- 1Department of Cardiology, Shaanxi Provincial People’s Hospital, Xi’an, China
- 2Department of General Internal Medicine, Xi’an Jiaotong University Hospital, Xi’an, China
- 3Shaanxi Provincial Key Laboratory of Infection and Immune Diseases, Shaanxi Provincial People’s Hospital, Xi’an, China
Background: Epidemiological studies have linked various circulating cytokines to cardiovascular disease (CVD), which however remains uncertain whether these relationships represent causality or are due to bias. To address this question, we conducted a Mendelian randomization (MR) analysis to systematically investigate the causal effects of circulating cytokine levels on CVD development.
Methods: This study leveraged the summary statistic from respective genome-wide association study (GWAS) of 47 cytokines and four types of CVD. The cis-quantitative trait locus (cis-QTL) definition, derived from a GWAS meta-analysis comprising 31,112 participants of European descent, served as instruments for cytokines. A two-sample MR design was employed, followed by comprehensive sensitivity analyses to validate the robustness of results.
Results: The results of inverse-variance weighted method using cis-protein QTL (cis-pQTL) instruments, showed the causal effects of four cytokines (i.e., IL-1ra, MCSF, SeSelectin, SCF) on the risk of coronary artery disease (CAD). We also identified causal relationships between two cytokines (i.e., IL-2ra, IP-10) and heart failure (HF), as well as two cytokines (i.e., MCP-3, SeSelectin) and atrial fibrillation (AF), after controlling for false discovery rate (FDR). The use of cis-expression QTL (cis-eQTL) revealed additional causal associations between IL-1a, MIF and CAD, between IL-6, MIF, and HF, as well as between FGFBasic and AF. No significant sign was survived for stroke with FDR applied. Results were largely consistent across sensitivity analyses.
Conclusion: The present study provides supportive evidence that genetic predisposition to levels of certain cytokines causally affects the development of specific type of CVD. These findings have important implications for the creation of novel therapeutic strategies targeting these cytokines as a means of preventing and treating CVD.
Introduction
Cardiovascular disease (CVD), a cluster of disorders that impact the heart and/or blood vessels, is a foremost cause of death and disability worldwide. In 2019, it is estimated that 17.9 million deaths were attributed to CVD, ranking the first leading cause of all global death (1). CVD has a complex etiology and often develops over decades before an obvious symptomatic event. Early intervention is vital to reduce morbidity and mortality from CVD, which would produce a far-reaching influence on the public health burden. Therefore, improved understanding of the causal effect of different risk factors, especially at the microscale and molecular levels, can refine prevention strategies and enable novel targets for therapeutic intervention in CVD.
Cytokines act a crucial part in regulating the inflammatory response, altering vasoconstriction and impeding endothelium-dependent vasodilation, and therefore, they may offer potential targets for preventing CVD (2). Extensive epidemiological evidence has documented strong associations between cytokines and CVD. For instance, a meta-analysis comprising 29 cohort studies demonstrated that several cytokines, such as interleukin-6 (IL-6), IL-18, and tumour necrosis factor alpha (TNF-α), each were associated with the risk of developing coronary artery disease (CAD), in an approximately log-linear manner, independent of traditional risk factors (3). Another study involving 17,180 individuals found the positive relationship of circulating levels of monocyte chemoattractant protein-1 (MCP-1) with long-term risk of stroke (4). However, classical observational designs are prone to reverse causation and confounding that hinder causal inference, and conducting clinical trials on cytokine interventions are challenging.
Mendelian randomization (MR) is a robust technology that can address the limitations accompanying observational studies mentioned above and provides the highest level of evidence hierarchy other than randomized controlled trials by leveraging genetic variants as instrumental variables (IVs) (5). This method, when certain assumptions are satisfied, could determine causality of a given exposure-outcome association. Indeed, two successive MR analyses have helped identify the causal effect of IL-6 on the development of CAD and total CVD, indicating that IL-6 blockade may serve as a novel therapeutic target (6, 7). And the extent of this benefit may be directly proportional to the degree of reduction in levels of high-sensitivity C-reactive protein (hsCRP) (6, 7). Additionally, several MR studies have also explored the causal impact of circulating cytokines on stroke (8, 9). However, the most of existing efforts were focused on one particular type of CVD or a single pro-inflammatory cytokine, leaving other cardiovascular events such as heart failure (HF) and atrial fibrillation (AF) less explored. Herein, we applied a two-sample MR framework to systematically ascertain whether there was a causality between a broad range of cytokines and the risk of CVD which encompasses CAD, HF, AF, and stroke.
Methods
Study design
The flowchart of the current work is outlined in Figure 1. To begin, we selected genetic variants as IVs for circulating cytokines. Next, latest summary statistics from the corresponding genome-wide association studies (GWASs) for CAD, HF, AF, and stroke were collected. Then, we performed a two-sample MR study with inverse-variance weighted (IVW) method as our primary analysis. Finally, a series of sensitivity analyses were followed, including MR-Egger regression, weighted-median, contamination mixture (ConMix), and MR-Pleiotropy Residual Sum and Outlier (MR-PRESSO).
Data sources and instrumental variable selection
The present study relies on publicly available summary statistics from published studies, and therefore no additional ethical approval from the institutional review board was required. The characteristics of data used in this study are shown in Table 1.
