Differential Associations of White Matter Brain Age With Language-Related Mechanisms in Word-Finding Ability Across the Adult Lifespan
- 1Molecular Imaging Centre, National Taiwan University, Taipei, Taiwan
- 2Institute of Medical Device and Imaging, National Taiwan University College of Medicine, Taipei, Taiwan
- 3AcroViz Technology Inc., Taipei, Taiwan
- 4Department of Radiology, Wan Fang Hospital, Taipei Medical University, Taipei, Taiwan
- 5Department of Radiology, College of Medicine, Taipei Medical University, Taipei, Taiwan
Research on cognitive aging has established that word-finding ability declines progressively in late adulthood, whereas semantic mechanism in the language system is relatively stable. The aim of the present study was to investigate the associations of word-finding ability and language-related components with brain aging status, which was quantified by using the brain age paradigm. A total of 616 healthy participants aged 18–88 years from the Cambridge Centre for Ageing and Neuroscience databank were recruited. The picture-naming task was used to test the participants’ language-related word retrieval ability through word-finding and word-generation processes. The naming response time (RT) and accuracy were measured under a baseline condition and two priming conditions, namely phonological and semantic priming. To estimate brain age, we established a brain age prediction model based on white matter (WM) features and estimated the modality-specific predicted age difference (PAD). Mass partial correlation analyses were performed to test the associations of WM-PAD with the cognitive performance measures under the baseline and two priming conditions. We observed that the domain-specific language WM-PAD and domain-general WM-PAD were significantly correlated with general word-finding ability. The phonological mechanism, not the semantic mechanism, in word-finding ability was significantly correlated with the domain-specific WM-PAD. In contrast, all behavioral measures of the conditions in the picture priming task were significantly associated with chronological age. The results suggest that chronological aging and WM aging have differential effects on language-related word retrieval functions, and support that cognitive alterations in word-finding functions involve not only the domain-specific processing within the frontotemporal language network but also the domain-general processing of executive functions in the fronto-parieto-occipital (or multi-demand) network. The findings further indicate that the phonological aspect of word retrieval ability declines as cerebral WM ages, whereas the semantic aspect is relatively resilient or unrelated to WM aging.
Highlights
- Differential associations of white matter (WM) brain age with word-finding ability were observed.
- Domain-specific language system in the phonological component, but not the semantic component, was correlated with WM brain age.
- Word finding functions involve not only the domain-specific processing but also the domain-general processing of executive functions.
- WM brain age is a potential indicator of cognitive decline in language-related word-finding ability.
Introduction
Older adults experience problems in daily word-finding tasks such as recalling precise names of people or objects (Labarge, 1986; Sunderland et al., 1986; Schmitter-Edgecombe et al., 2000). Such performance impairment manifests as slow efficiency, increased pauses during speech production, or being unable to remember phonology when intending to express a known word (Obler and Albert, 1981; Nicholas et al., 1985; Kemper et al., 1990; Cheung and Kemper, 1992; Orange and Purves, 1996). The increased word-finding difficulties constitute a major complaint among older adults.
Two potential cognitive mechanisms underlying these impairments in word retrieval and generation ability have been identified. The first posits that normal aging leads to a decreased ability in language-related ability such as the stored conceptual representations and lexical knowledge; the second posits that normal aging causes a general decline in the executive functions involved in retrieval mechanisms. Working memory and executive functions required for the word retrieval processes involve inhibitory control, lexical selection, and competition monitoring. Earlier studies on cognitive aging found that unlike other cognitive functions that are sensitive to aging, such as working memory and executive functions (Daniels et al., 2006; Dennis and Cabeza, 2008; Collette et al., 2009; Higby et al., 2019), language abilities (e.g., lexical knowledge and semantic representation) remain stable and even improve with age (Kave et al., 2009; Salthouse, 2009; Meyer and Federmeier, 2010; Verhaegen and Poncelet, 2013).
However, some researchers explained the causes of word retrieval failure from other aspects of language processes such as phonological processes (Taylor and Burke, 2002; Ouyang et al., 2020), an age-related decrease of connectivity in the language system (Burke et al., 1991) and stronger lexical competition (Lagrone and Spieler, 2006). Two potential theories have been developed to provide possible interpretations for the age-related decline in language processes. One which is called the inhibition deficit theory (IDT) suggests that the elderly show decreased control of the information processing and become more distracted in both semantic and phonological related mechanisms (Hasher et al., 1991). The other, called the transmission deficit hypothesis (TDH), states that the phonological mechanism weakens with age and results in word retrieval failure, but the semantic mechanism is preserved (Mackay and Burke, 1990).
When linking behavioral performance with neural activities, neuroimaging studies have supported the frontotemporal system as the neural substrate of language function (Van Der Lely and Pinker, 2014; Chang et al., 2015; and Lopez-Barroso and De Diego-Balaguer, 2017; Campbell and Tyler, 2018). Other studies have extended the domain-specific language network (LN) to a domain-general system or multi-demand network including the frontoparietal connections (Duncan, 1995; Unsworth et al., 2014; Cole et al., 2015; Duncan et al., 2017) and observed an association between the age-related decline of the domain-general system or multi-demand network and that of executive functions such as inhibition, attention, and working memory (Duncan et al., 2017; Chen P.-Y. et al., 2020).
Multiple neuroimaging studies have demonstrated that cognitive decline is related to the deterioration of brain structure and function (Archer et al., 2018; Fletcher et al., 2018). Previous studies examined the biological relationships of language and memory abilities with white matter (WM) tracts across adulthoods using diffusion magnetic resonance imaging (MRI) techniques (Ziegler et al., 2010; Stamatakis et al., 2011; Sexton et al., 2014; Feng et al., 2016; Houston et al., 2019). For example, the fronto-temporal link of the inferior fronto-occipital fasciculus (IFOF) might be involved in the figure-recall ability (Voineskos et al., 2012). The IFOF might also play a role in language function according to the investigation of intraoperative electrostimulation to this pathway (Duffau et al., 2005). It has been reported that the posterior projections of the corpus callosum might contribute to the domains in memory and executive function (Voineskos et al., 2012). These findings of varying relationships of different WM tracts with language and memory functions support that the integrity of structural connectivity may contribute to the performance of language and memory functions.
To our knowledge, the performance of word retrieval ability might alter along the dimension of chronological age, but it is unclear whether such ability is related to the biological age of brain structures. The growing body of neuroimaging studies has developed brain age prediction models that involve machine learning techniques to evaluate and quantify an individual’s status of brain aging (Cole and Franke, 2017; Franke and Gaser, 2019). The assumption underlying this approach is that the biological aging process of the brain in a cognitively normal population is chronological, so brain age prediction models are created by estimating the regression pattern between neuroimaging features and chronological age in a normative population. The established models are then applied to the cohort of interest to predict individuals’ brain age. The inference of brain age prediction models can be used to quantify the status of brain aging by calculating the discrepancy between the chronological and biological age of the brain (Smith et al., 2019). The difference between predicted age and chronological age is defined as the predicted age difference (PAD) of the brain, which represents the degree of deviation from the chronological patterns of brain aging in a normative population. The PAD can be regarded as a non-chronological age variable and has been employed to predict the integrity of brain structures, the increased risks of dementia, and the degree of domain-specific cognitive decline (Gaser et al., 2013; Boyle et al., 2020; Cole, 2020). Also, the PAD of WM (WM-PAD) could be used to detect aberrant brain aging in neurodegenerative diseases and psychiatric disorders (Chen et al., 2019, 2020c), and reflect the biological associations with several cognitive functions (Chen et al., 2020a). Employing the brain age paradigm, we aimed to test the hypothesis of word retrieval ability was associated with the biological age of WM.
In the current study, we investigated whether the retrieval ability is a domain-specific language process or requires domain-general mechanisms incorporation, further we examined the association of semantic and phonological components in the language system involved in word-finding ability with the brain aging of WM. We employed the picture-naming task to evaluate word retrieval ability and the semantic and phonological mechanisms involved in a population-based lifespan adult cohort, and the brain age paradigm was used to assess the degree of WM aging. Figure 1 illustrates the experimental design of the present study. Compared to the relationship between cognitive decline and chronological age reported in previous studies (Stevens et al., 2008; Ward et al., 2013; Baciu et al., 2016), we hypothesized that the WM-PAD can differentiate whether the retrieval ability is a domain-specific language process or requires domain-general mechanisms incorporation and further manifest different associations patterns with semantic and phonological mechanisms of language system involved in word-finding ability. Our findings could advance the understanding of the biological aging effect of WM in the word-finding ability.
