Generative adversarial network-based attenuation correction for 99mTc-TRODAT-1 brain SPECT
- 1Biomedical Imaging Laboratory (BIG), Department of Electrical and Computer Engineering, Faculty of Science and Technology, University of Macau, Taipa, Macau SAR, China
- 2Center for Cognitive and Brain Sciences, Institute of Collaborative Innovation, University of Macau, Taipa, Macau SAR, China
- 3Department of Nuclear Medicine, Show Chwan Memorial Hospital, Lukong Town, Changhua County, Taiwan
- 4Department of Neurology, Show Chwan Memorial Hospital, Lukong Town, Changhua County, Taiwan
- 5Department of Nuclear Medicine, Chang Bing Show Chwan Memorial Hospital, Lukong Town, Changhua County, Taiwan
Background: Attenuation correction (AC) is an important correction method to improve the quantification accuracy of dopamine transporter (DAT) single photon emission computed tomography (SPECT). Chang's method was developed for AC (Chang-AC) when CT-based AC was not available, assuming uniform attenuation coefficients inside the body contour. This study aims to evaluate Chang-AC and different deep learning (DL)-based AC approaches on 99mTc-TRODAT-1 brain SPECT using clinical patient data on two different scanners.
Methods: Two hundred and sixty patients who underwent 99mTc-TRODAT-1 SPECT/CT scans from two different scanners (scanner A and scanner B) were retrospectively recruited. The ordered-subset expectation-maximization (OS-EM) method reconstructed 120 projections with dual-energy scatter correction, with or without CT-AC. We implemented a 3D conditional generative adversarial network (cGAN) for the indirect deep learning-based attenuation correction (DL-ACμ) and direct deep learning-based attenuation correction (DL-AC) methods, estimating attenuation maps (μ-maps) and attenuation-corrected SPECT images from non-attenuation-corrected (NAC) SPECT, respectively. We further applied cross-scanner training (cross-scanner indirect deep learning-based attenuation correction [cull-ACμ] and cross-scanner direct deep learning-based attenuation correction [call-AC]) and merged the datasets from two scanners for ensemble training (ensemble indirect deep learning-based attenuation correction [eDL-ACμ] and ensemble direct deep learning-based attenuation correction [eDL-AC]). The estimated μ-maps from (c/e)DL-ACμ were then used in reconstruction for AC purposes. Chang's method was also implemented for comparison. Normalized mean square error (NMSE), structural similarity index (SSIM), specific uptake ratio (SUR), and asymmetry index (%ASI) of the striatum were calculated for different AC methods.
Results: The NMSE for Chang's method, DL-ACμ, DL-AC, cDL-ACμ, cDL-AC, eDL-ACμ, and eDL-AC is 0.0406 ± 0.0445, 0.0059 ± 0.0035, 0.0099 ± 0.0066, 0.0253 ± 0.0102, 0.0369 ± 0.0124, 0.0098 ± 0.0035, and 0.0162 ± 0.0118 for scanner A and 0.0579 ± 0.0146, 0.0055 ± 0.0034, 0.0063 ± 0.0028, 0.0235 ± 0.0085, 0.0349 ± 0.0086, 0.0115 ± 0.0062, and 0.0117 ± 0.0038 for scanner B, respectively. The SUR and %ASI results for DL-ACμ are closer to CT-AC, Followed by DL-AC, eDL-ACμ, cDL-ACμ, cDL-AC, eDL-AC, Chang's method, and NAC.
Conclusion: All DL-based AC methods are superior to Chang-AC. DL-ACμ is superior to DL-AC. Scanner-specific training is superior to cross-scanner and ensemble training. DL-based AC methods are feasible and robust for 99mTc-TRODAT-1 brain SPECT.
Introduction
Dopamine transporter (DAT) single photon emission computed tomography (SPECT) is well established and widely used for Parkinson's disease (PD) diagnosis. The current PD diagnosis from DAT SPECT is mainly based on the visual assessment of the decreased striatal uptakes and the asymmetry of left and right striatum uptake for indirect measurement of DAT decrement (1). Iodine 123-radiolabeled 2β-carbomethoxy-3β-(4-iodophenyl)-N-(3-fluoropropyl) nortropane (123I-FP-CIT) (2) and 99mTc-[2[[2-[[[3-(4-chlorophenyl)-8-methyl-8-azabicyclo[3,2,1]-oct-2-yl]-methyl](2-mercaptoethyl) amino]ethyl]amino]ethane-thiolato(3-)-N2,N2′,S2,S2]oxo-[1R-(exo-exo)])) (99mTc-TRODAT-1) (3) are two common tracers for DAT SPECT, with the former more common in Western countries and the latter more common in Asia. 123I-FP-CIT is a European Medicines Agency (EMA) and U.S. Food and Drug Administration (FDA)-approved tracer to differentiate PD from essential tremor. Compared to 123I-FP-CIT, 99mTc-TRODAT-1 has a lower binding ratio of the striatum, lower thyroid uptake, and can be produced at a lower cost without a cyclotron. Though the clinical utility of CT-based attenuation correction (AC) (4) is controversial in 123I-FP-CIT SPECT (5), CT-based AC has been proven to improve SPECT image quality and quantification accuracy in DAT SPECT (6, 7). In hybrid SPECT/CT systems, CT scans can be used as attenuation maps (μ-maps) for AC in brain SPECT reconstructions. However, many existing SPECT-only systems or recently proposed dedicated brain SPECT images (8) are not integrated with CT scanners. In addition, the extra CT radiation dose in SPECT/CT poses substantial health concerns (9) and is not routinely performed in some centers. Potential mismatches between SPECT and CT images due to the involuntary and voluntary movements of PD patients can also degrade AC performance (10, 11). CT-less AC is thus of significant research and clinical impact for DAT SPECT.
Chang's AC method is a conventional CT-less AC method for brain SPECT that assumes a uniform attenuation coefficient for the volume of interest (VOI) (12). However, the assumption of a uniform μ-map would introduce estimation errors to AC, especially for bones. Deep learning (DL) methods recently emerged as a promising alternative for SPECT AC (13). Shi et al. (14) and Yang et al. (15) first performed DL-based AC on SPECT. Shi et al. (14) generated μ-maps from non-attenuation-corrected (NAC) SPECT images (indirect deep learning-based attenuation correction [DL-ACμ]) using a 3D conditional generative adversarial network (cGAN) for myocardial perfusion (MP) SPECT. Yang et al. (15) and Chen et al. (16) estimated AC MP SPECT images directly from NAC MP SPECT images (direct deep learning-based attenuation correction [DL-AC]) using different deep convolutional neural networks. Chen et al. (17) and Du et al. (18) compared the AC performance of DL-AC and DL-ACμ and demonstrated that indirect estimation of μ-maps is superior to direct estimation of AC SPECT on MP SPECT. Chen et al. (19) further investigated the feasibility of transfer learning-based AC for MP SPECT images from different scanners, tracers, and acquisition protocols. For brain SPECT, Sakaguchi et al. (20) developed a 2D convolutional neural networks (CNN)-based autoencoder for the direct generation of AC from NAC images for brain perfusion SPECT. Murata et al. (21) compared Chang's AC with a 2D autoencoder and U-Net for DL-AC for brain perfusion SPECT. Chen et al. have proposed CNN-based μ-map generation for brain perfusion SPECT (22) and 123I-FP-CIT SPECT (23) using NAC SPECT input in simulations, demonstrating improved absolute quantification accuracy. A diagram explaining DL-ACμ and DL-AC is shown in Figure 1. In this study, we implemented a conventional first-order Chang's method and a 3D cGAN for DL-AC and DL-ACμ, respectively. We then compared their performance for 99mTc-TRODAT-1 brain SPECT based on clinical data from two scanners in a single center with different acquisition protocols, field-of-view, and voxel sizes.