For the cytokines as exposures, we acquired the IVs from the most up-to-date GWAS meta-analysis of three independent sources (10) [i.e., the SCALLOP consortium (11), the INTERVAL study (12), and a the Northern Finland Birth Cohort 1966 (13)], which include a total of 31,112 subjects of European ancestry. Details on the methods of SNP selection and meta-analysis can be found in the original paper (10).
For the outcomes, the summary-level data for CAD (122,733 cases and 424,528 controls), HF (47,309 cases and 930,014 controls), AF (60,620 cases and 970,216 controls), and stroke (40,585 cases and 40,6111 controls) were derived from CARDIoGRAMplusC4D Consortium (14), HERMES Consortium (15), a GWAS conducted by Nielsen et al. (16), and MEGASTROKE Consortium (17), respectively. These study populations were predominantly of European descent. There is no obvious sample overlap between the GWAS meta-analyses of cytokines and four investigated cardiovascular diseases.
MR relies on three IV assumptions to ensure the accuracy of causal inferences (1): IVs must truly be associated with exposures (2), independent of confounders, and (3) affect outcomes solely through exposures, not through any other pathways. To better ensure that the selected IVs satisfy the above three assumptions, especially for the first and the third, we here used cis quantitative trait locus (QTLs) as IVs to enhance instrument strength, including cis-protein QTLs (cis-pQTLs, gene range ± 500kb) and cis-expression QTLs (cis-eQTLs, gene range ± 500kb). These cis-QTLs, located at or close to the gene of origin, naturally have a stronger correlation with the gene expression and thereafter protein concentrations than other variants. For our main analysis, we used cis-pQTLs as the IVs, and for complementary analysis, we used cis-eQTLs since cis-eQTLs may capture the effects of pQTLs through gene expression, although not all pQTLs are represented by eQTLs (18). Specifically, cis-pQTLs and cis-eQTLs were associated with the circulating cytokine levels and their gene expression aggregated across tissues, respectively, both met a significant threshold of 1E-4. Of note, to better balance the number and strength of instrumental variables and obtain potentially informative results, a relatively loose threshold of 1E-4 was selected as an alternative to the 5E-8 threshold used in traditional GWAS. We excluded palindromic variants with a minor allele frequency (MAF) greater than 0.05 and performed clumping by setting a pairwise linkage disequilibrium (LD) cutoff of r2 < 0.1. The alleles of the QTLs were harmonized between the exposure and the outcome to ensure proper alignment of effects. Detailed information on the characteristics of the QTLs used as IVs can be found in the Supplementary Tables 1, 2.
Statistical analyses
We incorporated separate analyses using two different sets of IVs (cis-pQTL and cis-eQTL) to assess the links between genetically predicted circulating cytokine levels and the risk of each CVD outcome. For cases where there exists only one single QTL, the classic Wald ratio was adopted to gain MR estimates. Otherwise, the random-effects IVW model using a meta-analysis approach was performed to combine the Wald ratios of multiple QTLs to obtain overall MR estimates (19). IVW is generally considered to provide unbiased estimates of the causal effect of the exposure on the outcome, provided that the three assumptions mentioned above are met. Furthermore, we conducted sensitivity analyses using four pleiotropy- robust methods, namely MR-Egger regression (20), weighted-median method (21), ConMix (22), and MR-PRESSO (23). Different methods could yield valid results under different model assumptions with the criterion relaxed to some extent. The weighted-median estimator is capable of providing a valid estimation even when 50% IVs are invalid. The MR-Egger regression is sensitive to the presence of horizontal pleiotropy across IVs, but it depends on the Instrument Strength Independent of Direct Effect (InSIDE) assumption, where the genetic variant used as an instrument affects the outcome only through the exposure of interest and not through any other pathway that could confound the association between the exposure and the outcome. The method ConMix could estimate the causal effect with implicitly distinguishing valid and invalid IVs using an underlying mixture model. MR-PRESSO can obtain consistent causal estimates by horizontal pleiotropic outlier (if it is noted) removal. These methods rely on different assumptions to each other which are difficult to prove, and if the results from all of these different analyses are largely consistent, then the investigator can be more confident in drawing conclusions regarding causality. Moreover, the Cochran’s Q test in the IVW and the intercept from MR-Egger method were deployed to test the heterogeneity and horizontal pleiotropy, with a P-value < 0.05, respectively. The original paper provided information on the proportion of variance (R2) that each cytokine has explained by the QTLs and F-statistics that quantified the strength of the IVs (10). We further calculated the cumulative F-statistics and an F-statistic > 10 was considered to avoid weak instrument bias (24). The statistical power of our entire MR analyses was estimated utilizing the non-centrality parameter-based approach proposed by Brion et al. on the online tool (https://shiny.cnsgenomics.com/mRnd/) (25). Lastly, correlation analysis was done to illustrate the mutual corroboration or complementary of results under two instruments strategies.
All statistical analyses were implemented using the “TwoSampleMR” (version 0.5.6), “MendelianRandomization” (version 0.6.0), and “MR-PRESSO” (version 1.0) packages in R (version 4.1.2). To address the issue of multiple comparisons for the numerous cytokines, we performed the stratified false discovery rate (FDR) approach using Benjamini-Hochberg procedure for IVW analysis, i.e., estimating adjusted P-value separately for each CVD outcome (26). Additionally, we also utilized the aggregated FDR correction to complement our results. The statistical significance was defined using a threshold of adjusted P-value < 10%.
