- 1Department of General Surgery, Shanghai Ninth People’s Hospital Affiliated to Shanghai Jiao Tong University School of Medicine, Shanghai, China
- 2Department of Urology, Shanghai General Hospital, Shanghai Jiao Tong University School of Medicine, Shanghai, China
Background: Hepatocellular carcinoma (HCC) is one of the most common cancers with high mortality in the world. HCC screening and diagnostic models are becoming effective strategies to reduce mortality and improve the overall survival (OS) of patients. Here, we expected to establish an effective novel diagnostic model based on new genes and explore potential drugs for HCC therapy.
Methods: The gene expression data of HCC and normal samples (GSE14811, GSE60502, GSE84402, GSE101685, GSE102079, GSE113996, and GSE45436) were downloaded from the Gene Expression Omnibus (GEO) dataset. Bioinformatics analysis was performed to distinguish two differentially expressed genes (DEGs), diagnostic candidate genes, and functional enrichment pathways. QRT-PCR was used to validate the expression of diagnostic candidate genes. A diagnostic model based on candidate genes was established by an artificial neural network (ANN). Drug sensitivity analysis was used to explore potential drugs for HCC. CCK-8 assay was used to detect the viability of HepG2 under various presentative chemotherapy drugs.
Results: There were 82 DEGs in cancer tissues compared to normal tissue. Protein–protein interaction (PPI), Gene Ontology (GO), and Kyoto Encyclopedia of Genes and Genomes (KEGG) enrichment analyses and infiltrating immune cell analysis were administered and analyzed. Diagnostic-related genes of MT1M, SPINK1, AKR1B10, and SLCO1B3 were selected from DEGs and used to construct a diagnostic model. The receiver operating characteristic (ROC) curves were 0.910 and 0.953 in the training and testing cohorts, respectively. Potential drugs, including vemurafenib, LOXO-101, dabrafenib, selumetinib, Arry-162, and NMS-E628, were found as well. Vemurafenib, dabrafenib, and selumetinib were observed to significantly affect HepG2 cell viability.
Conclusion: The diagnostic model based on the four diagnostic-related genes by the ANN could provide predictive significance for diagnosis of HCC patients, which would be worthy of clinical application. Also, potential chemotherapy drugs might be effective for HCC therapy.
Introduction
Hepatocellular carcinoma (HCC) is an increasingly serious public health problem, and it is gradually becoming one of the main causes of cancer mortality in the world (Villanueva, 2019). As most cases of HCC occur in patients with underlying chronic liver diseases like hepatitis B virus (HBV) infection and varying degrees of cirrhosis, the diagnosis of HCC in humans is challenging (Vogel and Saborowski, 2020; Yang and Heimbach, 2020). Most HCC patients are diagnosed when they have obvious clinical symptoms appear or are at advanced stages of the disease, which reduces or even precludes the effective use of curative therapy (Ayuso et al., 2018; Rastogi, 2018). Therefore, identifying candidate biomarkers and constructing novel diagnostic models could be useful in distinguishing HCC patients from normal people, which would improve the overall survival (OS) of HCC patients.
In the clinic, the detection of serum tumor markers has been widely used for its advantages of the noninvasive method (Han et al., 2020). However, the information provided by the conventional assays for carcinoembryonic antigen (CEA) and carbohydrate antigen 19-9 (CA19-9) is not specific or sensitive enough (Cui et al., 2016; Edoo et al., 2019). Thus, developing novel diagnostic biomarkers is necessary for early detection of HCC. Meanwhile, HCC patients could not get timely treatment because of multidrug resistance or might have suffered from severe drug-related adverse effects from chemotherapy (Zhang et al., 2016). Calling for novel and effective drugs for HCC patients is an eternal theme of the times.