Materials and methods
Clinical dataset
Two hundred and sixty anonymized patients (Table 1) who underwent 99mTc-TRODAT-1 scan in two widely used clinical SPECT/CT systems (Infinia Hawkeye, GE Healthcare, Wauwatosa, WI, USA; Symbia, Siemens Healthineers, Erlangen, Germany) from two affiliated centers were retrospectively recruited under the local ethics approval (SCMH_IRB No: 1110704). Unpaired t-test on age and χ2 test on gender showed no significant difference between the training-validation dataset and the testing dataset (all ps>0.05). The acquisition protocols of the two scanners are shown in Table 2. CT was acquired for SPECT AC after the SPECT scan. The CT was manually registered to SPECT by the scanner software and converted to the μ-map using a bilinear model (4). No mismatches were observed between the CT and SPECT images in this study.
SPECT projections were reconstructed with dual-energy window scatter correction (24), with or without CT-based AC. The SPECT images with a matrix size of 64 × 64 × 64 and a voxel size of 0.4418 cm/voxel from scanner A were resampled to a matrix size of 128 × 128 × 128 and a voxel size of 0.2761 cm/voxel. We implemented the first-order Chang's method for conventional CT-less AC in brain SPECT. The uniform μ-maps were based on the NAC SPECT brain mask. An intensity threshold of 2 was used to separate the brain from the background. The masks were applied with uniform attenuation coefficients of 0.148 cm−1 (25). The 0.148 cm−1 attenuation coefficients were then converted to 0.0408 voxel−1 for scanner A and 0.0399 voxel−1 for scanner B by multiplying with a voxel size of 0.2761 cm/voxel and 0.2697 cm/voxel to get the Chang's method μ-maps, which were used in reconstruction for Chang-AC.
Conditional generative adversarial network
We implemented a 3D cGAN (18, 26) using Tensorflow on an NVIDIA GeForce RTX 3090 GPU with 24 GB RAM. An Adam optimizer was applied using an adaptive learning rate with an initial value of 0.001 and trained for up to 400 epochs. For our cGAN (Figure 2), a U-Net-based generator is trained to generate a realistic μ-map or AC SPECT, while a CNN-based discriminator is trained to differentiate between the true μ-map and the generated μ-map or between the true AC SPECT and the generated AC SPECT. The U-Net-based generator consists of encoder, bottleneck, and decoder layers. Each layer contains a 3 × 3 × 3 convolution, a batch normalization, and a leaky rectified linear unit (LeakyReLU). For encoder layers, a down-sampling layer of 2 × 2 × 2 max pooling was connected to down-sample feature maps. For bottleneck layers, a dropout layer with a 50% dropout rate was used to avoid over-fitting. For decoder layers, the up-sampling layer was used to recover the input image size. Skip connection was applied for DL-AC but not for DL-ACμ due to the large structural difference between SPECT and CT images (18, 27).
L1 loss was used to train the generator as it can enforce low-frequency correctness and encourage less blurring as compared to the commonly used L2 (28). The cross-entropy-based discriminator loss (LD) was used to train the discriminator. The discriminator loss combined with generator loss (L1) was used to train the generator.
The objective function of cGAN can be expressed as follows:
where λ is an adjustable parameter (28) [λ = 20 in this study (14)] used to control the balance between objective function VLD(G, D) and VL1(G).
Whole images instead of patches were used as training input to provide more information on the 3D patient contour (18). The patient dataset of each scanner was divided into 90, 10, and 30 for training, validation, and testing, respectively. Data augmentation by horizontal and vertical flips was used to enhance the training dataset, i.e., a total of 270 datasets were used for training. As supervised learning methods, the AC SPECT and NAC SPECT images were paired to train DL-AC, while the μ-maps and NAC SPECT images were paired to train DL-ACμ. The input NAC SPECT images for DL-ACμ were normalized to a range of [0, 1], better matching the range of attenuation coefficients from the μ-maps. Network hyperparameters were chosen based on our previous DL-based AC for MP SPECT, i.e., 3 layers and 48 feature maps for DL-ACμ and 2 layers and 48 feature maps for DL-AC (18).
To confirm the robustness of the DL-based AC methods, we applied cross-scanner training (cross-scanner indirect deep learning-based attenuation correction [cDL-ACμ] and cross-scanner direct deep learning-based attenuation correction [cDL-AC]), i.e., testing data from scanner A based on a model trained from scanner B and vice versa. We also merged the datasets from two scanners for ensemble training (ensemble indirect deep learning-based attenuation correction [eDL-ACμ] and ensemble direct deep learning-based attenuation correction [eDL-AC]). The same data augmentation technique used in scanner-specific training was applied in cross-scanner and ensemble training.
Data analysis
Normalized mean square error (NMSE) and structural similarity index (SSIM) for the whole brain were computed on the NAC, Chang's AC, and DL-based AC images as compared to the CT-AC SPECT images. The count profile across the striatum region was also drawn to measure the count distribution of different AC methods.
Specific uptake ratio (SUR) (Equation 2) of the whole striatum to the background regions and asymmetry index (%ASI) (Equation 3) of the left and right striatum were calculated based on the striatal VOIs delineated by an experienced nuclear medicine physician (Figure 3). The 2D striatal regions of interest (ROIs) were delineated slice by slice, stacking to form a 3D VOI. For the background, a 2D ROI (10 pixels × 6 pixels) in the cerebellum region was chosen, excluding ventricular regions. Bland–Altman plots were applied to SUR and %ASI results to evaluate the potential difference between different AC methods as compared to CT-AC. A paired t-test was performed on NMSE, SSIM between different DL-based methods, and on SUR and %ASI each between AC method and CT-AC. Bonferroni correction was applied for tests with multiple comparisons.
Results
Figures 4A, B shows sample Chang's μ-map, DL-ACμ, cDL-ACμ, and eDL-ACμ generated μ-maps of scanner A and corresponding error maps using CT-based μ-map as reference. The brain contours are well recovered, while the bony structures could be better restored for DL-ACμ. Figures 4C, D shows SPECT images of different AC methods and corresponding error maps using CT-AC SPECT as a reference. Three axial slices containing the highest striatum counts are displayed for comparison. All DL-based AC methods show improved image quality as compared to NAC and Chang-AC, while DL-ACμ is better than DL-AC from a visual assessment based on the error maps. The errors are increased by cross-scanner and ensemble training, while c/eDL-ACμ has fewer errors than the corresponding c/eDL-AC. Figures 5A, B shows the sample results of Chang's μ-maps, different DL-ACμ generated μ-maps, and SPECT images of different AC methods on scanner B. The results are similar to scanner A. Both DL-based AC methods are better than Chang-AC and NAC, while DL-ACμ is better than DL-AC. Though the cross-scanner and ensemble training DL-based methods show performance degradation compared to scanner-specific training, they still show fewer errors than Chang-AC. The 2D count profiles of 40 pixels across the striatum region by different AC methods for the same patients are shown in Figure 6. The profiles of all DL-based AC methods are much closer to CT-AC compared to Chang-AC and NAC, while the profile of DL-ACμ is also less deviated from CT-AC as compared to DL-AC. eDL-ACμ has errors between DL-ACμ and DL-AC, followed by cDL-ACμ, cDL-AC, and eDL-AC.