Results
To unravel the causal effect of all analyzable cytokines (shown in Table 2) on the risk of four types of CVD (i.e., CAD, HF, AF, stroke), two-sample MR tests were carried out. Of 35 cytokines analyzed, 31 cytokines possessed cis-pQTL instruments, explaining 0.1% to 28.9% of the phenotypic variance, and 27 cytokines possessed cis-eQTL instruments, explaining 0.04% to 13.0% of the phenotypic variance. Cumulative F-statistics of cis-pQTL for 29 out of 31 cytokines were greater than 10, proving the good strength of genetic instruments (Table 2). Detailed MR results for the causal relationship of interests are shown in Supplementary Table 3 (based on the cis-pQTL instruments) and Supplementary Table 4 (based on the cis-eQTL instruments). The visualization of all IVW results is presented in Figure 2 and only significant IVW results (stratified FDR < 10%) with sensitivity analyses are shown in Figure 3. Furthermore, the results of the two correction strategies (stratified FDR vs. aggregated FDR) did not differ significantly.
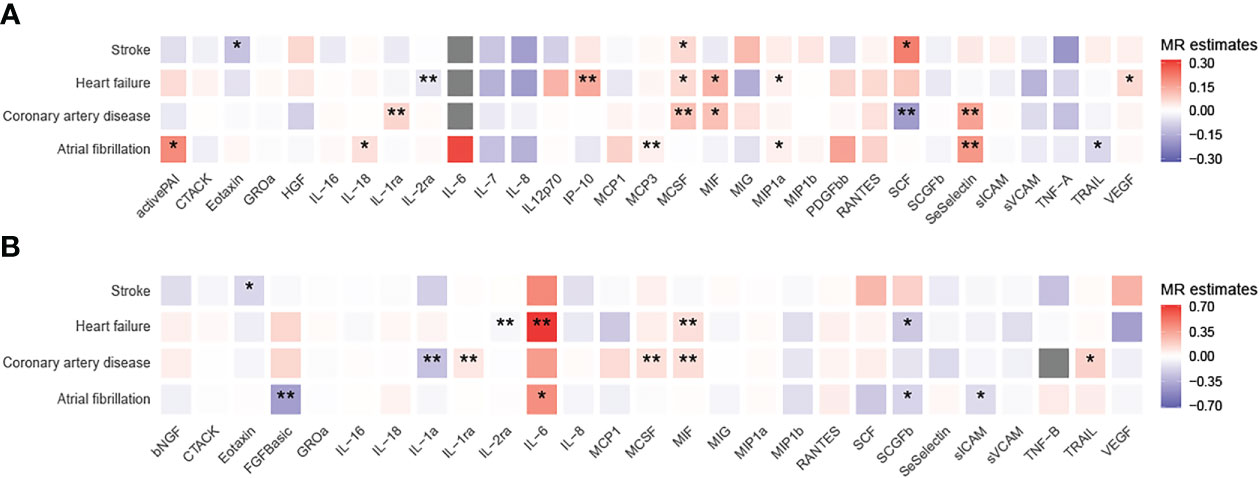
Figure 2 Heatmaps of the causal associations between cytokines and cardiovascular diseases. Shown are the results of the IVW method based on the (A) cis-pQTL and (B) cis-eQTL instruments. Red boxes indicate positive associations, blue boxes negative associations, and grey boxes associations for which no instrument was available. One asterisk denotes that the associations is nominally significant (P < 0.05). Two asterisks denote that the association withstands multiple comparison correction (stratified FDR < 10%).
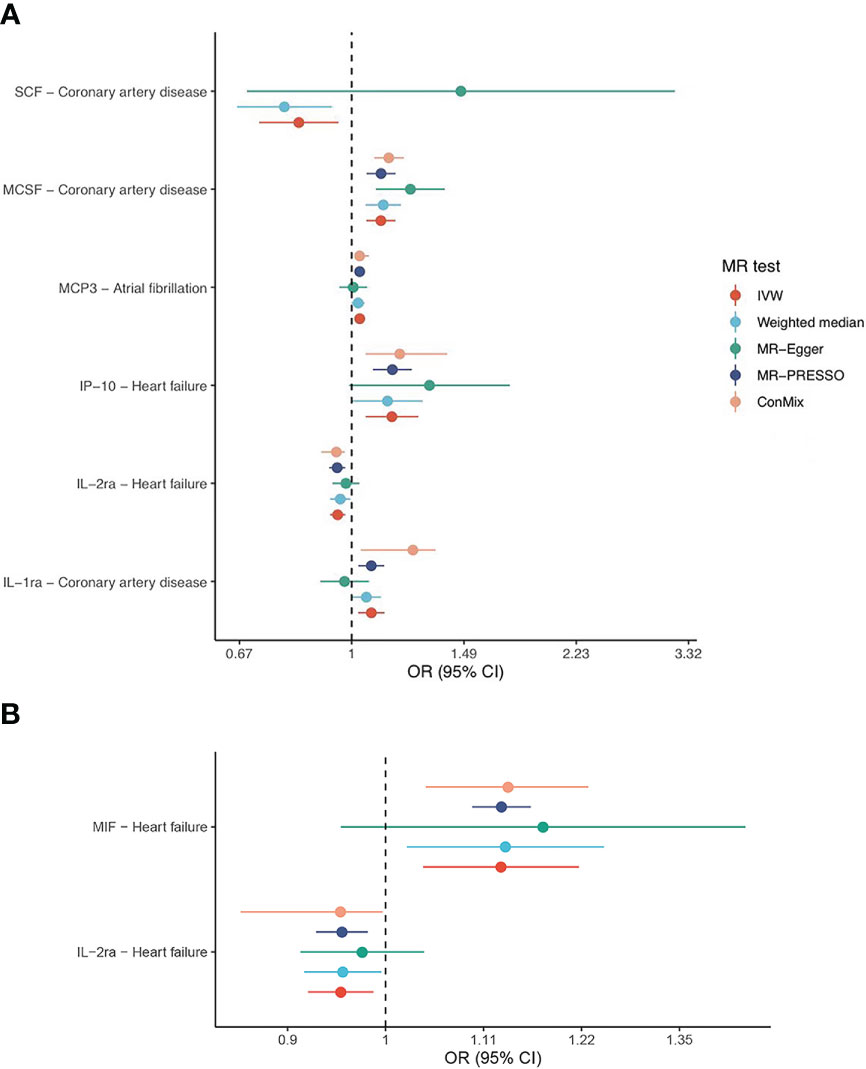
Figure 3 Forest plots of IVW and sensitivity analyses. Shown are the associations that withstand multiple comparison correction (stratified FDR < 10%) based on the (A) cis-pQTL and (B) cis-eQTL instruments.
