/j.ebiom.2019.05.064
Ait-Belgnaoui, A., Colom, A., Braniste, V., Ramalho, L., Marrot, A., Cartier, C., et al. (2014). Probiotic gut effect prevents the chronic psychological stress-induced brain activity abnormality in mice. Neurogastroenterol. Motil. 26, 510–520. doi: 10.1111/nmo.12295
Arnoldussen, I., Wiesmann, M., Pelgrim, C., Wielemaker, E., van Duyvenvoorde, W., Amaral-Santos, P., et al. (2017). Butyrate restores HFD-induced adaptations in brain function and metabolism in mid-adult obese mice. Int. J. Obes. 41, 935–944.
Baccaro, A., Wang, Y., Candido, M., Conforto, A., Brunoni, A., Leite, C., et al. (2019). Post-stroke depression and cognitive impairment: Study design and preliminary findings in a Brazilian prospective stroke cohort (EMMA study). J. Affect. Disord. 245, 72–81. doi: 10.1016/j.jad.2018.10.003
Benakis, C., Brea, D., Caballero, S., Faraco, G., Moore, J., Murphy, M., et al. (2016). Commensal microbiota affects ischemic stroke outcome by regulating intestinal γδ T cells. Nat. Med. 22, 516–523. doi: 10.1038/nm.4068
Benakis, C., and Liesz, A. (2022). The gut-brain axis in ischemic stroke: Its relevance in pathology and as a therapeutic target. Neurol. Res. Pract. 4:57. doi: 10.1186/s42466-022-00222-8
Berer, K., Gerdes, L., Cekanaviciute, E., Jia, X., Xiao, L., Xia, Z., et al. (2017). Gut microbiota from multiple sclerosis patients enables spontaneous autoimmune encephalomyelitis in mice. Proc. Natl. Acad. Sci. U.S.A. 114, 10719–10724. doi: 10.1073/pnas.1711233114
Bravo, J., Forsythe, P., Chew, M., Escaravage, E., Savignac, H., Dinan, T., et al. (2011). Ingestion of Lactobacillus strain regulates emotional behavior and central GABA receptor expression in a mouse via the vagus nerve. Proc. Natl. Acad. Sci. U.S.A. 108, 16050–16055. doi: 10.1073/pnas.1102999108
Cai, W., Mueller, C., Li, Y., Shen, W., and Stewart, R. (2019). Post stroke depression and risk of stroke recurrence and mortality: A systematic review and meta-analysis. Ageing Res. Rev. 50, 102–109. doi: 10.1016/j.arr.2019.01.013
Cantarel, B., Waubant, E., Chehoud, C., Kuczynski, J., DeSantis, T., Warrington, J., et al. (2015). Gut microbiota in multiple sclerosis: Possible influence of immunomodulators. J. Investig. Med. 63, 729–734. doi: 10.1097/JIM.0000000000000192
Chen, R., Xu, Y., Wu, P., Zhou, H., Lasanajak, Y., Fang, Y., et al. (2019). Transplantation of fecal microbiota rich in short chain fatty acids and butyric acid treat cerebral ischemic stroke by regulating gut microbiota. Pharmacol. Res. 148:104403. doi: 10.1016/j.phrs.2019.104403
Derrien, M., Vaughan, E., Plugge, C., and de Vos, W. (2004). Akkermansia muciniphila gen. nov., sp. nov., a human intestinal mucin-degrading bacterium. Int. J. Syst. Evol. Microbiol. 54(Pt 5), 1469–1476. doi: 10.1099/ijs.0.02873-0
Desai, M., Seekatz, A., Koropatkin, N., Kamada, N., Hickey, C., Wolter, M., et al. (2016). A dietary fiber-deprived gut microbiota degrades the colonic mucus barrier and enhances pathogen susceptibility. Cell 167, 1339–53.e21.