With the improvement of bioinformatics technology, differentially expressed genes (DEGs) by systematic bioinformatics analysis could be employed to select candidate genes and underlying pathways that were related to the occurrence and progression of HCC for diagnosis (Wang et al., 2018; Li et al., 2021). Moreover, an artificial neural network (ANN) is a classic machine learning method, which is often used for modeling construction (Zhong et al., 2019; Mai et al., 2020). In this study, we first retrieved transcriptional expression data of patients with HCC patients from GEO datasets and found 82 DEGs between normal and HCC tissues. Next, the possible functional mechanisms were explored by protein–protein interaction (PPI), Kyoto Encyclopedia of Genes and Genomes (KEGG), and Gene Ontology (GO) enrichment analyses. Then, metallothionein 1M (MT1M), solute carrier organic anion transporter family member 1B3 (SLCO1B3), serine protease inhibitor Kazal type 1 gene (SPINK1), and aldo–keto reductase family 1B10 (AKR1B10) were found and used as candidate biomarkers to construct an artificial neural network (ANN) model. Further validation of diagnostic-related genes was performed by QRT-PCR in HepG2 and HL7702 cell lines. The potential drug for HCC therapy, including vemurafenib, LOXO-101, dabrafenib, selumetinib, Arry-162, and NMS-E628, were found based on four diagnostic-related genes. Vemurafenib, dabrafenib, and selumetinib might have a broad application prospect in HCC.
Methods
Datasets
The RNA microarray data of HCC samples and corresponding normal liver tissue were retrieved from the GEO dataset (https://www.ncbi.nlm.nih.gov/geo/). The datasets of GSE14811, GSE60502, GSE84402, GSE101685, GSE102079, GSE113996, and GSE45436 were downloaded and divided into training and testing cohorts. The “sva” package of R was used to ensure that the GEO datasets were batch effects-corrected before bioinformatics analysis to avoid generating less reliable results (Leek et al., 2012). The first six datasets were classified as training cohorts and used for diagnostic model construction. The latter was classified as a testing cohort and used for validation.
Identification of differentially expressed genes
Normalization of the count matrix was performed with the trimmed mean of the M-values normalization method of the edgeR (R package). The limma R package was used to identify differentially expressed genes (DEGs) in the construction cohort. The screening standards of DEGs for functional enrichment analysis were |log2FC|> 1 and FDR<0.05. The screening standards of DEGs for diagnostic-related genes used for ANN model establishment and drug-sensitive analysis were |log2FC|> 2 and FDR<0.05.
Functional enrichment analysis
For protein–protein interaction (PPI), we used the String (Protein–Protein Interaction Networks, V: 10.5) database (https://string-db.org/). Kyoto Encyclopedia of Genes and Genomes (KEGG) and Gene Ontology (GO) enrichment analyses of the DEGs were performed by using the R clusterProfiler package, including the packages of “GOplot,” “ggplot2,” “stringi,” “colorspace,” and “digest”. Then, the pathway and process enrichment analyses were carried out using Metascape (Metascape, http://metascape.org). As for infiltrating immune cell analysis, the R package of “e1071,” “corrplot,” and “vioplot” were used for analysis and depicting differences. The 22 representative immune cells and gene expressions in every kind of immune cell to distinguish immune cells from each other are shown in Supplementary Table S1.
Construction and validation of the diagnostic model
The artificial neural network (ANN) classifier was a feed-forward neural network with three layers, which included input nodes, a hidden layer, and output nodes. The multi-layer perceptron method was incorporated, and training of the network was based on the feed-forward back propagation method to adjust the internal factors of the network, which reduced the overall errors during the repeated development cycles. The ANN model learned to connect the relations between the input and output layers by adjusting the weights and biases of the hidden layer. Here, we used the training cohort (GSE14811, GSE60502, GSE84402, GSE101685, GSE102079, and GSE113996) to establish the ANN diagnostic model, and the testing cohort (GSE45436) was used as the validation one. There were 221 normal tissues and 284 HCC tissues in the training cohort, while 41 normal tissues and 93 HCC tissues were in the testing cohort.