Using the cis-pQTL instruments in IVW analysis, genetic predicated higher levels of four cytokines had a suggestive association with an increased risk of CAD, including IL-1ra (interleukin-1 receptor antagonist, odds ratio [OR]: 1.07, 95% confidence interval [CI]: 1.02-1.12, P: 0.004), MCSF (macrophage colony-stimulating factor, OR: 1.11, 95% CI: 1.05-1.17, P: 8.88E-05), MIF (macrophage inflammatory protein, OR: 1.11, 95% CI: 1.02-1.22, P: 0.021), SeSelectin (soluble E-selectin, OR: 1.17, 95% CI: 1.04-1.31, P: 0.007), while one cytokine, SCF (stem cell factor, OR: 0.83, 95% CI: 0.72-0.95, P: 0.009), to a lower risk of CAD. After stratified FDR correction, the significance of MIF results was not remained. As expected, when using the cis-eQTL instruments and similarly estimated by IVW, most of findings from our main analysis including IL-1ra, MCSF were replicated, yet the MIF (OR: 1.13, 95% CI: 1.06-1.22, P: 4.15E-04) maintained notable significant relationship with CAD risk. In addition, we observed the novel evidence regarding IL-1a (OR: 0.77, 95% CI: 0.64-0.92, P: 0.004).
Likewise, tested by IVW method using the cis-pQTL instruments, six cytokines (IL-2ra, IP-10 [interferon gamma-induced protein 10], MCSF, MIF, MIP1a, and VEGF [vascular endothelial growth factor]) showed suggestive association with the risk of HF, six cytokines (activePAI [active plasminogen activator inhibitor-1], IL-18, MCP-3, MIP1a, SeSelectin, and TRAIL [TNF-related apoptosis inducing ligand]) with AF, and three cytokines (Eotaxin, MCSF, and SCF) with stroke. When considering the stratified FDR of 10% or less, only two cytokines, IL-2ra (OR: 0.95, 95% CI: 0.93-0.98, P: 2.93E-04) and IP-10 (OR: 1.15, 95% CI: 1.05-1.27, P: 0.003), reached a statistical significance (stratified FDR < 10%) for HF, as well as two cytokines, MCP-3 (OR: 1.03, 95% CI: 1.01-1.05, P: 0.003) and SeSelectin (OR: 1.19, 95% CI: 1.07-1.32, P: 0.002), for AF. No significant sign was survived for stroke after stratified FDR correction. With cis-eQTL instruments and stratified FDR adjustment applied, the additional associations between IL-6 (OR: 2.01, 95% CI: 1.27-3.17, P: 0.003), MIF (OR: 1.13, 95% CI: 1.04-1.22, P: 0.004) and HF, as well as FGFBasic (basic fibroblast growth factor) and AF (OR: 0.66, 95% CI: 0.5-0.84, P: 0.001) were captured.
Focused on the associations that withstand multiple comparison correction (stratified FDR < 10%), their corresponding sensitivity analyses indicated roughly the same estimates although several methods yield wide CIs due to less statistical power (refer to Figure 3). Further examination revealed little evidence of heterogeneity (majority of P-value of Cochran Q statistic > 0.05) or horizontal pleiotropy (majority of P-value of MR-Egger intercept > 0.05), as shown in the Supplementary Tables 5, 6.
Correlation analysis suggested a moderate correlation of IVW estimates obtained using the cis-pQTL and cis-eQTL instruments (correlation coefficient: 0.41, P: 7.9E-05, Figure 4). Their moderate correlation, combined with the biological background foreshadowed earlier, validated the rationality of using cis-pQTL instruments for the main analysis and cis-eQTL as a complement. Furthermore, sufficient statistical power was achieved in our MR study to detect the causal associations of cytokines with CVD outcomes, provided they were true. By complementing the statistical power of the two instruments, we attained a power of 100% to detect an OR of 1.2/0.83 for the majority of the associations (Supplementary Tables 7, 8).