Dinan, T., and Cryan, J. (2013). Melancholic microbes: A link between gut microbiota and depression? Neurogastroenterol. Motil. 25, 713–719. doi: 10.1111/nmo.12198
Dodiya, H., Forsyth, C., Voigt, R., Engen, P., Patel, J., Shaikh, M., et al. (2018). Chronic stress-induced gut dysfunction exacerbates Parkinson’s disease phenotype and pathology in a rotenone-induced mouse model of Parkinson’s disease. Neurobiol. Dis. 135:104352. doi: 10.1016/j.nbd.2018.12.012
Elias, S., Benevides, M., Martins, A., Martins, G., Marcos, A., and Nunes, J. (2022). Factors associated with post-stroke depression in the acute phase of ischemic stroke: A cross-sectional study. Clin. Neurol. Neurosurg. 223:107505. doi: 10.1016/j.clineuro.2022.107505
Fantu, E., Hailu, W., Bekele, N., Tsegaye, T., Tadesse, M., and Asres, M. (2022). Determinants of post-stroke depression among stroke survivors at University of Gondar Hospital, Northwest Ethiopia: A case-control study. BMC Neurol. 22:446. doi: 10.1186/s12883-022-02982-x
Ganesh, B., Klopfleisch, R., Loh, G., and Blaut, M. (2013). Commensal Akkermansia muciniphila exacerbates gut inflammation in Salmonella Typhimurium-infected gnotobiotic mice. PLoS One 8:e74963. doi: 10.1371/journal.pone.0074963
GBD 2015 Neurological Disorders Collaborator Group (2017). Global, regional, and national burden of neurological disorders during 1990-2015: A systematic analysis for the Global Burden of Disease Study 2015. Lancet Neurol. 16, 877–897. doi: 10.1016/s1474-4422(17)30299-5
Hackett, M., Köhler, S., O’Brien, J., and Mead, G. (2014). Neuropsychiatric outcomes of stroke. Lancet Neurol. 13, 525–534. doi: 10.1016/s1474-4422(14)70016-x
Hackett, M., and Pickles, K. (2014). Part I: Frequency of depression after stroke: An updated systematic review and meta-analysis of observational studies. Int. J. Stroke 9, 1017–1025. doi: 10.1111/ijs.12357
Harach, T., Marungruang, N., Duthilleul, N., Cheatham, V., Mc Coy, K., Frisoni, G., et al. (2017). Erratum: Reduction of Abeta amyloid pathology in APPPS1 transgenic mice in the absence of gut microbiota. Sci. Rep. 7:46856. doi: 10.1038/srep46856
Heintz-Buschart, A., Pandey, U., Wicke, T., Sixel-Doring, F., Janzen, A., Sittig-Wiegand, E., et al. (2018). The nasal and gut microbiome in Parkinson’s disease and idiopathic rapid eye movement sleep behavior disorder. Mov. Disord. 33, 88–98. doi: 10.1002/mds.27105
Kang, H., Stewart, R., Kim, J., Jang, J., Kim, S., Bae, K., et al. (2013). Comparative validity of depression assessment scales for screening poststroke depression. J. Affect. Disord. 147, 186–191. doi: 10.1016/j.jad.2012.10.035
Kennedy, P., Cryan, J., Dinan, T., and Clarke, G. (2017). Kynurenine pathway metabolism and the microbiota-gut-brain axis. Neuropharmacology 112(Pt B), 399–412.
Kong, F., Hua, Y., Zeng, B., Ning, R., Li, Y., and Zhao, J. (2016). Gut microbiota signatures of longevity. Curr. Biol. 26, R832–R833. doi: 10.1016/j.cub.2016.08.015
Ladwig, S., Ziegler, M., Sudmeyer, M., and Werheid, K. (2022). The post-stroke depression risk scale (PoStDeRiS): Development of an acute-phase prediction model for depression 6 months after stroke. J. Acad. Consult. Liaison Psychiatry 63, 144–152. doi: 10.1016/j.jaclp.2021.08.003
Li, B., He, Y., Ma, J., Huang, P., Du, J., Cao, L., et al. (2019). Mild cognitive impairment has similar alterations as Alzheimer’s disease in gut microbiota. Alzheimers Dement. 15, 1357–1366. doi: 10.1016/j.jalz.2019.07.002
Li, S., Guo, B., Yang, Q., Yin, J., Tian, L., Zhu, H., et al. (2022). Evaluation of depression status and its influencing factors in convalescent elderly patients with first-episode stroke. Asian J. Psychiatr. 77:103252. doi: 10.1016/j.ajp.2022.103252
Ling, Y., Gong, T., Zhang, J., Gu, Q., Gao, X., Weng, X., et al. (2020a). Gut microbiome signatures are biomarkers for cognitive impairment in patients with ischemic stroke. Front. Aging Neurosci. 12:511562. doi: 10.3389/fnagi.2020.511562
Ling, Y., Gu, Q., Zhang, J., Gong, T., Weng, X., Liu, J., et al. (2020b). Structural change of gut microbiota in patients with post-stroke comorbid cognitive impairment and depression and its correlation with clinical features. J. Alzheimers Dis. 77, 1595–1608. doi: 10.3233/JAD-200315
Ling, Z., Cheng, Y., Chen, F., Yan, X., Liu, X., Shao, L., et al. (2022). Changes in fecal microbiota composition and the cytokine expression profile in school-aged children with depression: A case-control study. Front. Immunol. 13:964910. doi: 10.3389/fimmu.2022.964910