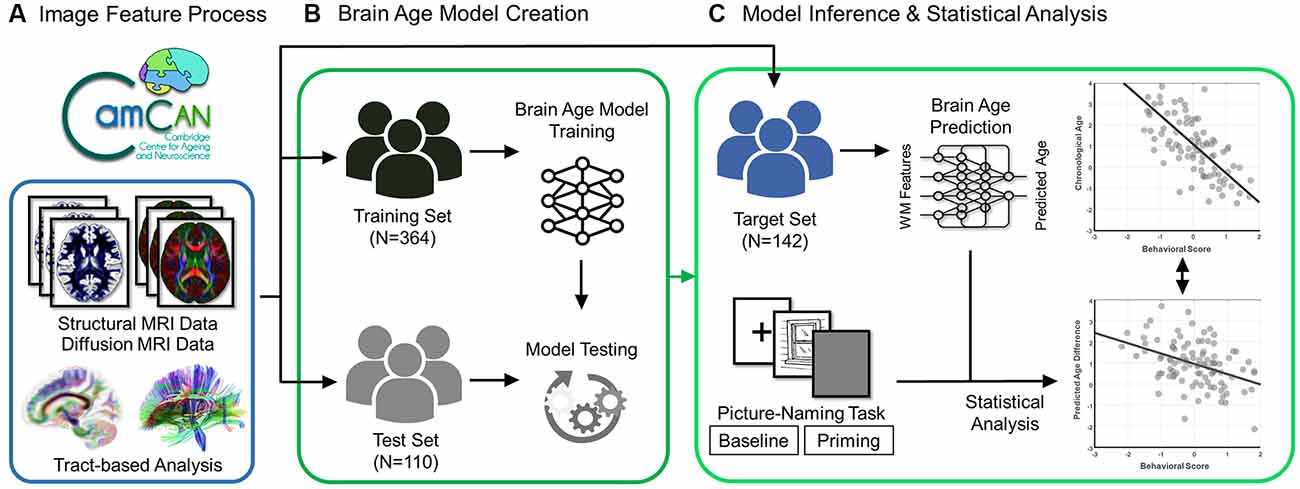
Figure 1. Flowchart of experiment design. The brain scans (structural and diffusion MRI data) and behavioral data (scores of the picture-naming task in baseline and priming conditions) were acquired from the participants in the Cam-CAN Repository. (A) The diffusion MRI data went through the tract-based analysis. (B) The processed data were used to create and test the brain age prediction model in the training and test sets, respectively. (C) Eventually, the established brain age model was applied to the target set to predict the value of the predicted age difference (PAD). The statistical analysis was further conducted to estimate the association of behavioral scores with chronological age and predicted age difference in the target set.
Materials and Methods
Participants
The participants were recruited from the Cambridge Center for Ageing and Neuroscience (Cam-CAN) project1. A detailed description of the recruitment process has been reported in the studies by Shafto et al. (2014) and Taylor et al. (2017). The East of England–Cambridge Central Research Ethics Committee approved the study protocol. After providing written informed consent, all participants underwent a diverse set of neuropsychological tests, cognitive tasks, and MRI scanning. Participants with a Mini-Mental State Examination score of 24 or lower, those with a poor fluency in English, those with poor vision or hearing, those with self-reported substance abuse, and those who were currently experiencing serious health problems were excluded. Participants who did not meet the safety and health-related criteria for MRI were also excluded.
A total of 616 participants aged 18–88 years were recruited. The participants were assigned to one of three groups, namely the training, test, and target groups. These three groups were used to create brain age prediction models, test model performance, and estimate brain age measures for statistical analyses of the experiments, respectively. Specifically, participants who had complete cognitive measurements were preferentially assigned to the target group. The remaining participants were split into the training and test groups through a conditional random approach to guarantee statistically identical age and sex distributions between the groups. Table 1 lists the demographic information of the participants in the three groups.
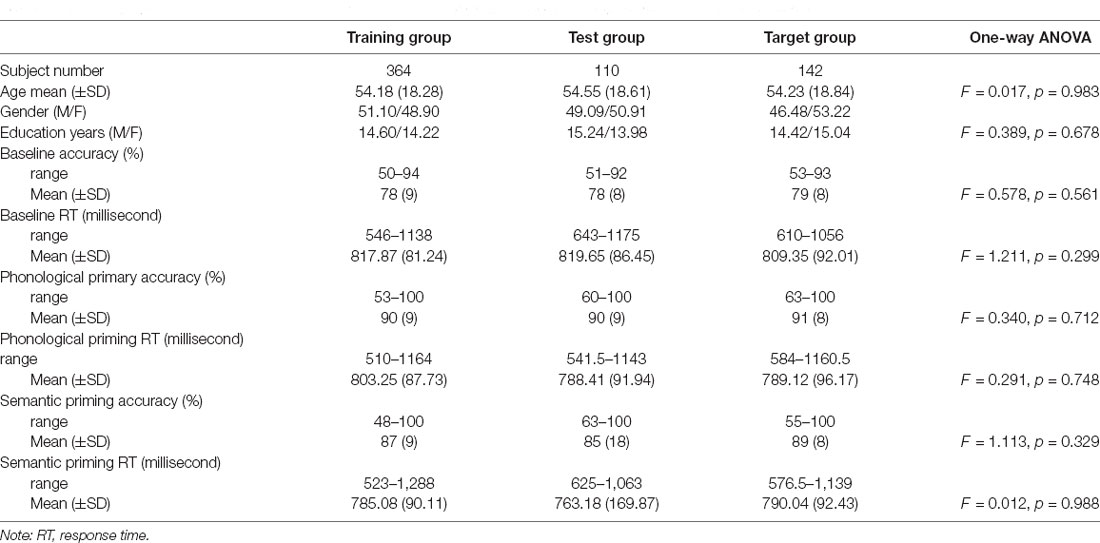
Table 1. Demographics and cognitive performance of the picture-naming task from healthy adults in the Cam-CAN cohort.
Behavioral Task
The picture-naming task was used to test language-related explicit and implicit effects involving word finding and word generation. The task measured the naming response time (RT) and accuracy (ACC) under the baseline condition and two priming conditions (i.e., phonological and semantic priming). The participants were asked to name every picture as quickly and accurately as possible. The baseline condition required an individual to consciously recall language-related cognitive ability (explicit effect). It included 200 pictures of common objects presented in a pseudorandom order. The baseline experiment procedure consisted of a fixation point displayed for 500 ms, followed by the target picture displayed for 750 ms, and finally, a blank screen displayed for 1,000 ms. The priming condition probed an individual’s unconscious language-related ability (implicit effect), activated by specific cues. The priming condition consisted of one baseline picture preceded by a prime picture, which was unrelated, phonologically related, or semantically related to the baseline pictures. Each trial consisted of 500 ms fixation, followed by 750 ms of the prime picture and 1,000 ms blank screen, and proceeded by 750 ms of the target picture and 2,500 ms of a blank screen.
Phonologically related priming pairs included overlapping phonology (first two phonemes in English; e.g., glass–glove or first phonemes in English; e.g., ring–ruler). Semantically related priming pairs included semantic relationships (e.g., tiger–cat or cabinet–box). Unrelated pairs were neither semantically nor phonologically related. Table 1 provides the demographic information and cognitive performance on the picture-naming task of the healthy participants in the study. The paired t-tests of ACC and RT between primed and unrelated conditions were conducted with the estimation of the Cohen’s d for the effect size to evaluate the priming effects (Table 2). To evaluate the cognitive performance in the target group (N = 142), the correlation matrix approach adjusting chronological age and education was employed to examine the associations between the cognitive scores (i.e., ACC and RT) under each condition of tasks (Table 3). Cognitive performance on the picture-naming task was assessed for its correlation with the chronological age variable (Table 4).
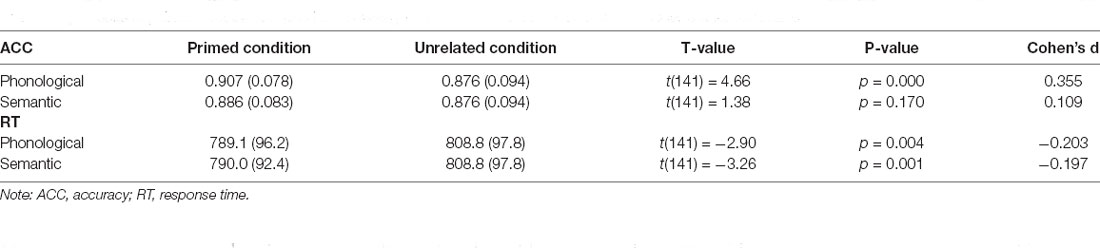
Table 2. Results of paired t-test of ACC and RT between primed and unrelated conditions with Cohen’s d for effect size.
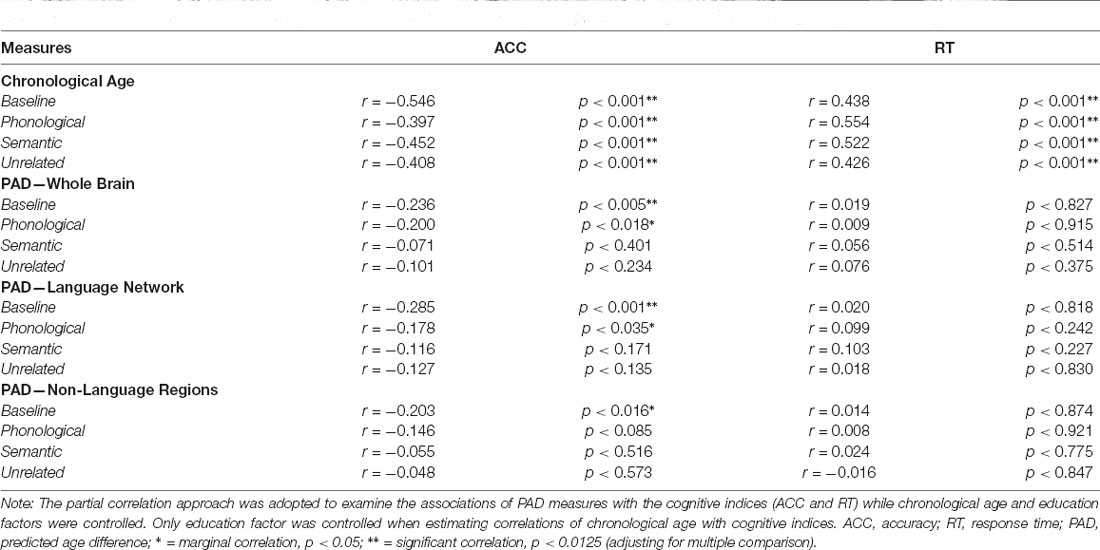
Table 4. Partial correlations (r) between cognitive performances (ACC and RT) of the picture naming task with chronological age and brain age.
