- Department of Gastroenterology, The First Affiliated Hospital of Bengbu Medical College, Bengbu, Anhui, China
Objective: The Lactate-to-Albumin Ratio (LAR) has been applied as a new predictor in sepsis, heart failure, and acute respiratory failure. However, the role of LAR in predicting all-cause mortality in patients with acute pancreatitis has not been evaluated. Therefore, this study aimed to elucidate the correlation between LAR and 28-d all-cause mortality in patients with Acute Pancreatitis (AP).
Methods: This study is a retrospective cohort study with the data from the MIMIC-IV (v1.0) database. We included adult patients with acute pancreatitis who were admitted to the intensive care unit in the study. The primary outcome was to evaluate the ability of LAR to predict death at 28-d of hospital admission in patients with AP.
Results: A total of 539 patients with acute pancreatitis were included in this study. They were divided into a survival group (486 patients) and a death group (53 patients) according to whether they survived within 28-d of admission, and the mortality rate of patients within 28-d of admission was 9.8%. LAR was shown to be an independent predictor of all-cause mortality within 28-d of admission in patients with AP by multivariate COX regression analysis (HR, 1.59; 95% CI, 1.23 - 2.05; P < 0.001). the Area Under the Curve (AUC) value for LAR was 74.26% (95% CI: 67.02% - 81.50%), which was higher than that for arterial blood lactate (AUC = 71.25%) and serum albumin (AUC = 65.92%) alone. It was not inferior even when compared to SOFA (AUC = 75.15%). The optimal cutoff value for separating the survival and death groups according to Receiver Operating Characteristic (ROC) was found to be 1.1124. plotting Kaplan-Meier analysis with this cutoff value showed that patients with LAR ≥ 1.1124 had significantly higher all-cause mortality within 28-d of admission than those with LAR < 1.1124 (P < 0.001). The final subgroup analysis showed no significant interaction of LAR with each subgroup (P for interaction: 0.06 - 0.974).
Conclusion: LAR can be used as an independent predictor of all-cause mortality in AP patients within 28-d of admission, with superior prognostic performance than arterial blood lactate or serum albumin alone.
1 Introduction
Acute pancreatitis (AP) admissions are one of the most common causes of hospitalization for gastrointestinal disorders, with an annual incidence of 13-45 cases per 100,000 for this disease (1). AP is an flammatory state of the pancreas in which initial disintegration of the alveolar cells and activation of pancreatic proteases leads to local injury, which with progression can cause systemic inflammatory response syndrome and even organ failure. The prognosis of AP depends on the severity of the disease, with approximately 75-80% of patients progressing slowly and being cured by intravenous fluid therapy and supportive care alone (2, 3). However, about 20% of patients progress to moderate or severe acute pancreatitis with critical conditions such as pancreatic or peripancreatic tissue necrosis and organ failure, with an overall mortality rate of 20% to 40% (4, 5). Therefore, early assessment of disease severity and preparation of interventions to reduce mortality is essential. Current scores that can assess the severity of AP include Ranson criteria (6), Balthazar grade (7), Acute Physiology and Chronic Health Evaluation II (APAChE-II) (6), Bedside Index for Severity in AP (BISAP) (8), etc. These scores usually require the collection of multiple indicators to complete the assessment of the patient’s condition, which increases the risk of death in some patients by missing the optimal time for treatment. For this reason, a simple, cost-effective and high-sensitivity indicator to forecast the severity of acute pancreatitis is a matter of urgent necessity.
It has been demonstrated that lactate, as a product of anaerobic metabolism, is reflective of tissue hypoperfusion and the seriousness of cellular hypoxia. It is as well able to predict organ failure and the mortality rate in critically ill patients (9, 10). However, liver dysfunction or abnormal proteolytic metabolism and metformin intake can result in abnormal lactate levels as well (11, 12). Therefore, the single use of lactate level prediction does not ensure a credible consequence. Meanwhile, we found in previous studies that albumin acts as a negative acute phase reactant, and it has been shown to correlate with inflammation severity, disease prognosis and mortality. In physiological mechanisms, serum albumin plays various roles as extracellular antioxidant, buffer, immunomodulator, detoxifier and transporter protein in plasma (13, 14). However, the patient’s nutritional status or chronic inflammation can also affect albumin levels. Therefore, prediction based on albumin levels alone may also have limitations (15). Several studies have evaluated the validity of the ratio of lactate to albumin (LAR) as a predictor in diseases such as infectious shock or severe sepsis and cardiac arrest (16–18). However, it is unclear about the relationship between LAR and mortality in patients with AP. Therefore, we obtained information on hospitalizations of patients with AP admitted between 2008-2019 through the Medical Information Mart for Intensive Care IV version 1.0 [MIMIC-IV (v1.0)] database, which aimed to analyze the relationship between LAR and acute all-cause mortality within 28-d hospital of admissions in patients with AP. And the SOFA score was used as an indicator of the severity of disease in the included population.
2 Methods
2.1 Database introduction
The data in this study are all obtained from the MIMIC-IV (v1.0) database, which is a large, publicly accessible database that was developed and managed by the MIT Computational Physiology Laboratory (https://physionet.org/content/mimiciv/1.0/). This database covers information on all patients admitted to the Beth Israel Deaconess Medical Center (BIDMC) during the years from 2008 to 2019. Each patient’s length of stay, laboratory tests, medication treatment, vital signs and other comprehensive information is recorded. And for the protection of patient privacy, all personal information is de-identified, with random codes used to replace patient identification, therefore we do not need informed consent and ethical approval of patients. The MIMIC-IV (v1.0) database can be downloaded from the PhysioNet online forum (https://physionet.org/). To access this database, the first author of this study, Qiang Liu, completed the Collaborative Institutional Training Initiative (CITI) course and passed both the “Conflicts of Interest” and “Data or Specimens Only Research” exams (ID: 50082616). The research team was finally qualified to use the database and extract data.
2.2 Population selection criteria
A total of 523,740 admissions were recorded in the MIMIC-IV database, of which 76,540 were admitted to the ICU. Hospital admission information for patients with AP was extracted according to International Classification of Diseases, 9th Revision (ICD - 9) code 577.0 and International Classification of Diseases, 10th Revision (ICD-10) codes K85-K85.92, with a total of 6222 patients included, of whom 1345 had been admitted to the ICU. After further screening, patients who meet the following criteria will be excluded (1): patients younger than 18 years of age at the time of the first admission; (2) patients admitted repeatedly for acute pancreatitis, for whom only the first admission data were retained; (3) patients who stayed in the ICU for less than 24 hours; (4) patients admitted with end-stage renal disease, cirrhosis or malignancy; (5) patients without recorded blood lactate and serum albumin data within 24 hours of admission. Ultimately, 539 patients were enrolled in this study (Figure 1).