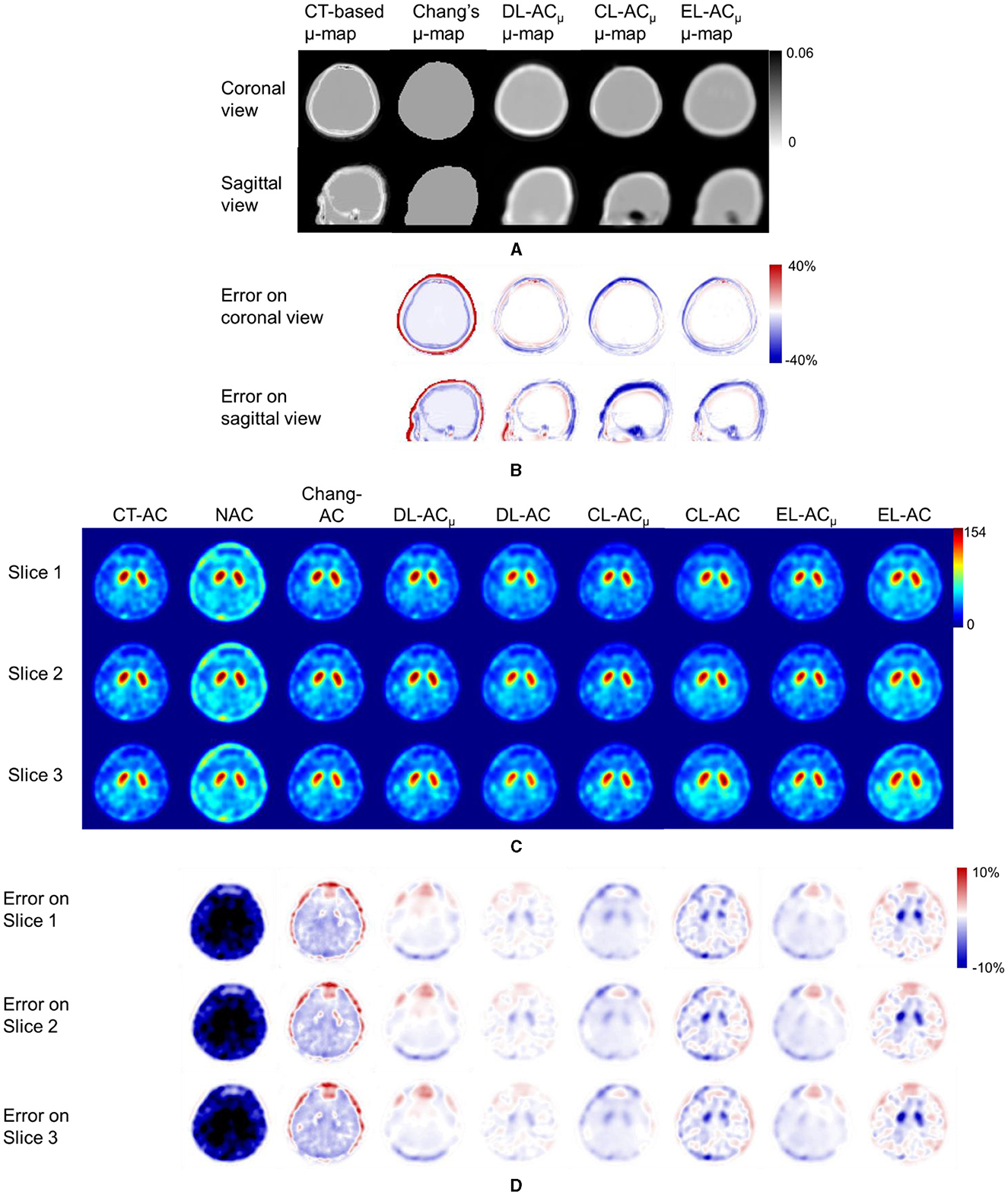
Figure 4. Representative image results of a 38-year-old male patient on scanner A. (A) Sample μ-maps generated by different methods. (B) Corresponding error maps of different μ-maps as compared to CT-based μ-map. (C) Sample axial slices with the highest striatum uptake from different AC SPECT images. (D) Corresponding error maps of different AC methods as compared to CT-AC.
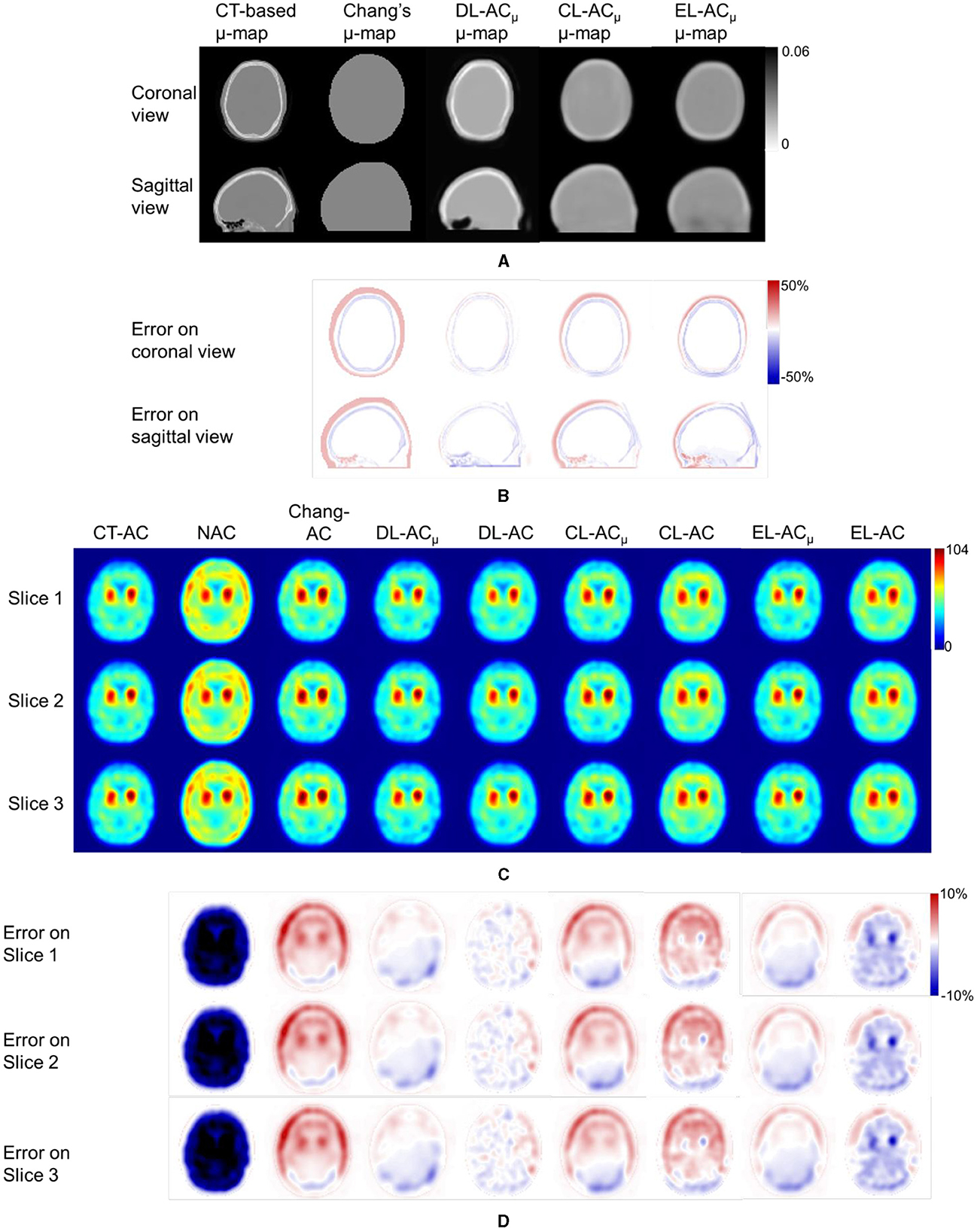
Figure 5. Representative results of a 71-year-old female patient on scanner B. (A) Sample μ-maps generated by different methods. (B) Corresponding error maps of different μ-maps as compared to CT-based μ-map. (C) Sample axial slices with the highest striatum uptake from different AC SPECT images. (D) Corresponding error maps of different AC methods as compared to CT-AC.