Liu, J., Jin, Y., Ye, Y., Tang, Y., Dai, S., Li, M., et al. (2021). The neuroprotective effect of short chain fatty acids against sepsis-associated encephalopathy in mice. Front. Immunol. 12:626894. doi: 10.3389/fimmu.2021.626894
Liu, Y., Kong, C., Gong, L., Zhang, X., Zhu, Y., Wang, H., et al. (2020). The association of post-stroke cognitive impairment and gut microbiota and its corresponding metabolites. J. Alzheimers Dis. 73, 1455–1466. doi: 10.3233/jad-191066
Maaijwee, N., Tendolkar, I., Rutten-Jacobs, L., Arntz, R., Schaapsmeerders, P., Dorresteijn, L., et al. (2016). Long-term depressive symptoms and anxiety after transient ischaemic attack or ischaemic stroke in young adults. Eur. J. Neurol. 23, 1262–1268. doi: 10.1111/ene.13009
Pan, C., Li, G., Jing, P., Chen, G., Sun, W., Miao, J., et al. (2022). Structural disconnection-based prediction of poststroke depression. Transl. Psychiatry 12:461. doi: 10.1038/s41398-022-02223-2
Patrizz, A., Dono, A., Zorofchian, S., Hines, G., Takayasu, T., Husein, N., et al. (2020). Glioma and temozolomide induced alterations in gut microbiome. Sci. Rep. 10:21002. doi: 10.1038/s41598-020-77919-w
Rong, H., Xie, X., Zhao, J., Lai, W., Wang, M., Xu, D., et al. (2019). Similarly in depression, nuances of gut microbiota: Evidences from a shotgun metagenomics sequencing study on major depressive disorder versus bipolar disorder with current major depressive episode patients. J. Psychiatr. Res. 113, 90–99. doi: 10.1016/j.jpsychires.2019.03.017
Sacco, R., Kasner, S., Broderick, J., Caplan, L., Connors, J., Culebras, A., et al. (2013). An updated definition of stroke for the 21st century: A statement for healthcare professionals from the American Heart Association/American Stroke Association. Stroke 44, 2064–2089. doi: 10.1161/STR.0b013e318296aeca
Singh, V., Roth, S., Llovera, G., Sadler, R., Garzetti, D., Stecher, B., et al. (2016). Microbiota dysbiosis controls the neuroinflammatory response after stroke. J. Neurosci. 36, 7428–7440. doi: 10.1523/jneurosci.1114-16.2016
Sun, J., Xu, J., Ling, Y., Wang, F., Gong, T., Yang, C., et al. (2019). Fecal microbiota transplantation alleviated Alzheimer’s disease-like pathogenesis in APP/PS1 transgenic mice. Transl. Psychiatry 9:189. doi: 10.1038/s41398-019-0525-3
Tian, X., Xia, G., Zhang, M., Tan, C., Yang, M., Wang, H., et al. (2022). Effect of enteral nutrition on the intestinal microbiome and risk of death in ischemic stroke patients. JPEN J. Parenter. Enteral Nutr. 46, 1847–1858. doi: 10.1002/jpen.2370
Wang, J., Zhong, Y., Zhu, H., Mahgoub, O., Jian, Z., Gu, L., et al. (2022). Different gender-derived gut microbiota influence stroke outcomes by mitigating inflammation. J. Neuroinflammation 19:245. doi: 10.1186/s12974-022-02606-8
Yi, J., Lu, J., Yang, A., and Marsh, E. (2022). In-hospital predictors of post-stroke depression for targeted initiation of selective serotonin reuptake inhibitors (SSRIs). BMC Psychiatry 22:722. doi: 10.1186/s12888-022-04378-0
Zhang, F., Ping, Y., Jin, X., Hou, X., and Song, J. (2023). White matter hyperintensities and post-stroke depression: A systematic review and meta-analysis. J. Affect. Disord. 320, 370–380. doi: 10.1016/j.jad.2022.09.166
Keywords: 16s rRNA, stroke, gut microbiota, depression, microbial signatures
Citation: Yao S, Xie H, Wang Y, Shen N, Chen Q, Zhao Y, Gu Q, Zhang J, Liu J, Sun J and Tong Q (2023) Predictive microbial feature analysis in patients with depression after acute ischemic stroke. Front. Aging Neurosci. 15:1116065. doi: 10.3389/fnagi.2023.1116065
Received: 05 December 2022; Accepted: 06 March 2023;
Published: 23 March 2023.
Edited by:
Touqeer Ahmed, Johns Hopkins Medicine, Johns Hopkins University, United StatesReviewed by:
Jun-Jie Zhang, Indiana University Bloomington, United StatesHuidi Wang, Southern Medical University, China
Copyright © 2023 Yao, Xie, Wang, Shen, Chen, Zhao, Gu, Zhang, Liu, Sun and Tong. This is an open-access article distributed under the terms of the Creative Commons Attribution License (CC BY). The use, distribution or reproduction in other forums is permitted, provided the original author(s) and the copyright owner(s) are credited and that the original publication in this journal is cited, in accordance with accepted academic practice. No use, distribution or reproduction is permitted which does not comply with these terms.
*Correspondence: Jiaming Liu, wzjiaming_liu@163.com; Jing Sun, sunjwz@126.com; Qiuling Tong, tongqiuling2013@126.com
†These authors have contributed equally to this work