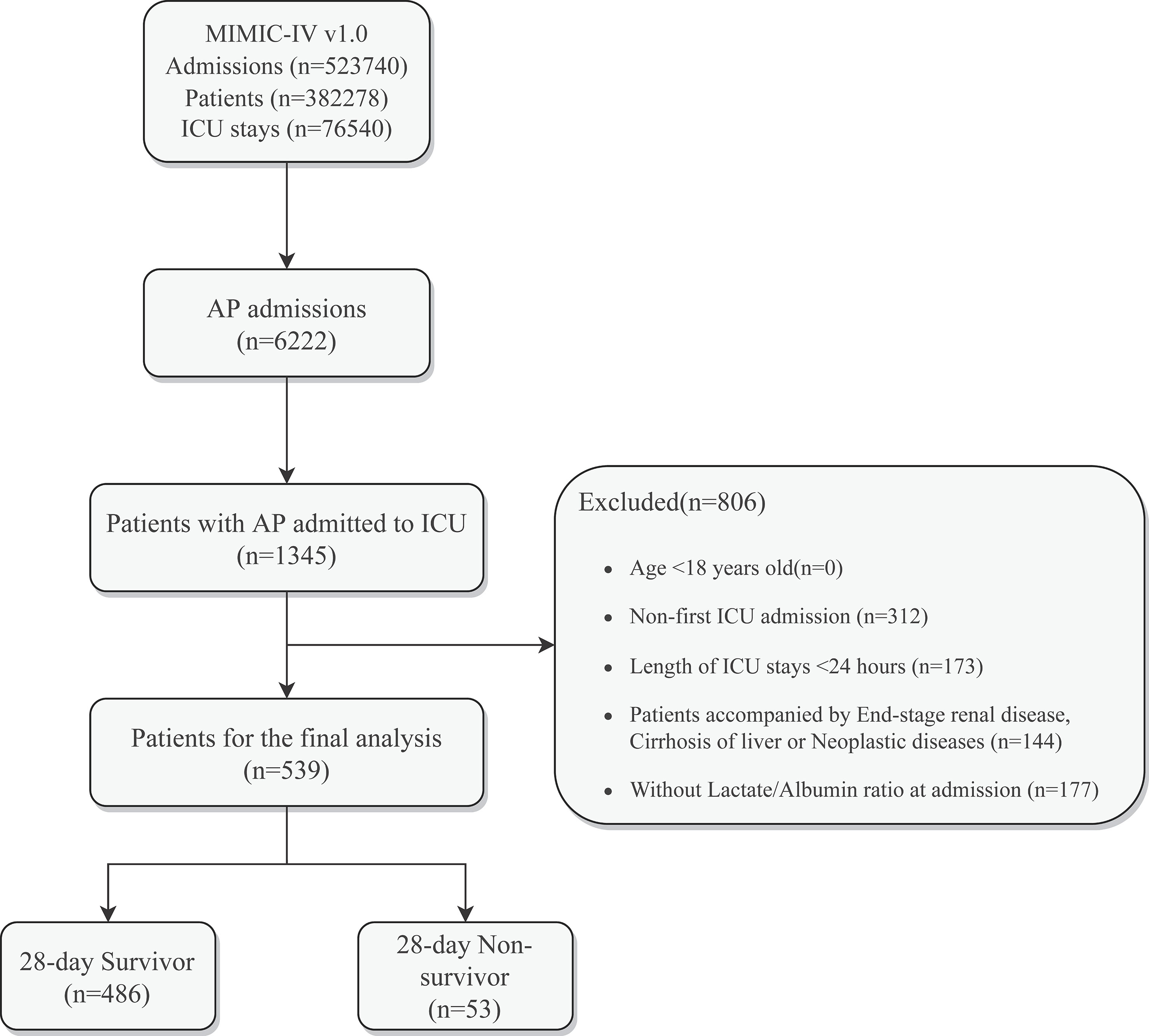
Figure 1 Schematic diagram of study sample selection steps. MIMIC, Medical Information Mart for Intensive Care; AP, Acute Pancreatitis; ICU, intensive care unit; LAR, Lactate/Albumin Ratio.
2.3 Data extraction
The LAR was chosen as the primary study variable. Blood lactate concentration and serum albumin were measured for the first time after admission in order to minimize the interference of lactate and albumin values caused by subsequent treatment. Extraction of potential confounders included Demographics (Age, Gender, Race), Vital Signs (Heart Rate, Systolic Blood Pressure, Diastolic Blood Pressure, Mean Arterial Pressure, Respiratory Rate), Clinical Treatment (Vasopressin, Octreotide, Statins, Betablockers, and Metformin use, Mechanical Ventilation, Continuous Renal Replacement Therapy, Endoscopic Retrograde Cholangiopancreatography), Comorbidities (Acute Kidney Injury, Sepsis, Respiratory Failure, Heart Failure, Atrial Fibrillation, Hypertension, Diabetes, Obesity), Laboratory Indicators (Red Blood Cells, White Blood Cells, Erythrocyte Distribution Width, Platelets, Hemoglobin, Lymphocyte percentage, Erythrocyte Specific Volume, Total Bilirubin, Glutathione, Serum Glucose, Serum Creatinine, Blood Urea Nitrogen, Anion Gap, Prothrombin Time, International Normalized Ratio, Serum Potassium, Serum Sodium, Serum Calcium), and Sequential Organ Failure (SOFA). The data extraction tool used PostgresSQL software (v13.7.1) and Navicate Premium software (version 15) to extract data through a running Structured Query Language (SQL). All code used for the Calculation of Demographic Characteristics, Laboratory Indicators, Comorbidities and Severity Scores were obtained from the GitHub website (GitHub - MIT-LCP/mimic-iv: Deprecated. For the latest MIMIC-IV code, please refer to: https://github.com/MIT-LCP/mimic-code).
2.4 Grouping and endpoint events
In this study, patient subgroups were divided into a 28-d admission survival group (n=486) and a 28-d admission death group (n=53). The primary study endpoint was death from any cause within 28-d of admission. 28-Days All-Cause Mortality was defined as the ratio of the total number of deaths from all causes during a 28-d hospital stay to the average population of that population during the same period.
2.5 Management of missing data and outliers
To avoid bias, variables are excluded when they have more than 15% missing values, such as HbA1c, ipid profile, C-reactive protein, high sensitivity CRP, Aspartate aminotransferase, and patient height or weight. Variables with missing values greater than 5% and less than 15% (Total bilirubin, Lymphocyte percentage, PT, INR) are processed using multiple interpolations (MI) (19) to select the relative optimal set of data to fill in the missing values. On the other hand, variables with missing values less than 5% (Heart rate, Systolic Blood Pressure, Diastolic Blood Pressure, Mean Arterial Pressure, Respiratory Rate, Red Blood Cells, White Blood Cells, Red Blood Cell Distribution Width, Platelets, Hemoglobin, Erythrocyte Specific Volume, Glutathione Transaminase, Blood Urea Nitrogen, Serum Calcium) are replaced with the mean of that variable. Variables with abnormal values are handled by the winsorize method, using the winsor2 command, with 1% and 99% as cut-off points. Missing and abnormal data are processed using the STATA software (Version 17).