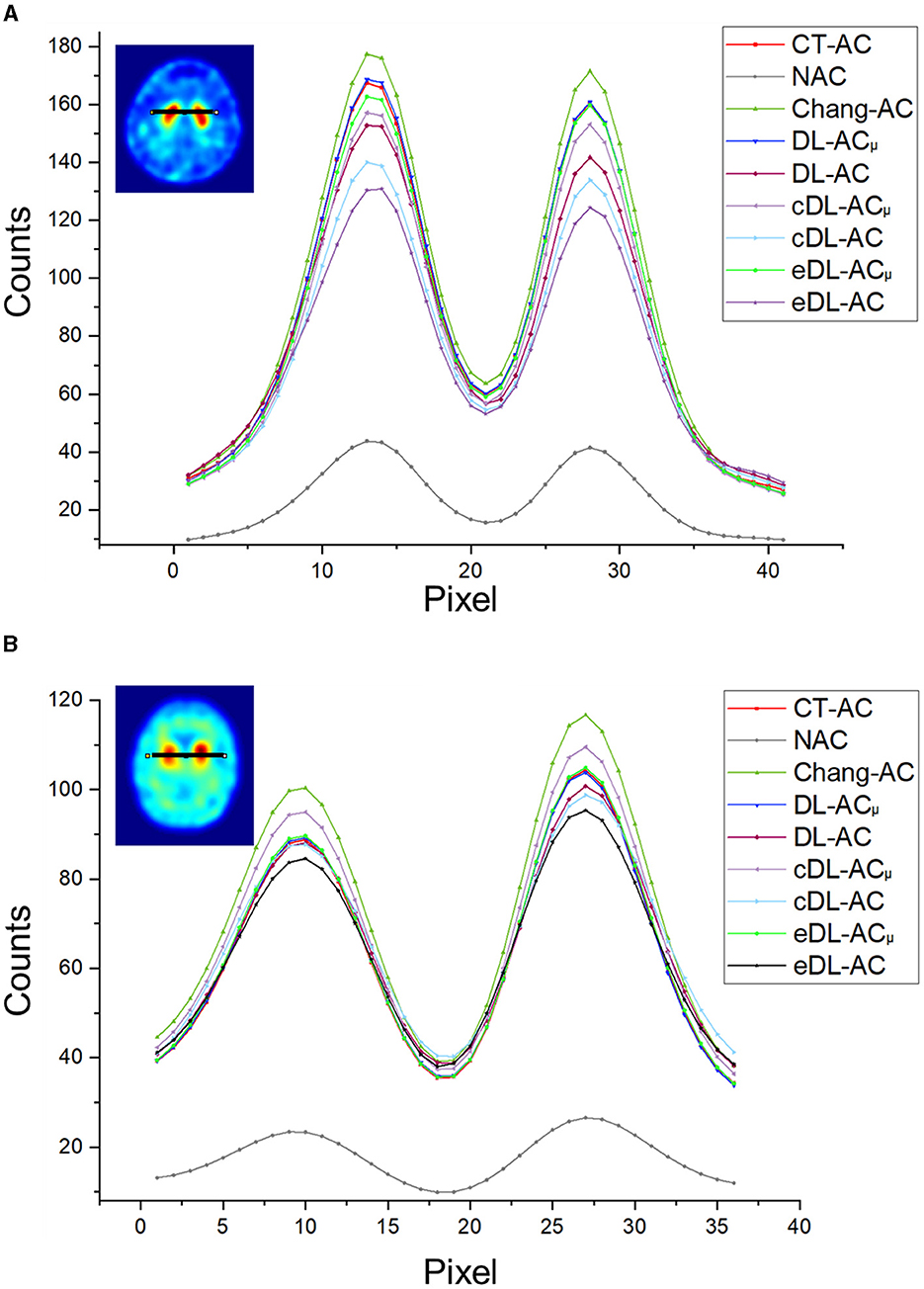
Figure 6. Count profiles of different AC methods of (A) a 38-year-old male patient on scanner A from Figure 4 and (B) a 71-year-old female patient on scanner B from Figure 5.
The NMSE and SSIM results of the two scanners are shown in Tables 3, 4. For μ-maps, DL-ACμ achieves the lowest NMSE and highest SSIM (all ps < 0.001), followed by eDL-ACμ and cDL-ACμ for both scanners. All DL-based methods have generated better μ-maps than Chang's μ-maps for both scanners. For SPECT images, the NMSE of all AC methods is lower than NAC. All DL-based AC methods are better than Chang-AC. DL-ACμ has a significantly lower NMSE than DL-AC (p < 0.05) for both scanners. The NMSE values of eDL-ACμ and cDL-ACμ are lower than eDL-AC and cDL-AC, respectively. The SSIM follows the same trend as the NMSE. The SUR and %ASI results are shown in Table 5. There is no significant difference between DL-ACμ/DL-AC and CT-AC (p > 0.05) on SUR for both scanners. There is also no significant difference between DL-ACμ/DL-AC/eDL-ACμ/cDL-ACμ and CT-AC (p > 0.05) on %ASI for both scanners. Cross-scanner and ensemble training increase the errors on SUR and %ASI scanner-specific training.
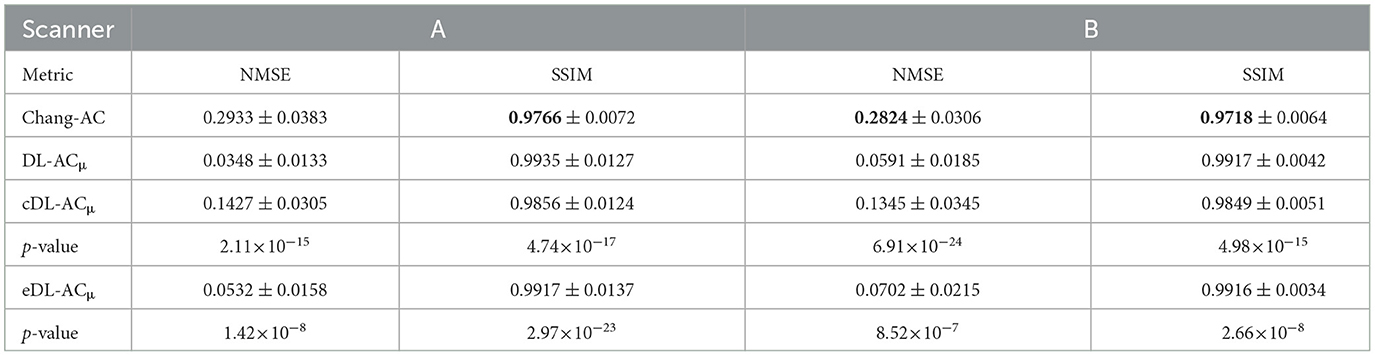
Table 3. NMSE and SSIM measurements on μ-maps generated by different methods on 30 tested patients of scanner A and 30 tested patients of scanner B.
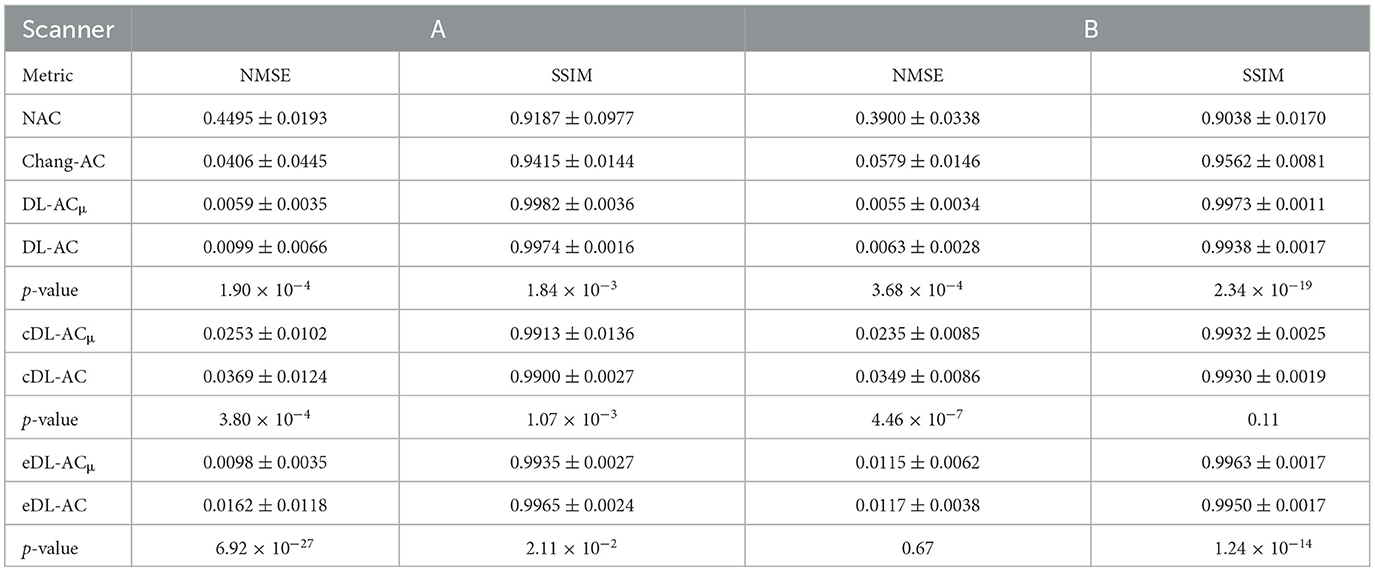
Table 4. NMSE and SSIM measurements on SPECT images with different AC methods on 30 tested patients of scanner A and 30 tested patients of scanner B.