Image Acquisition
All participants were scanned using a 3-Tesla MRI scanner (TIM–Trio, Siemens, Erlangen, Germany) at the Medical Research Council Cognition Brain and Sciences Unit, Cambridge, United Kingdom. High-resolution structural MR images were acquired using a three-dimensional rapid acquisition gradient-echo sequence with preparation pulses for T1-weighted contrast. The following imaging parameters were used: TR/TE = 2,250/2.99 ms, inversion time = 900 ms, flip angle = 9°, field of view(FOV) = 256 × 240 × 192 mm3, resolution = 1 mm isotropic, and acquisition time of approximately 4.5 min. Diffusion-weighted images were acquired using a spin-echo sequence with two refocusing pulses to minimize distortion induced by eddy currents. The acquisition scheme entailed 30 diffusion gradient directions for each of the two diffusion sensitivity values (b-value) of 1,000 and 2,000 s/mm2 and three images with a b-value of 0 s/mm2. The imaging parameters were as follows: TR/TE = 9100/104 ms, FOV = 192 × 192 mm2, voxel size = 2 mm isotropic, 66 axial slices, number of averages = 1, and acquisition time = approximately 10 min.
Quality Assurance of Diffusion-Weighted Images
All diffusion data sets underwent quality assurance procedures, including examinations of the signal-to-noise ratio (SNR), the degree of alignment between T1-weighted images and the GFA map, and the detection of abrupt head motion. SNR was evaluated through the calculation of the mean signal of an object divided by the SD of the background noise. In practice, the signal was determined using a central square of an image for each slice, and the noise from four corner regions was averaged. Diffusion data sets were included in the study if they had an SNR higher than the mean SNR minus 2.5 SDs of all participants. The degree of within-participant alignment between T1-weighted images and the GFA map was evaluated through the calculation of the spatial correlation between the WM tissue probability map derived from T1-weighted images and the GFA map. Data sets with correlation coefficients higher than the mean spatial correlation minus 2.5 SDs were included in the study. Instances of abrupt head motion or other visible artifacts were manually identified and removed. The correction algorithm for motion and eddy currents was used to detect and replace slices affected by signal loss due to bulk motion during acquisition (Andersson and Sotiropoulos, 2016). Of the initial 636 images, 616 (96.9%) underwent the quality assurance procedures and qualified for subsequent analyses.
Diffusion Index Estimation Using Regularized Mean Apparent Propagator Algorithm
We used regularized mean apparent propagator (ReMAP) MRI algorithm to reconstruct the diffusion propagator from the diffusion MRI signal (Ozarslan et al., 2013; Hsu and Tseng, 2018). ReMAP MRI fits the diffusion MRI signal with a linear combination of Hermite functions so that the diffusion propagator can be represented by a few coefficients, serving as an efficient dimension reduction method. The coefficients of the diffusion propagator, orientation distribution function (ODF), and diffusion tensor were determined, from which various diffusion indices could be calculated. In this study, we calculated generalized fractional anisotropy (GFA) and mean diffusivity (MD) to represent the microstructural integrity of WM tracts. The GFA value was the standard deviation (SD) of the ODF in all radial directions divided by the norm of the ODF (Tuch, 2004). The value of MD was the mean of the first, second, and third eigenvalues of the diffusion tensor. GFA changes in response to the strength of anisotropic diffusion in the microstructure. MD is the diffusion index that is the most sensitive to age, and it has been considered useful for the prediction of brain age (Cox et al., 2016; Chen et al., 2020b).
Tract-Based Analysis for Diffusion-Weighted Images
We used an in-house analytic method, tract-based automatic analysis (TBAA; Chen et al., 2015), to sample the diffusion index values along the tracts across major WM regions. Figure 2A illustrates the entire process of diffusion index computation and tract-based analysis. In brief, TBAA was used to register diffusion-weighted images of all study participants to a standard template (Hsu et al., 2015), in which tractograms of 76 major WM tracts were developed using deterministic tractography. After registration was complete, the position coordinates of 76 tracts were transformed from the template space to the native space of each participant. Diffusion index values of GFA and MD were sampled along with the coordinates of each tract and averaged to obtain the mean GFA and MD indices for each WM tract. Subsequently, 76 GFA and 76 MD values were obtained from each participant as features for brain age estimation.
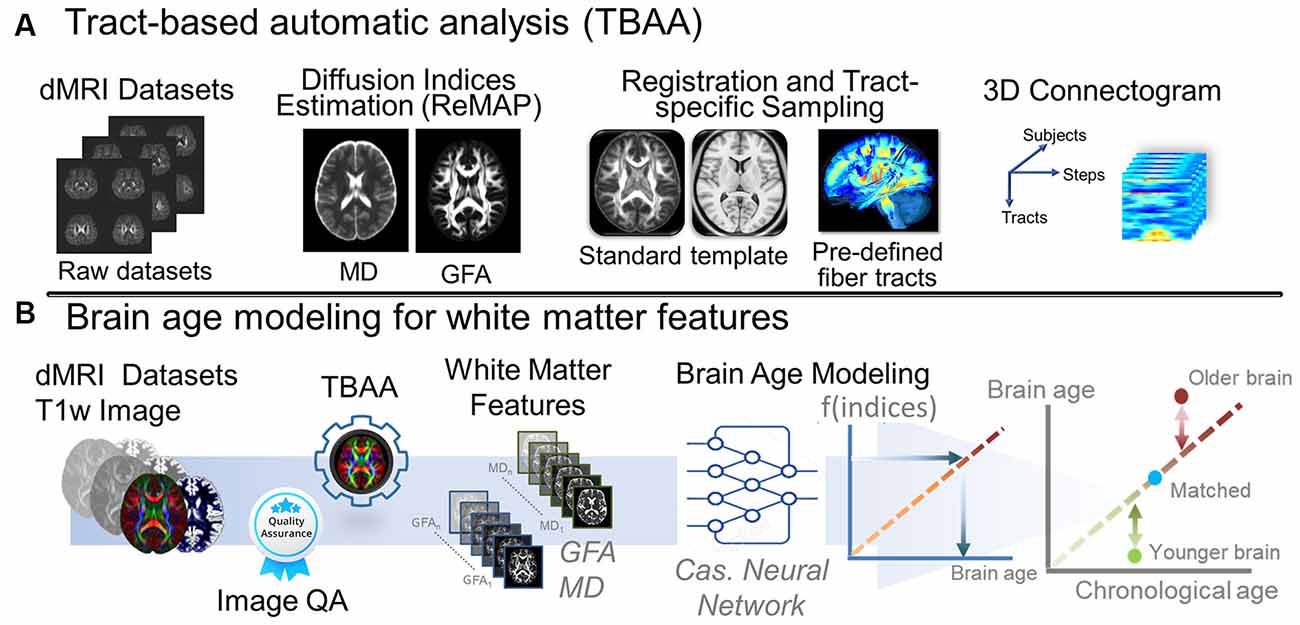
Figure 2. Analytic pipeline of diffusion MRI reconstruction and the corresponding brain age modeling for white matter features. (A) The pipeline depicts the pipeline of TBAA. (B) The pipeline displays the brain age modeling for WM. Abbreviation: dMRI, diffusion magnetic resonance imaging; MD, mean diffusivity; GFA, generalized fractional anisotropy; QA, quality assurance; Cas, cascade; ReMAP, regularized mean apparent propagator reconstruction.
Brain Age Prediction Based on WM Features Using Machine Learning
To estimate the brain age index of the participants, we established brain age prediction models based on the WM features from the training set. To differentiate biological underpinnings of language-related cognitive ability, we created three types of brain age models: models derived from features of the whole brain (WB), language network (LN), and non-language regions (NLR). The WB brain age model provided brain age metrics based on entire WM features (i.e., 76 GFA and 76 MD values). The LN brain age model was built based on the features defined in the language network (Van Der Lely and Pinker, 2014; Chang et al., 2015; Lopez-Barroso and De Diego-Balaguer, 2017); there were 14 tract bundles on language network by our definition, so the LN brain age metrics were estimated using 14 GFA and 14 MD values. Table 5 provides the constitution of the language network in WM. The NLR brain age model was created using the features which were not defined in the language network (i.e., 62 GFA and 62 MD values). Also, all three brain age models contained sex variables as another predictor. In practice, the brain age models were designed to have a 12-layer feedforward cascade neural network, and the related information of model design can be found in our previous study (Chen et al., 2020b). A 10-fold cross-validation procedure was performed to estimate the performance of the brain age model in the training phase. Next, an independent test set was used to evaluate the generalizability of the models. The modeling procedures were implemented using MATLAB R2019a (MathWorks Inc., Natick, MA, USA) with an NVIDIA GeForce RTX 2080Ti (NVIDIA Inc., Santa Clara, CA, USA) graphics processing unit for accelerated computing. The technical details of brain age prediction are provided in the Supplementary Material. The model performance was evaluated according to the Pearson correlation coefficient (Rho) and mean absolute error (MAE) between the predicted age and chronological age. Figure 2B displays the brain age modeling for WM.