2.6 Statistical analysis
The normality of continuous variables was assessed by the Kolmogorov-Smirnov test. Continuous variables were expressed as mean ± SD for normally distributed variables, median (IQR) for non-normally distributed continuous variables, and numbers (%) for categorical variables. When analyzing baseline characteristics, continuous variables were compared by T-test or One-Way ANOVA, and categorical variables were compared by Pearson’s χ2 test and Fisher’s test. Potential risk factors were identified by using One-Way Cox Regression analysis, and variables with p-values less than 0.1 were included in Multi-Way Cox Regression analysis to identify independent risk factors for in-hospital mortality. Receiver Operating Characteristic (ROC) analysis was used to assess the predictive ability of Lactate, Albumin, LAR, and SOFA for mortality at 28-d of admission, and the sensitivity and specificity of each index, as well as to calculate the Area Under Curve (AUC). The optimal cut-off value of LAR was determined by the Youden index, and this was used to divide the LAR into high value and low value groups. The unadjusted survival curves were then plotted by the Kaplan-Meier (KM) method, and the two groups of curves were compared using the log Rank test. Finally, we also performed a Subgroup analysis to investigate whether LAR had any effect in different subgroups, including Age, Gender, Race, Acute Kidney Injury, Sepsis, Obesity, Hypertension, Respiratory Failure, Diabetes, Atrial Fibrillation, Heart Failure and Serum Sodium Concentration. All analyses were performed using Free statistics software version 1.6 and the statistical package R 4.1.1. A two-tailed test indicating P < 0.05 is considered statistically significant.
3 Results
3.1 Baseline demographic and clinical characteristics
The baseline characteristics of the 28-d admission survival and non-survival groups are listed in Table 1. A total of 539 patients matched the inclusion criteria. That includes 227 (42.1%) females and 312 (57.9%) males. The median age of the patients is 57.0(45.0,71.0) years. There were 329 (61%) whites admitted to the hospital. The 28-d post-admission mortality rate was 9.8%. We noticed that AP non-surviving patients are older (P < 0.001) and had lower SBP, DBP, and MBP, while higher RR and SOFA when compared to the 28-d survival group. CRRT and Vasopressin accounted for 50.9% and 58.5% of the treatment management of patients in the non-survival group, and 71.7% had respiratory failure complications, but only 34% of non-surviving patients had combined heart failure. Laboratory indicators showed that LAR at admission was higher in the non-survival group than in the survival group (1.2 [0.6, 1.9] vs 0.6 [0.4, 0.9], P < 0.001). And RDW, Serum Creatinine, BUN, PT, INR, Albumin, and Lactate were all remarkably higher (P < 0.05) than in the survival group, while the remaining covariates were not significantly different in the two groups (P > 0.05).
3.2 The LAR is an independent risk factor for all-cause mortality at 28-d of hospital admission
Covariates with obvious differences in Table 1 (P < 0.05) were included in the univariate COX regression analysis. Results demonstrated that unadjusted LAR was significantly associated with all-cause mortality within 28-d of hospital admission (HR, 1.45; 95% CI, 1.6 - 1.82; P < 0.001). Covariates with P < 0.1 in Table 2 and risk factors with potential were then included in multivariate COX regression analyses. Table 3 presents adjusted analyses of LAR and in-hospital 28-d all-cause mortality in patients with AP using COX proportional risk models. In the model I, LAR was significantly associated with in-hospital 28-d all-cause mortality (HR, 1.46; 95% CI, 1.15 - 1.85; P < 0.002) after adjusting for Age, Sex and Race. In a further, after adjusting for Age, Sex, Race, AF, Serum Creatinine, and Serum Sodium in Model II, LAR remained an independent predictor (HR, 1.59; 95% CI, 1.23 - 2.05; P < 0.001).
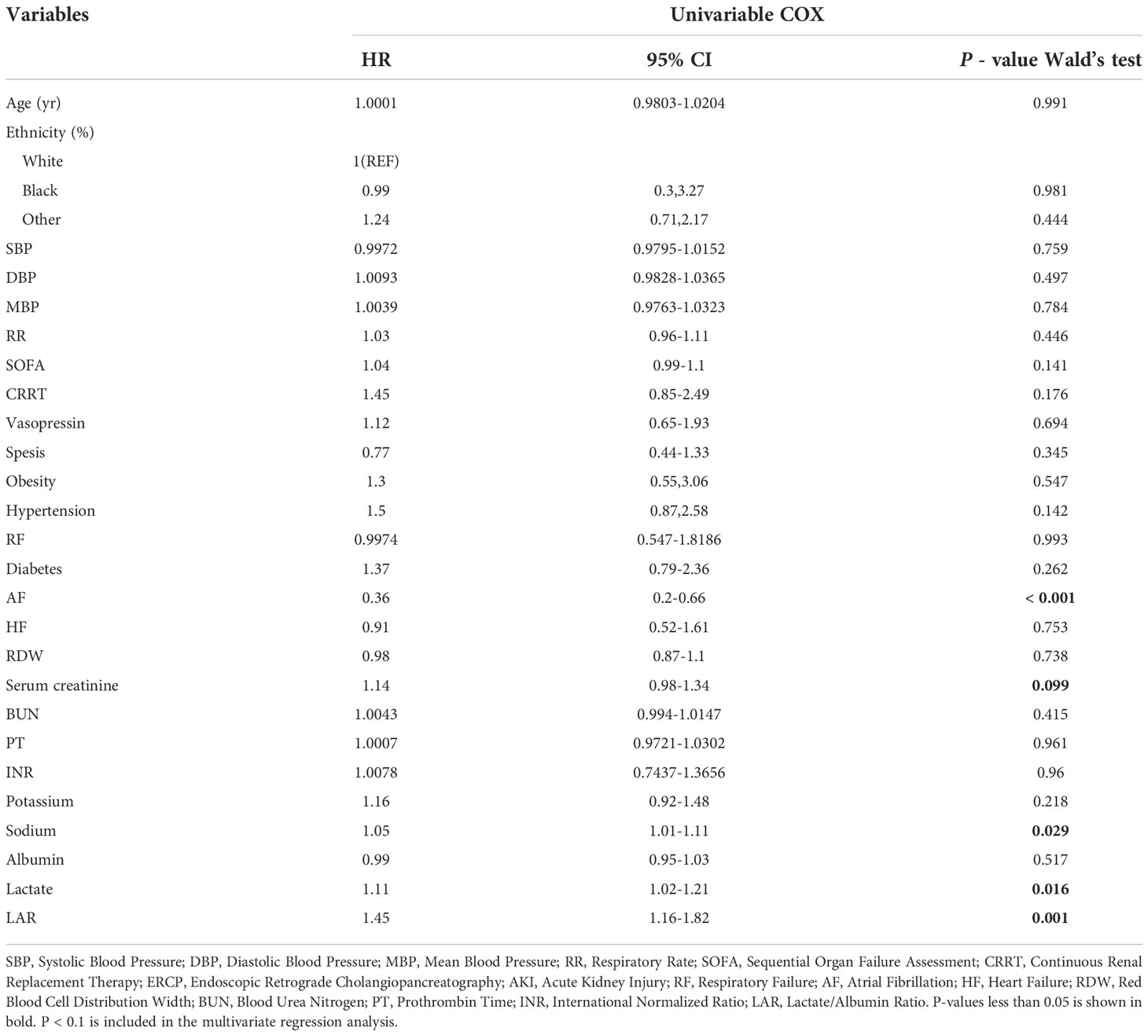
Table 2 Univariate COX analysis of risk factors for death within 28-d in patients by logistic regression analysis.