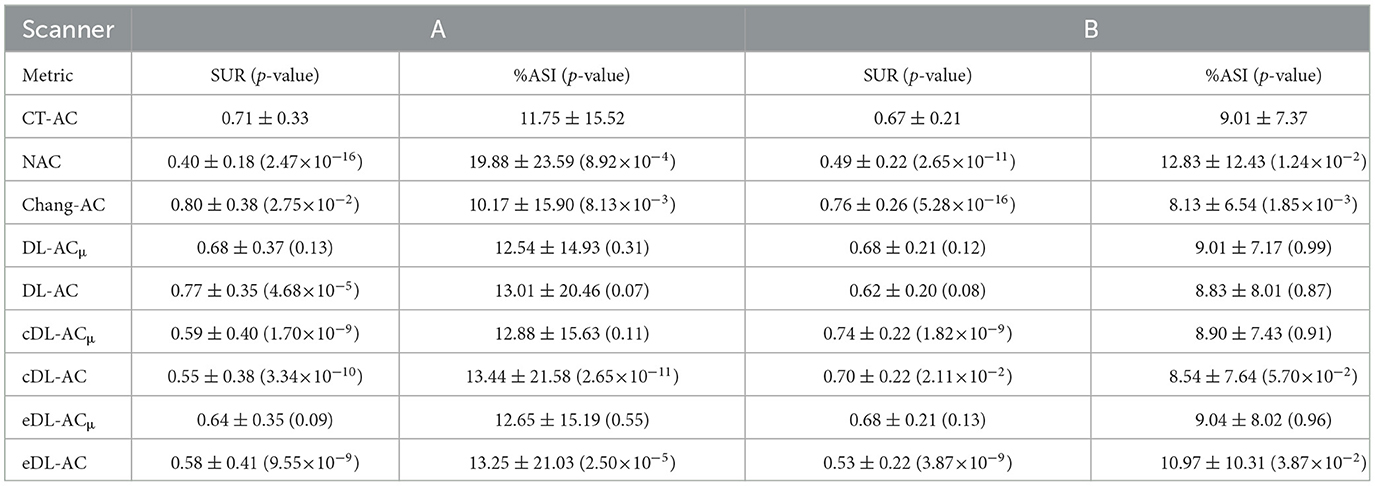
Table 5. SUR and %ASI measurements on SPECT images with different AC methods on 30 tested patients of scanner A and 30 tested patients of scanner B.
The Bland–Altman plots of SUR processed by NAC, Chang-AC, and DL-based methods using CT-AC as a reference are shown in Figure 7. NAC shows lower SUR values (mean difference of −0.3984 for scanner A and −0.1770 for scanner B) than CT-AC. Chang-AC shows an overestimated attenuation (mean difference of +0.2532 for scanner A and +0.1262 for scanner B). All DL-based AC methods have a narrower distribution than NAC and Chang-AC, except cDL-AC and eDL-AC. Both DL-ACμ and DL-AC have similar SUR values to CT-AC. DL-ACμ shows a narrower distribution compared to DL-AC with a smaller standard deviation, i.e., 95% confidence interval (CI) of [−0.0733, 0.1948] vs. [−0.1780, 0.1398] for scanner A and [−0.0291, 0.0469] vs. [−0.1424, 0.0469] for scanner B. Figure 8 shows the Bland–Altman plots of the %ASI results, which are similar to those of SUR. The 95% CI with CT-AC for NAC, Chang-AC, DL-ACμ, and DL-AC are [−53.65, 69.51], [−21.44, 20.38], [−3.798, 5.993], [−6.203, 8.186] for scanner A and [−11.59, 19.25], [−8.300, 7.009], [−3.345, 3.352], [−5.583, 4.675] for scanner B, respectively. For cross-scanner and ensemble training, the 95% CI with CT-AC for cDL-ACμ, cDL-AC, eDL-ACμ, and eDL-AC are [−16.30, 22.87], [−18.57, 24.87], [−6.203, 8.186], [−17.94, 28.38] for scanner A, and [−5.025, 8.762], [−7.441, 8.005], [−5.893, 7.168], [−9.158, 8.832] for scanner B.
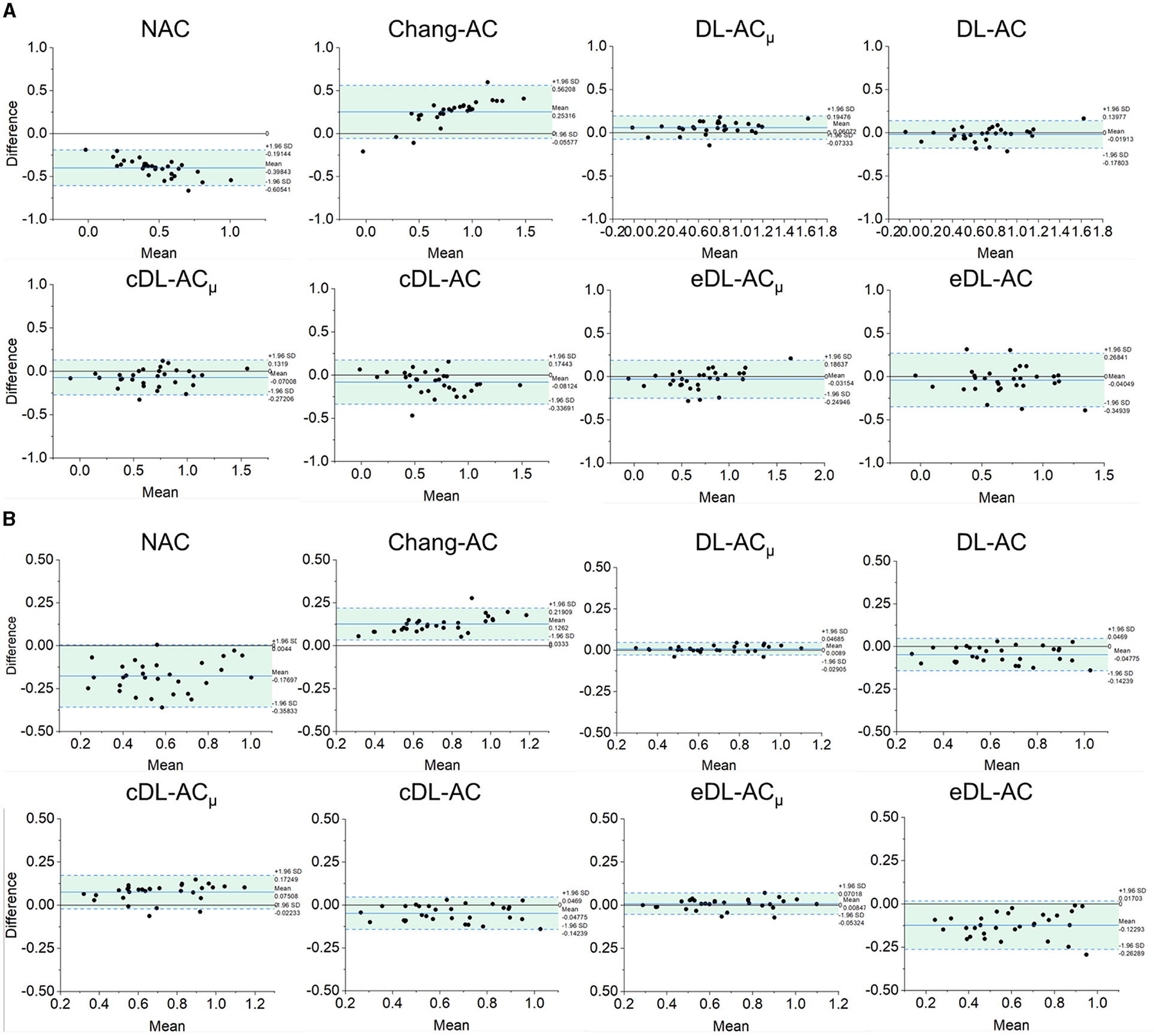
Figure 7. Bland–Altman plots of SUR results of different AC methods, using CT-AC as the reference of (A) scanner A and (B) scanner B (the dotted lines indicate 95% CI, and the blue lines indicate the mean values).