Discussion
In our MR analysis, we systematically assessed the causal roles of circulating cytokine levels in the four CVD outcomes. The results provided the strong evidence in favor of the causal relationships of genetic predicted levels of IL-1ra, MCSF, SCF, and SeSelectin with CAD, genetic predicted levels of IL-2ra and IP-10 with HF, as well as genetic predicted levels of MCP-3 and SeSelectin with AF. There was also suggestive evidence supporting causal effect of IL-1a, MIF concentrations on CAD, of IL-6, MIF concentrations on HF, as well as of FGFBasic concentrations on AF. The relationship of circulating cytokine concentrations with stroke were attenuated after correction for multiple comparisons. Using the power of molecular genetic markers as IVs, particularly through the use of both pQTL and eQTL instruments, our study was able to overcome potential biases and confounders that arise in observational studies. Our results support the hypothesis that manipulating cytokine levels may represents a promising therapeutic strategy for CVD.
Our findings strengthened or extended existing observational evidence, pointing to an essential role played by certain cytokines, including IP-10, IL-6, IL-1ra, and SCF, in the development of CVD. A study combining data from two prospective cohorts suggested that higher concentration of IP-10 was related to the elevated risk of HF but not CAD or stroke, which largely aligns with our own results (27). In addition, our findings regarding IL-6, based on the eQTL instruments, in relation to HF was in line with the results from a case-cohort study (28). A meta-analysis of six population-based cohorts reported that serum IL-1ra level was positively related to the risk of total CVD (29). A 19.2-year follow-up study with 4,742 participants demonstrated that individuals with high levels of SCF have a decreased risk of cardiovascular events (30). Our results add further specificity to these findings, indicating that the IL-1ra and SCF were more likely to represent the causal factors for CAD risk. This conclusion was also confirmed by a recent MR study that used a different set of IVs (31). Nevertheless, the aforementioned MR analysis gave the evidence for the involvement of IL-6 on CAD and AF, which was also consistent with the findings from two previous MR studies that we foreshadowed in the introduction section (6, 7). Due to the limited availability of valid pQTLs for IL-6 and a single SNP as the only eQTL instrument, our study may be underpowered to fully establish its relationship with CVD outcomes. Georgakis et al. found that genetic predicted circulating levels of MCP-1 was positively associated with stroke risk (8), while our study failed to capture this signal. This may be due to different sources of IVs (three independent cohorts v.s. two independent cross-sectional surveys) (32) and different selection criteria (P < 1e-4 v.s. FDR < 5%). More studies are needed to further explore. Notably, our study offered some novel insights regarding MCP-3, MCSF, and SeSelectin, which have not been previously found or minimally explored in direct relation to CVD.
Cytokines may have both direct and indirect effects on the cardiovascular system. Direct effects include alterations in the function of the heart and blood vessels such as increased heart rate, reduced blood flow, and changed blood pressure regulation (33, 34). The relationship between cytokines and CVD is complex and multilayered, with the key indirect mechanism mainly being inflammation and oxidative stress (2, 35). Inflammatory cytokines like TNF and IL-6 activate immune cells, such as monocytes and macrophages. On the one hand, activated macrophages release various inflammatory molecules and reactive oxygen species (ROS) which lead to inflammation, oxidative stress, and ultimately, endothelial dysfunction (36). On the other hand, this accumulation of immune cells and other degenerative material in the inner layer of artery walls could lead to the development of atheroma, contributing to cardiovascular events (37). More seriously, oxidative stress and inflammation have a mutually reinforcing positive feedback loop (38, 39). In contrast, anti-inflammatory cytokines like IL-1ra, which was also supported by our study, has ability to block inflammatory signals from IL-1 by binding to the IL-1 receptor (40). In addition, thrombosis caused by platelet activation and increased heart rate and blood pressure caused by adrenergic activation were also believed to be potential causative pathways induced by cytokines in the development of CVD.
One main strength of the current study is the broad scope of cytokines that we covered, as well as the substantial sample size for each trait of interest that we used, especially for cytokines, which is larger than previously used GWAS (31,112 v.s. 8,293) (32). Another important strength is the utilization of QTLs as IVs, which are in close proximity to the encoding gene region, minimizing the likelihood of horizontal pleiotropy (41). Several limitations should be acknowledged. Firstly, as mentioned early, although a relatively lenient threshold was applied, the limited number of instruments for several cytokines, such as IL-6 and/or MIF, due to the cis-instrument definition approach, may result in a less statistical power, especially for MR sensitivity analysis which requires a higher number of instruments. Additionally, because of the high correlation between cytokines, particularly within a category, such as the ILs family, as well as correlations among four types of CVD, the naive multiple comparison adjustment may be excessive, further affecting the false negative. We hereby reminded that even in cases of negative results, complete exclusion of causality cannot be ensured and thus such results should be interpreted with caution. Secondly, the expression of certain cytokines can be influenced by age and the changing external setting, such as a bimodal curve that has been described for IL-1ra expression throughout the life stage (42). The estimates of a lifetime effect of cytokines on CVD provided by MR may not deliver much clinical meaningful for age-specific interventions. There may be non-liner effects or interactions between cytokines that are not captured by the present study. An age-specific MR analysis especially with individual-level data was warranted in future endeavors. Thirdly, even though a wide panel of cytokines was investigated, some other important kinds of cytokines like IL-1β (43) and IL-10 (44), known for their direct role in targeting inflammation in atherosclerosis, were not analyzed in our study due to the lack of available QTL instruments for these cytokines. Lastly, due to the inaccessibility of the full summary statistics for cytokines, we were unable to conduct the colocalization analysis, which are valuable in strengthening the observed MR associations in helping identify associations that may have arisen due to confounding by LD. Further studies are warranted to strengthen our findings with colocalization when full summary statistics for cytokines are available.