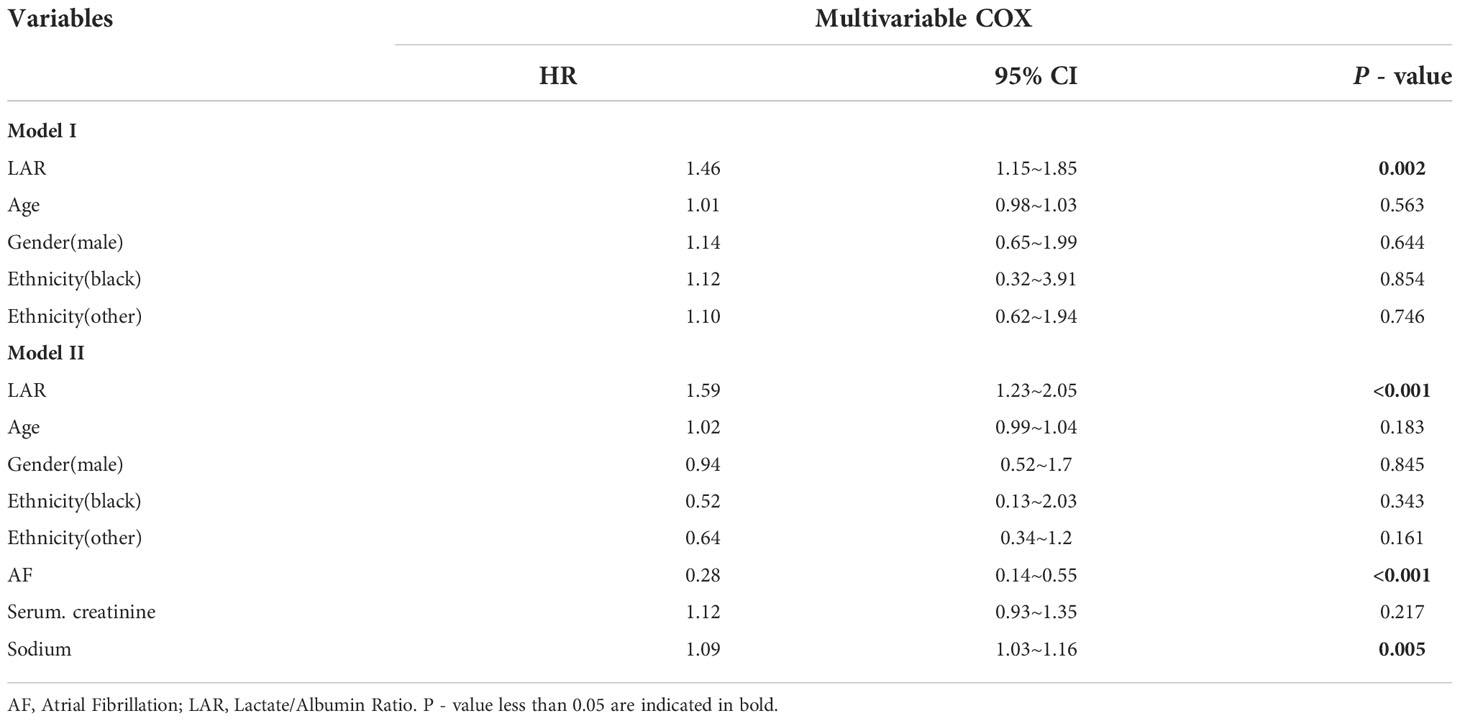
Table 3 Multivariate COX analysis of risk factors for death in patients within 28-d by logistic regression analysis.
3.3 ROC curve analysis and Kaplan–Meier curve
We plotted ROC curves for the four indicators of LAR, Lactate, Albumin and SOFA for predicting all-cause mortality within 28-d of admission in patients with AP, and the information in Figure 2 is listed in Table 4. The AUC of LAR [74.262% (95% CI: 67.024% - 81.501%)] was obviously superior to Lactate [71.252% (95% CI: 64.102% - 78.401%)] and Albumin [65.917% (95% CI: 57.529% - 74.306%)]. Not even inferior to the SOFA [75.153% (95% CI: 68.208% - 82.099%)]. Consequently, LAR has a significant predictive advantage. We meanwhile obtained the best cut-off value of 1.1124 for LAR, which had a sensitivity of 56.6% and a specificity of 82.7%. According to the optimal cut-off value, AP patients were divided into high admission LAR group (LAR ≥ 1.1124, n = 114) and low admission LAR group (LAR < 1.1124, n = 425). And Kaplan-Meier survival analysis curves were plotted (Figure 3), which showed that patients in the high-value group had a significantly higher mortality rate than those in the low-value group (P < 0.001).