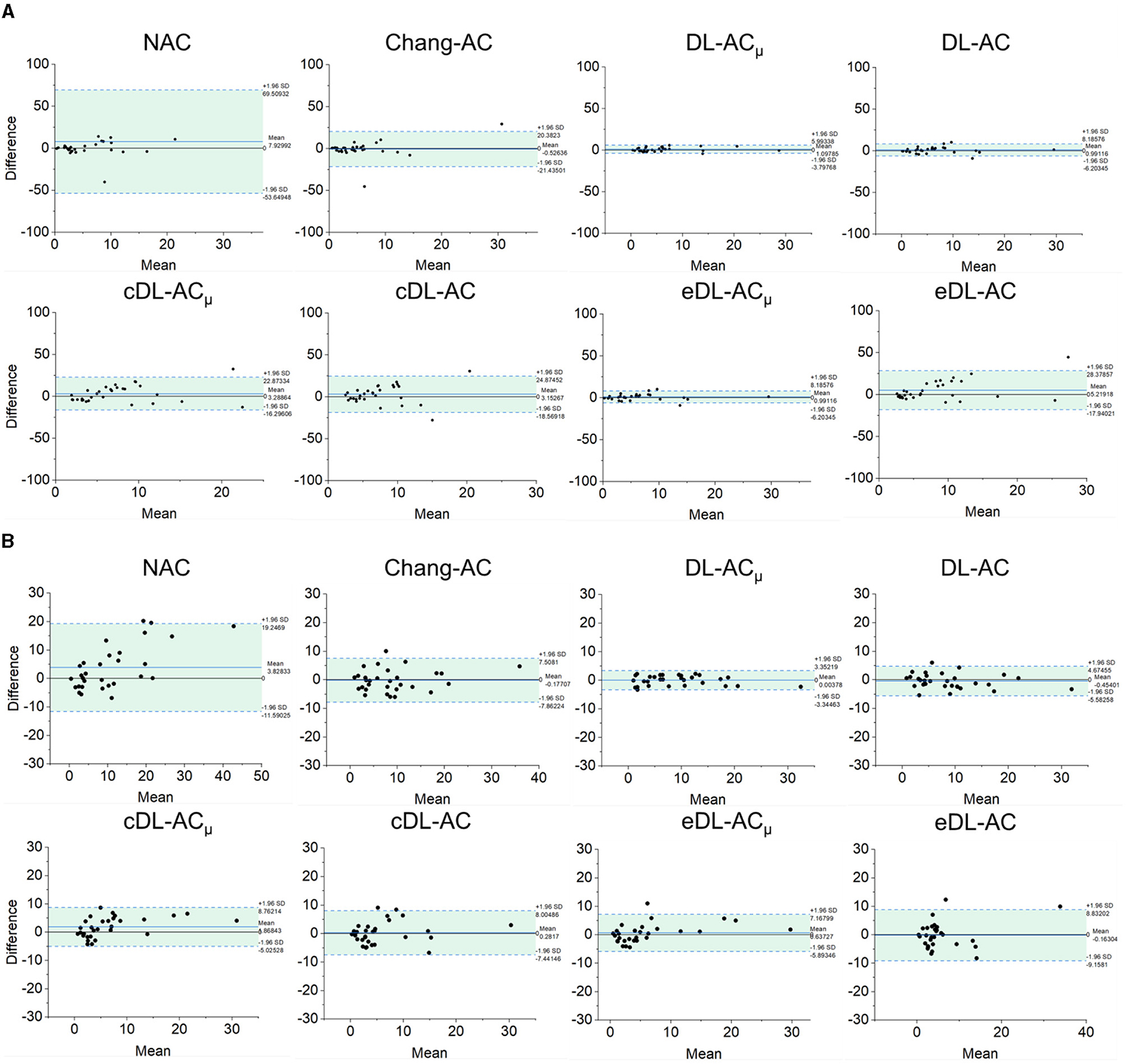
Figure 8. Bland–Altman plots of %ASI results of different AC methods, using CT-AC as the reference of (A) scanner A and (B) scanner B (the dotted lines indicate 95% CI, and the blue lines indicate the mean values).
Discussion
Our study is the first DL-based AC study using clinical 99mTc-TRODAT-1 brain SPECT data. In addition, we recruited patient data from two scanners with different acquisition protocols, field-of-view, and voxel sizes to show the robustness of DL-based AC methods on different scanners. Despite slight differences between the two scanners, e.g., brain orientation and field-of-view, spatial resolution, and CT slice thickness, their results showed similar trends for different AC methods. Murata et al. (21) demonstrate that 2D autoencoder and U-Net-based direct DL-AC are better than NAC and Chang's AC for brain perfusion SPECT. Chen et al. (23) suggest that CNN-estimated μ-map could be a promising substitute for CT-based μ-map for 123I-FP-CIT scans. Our results are consistent with theirs in that DL-based AC is better than NAC and Chang's method. We further demonstrate that the generation of μ-map is superior to the direct generation of AC SPECT for 99mTc-TRODAT-1 SPECT.
Furthermore, to the best of our knowledge, there is also no comparison of Chang's method with direct and indirect DL-based AC methods for DAT SPECT. As expected, the results show that Chang-AC has generally better image quality and quantitative results than NAC. However, the assumption of uniform attenuation coefficients over the whole brain is obviously problematic for brain SPECT, as attenuation coefficients for skull bone (0.21~0.27 cm−1), soft tissues (0.14~0.15 cm−1), and nasal cavity or mouth (~0 cm−1) are quite different based on the measurements on our data, consistent with previous measurements (4). There are also potential errors in the estimation of the brain contour on SPECT-reconstructed images in Chang's method. In this study, Chang's μ-maps are ~2–4 voxels larger than the original CT-based μ-maps in three dimensions (Figure 4). This is probably attributed to the inherent limitation of the use of thresholding method to generate Chang's μ-maps (25). A larger μ-map would lead to an overestimation of attenuation outside the skull region, i.e., the red rim in Figures 4B, 5B. Thus, DL-based methods could provide a promising alternative.
For DL-ACμ, the μ-maps can generally be estimated well for both scanners. That could be attributed to NAC SPECT images providing a rough estimate of the brain contour based on the background uptake. For all quantitative indices, all AC methods significantly improve the image quality as compared to NAC, while both DL-based methods are significantly better than Chang-AC (Figures 4–8). The use of a DL-generated μ-map for the AC purpose outperforms a direct DL-AC approach, even with cross-scanner and ensemble training, showing better robustness of indirect DL-ACμ than direct DL-AC, which is consistent with our previous MP SPECT study (18).
Although the cross-scanner and ensemble training models have better NMSE and SSIM than Chang-AC, their performance is still inferior to scanner-specific training. This could be caused by the differences between the two scanners' data, e.g., voxel size, injection dose, acquisition time, and patient positioning, leading to slightly different SPECT and CT image characteristics. Transfer learning aims to address this problem by fine-tuning the pre-trained model with a small dataset from the target scanner (19). This study is beyond the scope of this study and is ongoing in our group (29).
The SUR and %ASI are highly related to the clinical diagnosis of PD by the detection of decrement in DAT and asymmetry uptake in the left and right striatum. NAC has a much lower SUR than other AC methods, as counts are substantially attenuated toward the center of the brain, where the striatum is located. Chang-AC shows an overestimated SUR compared to CT-AC, which has been reported by previous studies (25, 30), yet it is still better than NAC. Thus, NAC may lead to a false positive, and Chang-AC may lead to a false negative diagnosis. All AC methods have better SUR and %ASI results than NAC. For DL-based methods, the SUR and %ASI values are much closer to CT-AC compared to Chang-AC except for cross-scanner-tested cDL-AC and ensemble-trained eDL-AC, indicating an improved count recovery in both the striatal region and background. DL-ACμ is the best AC method for SUR and %ASI. For (c/e)DL-ACμ, we have applied normalization to SPECT image intensity, which is not applied on (c/e)DL-AC to keep the counts invariable. Our proposed DL-based AC can improve quantitative accuracy, image quality, and clinical diagnosis accuracy of DAT SPECT, reducing the radiation dose (31, 32), the additional scan time of CT scans, and potential mismatches concern between SPECT and CT images for AC and providing a CT-less AC option for SPECT without integrated CT.