Conclusion
To conclude, based on innovative IVs that incorporate gene expression relevance and large genetic association data, this MR study comprehensively examined the causal influence of circulating cytokine levels on four major CVDs. Our MR study provides robust evidence that the levels of certain cytokines were associated with the development of CVD and highlights the importance of considering cytokines as potential targets for the prevention and management of CVD. Further research, if possible, clinical trials, are necessary to validate these findings and delve into the underlying biological mechanisms specifically at the specific cytokine level.
Data availability statement
The original contributions presented in the study are included in the article/Supplementary material. Further inquiries can be directed to the corresponding author.
Ethics statement
Ethical review and approval was not required for the study on human participants in accordance with the local legislation and institutional requirements. The patients/participants provided their written informed consent to participate in this study.
Author contributions
RZ and TW were the major contributors in conceptualisation. TW, ZZ and LL analyzed the data. ZZ, BL, MW, WZ, QC, and FL verified the correctness of the data. TW, ZZ and RZ were major contributors in writing the manuscript. All authors contributed to the article and approved the submitted version.
Funding
This study was supported by the Qin Chuangyuan Traditional Chinese Medicine Innovation Research and Development Transformation Project (No.2022-QCYZH-022), Key Program for the Traditional Chinese Medicine Inheritance and Innovation and “Qin Medicine” Development [No. 2021-03-22-001], the Key Basic Natural Science Foundation of Shaanxi Province [No. 2022JZ-47], the Key Industrial Innovation Chain Project in Shaanxi Province of China [No. 2021ZDLSF02-03 and 2020ZDLSF01-08], the Shaanxi Provincial Health and Health Research Fund Project [No. 2022D024], and the Natural Science Foundation of Shaanxi Province [No.2023-YBSF-086 and No.2021JQ-911].
Acknowledgments
The data analyzed in this study was provided by Bouras’s Lab, SCALLOP Consortium, Karhunen’s Lab, INTERVAL Study, CARDIoGRAMplusC4D Consortium, HERMES Consortium, Nielsen’s Lab, and MEGASTROKE Consortium. We gratefully acknowledge their contributing studies and the participants in the corresponding studies without whom this effort would not be possible.
Conflict of interest
The authors declare that the research was conducted in the absence of any commercial or financial relationships that could be construed as a potential conflict of interest.
Publisher’s note
All claims expressed in this article are solely those of the authors and do not necessarily represent those of their affiliated organizations, or those of the publisher, the editors and the reviewers. Any product that may be evaluated in this article, or claim that may be made by its manufacturer, is not guaranteed or endorsed by the publisher.
Supplementary material
The Supplementary Material for this article can be found online at: https://www.frontiersin.org/articles/10.3389/fimmu.2023.1175421/full#supplementary-material
References
1. Roth GA, Mensah GA, Fuster V. The global burden of cardiovascular diseases and risks: a compass for global action. J Am Coll Cardiol (2020) 76(25):2980–1. doi: 10.1016/j.jacc.2020.11.021
2. Amin MN, Siddiqui SA, Ibrahim M, Hakim ML, Ahammed MS, Kabir A, et al. Inflammatory cytokines in the pathogenesis of cardiovascular disease and cancer. SAGE Open Med (2020) 8:2050312120965752. doi: 10.1177/2050312120965752
3. Kaptoge S, Seshasai SR, Gao P, Freitag DF, Butterworth AS, Borglykke A, et al. Inflammatory cytokines and risk of coronary heart disease: new prospective study and updated meta-analysis. Eur Heart J (2014) 35(9):578–89. doi: 10.1093/eurheartj/eht367
4. Georgakis MK, Malik R, Bjorkbacka H, Pana TA, Demissie S, Ayers C, et al. Circulating monocyte chemoattractant protein-1 and risk of stroke: meta-analysis of population-based studies involving 17 180 individuals. Circ Res (2019) 125(8):773–82. doi: 10.1161/CIRCRESAHA.119.315380
5. Davies NM, Holmes MV, Davey Smith G. Reading mendelian randomisation studies: a guide, glossary, and checklist for clinicians. BMJ (2018) 362:k601. doi: 10.1136/bmj.k601
6. Interleukin-6 Receptor Mendelian Randomisation Analysis C, Swerdlow DI, Holmes MV, Kuchenbaecker KB, Engmann JE, Shah T, et al. The interleukin-6 receptor as a target for prevention of coronary heart disease: a mendelian randomisation analysis. Lancet (2012) 379(9822):1214–24. doi: 10.1016/S0140-6736(12)60110-X