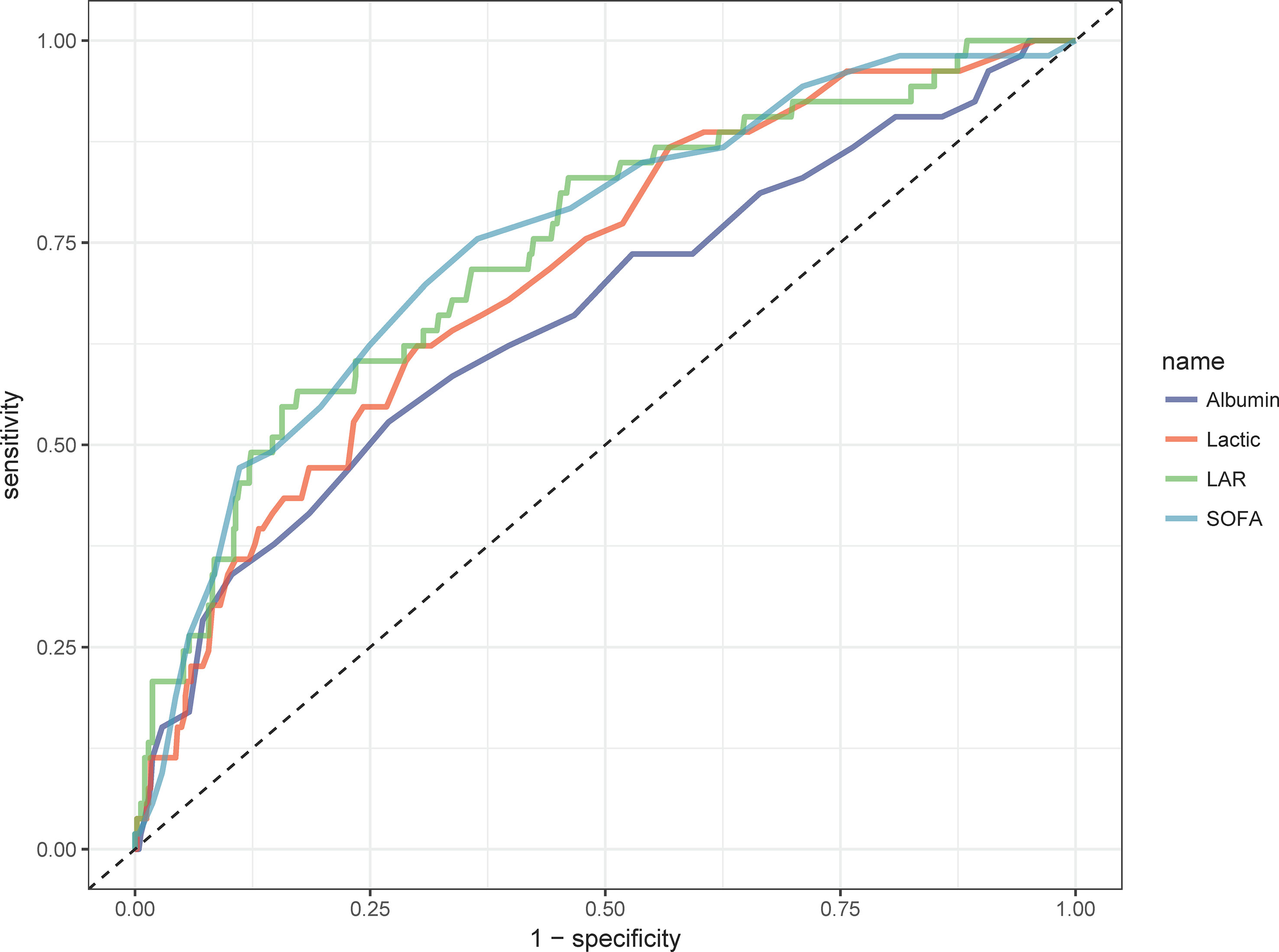
Figure 2 ROC curves of peripheral differential leukocyte count correlate for predicting in-hospital mortality. The green solid line indicates the ROC curve of the LAR. The red solid line indicates the ROC curve for Lactate. Deep blue indicates the ROC curve of Albumin. LAR, lactate/albumin ratio; SOFA, Sequential Organ Failure Assessment.

Table 4 Information of ROC curves in Figure 2.
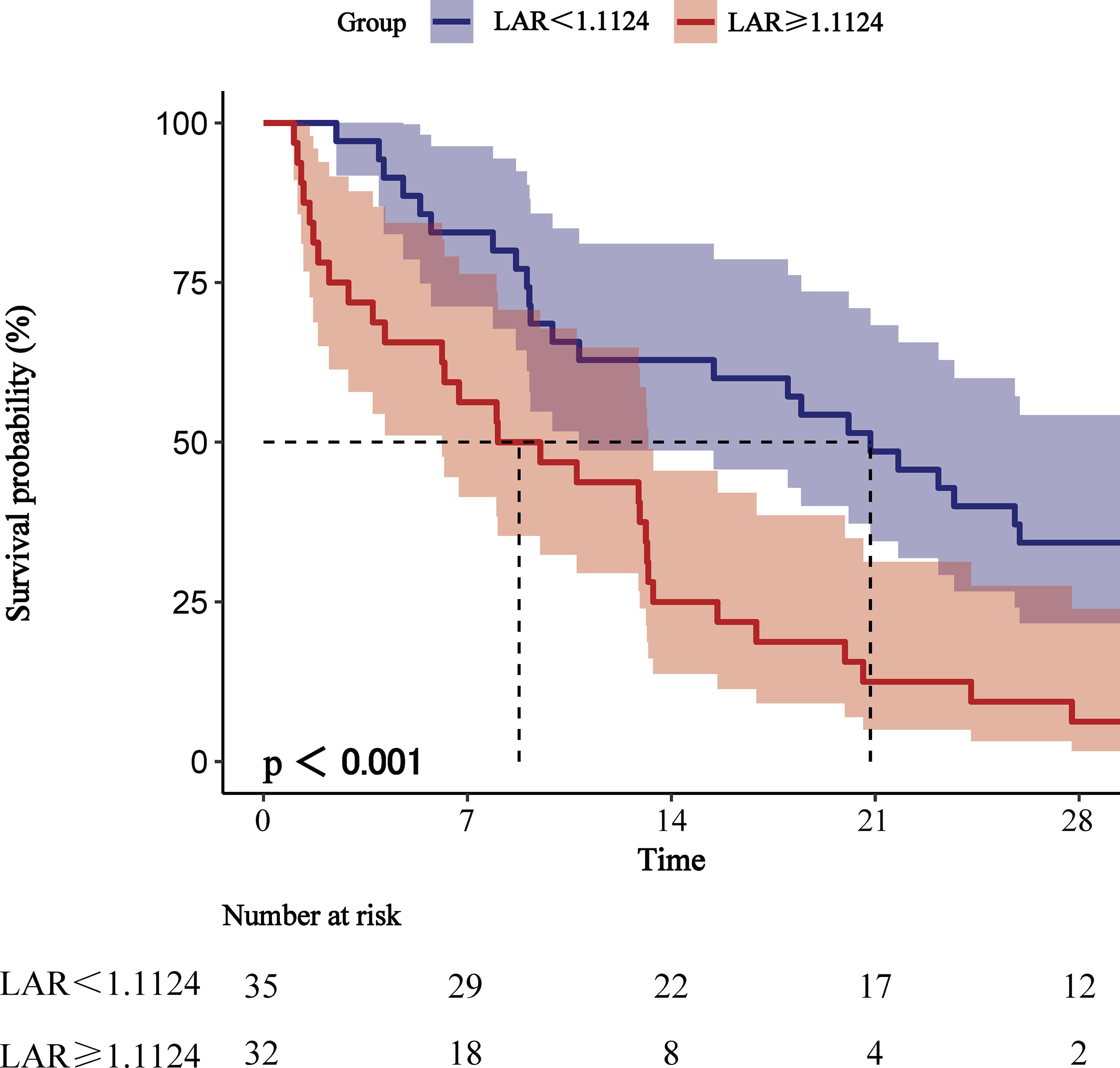
Figure 3 Kaplan-Meier survival analysis curves for all-cause mortality within 28-d of hospital admission.
3.4 Subgroup analysis
Figure 4 indicates as to whether the correlation between LAR and all-cause mortality at 28-d of hospital admission in patients with AP was stable across subgroups. When the stratified analysis was performed for Age, Gender, Race, Race, Acute Kidney Injury, Sepsis, Obesity, Hypertension, Respiratory Failure, Diabetes, Atrial Fibrillation, Heart Failure and Serum Sodium, the forest plot (Figure 4) showed no significant interaction of LAR with each subgroup (P for interaction: 0.084-0.769). This evidences that LAR is an independent prognostic factor.