There are certain limitations to this study. Involuntary head motion is commonly observed in patients with neurodegenerative disease (33). It may lead to a mismatch between SPECT and CT data (34–36), which could be reduced by registration (37) and motion-tracking methods (38). Previously, we showed that this mismatch compromises DL-based AC performance, but it is still better than NAC in MP SPECT (18). We expect a similar result for DL-based AC in DAT SPECT.
Conclusion
This is the first clinical evaluation of DL-based AC methods for DAT SPECT. Both DL-based methods improve image quality and quantitative accuracy as compared to Chang-AC and NAC. DL-ACμ is consistently better than DL-AC on clinical patient data on two scanners with different acquisition protocols and post-processing parameters.
New knowledge gained
Deep learning-based attenuation correction (AC) is feasible for DAT SPECT. Indirect generation of attenuation maps is better and more robust than direct generation of attenuation-corrected SPECT images from non-attenuation-corrected SPECT images and Chang's AC for DAT SPECT.
Data availability statement
Patient data are not allowed to be shared with the public due to patient confidentially. Requests to access the datasets should be directed to P-YC (paiyibox@gmail.com) and G-UH (106143@gmail.com).
Ethics statement
The studies involving human participants were reviewed and approved by Institutional Review Board of Chang Bing Show Chwan Memorial Hospital (IRB number: 1110704). The written consent was waived due to the retrospective nature of this study.
Author contributions
YD and GM were the primary authors of the manuscript. YD was mainly responsible for data processing and analysis. HJ, ZP, and JS were mainly responsible for data analysis and manuscript revision. C-NL was mainly responsible for data collection. HJ, C-NL, P-YC, and G-UH were responsible for clinical interpretation. GM and G-UH were responsible for data interpretation and study integration. All authors contributed to the article and approved the submitted version.
Funding
This study received an External Industrial Research Collaboration Grant (EF062/FST/MSP/2023/ZJFCYLKJ) from Zhejiang Fuchuan Medical Technology Co., Ltd. The funder was not involved in the study design, collection, analysis, interpretation of data, the writing of this article, or the decision to submit it for publication. This study was also supported by a research grant from Show Chwan Memorial Hospital (IRD-110015).
Conflict of interest
The authors declare that the research was conducted in the absence of any commercial or financial relationships that could be construed as a potential conflict of interest.
Publisher's note
All claims expressed in this article are solely those of the authors and do not necessarily represent those of their affiliated organizations, or those of the publisher, the editors and the reviewers. Any product that may be evaluated in this article, or claim that may be made by its manufacturer, is not guaranteed or endorsed by the publisher.
Abbreviations
SPECT, single photon emission computed tomography; DAT, dopamine transporter; 123I-FP-CIT, Iodine 123-radiolabeled 2β-carbomethoxy-3β-(4-iodophenyl)-N-(3-fluoropropyl) nortropane; 99mTc-TRODAT-1, 99mTc-[2[[2-[[[3-(4-chlorophenyl)-8-methyl-8-azabicyclo[3,2,1]-oct-2-yl]-methyl](2-mercaptoethyl)amino]ethyl]amino]ethane-thiolato(3-)-N2,N2′,S2,S2]oxo-[1R-(exoexo)]); AC, attenuation correction; μ-map, attenuation map; DL, deep learning; cGAN, conditional generative adversarial network; CNN, convolutional neural networks; OS-EM, ordered-subset expectation-maximization; DL-ACμ, indirect deep learning-based attenuation correction; DL-AC, direct deep learning-based attenuation correction; cDL-ACμ, cross-scanner indirect deep learning-based attenuation correction; cDL-AC, cross-scanner direct deep learning-based attenuation correction; eDL-ACμ, ensemble indirect deep learning-based attenuation correction; eDL-AC, ensemble direct deep learning-based attenuation correction; NMSE, normalized mean square error; SSIM, structural similarity index; SUR, specific uptake ratio; %ASI, asymmetry index.
References
1. Suwijn SR, van Boheemen CJM, de Haan RJ, Tissingh G, Booij J, de Bie RMA. The diagnostic accuracy of dopamine transporter SPECT imaging to detect nigrostriatal cell loss in patients with Parkinson's disease or clinically uncertain parkinsonism: a systematic review. EJNMMI Res. (2015) 5:12. doi: 10.1186/s13550-015-0087-1
2. Booij J, Tissingh G, Boer GJ, Speelman JD, Stoof JC, Janssen AG, et al. [123I]FP-CIT SPECT shows a pronounced decline of striatal dopamine transporter labelling in early and advanced Parkinson's disease. J Neurol Neurosurg Psychiatry. (1997) 62:133–40. doi: 10.1136/jnnp.62.2.133
3. Kung HF, Kim H-J, Kung M-P, Meegalla SK, Plössl K, Lee H-K. Imaging of dopamine transporters in humans with technetium-99m TRODAT 1. Eur J Nucl Med. (1996) 23:1527–30. doi: 10.1007/BF01254479
4. Patton JA, Turkington TG. SPECT/CT physical principles and attenuation correction. J Nucl Med Technol. (2008) 36:1–10. doi: 10.2967/jnmt.107.046839
5. Lapa C, Spehl TS, Brumberg J, Isaias IU, Schlögl S, Lassmann M, et al. Influence of CT-based attenuation correction on dopamine transporter SPECT with [(123)I]FP-CIT. Am J Nucl Med Mol Imaging. (2015) 5:278–86.
6. Bieńkiewicz M, Górska-Chrzastek M, Siennicki J, Gajos A, Bogucki A, Mochecka-Thoelke A, et al. Impact of CT based attenuation correction on quantitative assessment of DaTSCAN (123 I-Ioflupane) imaging in diagnosis of extrapyramidal diseases. Nuclear Medicine Review. (2008) 11:53–8.
7. Rajeevan N, Zubal IG, Ramsby SQ, Zoghbi SS, Seibyl J, Innis RB. Significance of nonuniform attenuation correction in quantitative brain SPECT imaging. Journal of Nuclear Medicine. (1998) 39:1719–26.