Partial Correlation Analysis of Brain Age Measures With Behavioral Task Performance
Partial correlation analysis was employed to address the relationship between age measures (i.e., chronological age and brain age) and language-related cognitive abilities in the target group (N = 142). To quantify the extent of an individual’s brain aging, the PAD was calculated by subtracting chronological age from predicted age (i.e., predicted age − chronological age) to represent the biological age of the brain. In this experiment, we estimated three PAD measures, namely PAD-WM, PAD-LN, and PAD-NLR. Notably, the PAD was correlated with chronological age in the training phase, which represents a statistical bias in regression estimation (Smith et al., 2019). To minimize this bias, the partial correlation approach adjusting chronological age and education was employed to examine the associations of the PADs with the cognitive scores (i.e., ACC and RT) under each condition of tasks. In contrast, the partial correlation analysis only adjusted the education factor when estimating the correlation of cognitive scores with chronological age (Table 4). The Bonferroni correction was used to address multiple comparison problems.
Results
Brain Age Prediction Model
The prediction models estimated participants’ brain age with acceptable performance in the training, test, and target groups. For the WB model, the model performance was Rho = 0.951 and MAE = 4.53 years in the training group (N = 364), Rho = 0.907 and MAE = 6.61 years in the test group (N = 110), and Rho = 0.910 and MAE = 6.11 years in the target group (N = 142). For the LN model, the model performance was Rho = 0.905 and MAE = 6.13 years in the training group, Rho = 0.802 and MAE = 8.68 years in the test group, and Rho = 0.831 and MAE = 7.74 years in the target group. For the NLR model, the model performance was Rho = 0.955 and MAE = 4.33 years in the training group, Rho = 0.904 and MAE = 6.72 years in the test group, and Rho = 0.903 and MAE = 6.57 years in the target group. Besides the WB model, we observed that the NLR model provided better prediction than the LN model. This might result from the number of features employed by the NLR brain age model. The age-related bias in the brain age estimation was minimized by adjusting chronological age in the statistical analyses.
Cognitive Performance and Its Priming Effect
Before conducting correlation analysis, additional paired t-tests were used to examine if the primed condition was faster than the unrelated condition in the target group (N = 142; Table 2). Cohen’s d was also reported as the strength of the priming effect. Under the primed condition, the RTs were 789.1 (96.2) ms and 790.0 (92.4) ms in the phonological and semantic conditions, respectively. These RTs were significantly shorter (p = 0.004 and 0.001, respectively) than those in the unrelated condition, which was 808.8 (97.8) ms. In addition, the same statistical tests were applied to the measures of accuracy. We found that the accuracy at the phonological condition [(mean: 0.907, SD: (0.078)] was significantly better (p < 0.001) than that at the unrelated condition [0.876 (0.094)]; however, the accuracy at the semantic condition [0.886 (0.083)] was comparable to (p = 0.170) that at the unrelated condition.
To evaluate the cognitive performance in the target group (N = 142), the correlation matrix approach adjusting chronological age and education was employed to examine the associations between the cognitive scores (i.e., ACC and RT) under each condition of tasks (Table 3). All p-values were lower than 0.001. Both ACC and RT showed significant positive correlations across the task conditions but baseline ACC showed stronger linear relationships with all the tasks than baseline RT. Unrelated RT showed stronger linear relationships with all the tasks than unrelated ACC. For the correlation matrix examining the associations between the priming effects, each pair across ACC and RT represented similar linear relationships.
Correlation Between Behavioral Task Performance on the Picture-Naming and Chronological Age
The performance (measured according to ACC and RT) of participants in the target group (N = 142) under the baseline and priming conditions in the picture-naming task was significantly correlated with their chronological age (Table 4). ACC showed significant negative correlations (p < 0.001) with chronological age under the baseline and priming conditions, whereas RT exhibited significant positive correlations (p < 0.001). Therefore, the chronological age variable was a strong factor influencing participants’ performance on the picture-naming task.
Partial Correlations Between the WM PAD and Behavioral Task Performance on the Picture-Naming Task
Table 4 presents the partial correlations (r) between the cognitive indices (ACC and RT) in the picture-naming tasks and PAD measures of the target group (N = 142), and Figures 3–5 provide the results. The PAD-WB was significantly correlated with ACC under the baseline condition (r = −0.236, p = 0.005) but not with RT (r = 0.019, p = 0.827). Under the phonological-related priming condition, the PAD-WB was marginally associated with ACC (r = −0.200, p = 0.018; the Bonferroni-corrected threshold α = 0.0125) but not with RT (r = 0.009, p = 0.915). Similarly, the PAD-LN was significantly correlated with ACC under the baseline condition (r = −0.285, p = 0.001) but not with RT (r = 0.020, p = 0.818). Under the phonological-related priming condition, the PAD-LN was also marginally associated with ACC (r = −0.178, p = 0.035; the Bonferroni-corrected threshold α = 0.0125) but not with RT (r = 0.099, p = 0.242). However, the PAD-NLR was only marginally associated with ACC at the baseline condition (r = −0.203, p = 0.016). Under either the semantic-related priming or unrelated condition, all PAD measures (i.e., WB, LN, and NLR) did not exhibit significant associations with ACC or RT (Table 4).
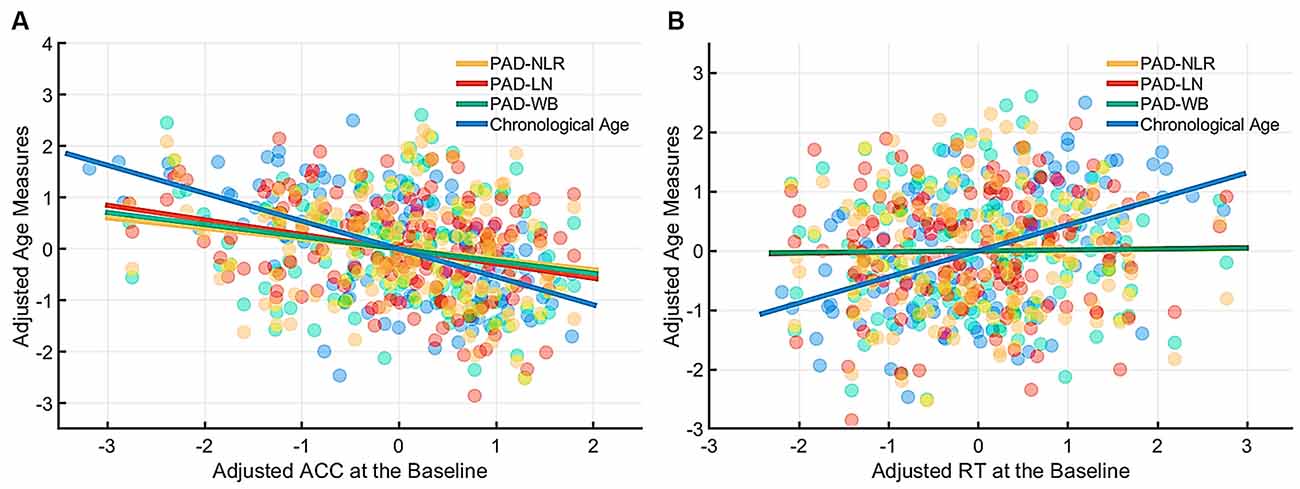
Figure 3. Partial correlation of cognitive indices (ACC and RT) at the baseline condition with age measures (chronological age and brain age). The brain age measures were significantly correlated with ACC under the baseline condition (A) but not with RT. (B) The lines of PAD (NLR, LN, and WB) were almost overlapped. Abbreviation: ACC, accuracy; RT, response time; PAD, predicted age difference; NLR, non-language regions; LN, language network; WB, whole brain.