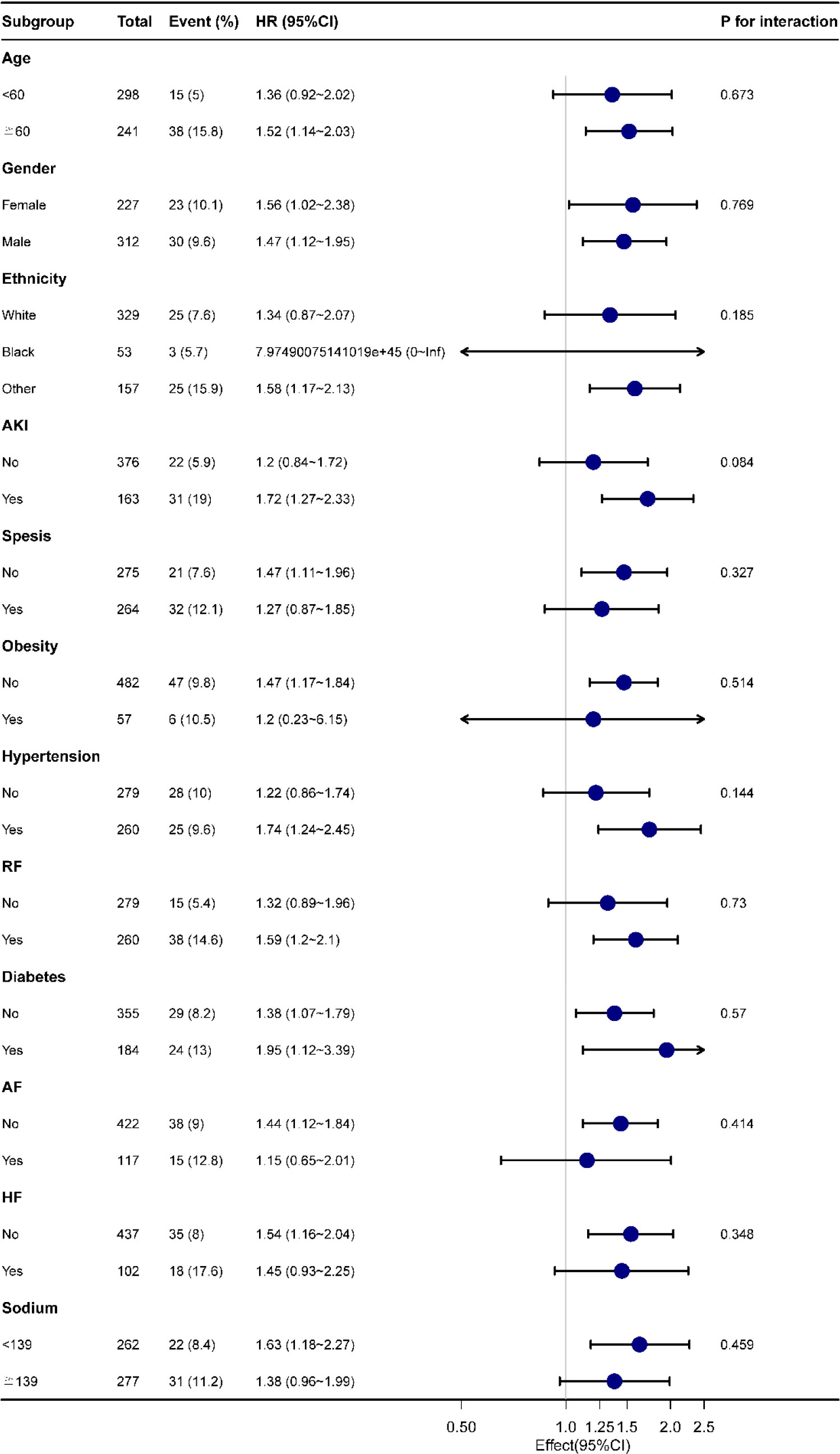
Figure 4 Forest plot for subgroup analysis of the relationship between hospital mortality and LAR. AKI, Acute Kidney Injury; RF, Respiratory Failure; AF, Atrial Fibrillation; HF, Heart Failure.
4 Discussion
The results of this retrospective study demonstrated that the LAR was an independent factor for all-cause mortality in AP patients at 28-d of hospital admission. Comparison by AUC values showed higher accuracy of LAR (74.262%) than lactate (71.252%) or albumin (65.917%) alone. It even compares favourably with SOFA (75.153%). Meanwhile, Kaplan-Meier survival analysis plots indicate that AP patients with LAR ≥1.1124 had significantly higher all-cause mortality within 28-d admissions than those with LAR < 0.001), as well as subgroup analysis further, supports our view.
In recent years, researchers have focused on developing systemic inflammatory biomarkers to predict the prognosis of patients with AP, including RDW (20), Neutrophil/Lymphocyte Ratio (NLR) (21), RDW/Platelet Ratio (RPR) (22), Glycemic/Lymphocyte Ratio (GLR) (23), C-Reactive Protein/Albumin ratio (24), etc. LAR has been applied as a new predictor for Sepsis, Heart Failure and Acute Respiratory Failure (25–27) with a good prediction of mortality. However, studies using LAR to predict the prognosis of AP patients have not been reported.
Lactate being an important indicator of tissue oxygenation, blood perfusion and metabolism in vivo, our study illustrated that hyperlactatemia is associated with poor prognosis in critically ill patients, which is consistent with previous research findings (9). Wu et al. similarly identified elevated lactate concentration as an independent risk factor for 28-d mortality in patients with SAP (28). However the interpretation of serum lactate levels is often complex, patients with liver disease can have abnormal lactate metabolism. Furthermore, drugs such as salbutamol and metformin may also cause abnormal elevations of lactate, but this result was not reflected in our study. In addition, some critically ill patients may instead have low lactate levels in venous blood, which reduces the reliability of using lactate alone to predict patient prognosis (29).
The pathogenesis of AP is intimately related to oxidative stress, as activation of the inflammatory response and recruitment of inflammatory cells lead to tissue damage in patients (30). In turn, albumin increases the production of several anti-inflammatory substances such as lipoxins, lysins and protectins to promote wound healing (27), that process consumes large amounts of albumin which could explain the poor prognosis due to reduced albumin levels. It has been reported that serum albumin is a strong predictor of mortality in elderly patients with sepsis (31). However, serum albumin levels are affected by chronic disease, nutritional support and inflammation, which may have limited prognostic value in a single measurement. Now, we make a ratio of blood lactate and serum albumin to predict more accurately the prognosis of AP patients by reducing the effect of a single factor on the regulatory mechanism through the inverse change caused by two different mechanisms (32).
Previously, Cakir et al. (including 1136 patients with an AUC of 0.869 for LAR, 0.816 for lactate, and 0.812 for albumin) results for sepsis studies showed that LAR was a better predictor of in-hospital mortality than the use of lactate or albumin alone (25). Moreover, Shin et al. (including 946 patients) confirmed that LAR was more accurate in predicting mortality in critically ill septic patients at 28-d of admission (15). And these conclusions are in agreement with the outcomes of our study. Thus, monitoring LAR can help to better manage patients with AP in clinical practice.