8. Stam MK, Verwer EE, Booij J, Adriaanse SM, de Bruin CM, de Wit TC. Performance evaluation of a novel brain-dedicated SPECT system. EJNMMI Physics. (2018) 5:4. doi: 10.1186/s40658-018-0203-1
9. Rausch I, Füchsel FG, Kuderer C, Hentschel M, Beyer T. Radiation exposure levels of routine SPECT/CT imaging protocols. Eur J Radiol. (2016) 85:1627–36. doi: 10.1016/j.ejrad.2016.06.022
10. Zhang D, Ghaly M, Mok GS. Interpolated CT for attenuation correction on respiratory gating cardiac SPECT/CT—a simulation study. Med Phys. (2019) 46:2621–8. doi: 10.1002/mp.13513
11. Zhang D, Yang B-H, Wu NY, Mok GSP. Respiratory average CT for attenuation correction in myocardial perfusion SPECT/CT. Ann Nucl Med. (2017) 31:172–80. doi: 10.1007/s12149-016-1144-1
12. Chang L-T, A. method for attenuation correction in radionuclide computed tomography. IEEE Trans Nucl Sci. (1978) 25:638–43. doi: 10.1109/TNS.1978.4329385
13. Chen X, Liu C. Deep-learning-based methods of attenuation correction for SPECT and PET. J Nucl Cardiol. (2022). doi: 10.1007/s12350-022-03007-3
14. Shi L, Onofrey JA, Liu H, Liu Y-H, Liu C. Deep learning-based attenuation map generation for myocardial perfusion SPECT. Eur J Nuclear Med Mol Imag. (2020) 47:10. doi: 10.1007/s00259-020-04746-6
15. Yang J, Shi L, Wang R, Miller EJ, Sinusas AJ, Liu C, et al. Direct attenuation correction using deep learning for cardiac SPECT: a feasibility study. J Nucl Med. (2021) 62:1645–52. doi: 10.2967/jnumed.120.256396
16. Chen X, Zhou B, Shi L, Liu H, Pang Y, Wang R, et al. CT-free attenuation correction for dedicated cardiac SPECT using a 3D dual squeeze-and-excitation residual dense network. J Nucl Cardiol. (2021). doi: 10.1007/s12350-021-02672-0
17. Chen X, Zhou B, Xie H, Shi L, Liu H, Holler W, et al. Direct and indirect strategies of deep-learning-based attenuation correction for general purpose and dedicated cardiac SPECT. Eur J Nucl Med Mol Imaging. (2022) 49:3046–60. doi: 10.1007/s00259-022-05718-8
18. Du Y, Shang J, Sun J, Wang L, Liu Y-H, Xu H, et al. Deep-learning-based estimation of attenuation map improves attenuation correction performance over direct attenuation estimation for myocardial perfusion SPECT. J Nucl Cardiol. (2022) 2022:1–16. doi: 10.1007/s12350-022-03092-4
19. Chen X, Hendrik Pretorius P, Zhou B, Liu H, Johnson K, Liu Y-H, et al. Cross-vender, cross-tracer, and cross-protocol deep transfer learning for attenuation map generation of cardiac SPECT. J Nucl Cardiol. (2022) 2022:1–13. doi: 10.1007/s12350-022-02978-7
20. Sakaguchi K, Kaida H, Yoshida S, Ishii K. Attenuation correction using deep learning for brain perfusion SPECT images. Ann Nucl Med. (2021) 35:589–99. doi: 10.1007/s12149-021-01600-z
21. Murata T, Yokota H, Yamato R, Horikoshi T, Tsuneda M, Kurosawa R, et al. Development of attenuation correction methods using deep learning in brain-perfusion single-photon emission computed tomography. Med Phys. (2021) 48:4177–90. doi: 10.1002/mp.15016
22. Chen Y, Goorden MC, Beekman FJ. Automatic attenuation map estimation from SPECT data only for brain perfusion scans using convolutional neural networks. Phys Med Biol. (2021) 66:065006. doi: 10.1088/1361-6560/abe557
23. Chen Y, Goorden MC, Beekman FJ. Convolutional neural network based attenuation correction for 123I-FP-CIT SPECT with focused striatum imaging. Phys Med Biol. (2021) 66:195007. doi: 10.1088/1361-6560/ac2470
24. Smith M, Jaszczaki R. Generalized dual-energy-window scatter compensation in spatially varying media for SPECT. Phys Med Biol. (1994) 39:531. doi: 10.1088/0031-9155/39/3/016
25. Lange C, Seese A, Schwarzenböck S, Steinhoff K, Umland-Seidler B, Krause BJ, et al. CT-based attenuation correction in I-123-ioflupane SPECT. PLoS ONE. (2014) 9:e108328. doi: 10.1371/journal.pone.0108328
27. Decuyper M, Maebe J, Van Holen R, Vandenberghe S. Artificial intelligence with deep learning in nuclear medicine and radiology. EJNMMI Physics. (2021) 8:81. doi: 10.1186/s40658-021-00426-y
28. Isola P, Zhu J-Y, Zhou T, Efros AA. (2017). “Image-to-image translation with conditional adversarial networks,” in Proceeding of the IEEE Conference on Computer Vision and Pattern Recognition (Hawaii, HI: IEEE). doi: 10.1109/CVPR.2017.632
29. Sun H, Du Y, Lu L, Lin C-N, Hung G-U, Chiu P-Y, et al. (2023). “Cross-tracer and cross-scanner transfer learning based attenuation map generation for brain SPECT,” in Abstract presented at Society of Nuclear Medicine and Molecular Imaging 2023 Annual Meeting (Chicago, IL).
30. Maltais DD, Jordan LG, Min H-K, Miyagawa T, Przybelski SA, Lesnick TG, et al. Confirmation of 123I-FP-CIT SPECT quantification methods in dementia with lewy bodies and other neurodegenerative disorders. J Nucl Med. (2020) 61:1628–35. doi: 10.2967/jnumed.119.239418
31. Law W-Y, Yang C-C, Chen L-K, Huang T-C, Lu K-M, Wu T-H, et al. Retrospective gating vs. prospective triggering for noninvasive coronary angiography: assessment of image quality and radiation dose using a 256-slice CT scanner with 270 ms gantry rotation. Academic Radiol. (2011) 18:31–9. doi: 10.1016/j.acra.2010.07.013
32. Lee Y-W, Yang C-C, Mok GS, Wu T-H. Infant cardiac CT angiography with 64-slice and 256-slice CT: comparison of radiation dose and image quality using a pediatric phantom. PLoS ONE. (2012) 7:e49609. doi: 10.1371/journal.pone.0049609
33. Tahmasian M, Bettray LM, van Eimeren T, Drzezga A, Timmermann L, Eickhoff CR, et al. A systematic review on the applications of resting-state fMRI in Parkinson's disease: does dopamine replacement therapy play a role? Cortex. (2015) 73:80–105. doi: 10.1016/j.cortex.2015.08.005
34. Lu Z, Chen G, Lyu Y, Chen Y, Mok GSP. Technical note: Respiratory impacts on static and respiratory gated (99m) Tc-MAA SPECT/CT for liver radioembolization: a simulation study. Med Phys. (2022) 49:5330–9. doi: 10.1002/mp.15682
35. Lyu Y, Chen G, Lu Z, Chen Y, Mok GSP. The effects of mismatch between SPECT and CT images on quantitative activity estimation—a simulation study. Z Med Phys. (2023) 33:54–69. doi: 10.1016/j.zemedi.2022.03.004
36. Lu Z, Chen G, Lin KH, Wu TH, Mok GSP. Evaluation of different CT maps for attenuation correction and segmentation in static (99m) Tc-MAA SPECT/CT for (90) Y radioembolization treatment planning: a simulation study. Med Phys. (2021) 48:3842–51. doi: 10.1002/mp.14991
37. Lu Z, Chen G, Jiang H, Sun J, Lin KH, Mok GSP, et al. and CT misregistration reduction in [(99m)Tc]Tc-MAA SPECT/CT for precision liver radioembolization treatment planning. Eur J Nucl Med Mol Imaging. (2023) 50:1–2. doi: 10.1007/s00259-023-06149-9
Keywords: deep learning, generative adversarial network, attenuation correction, dopamine transporter SPECT, 99mTc-TRODAT-1
Citation: Du Y, Jiang H, Lin C-N, Peng Z, Sun J, Chiu P-Y, Hung G-U and Mok GSP (2023) Generative adversarial network-based attenuation correction for 99mTc-TRODAT-1 brain SPECT. Front. Med. 10:1171118. doi: 10.3389/fmed.2023.1171118
Received: 21 February 2023; Accepted: 17 July 2023;
Published: 15 August 2023.
Edited by:
Giorgio Treglia, Ente Ospedaliero Cantonale (EOC), SwitzerlandReviewed by:
Carmelo Caldarella, Fondazione Policlinico Universitario A. Gemelli IRCCS, ItalyHamid Sabet, Massachusetts General Hospital and Harvard Medical School, United States
Copyright © 2023 Du, Jiang, Lin, Peng, Sun, Chiu, Hung and Mok. This is an open-access article distributed under the terms of the Creative Commons Attribution License (CC BY). The use, distribution or reproduction in other forums is permitted, provided the original author(s) and the copyright owner(s) are credited and that the original publication in this journal is cited, in accordance with accepted academic practice. No use, distribution or reproduction is permitted which does not comply with these terms.
*Correspondence: Pai-Yi Chiu, paiyibox@gmail.com; Guang-Uei Hung, 106143@gmail.com; Greta S. P. Mok, gretamok@um.edu.mo
†These authors share senior authorship