7. Georgakis MK, Malik R, Richardson TG, Howson JMM, Anderson CD, Burgess S, et al. Associations of genetically predicted IL-6 signaling with cardiovascular disease risk across population subgroups. BMC Med (2022) 20(1):245. doi: 10.1186/s12916-022-02446-6
8. Georgakis MK, Gill D, Rannikmae K, Traylor M, Anderson CD, Lee JM, et al. Genetically determined levels of circulating cytokines and risk of stroke. Circulation (2019) 139(2):256–68. doi: 10.1161/CIRCULATIONAHA.118.035905
9. Li Y, Lu J, Wang J, Deng P, Meng C, Tang H. Inflammatory cytokines and risk of ischemic stroke: a mendelian randomization study. Front Pharmacol (2021) 12:779899. doi: 10.3389/fphar.2021.779899
10. Bouras E, Karhunen V, Gill D, Huang J, Haycock PC, Gunter MJ, et al. Circulating inflammatory cytokines and risk of five cancers: a mendelian randomization analysis. BMC Med (2022) 20(1):3. doi: 10.1186/s12916-021-02193-0
11. Folkersen L, Gustafsson S, Wang Q, Hansen DH, Hedman AK, Schork A, et al. Genomic and drug target evaluation of 90 cardiovascular proteins in 30,931 individuals. Nat Metab (2020) 2(10):1135–48. doi: 10.1038/s42255-020-00287-2
12. Sun BB, Maranville JC, Peters JE, Stacey D, Staley JR, Blackshaw J, et al. Genomic atlas of the human plasma proteome. Nature (2018) 558(7708):73–9. doi: 10.1038/s41586-018-0175-2
13. Karhunen V, Gill D, Malik R, Ponsford M, Ahola-Olli A, Papadopoulou A, et al. Genetic study of circulating cytokines offers insight into the determinants, cascades and effects of systemic inflammation. medRxiv (2020). doi: 10.1101/2020.10.26.20219477
14. van der Harst P, Verweij N. Identification of 64 novel genetic loci provides an expanded view on the genetic architecture of coronary artery disease. Circ Res (2018) 122(3):433–43. doi: 10.1161/CIRCRESAHA.117.312086
15. Shah S, Henry A, Roselli C, Lin H, Sveinbjornsson G, Fatemifar G, et al. Genome-wide association and mendelian randomisation analysis provide insights into the pathogenesis of heart failure. Nat Commun (2020) 11(1):163. doi: 10.1038/s41467-019-13690-5
16. Nielsen JB, Thorolfsdottir RB, Fritsche LG, Zhou W, Skov MW, Graham SE, et al. Biobank-driven genomic discovery yields new insight into atrial fibrillation biology. Nat Genet (2018) 50(9):1234–9. doi: 10.1038/s41588-018-0171-3
17. Malik R, Chauhan G, Traylor M, Sargurupremraj M, Okada Y, Mishra A, et al. Multiancestry genome-wide association study of 520,000 subjects identifies 32 loci associated with stroke and stroke subtypes. Nat Genet (2018) 50(4):524–37. doi: 10.1038/s41588-018-0058-3
18. Consortium GT. The GTEx consortium atlas of genetic regulatory effects across human tissues. Science (2020) 369(6509):1318–30. doi: 10.1126/science.aaz1776
19. Burgess S, Butterworth A, Thompson SG. Mendelian randomization analysis with multiple genetic variants using summarized data. Genet Epidemiol (2013) 37(7):658–65. doi: 10.1002/gepi.21758
20. Burgess S, Thompson SG. Interpreting findings from mendelian randomization using the MR-egger method. Eur J Epidemiol (2017) 32(5):377–89. doi: 10.1007/s10654-017-0255-x
21. Bowden J, Davey Smith G, Haycock PC, Burgess S. Consistent estimation in mendelian randomization with some invalid instruments using a weighted median estimator. Genet Epidemiol (2016) 40(4):304–14. doi: 10.1002/gepi.21965
22. Burgess S, Foley CN, Allara E, Staley JR, Howson JMM. A robust and efficient method for mendelian randomization with hundreds of genetic variants. Nat Commun (2020) 11(1):376. doi: 10.1038/s41467-019-14156-4
23. Verbanck M, Chen CY, Neale B, Do R. Detection of widespread horizontal pleiotropy in causal relationships inferred from mendelian randomization between complex traits and diseases. Nat Genet (2018) 50(5):693–8. doi: 10.1038/s41588-018-0099-7
24. Burgess S, Thompson SG, Collaboration CCG. Avoiding bias from weak instruments in mendelian randomization studies. Int J Epidemiol (2011) 40(3):755–64. doi: 10.1093/ije/dyr036
25. Brion MJ, Shakhbazov K, Visscher PM. Calculating statistical power in mendelian randomization studies. Int J Epidemiol (2013) 42(5):1497–501. doi: 10.1093/ije/dyt179
26. Sun L, Craiu RV, Paterson AD, Bull SB. Stratified false discovery control for large-scale hypothesis testing with application to genome-wide association studies. Genet Epidemiol (2006) 30(6):519–30. doi: 10.1002/gepi.20164
27. Leavitt C, Zakai NA, Auer P, Cushman M, Lange EM, Levitan EB, et al. Interferon gamma-induced protein 10 (IP-10) and cardiovascular disease in African americans. PloS One (2020) 15(4):e0231013. doi: 10.1371/journal.pone.0231013