When patients with AP progress to severe acute pancreatitis (SAP), they will develop hypotension, multi-organ hypoperfusion failure, and adult respiratory distress syndrome due to the activation of the pro-inflammatory cascade response, which is its clinical manifestations similar to those of patients with infectious shock. To some extent, acute pancreatitis can be used as a model for sepsis (33). We included 49% of patients with concurrent sepsis in our study, while the number of patients who died within 28-d of admission was as high as 60.4%. By comparing the optimal cut-off values, our study LAR optimal cut-off value was 1.1124 (because our study albumin units were different), while the Chebl et al. study LAR optimal cut-off value was 0.115, which was nearly the same as our study (29). However, for different etiologies of admission, the optimal cut-off value still needs to be determined by prospective studies.
Of course, there are still some limitations to our study. First, our study is a single-center retrospective cohort study, which cannot elucidate the relationship between LAR and AP as prospective studies do, to the extent that our findings lack some persuasive power. Second, we studied the relationship between LAR and prognosis measured for the first time after hospital admission, which does not allow us to assess the prognostic impact of dynamic LAR. Finally, the population data for our study were from MIMIC-IV (v1.0), which was covering hospitalized patients between 2008-2019. As medical treatment has evolved and therapy regimens have been optimized, such a wide period does not guarantee consistency in treatment regimens for patients admitted to the hospital around that time, which we were unable to determine whether this would have biased the study results.
5 Conclusion
In our study, LAR could be used as an independent predictor of all-cause mortality in AP patients within 28-d of admission, which performed better prognostically than blood lactate or serum albumin alone, and was not inferior to SOFA. This will provide healthcare workers with a better tool for timely early intervention and clinic planning for poor patient outcomes. further validation of LAR as a readily available and objective biomarker is still needed in large-scale multicenter prospective studies.
Data availability statement
The datasets presented in this study can be found in online repositories. The names of the repository/repositories and accession number(s) can be found in the article/supplementary material.
Ethics statement
The studies involving human participants were reviewed and approved by the Institutional Review Board of the Massachusetts Institute of Technology and Beth Israel Deaconess Medical Center. Written informed consent for participation was not required for this study in accordance with the national legislation and the institutional requirements.
Author contributions
QL, DL, and HZ were jointly responsible for the conception, design, data collection, data analysis, and editing of the manuscript. MW and JY performed the data processing and data code review. QL and HZ drafted the manuscript. MW and QL reviewed the statistical analysis and literature search. QW and SY revised the manuscript and double-checked the statistical analysis results. All authors made substantial contributions and approved the content of the manuscript.
Funding
Natural Science Research Project of Anhui Educational Committee, No. KJ2021A0798.
Acknowledgments
We are very grateful for the contributions of our colleagues in the Department of Gastroenterology of the First Affiliated Hospital of Bengbu Medical College. We are also very grateful to the Physionet website for providing the MIMIC-IV database, and to Beth Israel Deaconess Medical Center for providing patient consultation data as well as the MIT Computational Physiology Laboratory team for developing and maintaining the database.
Conflict of interest
The authors declare that the research was conducted in the absence of any commercial or financial relationships that could be construed as a potential conflict of interest.
Publisher’s note
All claims expressed in this article are solely those of the authors and do not necessarily represent those of their affiliated organizations, or those of the publisher, the editors and the reviewers. Any product that may be evaluated in this article, or claim that may be made by its manufacturer, is not guaranteed or endorsed by the publisher.
Supplementary material
The Supplementary Material for this article can be found online at: https://www.frontiersin.org/articles/10.3389/fimmu.2022.1076121/full#supplementary-material
References
1. Goyal H, Awad H, Hu ZD. Prognostic value of admission red blood cell distribution width in acute pancreatitis: A systematic review. Ann Transl Med (2017) 5(17):342. doi: 10.21037/atm.2017.06.61
2. Vege SS, DiMagno MJ, Forsmark CE, Martel M, Barkun AN. Initial medical treatment of acute pancreatitis: American gastroenterological association institute technical review. Gastroenterology (2018) 154(4):1103–39. doi: 10.1053/j.gastro.2018.01.031
3. Baron TH, DiMaio CJ, Wang AY, Morgan KA. American Gastroenterological association clinical practice update: Management of pancreatic necrosis. Gastroenterology (2020) 158(1):67–75.e1. doi: 10.1053/j.gastro.2019.07.064
4. Schepers NJ, Bakker OJ, Besselink MG, Ahmed Ali U, Bollen TL, Gooszen HG, et al. Impact of characteristics of organ failure and infected necrosis on mortality in necrotising pancreatitis. Gut (2019) 68(6):1044–51. doi: 10.1136/gutjnl-2017-314657
5. Bang JY, Wilcox CM, Arnoletti JP, Varadarajulu S. Superiority of endoscopic interventions over minimally invasive surgery for infected necrotizing pancreatitis: Meta-analysis of randomized trials. Dig Endosc (2020) 32(3):298–308. doi: 10.1111/den.13470
6. Staubli SM, Oertli D, Nebiker CA. Assessing the severity of acute pancreatitis (Asap) in Switzerland: A nationwide survey on severity assessment in daily clinical practice. Pancreatology (2017) 17(3):356–63. doi: 10.1016/j.pan.2017.02.006
7. Xiao B, Xu HB, Jiang ZQ, Zhang J, Zhang XM. Current concepts for the diagnosis of acute pancreatitis by multiparametric magnetic resonance imaging. Quant Imaging Med Surg (2019) 9(12):1973–85. doi: 10.21037/qims.2019.11.10
8. Arif A, Jaleel F, Rashid K. Accuracy of bisap score in prediction of severe acute pancreatitis. Pak J Med Sci (2019) 35(4):1008–12. doi: 10.12669/pjms.35.4.1286
9. Haas SA, Lange T, Saugel B, Petzoldt M, Fuhrmann V, Metschke M, et al. Severe hyperlactatemia, lactate clearance and mortality in unselected critically ill patients. Intensive Care Med (2016) 42(2):202–10. doi: 10.1007/s00134-015-4127-0