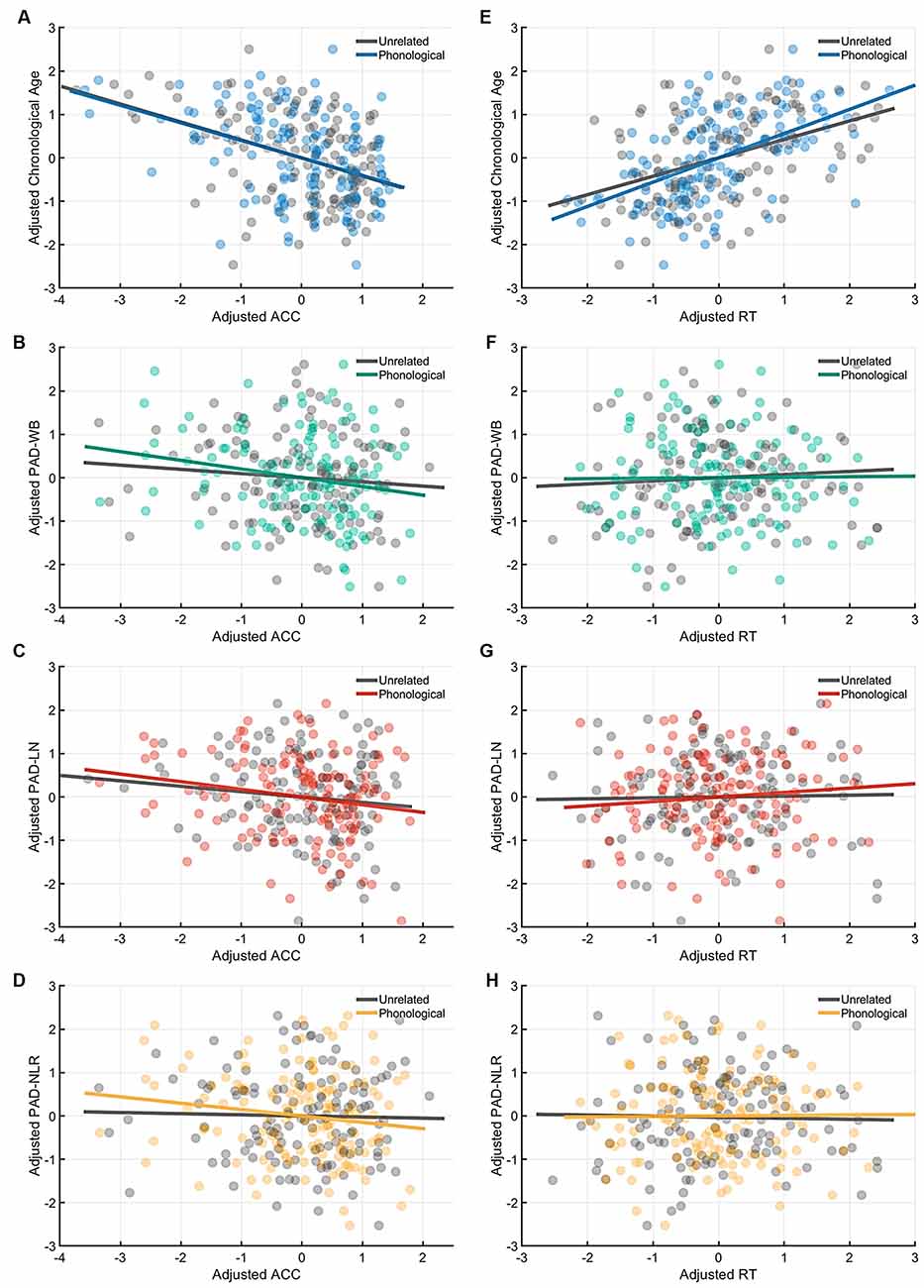
Figure 4. Partial correlation of the cognitive indices (ACC and RT) at the phonological-priming condition with age measures (chronological age and brain age). Under the phonological-related priming condition, chronological age was significantly correlated with ACC (A), and the PAD-WB and PAD-LN were marginally associated with ACC. (B–C) However, the PAD-NLR was not associated with ACC. (D) On the other hand, only chronological age was significantly correlated with RT (E), whereas all brain age measures were not correlated with RT (F–H). Abbreviation: ACC, accuracy; RT, response time; PAD, predicted age difference; NLR, non-language regions; LN, language network; WB, whole brain.
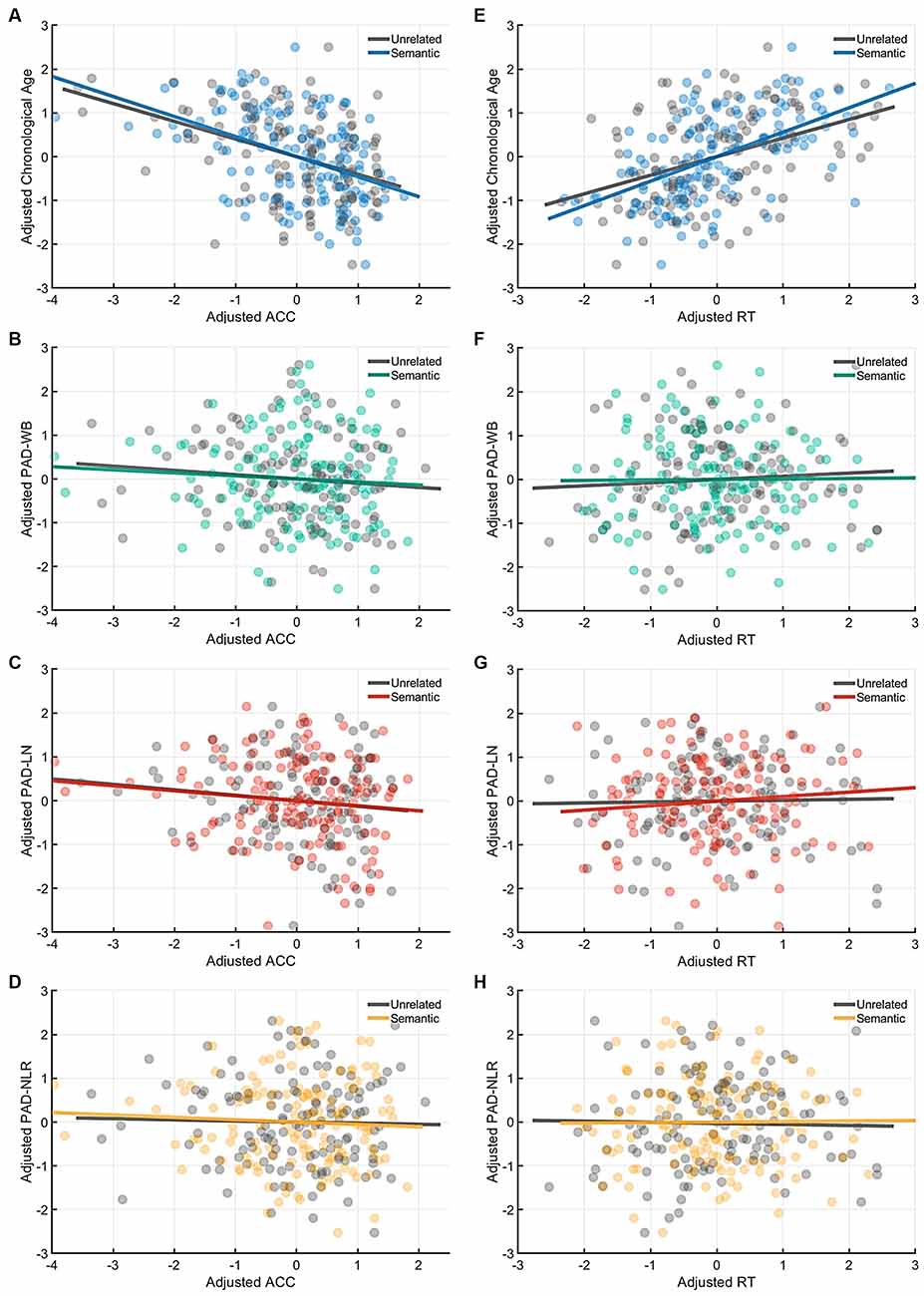
Figure 5. Partial correlation of the cognitive indices (ACC and RT) at the semantic-priming condition with age measures (chronological age and brain age). Under the semantic-related priming condition, chronological age was significantly correlated with ACC (A), but no brain age measures were associated with ACC. (B–D) Likewise, only chronological age was significantly correlated with RT (E), whereas all brain age measures were not correlated with RT (F–H). Abbreviation: ACC, accuracy; RT, response time; PAD, predicted age difference; NLR, non-language regions; LN, language network; WB, whole brain.
Discussion
To clarify the role of biological age of WM in word-finding ability, we investigated the associations of word-finding ability including semantic and phonological priming in language system with WM brain age; we tested the partial correlation between the performance indices (ACC and RT) in the picture-priming task and the language network, non-language network and whole brain of WM-PAD while adjusting for chronological age and education. Our results revealed two major findings: First, all WM-PADs in the language-network, non-language network, and over the whole-brain, which represented whole cerebral WM features instead of being confined to the frontotemporal language network, exhibited statistical associations with ACC in word retrieval functions while controlling the effect of chronological age. Such findings imply that cognitive alterations in word-finding functions involve not only the domain-specific language processing within the frontotemporal language network but also the domain-general processing of executive functions in the fronto-parieto-occipital (or multi-demand) network (Diachek et al., 2020). Second, the phonological priming ACC in the picture-naming task was associated with the language-network WM-PAD, whereas the semantic priming ACC in the picture-naming task exhibited no significant correlations. Chronological age was significantly associated with all conditions in the picture-priming task. The results suggest that for word-finding ability, the semantic mechanism in the language system might be more preserved than the phonological-related mechanism in the language system for the biological aging of WM. The findings further indicate that the phonological aspect of language slightly declines with increases in WM brain age, whereas the semantic aspect is relatively resistant or unrelated to WM aging.
Brain Age Vs. Chronological Age in Word-Finding Ability
Multiple studies on cognitive aging have evaluated the association between cognitive functions and chronological age (Mackay and Burke, 1990; Hasher et al., 1991; Burke and Shafto, 2004; Lagrone and Spieler, 2006). Research on cognitive aging has established that the ability to successfully retrieve proper words from stored knowledge and concepts in long–term memory progressively declines in late adulthood (Christensen et al., 1997; Davis et al., 2001; Fleischman et al., 2004). The impaired word-generation ability in older individuals may attribute to the decline in retrieval mechanism of executive functions instead of impaired semantic representation (Fisk and Sharp, 2004; Waters and Caplan, 2005; Daniels et al., 2006; Dennis and Cabeza, 2008; Collette et al., 2009; Baciu et al., 2016; Higby et al., 2019). In our results, the ACC in baseline condition in the picture-priming task was significantly negative-correlated with the WM-PAD across the language network, non-language network, and whole-brain (Table 4). The findings show that the general word-finding ability reflected by the baseline condition of the picture-priming task not only requires support from the domain-specific language network but the broad domain-general system as well. The finding supports the previous research that the word retrieval mechanism involves language-related processes such as searching for linguistic information that would help assess the target, it also interacts among attention and executive functions of the domain-general system such as monitoring and inhibition to suppress competing representations and enhance the efficient retrieval from the long–term memory (Nozari et al., 2016; Nozari and Novick, 2017; Higby et al., 2019).