28. Chia YC, Kieneker LM, van Hassel G, Binnenmars SH, Nolte IM, van Zanden JJ, et al. Interleukin 6 and development of heart failure with preserved ejection fraction in the general population. J Am Heart Assoc (2021) 10(11):e018549. doi: 10.1161/JAHA.120.018549
29. Herder C, de Las Heras Gala T, Carstensen-Kirberg M, Huth C, Zierer A, Wahl S, et al. Circulating levels of interleukin 1-receptor antagonist and risk of cardiovascular disease: meta-analysis of six population-based cohorts. Arterioscler Thromb Vasc Biol (2017) 37(6):1222–7. doi: 10.1161/ATVBAHA.117.309307
30. Bjorkbacka H, Yao Mattisson I, Wigren M, Melander O, Fredrikson GN, Bengtsson E, et al. Plasma stem cell factor levels are associated with risk of cardiovascular disease and death. J Intern Med (2017) 282(6):508–21. doi: 10.1111/joim.12675
31. Yuan S, Lin A, He QQ, Burgess S, Larsson SC. Circulating interleukins in relation to coronary artery disease, atrial fibrillation and ischemic stroke and its subtypes: a two-sample mendelian randomization study. Int J Cardiol (2020) 313:99–104. doi: 10.1016/j.ijcard.2020.03.053
32. Ahola-Olli AV, Wurtz P, Havulinna AS, Aalto K, Pitkanen N, Lehtimaki T, et al. Genome-wide association study identifies 27 loci influencing concentrations of circulating cytokines and growth factors. Am J Hum Genet (2017) 100(1):40–50. doi: 10.1016/j.ajhg.2016.11.007
33. Prabhu SD. Cytokine-induced modulation of cardiac function. Circ Res (2004) 95(12):1140–53. doi: 10.1161/01.RES.0000150734.79804.92
34. Vila E, Salaices M. Cytokines and vascular reactivity in resistance arteries. Am J Physiol Heart Circ Physiol (2005) 288(3):H1016–1021. doi: 10.1152/ajpheart.00779.2004
35. Mehra VC, Ramgolam VS, Bender JR. Cytokines and cardiovascular disease. J Leukoc Biol (2005) 78(4):805–18. doi: 10.1189/jlb.0405182
36. Higashi Y. Roles of oxidative stress and inflammation in vascular endothelial dysfunction-related disease. Antioxidants (Basel) (2022) 11(10):1958. doi: 10.3390/antiox11101958
37. Hansson GK, Hermansson A.. The immune system in atherosclerosis. Nat Immunol (2021) 12(3):204–12. doi: 10.1038/ni.2001
38. Voigt A, Rahnefeld A, Kloetzel PM, Kruger E. Cytokine-induced oxidative stress in cardiac inflammation and heart failure-how the ubiquitin proteasome system targets this vicious cycle. Front Physiol (2013) 4:42. doi: 10.3389/fphys.2013.00042
39. Bondia-Pons I, Ryan L, Martinez JA. Oxidative stress and inflammation interactions in human obesity. J Physiol Biochem (2012) 68(4):701–11. doi: 10.1007/s13105-012-0154-2
40. Volarevic V, Al-Qahtani A, Arsenijevic N, Pajovic S, Lukic ML. Interleukin-1 receptor antagonist (IL-1Ra) and IL-1Ra producing mesenchymal stem cells as modulators of diabetogenesis. Autoimmunity (2010) 43(4):255–63. doi: 10.3109/08916930903305641
41. Davey Smith G, Hemani G. Mendelian randomization: genetic anchors for causal inference in epidemiological studies. Hum Mol Genet (2014) 23(R1):R89–98. doi: 10.1093/hmg/ddu328
42. Decker ML, Grobusch MP, Ritz N. Influence of age and other factors on cytokine expression profiles in healthy children-a systematic review. Front Pediatr (2017) 5:255. doi: 10.3389/fped.2017.00255
43. Libby P. Interleukin-1 beta as a target for atherosclerosis therapy: biological basis of CANTOS and beyond. J Am Coll Cardiol (2017) 70(18):2278–89. doi: 10.1016/j.jacc.2017.09.028
Keywords: circulating cytokines, cardiovascular disease, Mendelian randomization, cis-quantitative trait locus, European
Citation: Wei T, Zhu Z, Liu L, Liu B, Wu M, Zhang W, Cui Q, Liu F and Zhang R (2023) Circulating levels of cytokines and risk of cardiovascular disease: a Mendelian randomization study. Front. Immunol. 14:1175421. doi: 10.3389/fimmu.2023.1175421
Received: 27 February 2023; Accepted: 12 May 2023;
Published: 25 May 2023.
Edited by:
Ping Zeng, Xuzhou Medical University, ChinaReviewed by:
Kevin Sean Kimbro, Morehouse School of Medicine, United StatesJacqueline Valverde-Villegas, UMR5535 Institut de Génétique Moléculaire de Montpellier (IGMM), France
Copyright © 2023 Wei, Zhu, Liu, Liu, Wu, Zhang, Cui, Liu and Zhang. This is an open-access article distributed under the terms of the Creative Commons Attribution License (CC BY). The use, distribution or reproduction in other forums is permitted, provided the original author(s) and the copyright owner(s) are credited and that the original publication in this journal is cited, in accordance with accepted academic practice. No use, distribution or reproduction is permitted which does not comply with these terms.
*Correspondence: Ronghuai Zhang, science_game@126.com