10. Doenyas-Barak K, Beberashvili I, Marcus R, Efrati S. Lactic acidosis and severe septic shock in metformin users: A cohort study. Crit Care (2016) 20:10. doi: 10.1186/s13054-015-1180-6
11. Katopodis P, Pappas EM, Katopodis KP. Acid-base abnormalities and liver dysfunction. Ann Hepatol (2022) 27(2):100675. doi: 10.1016/j.aohep.2022.100675
12. Park J, Hwang SY, Jo IJ, Jeon K, Suh GY, Lee TR, et al. Impact of metformin use on lactate kinetics in patients with severe sepsis and septic shock. Shock (2017) 47(5):582–7. doi: 10.1097/shk.0000000000000782
13. Artigas A, Wernerman J, Arroyo V, Vincent JL, Levy M. Role of albumin in diseases associated with severe systemic inflammation: Pathophysiologic and clinical evidence in sepsis and in decompensated cirrhosis. J Crit Care (2016) 33:62–70. doi: 10.1016/j.jcrc.2015.12.019
14. Goh SL, De Silva RP, Dhital K, Gett RM. Is low serum albumin associated with postoperative complications in patients undergoing oesophagectomy for oesophageal malignancies? Interact Cardiovasc Thorac Surg (2015) 20(1):107–13. doi: 10.1093/icvts/ivu324
15. Shin J, Hwang SY, Jo IJ, Kim WY, Ryoo SM, Kang GH, et al. Prognostic value of the Lactate/Albumin ratio for predicting 28-day mortality in critically ill sepsis patients. Shock (2018) 50(5):545–50. doi: 10.1097/shk.0000000000001128
16. Wang B, Chen G, Cao Y, Xue J, Li J, Wu Y. Correlation of Lactate/Albumin ratio level to organ failure and mortality in severe sepsis and septic shock. J Crit Care (2015) 30(2):271–5. doi: 10.1016/j.jcrc.2014.10.030
17. Lichtenauer M, Wernly B, Ohnewein B, Franz M, Kabisch B, Muessig J, et al. The Lactate/Albumin ratio: A valuable tool for risk stratification in septic patients admitted to ICU. Int J Mol Sci (2017) 18(9):1893. doi: 10.3390/ijms18091893
18. Kong T, Chung SP, Lee HS, Kim S, Lee J, Hwang SO, et al. The prognostic usefulness of the Lactate/Albumin ratio for predicting clinical outcomes in out-of-Hospital cardiac arrest: A prospective, multicenter observational study (Kocarc) study. Shock (2020) 53(4):442–51. doi: 10.1097/shk.0000000000001405
19. Austin PC, White IR, Lee DS, van Buuren S. Missing data in clinical research: A tutorial on multiple imputation. Can J Cardiol (2021) 37(9):1322–31. doi: 10.1016/j.cjca.2020.11.010
20. Zhang T, Liu H, Wang D, Zong P, Guo C, Wang F, et al. Predicting the severity of acute pancreatitis with red cell distribution width at early admission stage. Shock (2018) 49(5):551–5. doi: 10.1097/shk.0000000000000982
21. Kong W, He Y, Bao H, Zhang W, Wang X. Diagnostic value of neutrophil-lymphocyte ratio for predicting the severity of acute pancreatitis: A meta-analysis. Dis Markers (2020) 2020:9731854. doi: 10.1155/2020/9731854
22. Cetinkaya E, Senol K, Saylam B, Tez M. Red cell distribution width to platelet ratio: New and promising prognostic marker in acute pancreatitis. World J Gastroenterol (2014) 20(39):14450–4. doi: 10.3748/wjg.v20.i39.14450
23. Chen Y, Tang S, Wang Y. Prognostic value of glucose-to-Lymphocyte ratio in critically ill patients with acute pancreatitis. Int J Gen Med (2021) 14:5449–60. doi: 10.2147/ijgm.S327123
24. Kaplan M, Ates I, Akpinar MY, Yuksel M, Kuzu UB, Kacar S, et al. Predictive value of c-reactive Protein/Albumin ratio in acute pancreatitis. Hepatob Pancreat Dis Int (2017) 16(4):424–30. doi: 10.1016/s1499-3872(17)60007-9
25. Cakir E, Turan IO. Lactate/Albumin ratio is more effective than lactate or albumin alone in predicting clinical outcomes in intensive care patients with sepsis. Scand J Clin Lab Invest (2021) 81(3):225–9. doi: 10.1080/00365513.2021.1901306
26. Guo W, Zhao L, Zhao H, Zeng F, Peng C, Guo W, et al. The value of Lactate/Albumin ratio for predicting the clinical outcomes of critically ill patients with heart failure. Ann Transl Med (2021) 9(2):118. doi: 10.21037/atm-20-4519
27. Lu Y, Guo H, Chen X, Zhang Q. Association between Lactate/Albumin ratio and all-cause mortality in patients with acute respiratory failure: A retrospective analysis. PloS One (2021) 16(8):e0255744. doi: 10.1371/journal.pone.0255744
28. Wu M, Shi L, Zhang H, Liu H, Liu Y, Zhang W. Predictive value of arterial blood lactic acid concentration on the risk of all-cause death within 28 days of admission in patients with severe acute pancreatitis. Postgrad Med (2022) 134(2):210–6. doi: 10.1080/00325481.2022.2027189
29. Bou Chebl R, Geha M, Assaf M, Kattouf N, Haidar S, Abdeldaem K, et al. The prognostic value of the Lactate/Albumin ratio for predicting mortality in septic patients presenting to the emergency department: A prospective study. Ann Med (2021) 53(1):2268–77. doi: 10.1080/07853890.2021.2009125
30. Xu X, Ai F, Huang M. Deceased serum bilirubin and albumin levels in the assessment of severity and mortality in patients with acute pancreatitis. Int J Med Sci (2020) 17(17):2685–95. doi: 10.7150/ijms.49606
31. Arnau-Barrés I, Güerri-Fernández R, Luque S, Sorli L, Vázquez O, Miralles R. Serum albumin is a strong predictor of sepsis outcome in elderly patients. Eur J Clin Microbiol Infect Dis (2019) 38(4):743–6. doi: 10.1007/s10096-019-03478-2
32. Gharipour A, Razavi R, Gharipour M, Mukasa D. Lactate/Albumin ratio: An early prognostic marker in critically ill patients. Am J Emerg Med (2020) 38(10):2088–95. doi: 10.1016/j.ajem.2020.06.067
Keywords: lactate/albumin ratio, acute pancreatitis, all-cause mortality, 28-days, prognosis, cohort
Citation: Liu Q, Zheng H-L, Wu M-M, Wang Q-Z, Yan S-J, Wang M, Yu J-J and Li D-P (2022) Association between lactate-to-albumin ratio and 28-days all-cause mortality in patients with acute pancreatitis: A retrospective analysis of the MIMIC-IV database. Front. Immunol. 13:1076121. doi: 10.3389/fimmu.2022.1076121
Received: 21 October 2022; Accepted: 28 November 2022;
Published: 14 December 2022.
Edited by:
Andrea Baragetti, University of Milan, ItalyReviewed by:
Antonina Giammanco, University of Palermo, ItalyMuntazir Mushtaq, National Bureau of Plant Genetic Resources (ICAR), India
Copyright © 2022 Liu, Zheng, Wu, Wang, Yan, Wang, Yu and Li. This is an open-access article distributed under the terms of the Creative Commons Attribution License (CC BY). The use, distribution or reproduction in other forums is permitted, provided the original author(s) and the copyright owner(s) are credited and that the original publication in this journal is cited, in accordance with accepted academic practice. No use, distribution or reproduction is permitted which does not comply with these terms.
*Correspondence: Da-Peng Li, byfyldp@126.com
†These authors have contributed equally to this work and share first authorship