WM Brain Age and Language-Related Mechanisms in Word-Finding Ability
In this study, ACC for phonological priming conditions showed a negative correlation with both the language-network WM-PAD and whole-brain WM-PAD but no significant relationship with non-language network WM-PAD (Table 4), meaning that the decline of ACC for phonological priming was associated with a higher WM-PAD and the phonological priming focuses on domain-specific language system. Older adults experience difficulties in retrieving and producing proper words because of weakening processes related to the phonological aspects of language ability (Taylor and Burke, 2002; Ossher et al., 2013; Rizio et al., 2017). Our results support those of earlier studies and further demonstrate that the biological relationship of WM brain age with the phonological mechanism in the language system occurs under conditions of phonological priming. The results imply that phonological priming might be affected by the biological aging of WM.
Contrary to the phonological component in the language system, the semantic component exhibited no significant linear relationships with WM-PAD. Previous behavioral studies have revealed that semantic processes, conceptual knowledge, and information are preserved during normal aging (Waters and Caplan, 2005; Kave et al., 2009; Salthouse, 2009; Meyer and Federmeier, 2010; Verhaegen and Poncelet, 2013). Multiple studies on cognitive aging have reported that language abilities such as lexical knowledge and semantic representation remain stable and even improve with age (Kave et al., 2009; Salthouse, 2009; Meyer and Federmeier, 2010; Verhaegen and Poncelet, 2013). The discrepancy between phonological and semantic components might implicate that, in the normal aging process, the biological effect of WM affects the retrieval ability of executive functions and word production speed more than the loss of conceptual or lexical knowledge (semantic component). The current finding supports the transmission deficit hypothesis (TDH) stating the phonological mechanism weakens with age and results in word retrieval failure, but the semantic mechanism is preserved (Mackay and Burke, 1990). TDH assumed that the semantic function requires multiple connections between the lexical component of a word and its semantic representation. and the multiplicity of connections may make it resistant to word-finding failure. In contrast, the phonological representation shows one-to-one mapping to the lexical component of a word, so it becomes vulnerable to aging (Burke and Shafto, 2004).
Previous studies have reported a chronological age-related decline in word-finding ability reflected by reduced retrieval speed (RT) or ACC (Stevens et al., 2008; Rizio et al., 2017). Consistently, we found that both ACC and RT were significantly correlated with chronological age. However, by using the WM brain age instead, our results showed that the WM-PAD was significantly associated with the cognitive measures in ACC but not in RT. This implicates that ACC and RT might reflect distinct dimensions of word-finding capacity; the ACC index reflected an individual’s retrieval precision, and the RT index manifested an individual’s retrieval efficiency. This discrepancy in the current study implies that the WM-PAD may not fully explain the word retrieval efficiency measured by RT in the picture-priming task.
In contrast to the effect of chronological age, neuroimaging-based brain age measures can reflect the differential trajectory against chronological age that might attribute to individual variations in domain-specific cognitions (Cole et al., 2018) and represent the status along the dimension of biological aging. Our study investigated the domain-specific association between language-related mechanisms in word retrieval processes and WM aging (i.e., WM-PAD), and the results showed that the language components that participated in word-finding ability were correlated with the biological age of WM, suggesting that the effect of WM aging might selectively contribute to the changes in word-finding ability. These findings provided the genuine associations of WM aging with language-related mechanisms in word retrieval processes and could advance our understanding of differentiating the effects between chronological aging and biological aging in WM on general word-finding ability and further the language-related mechanisms in word retrieval processes.
Limitations
This study has some limitations. First, the picture-naming task adopted to assess word-finding ability in the current study is one of many cognitive tasks. Various tasks are used to assess cognitive functions, and a single task cannot fully assess the performance of complex cognitive functions such as language and memory. The picture-naming task, including the baseline and priming conditions, focuses on testing the abilities of conceptual recognition and word generation. The design of the picture priming task in our study did not include an encoding phase and the design of the priming conditions did not meet the conventional design of repetition priming which includes the encoding and the testing phase. Nevertheless, the present findings represent only a limited representation of cognitive aging involving language-related priming and word retrieval mechanism. Exploration of the associations of brain age with other cognitive tasks would provide a more complete picture of the brain–cognition relationships in cognitive aging. Second, the brain age models that we developed might not be optimal. For WM brain age, we used GFA and MD only on 76 major fiber tracts as our features of machine learning. We did not explore the whole spectrum of diffusion indices such as axial diffusivity, radial diffusivity, kurtosis, or neurite density, each of which represents distinct characteristics of WM microstructure. Adding some of the indices to brain age modeling might improve its association with cognitive aging. In this study, we built the brain age model using WM features over the entire brain. The null results for WM brain age might be attributed to the fact that the 76 major fiber tracts do not include the small fibers or U fibers in the brain because of limitations of the diffusion MRI technology used in this study. Therefore, the WM features in the present study do not capture the full scope of WM changes. Further studies are required to develop various brain age models to clarify the relationship between brain aging and cognitive aging.
Conclusion
By investigating the associations between the PAD and task indices of memory functions, we revealed the genuine effect of WM aging on word-finding ability and its language-related mechanisms regardless of the chronological age effect. All behavioral measures of picture priming tasks were associated with chronological age, whereas only general word retrieval ability and the phonological-related word-finding ability were correlated with WM brain age. The results suggest that chronological aging and brain aging have differential effects on word retrieval functions; additionally, a new paradigm is introduced to investigate brain correlates of cognitive aging using brain age modeling.
Data Availability Statement
The datasets presented in this study can be found in online repositories. The names of the repository/repositories and accession number(s) can be found below: https://www.cam-can.org/index.php?content=dataset.
Ethics Statement
The studies involving human participants were reviewed and approved by The East of England–Cambridge Central Research Ethics Committee. The patients/participants provided their written informed consent to participate in this study.
Author Contributions
P-YC: conceptualization, writing—original draft. C-LC: writing—original draft, formal analysis, and visualization. H-MT: formal analysis and visualization. Y-CH: software. W-YT: conceptualization, writing—original draft, and resources. All authors contributed to the article and approved the submitted version.
Funding
This research was supported in part by Taiwan National Health Research Institute (Grant number: NHRI-EX109-10928NI; W-YT) and Taipei Medical University (Grant number: 110-swf-03; WC and C-WH). Cam-CAN research was supported by the Biotechnology and Biological Sciences Research Council (Grant #BB/H008217/1). This project has also received funding from the European Union’s Horizon 2020 research and innovation program (Grant agreement #732592).
Conflict of Interest
Y-CH was employed by the company AcroViz Technology Inc., Taipei, Taiwan. The remaining authors declare that the research was conducted in the absence of any commercial or financial relationships that could be construed as a potential conflict of interest.
Publisher's Note
All claims expressed in this article are solely those of the authors and do not necessarily represent those of their affiliated organizations, or those of the publisher, the editors and the reviewers. Any product that may be evaluated in this article, or claim that may be made by its manufacturer, is not guaranteed or endorsed by the publisher.
Acknowledgments
We thank the members of the Cam-CAN team, who provided valuable assistance in recruiting participants, developing the protocol, conducting tests, and overseeing data management. We also thank the Cam-CAN respondents and their primary care team at Cambridge for their participation in this study.
Footnotes
Supplementary Material
The Supplementary Material for this article can be found online at: https://www.frontiersin.org/articles/10.3389/fnagi.2021.701565/full#supplementary-material.
References
Andersson, J. L. R., and Sotiropoulos, S. N. (2016). An integrated approach to correction for off-resonance effects and subject movement in diffusion MR imaging. NeuroImage 125, 1063–1078. doi: 10.1016/j.neuroimage.2015.10.019
Archer, J. A., Lee, A., Qiu, A., and Chen, S. A. (2018). Working memory, age and education: a lifespan fMRI study. PLoS One 13:e0194878. doi: 10.1371/journal.pone.0194878
Baciu, M., Boudiaf, N., Cousin, E., Perrone-Bertolotti, M., Pichat, C., Fournet, N., et al. (2016). Functional MRI evidence for the decline of word retrieval and generation during normal aging. AGE 38:3. doi: 10.1007/s11357-015-9857-y
Boyle, R., Jollans, L., Rueda-Delgado, L. M., Rizzo, R., Yener, G. G., Mcmorrow, J. P., et al. (2020). Brain-predicted age difference score is related to specific cognitive functions: a multi-site replication analysis. Brain Imaging Behav. 15, 327–345. doi: 10.1007/s11682-020-00260-3
Burke, D. M., MacKay, D. G., Worthley, J. S., and Wade, E. (1991). On the tip of the tongue: What causes word findings failures in young and older adults? J. Mem. Lang. 30, 542–579.
Burke, D. M., and Shafto, M. A. (2004). Aging and language production. Curr. Dir. Psychol. Sci. 13, 21–24. doi: 10.1111/j.0963-7214.2004.01301006.x
Campbell, K. L., and Tyler, L. K. (2018). Language-related domain-specific and domain-general systems in the human brain. Curr. Opin. Behav. Sci. 21, 132–137. doi: 10.1016/j.cobeha.2018.04.008
Chang, E. F., Raygor, K. P., and Berger, M. S. (2015). Contemporary model of language organization: an overview for neurosurgeons. J. Neurosurg. 122, 250–261. doi: 10.3171/2014.10.JNS132647
Chen, C.-L., Chen, P.-Y., Tung, Y.-H., Hsu, Y.-C., and Tseng, W.-Y. I. (2020a). Multimodal brain age gap as a mediating indicator in the relation between modifiable dementia risk factors and cognitive functioning. bioRxiv [Preprint]. doi: 10.1101/2020.09.23.309369
Chen, C.-L., Hsu, Y.-C., Yang, L.-Y., Tung, Y.-H., Luo, W.-B., Liu, C. M., et al. (2020b). Generalization of diffusion magnetic resonance imaging-based brain age prediction model through transfer learning. NeuroImage 217:116831. doi: 10.1016/j.neuroimage.2020.116831
Chen, C.-L., Hwang, T.-J., Tung, Y.-H., Yang, L.-Y., Hsu, Y.-C., Liu, C.-M., et al. (2020c). Multifaceted brain age measures reveal premature brain aging and associations with clinical manifestations in schizophrenia. medRxiv [Preprint]. doi: 10.1101/2020.11.09.20228064
Chen, C. L., Shih, Y. C., Liou, H. H., Hsu, Y. C., Lin, F. H., and Tseng, W. I. (2019). Premature white matter aging in patients with right mesial temporal lobe epilepsy: a machine learning approach based on diffusion mri data. NeuroImage Clin. 24:102033. doi: 10.1016/j.nicl.2019.102033
Chen, P.-Y., Chen, C.-L., Hsu, Y.-C., Cam, C. A. N., and Tseng, W.-I. (2020). Fluid intelligence is associated with cortical volume and white matter tract integrity within multiple-demand system across adult lifespan. NeuroImage 212:116576. doi: 10.1016/j.neuroimage.2020.116576
Chen, Y. J., Lo, Y. C., Hsu, Y. C., Fan, C. C., Hwang, T. J., Liu, C. M., et al. (2015). Automatic whole brain tract-based analysis using predefined tracts in a diffusion spectrum imaging template and an accurate registration strategy. Hum. Brain Mapp. 36, 3441–3458. doi: 10.1002/hbm.22854
Cheung, H., and Kemper, S. (1992). Competing complexity metrics and adults’ production of complex sentences. App. Psychol. 13, 53–76. doi: 10.1017/S0142716400005427
Christensen, H., Henderson, A. S., Griffiths, K., and Levings, C. (1997). Does ageing inevitably lead to declines in cognitive performance? a longitudinal study of elite academics. Per. Individual Differences 23, 67–78. doi: 10.1016/S0191-8869(97)00022-6
Cole, J. H. (2020). Multimodality neuroimaging brain-age in UK biobank: relationship to biomedical, lifestyle and cognitive factors. Neurobiol. Aging 92, 34–42. doi: 10.1016/j.neurobiolaging.2020.03.014
Cole, J. H., and Franke, K. (2017). Predicting age using neuroimaging: innovative brain ageing biomarkers. Trends Neurosci. 40, 681–690. doi: 10.1016/j.tins.2017.10.001
Cole, J. H., Marioni, R. E., Harris, S. E., and Deary, I. J. (2018). Brain age and other bodily ’ages’: implications for neuropsychiatry. Mol. Psychiatry 24, 266–281. doi: 10.1038/s41380-018-0098-1
Cole, M. W., Ito, T., and Braver, T. S. (2015). Lateral prefrontal cortex contributes to fluid intelligence through multinetwork connectivity. Brain Connect. 5, 497–504. doi: 10.1089/brain.2015.0357
Collette, F., Germain, S., Hogge, M., and Van Der Linden, M. (2009). Inhibitory control of memory in normal ageing: dissociation between impaired intentional and preserved unintentional processes. Memory 17, 104–122. doi: 10.1080/09658210802574146
Cox, S. R., Ritchie, S. J., Tucker-Drob, E. M., Liewald, D. C., Hagenaars, S. P., Davies, G., et al. (2016). Ageing and brain white matter structure in 3,513 UK Biobank participants. Nat. Commun. 7:13629. doi: 10.1038/ncomms13629
Daniels, K., Toth, J., and Jacoby, L. (2006). “The aging of executive functions,” in Lifespan Cognition: Mechanisms of Change, eds E. Bialystok and F. I. M. Craik (New York, NY: Oxford University Press), 96–111.
Davis, H. P., Trussell, L. H., and Klebe, K. J. (2001). A ten-year longitudinal examination of repetition priming, incidental recall, free recall and recognition in young and elderly. Brain Cogn. 46, 99–104. doi: 10.1016/s0278-2626(01)80043-9
Dennis, N. A., and Cabeza, R. (2008). “Neuroimaging of healthy cognitive aging,” in The Handbook of Aging and Cognition, eds F. I. M. Craik and T. A. Salthouse (New York, NJ: Psychology Press), 1–54.
Diachek, E., Blank, I., Siegelman, M., Affourtit, J., and Fedorenko, E. (2020). The domain-general multiple demand (MD) network does not support core aspects of language comprehension: a large-scale fmri investigation. J. Neurosci. 40, 4536–4550. doi: 10.1523/JNEUROSCI.2036-19.2020
Duffau, H., Gatignol, P., Mandonnet, E., Peruzzi, P., Tzourio-Mazoyer, N., and Capelle, L. (2005). New insights into the anatomo-functional connectivity of the semantic system: a study using cortico-subcortical electrostimulations. Brain 128, 797–810. doi: 10.1093/brain/awh423
Duncan, J. (1995). “Attention, intelligence and the frontal lobes,” in The Cognitive Neuroscience, ed M. S. Gazzaniga (Cambridge, MA: MIT Press),721–733.
Duncan, J., Chylinski, D., Mitchell, D. J., and Bhandari, A. (2017). Complexity and compositionality in fluid intelligence. Proc. Natl. Acad. Sci. U S A 114, 5295–5299. doi: 10.1073/pnas.1621147114
Feng, G., Chen, Q., Zhu, Z., and Wang, S. (2016). Separate brain circuits support integrative and semantic priming in the human language system. Cereb. Cortex 26, 3169–3182. doi: 10.1093/cercor/bhv148
Fisk, J. E., and Sharp, C. A. (2004). Age-related impairment in executive functioning: updating, inhibition, shifting and access. J. Clin. Exp. Neuropsychol. 26, 874–890. doi: 10.1080/13803390490510680
Fleischman, D. A., Wilson, R. S., Gabrieli, J. D., Bienias, J. L., and Bennett, D. A. (2004). A longitudinal study of implicit and explicit memory in old persons. Psychol. Aging 19, 617–625. doi: 10.1037/0882-7974.19.4.617
Fletcher, E., Gavett, B., Harvey, D., Farias, S. T., Olichney, J., Beckett, L., et al. (2018). Brain volume change and cognitive trajectories in aging. Neuropsychology 32, 436–449. doi: 10.1037/neu0000447
Franke, K., and Gaser, C. (2019). Ten years of brainage as a neuroimaging biomarker of brain aging: what insights have we gained? Front. Neurol. 10:789. doi: 10.3389/fneur.2019.00789
Gaser, C., Franke, K., Kloppel, S., Koutsouleris, N., Sauer, H., and Alzheimer’s Disease Neuroimaging Initiative (2013). BrainAGE in mild cognitive impaired patients: predicting the conversion to Alzheimer’s disease. PLoS One 8:e67346. doi: 10.1371/journal.pone.0067346
Hasher, L., Stoltzfus, E. R., Zacks, R. T., and Rypma, B. (1991). Age and inhibition. J. Exp. Psychol. Learn. Mem. Cogn. 17, 163–169. doi: 10.1037//0278-7393.17.1.163
Higby, E., Cahana-Amitay, D., Vogel-Eyny, A., Spiro, A., 3rd, Albert, M. L., and Obler, L. K. (2019). The role of executive functions in object- and action-naming among older adults. Exp. Aging Res. 45, 306–330. doi: 10.1080/0361073X.2019.1627492
Houston, J., Allendorfer, J., Nenert, R., Goodman, A. M., and Szaflarski, J. P. (2019). White matter language pathways and language performance in healthy adults across ages. Front. Neurosci. 13:1185. doi: 10.3389/fnins.2019.01185
Hsu, Y. C., Lo, Y. C., Chen, Y. J., Wedeen, V. J., and Isaac Tseng, W. Y. (2015). NTU-DSI-122: a diffusion spectrum imaging template with high anatomical matching to the ICBM-152 space. Hum. Brain Mapp. 36, 3528–3541. doi: 10.1002/hbm.22860
Hsu, Y. C., and Tseng, W. I. (2018) “An efficient regularization method for diffusion MAP-MRI estimation,” in Joint Annual Meeting ISMRM-ESMRM (Paris, France).
Kave, G., Samuel-Enoch, K., and Adiv, S. (2009). The association between age and the frequency of nouns selected for production. Psychol. Aging 24, 17–27. doi: 10.1037/a0014579
Kemper, S., Rash, S., Kynette, D., and Norman, S. (1990). Telling stories: the structure of adults’ narratives. Eur. J. Cogn. Psychol. 2, 205–228. doi: 10.1080/09541449008406205
Labarge, E. (1986). Performance of normal elderly on the boston naming test. Brain Lang. 27, 380–384. doi: 10.1016/0093-934x(86)90026-x
Lagrone, S., and Spieler, D. H. (2006). Lexical competition and phonological encoding in young and older speakers. Psychol. Aging 21, 804–809. doi: 10.1037/0882-7974.21.4.804
Lopez-Barroso, D., and De Diego-Balaguer, R. (2017). Language learning variability within the dorsal and ventral streams as a cue for compensatory mechanisms in aphasia recovery. Front. Hum. Neurosci. 11:476. doi: 10.3389/fnhum.2017.00476
Mackay, D. G., and Burke, D. M. (1990). “Cognition and aging: new learning and the use of old connections,” in Aging and Cognition: Knowledge Organization and Utilization, ed T. M. Hess (Amsterdam: North Holland), 213–263.
Meyer, A. M., and Federmeier, K. D. (2010). Event-related potentials reveal the effects of aging on meaning selection and revision. Psychophysiology 47, 673–686. doi: 10.1111/j.1469-8986.2010.00983.x
Nicholas, M., Obler, L., Albert, M., and Goodglass, H. (1985). Lexical retrieval in healthy aging. Cortex 21, 595–606. doi: 10.1016/s0010-9452(58)80007-6
Nozari, N., and Novick, J. (2017). Monitoring and control in language production. Curr. Dir. Psychol. Sci. 26, 403–410. doi: 10.1177/0963721417702419
Nozari, N., Freund, M., Breining, B., Rapp, B., and Gordon, B. (2016). Cognitive control during selection and repair in word production. Lang. Cogn. Neurosci. 31, 886–903. doi: 10.1080/23273798.2016.1157194
Obler, L. K., and Albert, M. L. (1981). “Language and aging: A neurobehavioral analysis,” in Aging: Communication Process and Disorders, ed B. G. A. Davis (New York: Grune & Stratton), 107–121.
Orange, J. B., and Purves, B. (1996). Conversational discourse and cognitive impairment: implications for Alzheimer’s disease. J. Speech-Lang. Pathol. Audiol. 20, 139–150.
Ossher, L., Flegal, K. E., and Lustig, C. (2013). Everyday memory errors in older adults. Neuropsychol. Dev. Cogn. B Aging Neuropsychol. Cogn. 20, 220–242. doi: 10.1080/13825585.2012.690365
Ouyang, M., Cai, X., and Zhang, Q. (2020). Aging effects on phonological and semantic priming in the tip-of-the-tongue: evidence from a two-step approach. Front. Psychol. 11:338. doi: 10.3389/fpsyg.2020.00338
Ozarslan, E., Koay, C. G., Shepherd, T. M., Komlosh, M. E., Irfanoglu, M. O., Pierpaoli, C., et al. (2013). Mean apparent propagator (MAP) MRI: a novel diffusion imaging method for mapping tissue microstructure. NeuroImage 78, 16–32. doi: 10.1016/j.neuroimage.2013.04.016
Rizio, A. A., Moyer, K. J., and Diaz, M. T. (2017). Neural evidence for phonologically based language production deficits in older adults: an fMRI investigation of age-related differences in picture-word interference. Brain Behav. 7:e00660. doi: 10.1002/brb3.660
Schmitter-Edgecombe, M., Vesneski, M., and Jones, D. W. R. (2000). Aging and word-finding: a comparison of spontaneous and constrained naming tests. Arch. Clin. Neuropsychol. 15, 479–493. doi: 10.1016/S0887-6177(99)00039-6
Sexton, C. E., Walhovd, K. B., Storsve, A. B., Tamnes, C. K., Westlye, L. T., Johansen-Berg, H., et al. (2014). Accelerated changes in white matter microstructure during aging: a longitudinal diffusion tensor imaging study. J. Neurosci. 34, 15425–15436. doi: 10.1523/JNEUROSCI.0203-14.2014
Shafto, M. A., Tyler, L. K., Dixon, M., Taylor, J. R., Rowe, J. B., Cusack, R., et al. (2014). The cambridge Center for ageing and neuroscience (Cam-CAN) study protocol: a cross-sectional, lifespan, multidisciplinary examination of healthy cognitive ageing. BMC Neurol. 14:204. doi: 10.1186/s12883-014-0204-1
Smith, S. M., Vidaurre, D., Alfaro-Almagro, F., Nichols, T. E., and Miller, K. L. (2019). Estimation of brain age delta from brain imaging. Neuroimage 200, 528–539. doi: 10.1016/j.neuroimage.2019.06.017
Stamatakis, E. A., Shafto, M. A., Williams, G., Tam, P., and Tyler, L. K. (2011). White matter changes and word finding failures with increasing age. PLoS One 6:e14496. doi: 10.1371/journal.pone.0014496
Stevens, W. D., Wig, G. S., and Schacter, D. L. (2008). “Implicit memory and priming,” in Learning and Memory: A Comprehensive Reference, eds J. H. Byrne et al. (Oxford: Elsevier), 623–641.
Sunderland, A., Watts, K., Baddeley, A. D., and Harris, J. E. (1986). Subjective memroy assessment and test performance in elderly adults. J. Gerontol. 41, 376–384. doi: 10.1093/geronj/41.3.376
Taylor, J. K., and Burke, D. M. (2002). Asymmetric aging effects on semantic and phonological processes: naming in the picture-word interference task. Psychol. Aging 17, 662–676. doi: 10.1037//0882-7974.17.4.662
Taylor, J. R., Williams, N., Cusack, R., Auer, T., Shafto, M. A., Dixon, M., et al. (2017). The cambridge Center for ageing and neuroscience (Cam-CAN) data repository: structural and functional MRI, MEG and cognitive data from a cross-sectional adult lifespan sample. NeuroImage 144, 262–269. doi: 10.1016/j.neuroimage.2015.09.018
Unsworth, N., Fukuda, K., Awh, E., and Vogel, E. K. (2014). Working memory and fluid intelligence: capacity, attention control and secondary memory retrieval. Cogn. Psychol. 71, 1–26. doi: 10.1016/j.cogpsych.2014.01.003
Van Der Lely, H. K., and Pinker, S. (2014). The biological basis of language: insight from developmental grammatical impairments. Trends Cogn. Sci. 18, 586–595. doi: 10.1016/j.tics.2014.07.001
Verhaegen, C., and Poncelet, M. (2013). Changes in naming and semantic abilities with aging from 50 to 90 years. J. Int. Neuropsychol. Soc. 19, 119–126. doi: 10.1017/S1355617712001178
Voineskos, A. N., Rajji, T. K., Lobaugh, N. J., Miranda, D., Shenton, M. E., Kennedy, J. L., et al. (2012). Age-related decline in white matter tract integrity and cognitive performance: a DTI tractography and structural equation modeling study. Neurobiol. Aging 33, 21–34. doi: 10.1016/j.neurobiolaging.2010.02.009
Ward, E. V., Berry, C. J., and Shanks, D. R. (2013). Age effects on explicit and implicit memory. Front. Psychol. 4:639. doi: 10.3389/fpsyg.2013.00639
Waters, G., and Caplan, D. (2005). The relationship between age, processing speed, working memory capacity and language comprehension. Memory 13, 403–413. doi: 10.1080/09658210344000459
Keywords: brain age prediction, white matter, diffusion MRI, word-finding ability, domain-specific language mechanisms
Citation: Chen P-Y, Chen C-L, Tseng H-M, Hsu Y-C, Huang C-WC, Chan WP and Tseng W-YI (2021) Differential Associations of White Matter Brain Age With Language-Related Mechanisms in Word-Finding Ability Across the Adult Lifespan. Front. Aging Neurosci. 13:701565. doi: 10.3389/fnagi.2021.701565
Received: 28 April 2021; Accepted: 30 July 2021;
Published: 03 September 2021.
Edited by:
Rosalba Morese, University of Italian Switzerland, SwitzerlandReviewed by:
Pietro Spataro, Mercatorum University, ItalySneha Shashidhara, University of Cambridge, United Kingdom
Copyright © 2021 Chen, Chen, Tseng, Hsu, Huang, Chan and Tseng. This is an open-access article distributed under the terms of the Creative Commons Attribution License (CC BY). The use, distribution or reproduction in other forums is permitted, provided the original author(s) and the copyright owner(s) are credited and that the original publication in this journal is cited, in accordance with accepted academic practice. No use, distribution or reproduction is permitted which does not comply with these terms.
*Correspondence: Wen-Yih I. Tseng, wytseng@ntu.edu.tw
† These authors have contributed equally to this work and share first authorship
‡ Data used in the article were obtained from Cambridge Centre for Ageing and Neuroscience (Cam-CAN) dataset (https://www.cam-can.org/index.php?content=dataset). As such, the investigators within the Cam-CAN contributed to the design and implementation of Cam-CAN and/or provided data but did not participate in the analysis or writing of this article. A complete listing of Cam-CAN investigators is available at https://www.cam-can.org/index.php?content=people