Cells and cell culture
The HepG2 cell line (Human HCC) and HL7702 (human normal cells) were purchased from the Chinese Academy of Medical Sciences (Beijing, China). The HepG2 cells were grown in Dulbecco’s modified Eagle’s medium (DMEM) supplemented with 10% fetal bovine serum (Life Technologies, Inc., Carlsbad, CA, United States), 1 mM sodium pyruvate, 0.1 mM non-essential amino acids, and 2 mM l-glutamine at 37°C and in 5% CO2 in a humidified incubator. The HL7702 cells were cultured in RPMI-1640 medium (Gibco, Rockville, MD, United States) supplemented with 10% Fetal Bovine Serum (Life Technologies, Inc., Carlsbad, CA, United States) at 37°C in a humidified 5% CO2 atmosphere.
Quantitative real-time PCR
Here, we used HepG2 and HL7702 to verify the expression of diagnostic genes. The total RNA was isolated by TRIzol reagent (Life Technologies Corporation, Carlsbad, CA, United States) under the manufacturer’s s directions. Then, 0.8 μg mRNA was used for synthesis of 20 μL cDNA using Superscript II reverse transcriptase and random hexamers (Invitrogen, Carlsbad, CA, United States). Q-RT PCR was further performed on an ABI PRISM 7300 Sequence Detection System with SYBR Green PCR Master Mix (Applied Biosystems). The primers used in this study were MT1M (forward 5′-ATTGAATTCGGATGGACCCCAACTGCTC-3′, reverse 5′-ATTCTCGAGTCAGGCACAGCAGCTG-3′), SLCO1B3(forward 5′-TCATAAACTCTTTGTTCTCTGCAA-3′, reverse 5′-GTTGGCAGGCATTGTCTTG-3′), SPINK1 (forward 5′-AACACTGGAGCTGACTCCCT-3′, reverse 5′-ATCAGTCCCACAGACAGGGT-3′), and AKR1B10 (forward 5′-CATATCCAGAGGAATGTGATTGTCA-3′, reverse 5′- AGACCTGAATGTTCTCAACAATGC-3′). GAPDH was the internal comparison. The mRNA expression of relative genes was calculated using the 2−ΔΔCt method with normalization to GAPDH expression.
Drug-sensitive analysis of the gene signatures
The transcriptional expression of NCI-60 human cancer cell lines was downloaded from the CellMiner project page (https://discover.nci.nih.gov/cellminer). Pearson’s correlation analysis was performed to determine the association between diagnostic genes and drug sensitivity.
Cell counting kit-8 (CCK-8 assay)
CCK-8 assay was used to detect the viability of HepG2 under various presentative chemotherapy drugs. The cells were resuspended, seeded in a 96-well plate (6 × 104 cells/well), cultured at an appropriate environment (37°C, 5% CO2.), and continually incubated for 2 h with 10 ul CCK-8 solution (Yeasen, Shanghai, China) added to each well. The absorbance of each well was measured at 450 nm and tested by a microplate reader (Bio-Rad, Hercules, CA). The calculation of cell viability was processed as follows: (Experimental group - blank control)/(Negative control - blank control) ×100%. The blank groups contained DMEM medium only, while the negative groups were set up with HepG2 and HL7702 cultured in DMEM-F12 without drugs. Vemurafenib (S1267) and Selumetinib (S1008) were obtained from Selleck Chemicals (Houston, TX, United States). Dabrafenib was purchased from Merck (Kenilworth, NJ, United States). Binimetinib and larotrectinib were provided by Med Chem Express (Shanghai, China).
Results
Identification of DEGs
A total of 221 normal samples and 284 tumor samples obtained from the GEO dataset formed the training cohort and participated in the identification of DEGs. The differential expression of 82 genes between the normal and HCC samples was identified by building a difference comparison matrix. A heatmap and volcano map showed 47 downregulated genes and 35 upregulated genes (Figures 1A and B). The protein–protein interaction (PPI) network and co-expression among these genes are presented in Figure 1C.
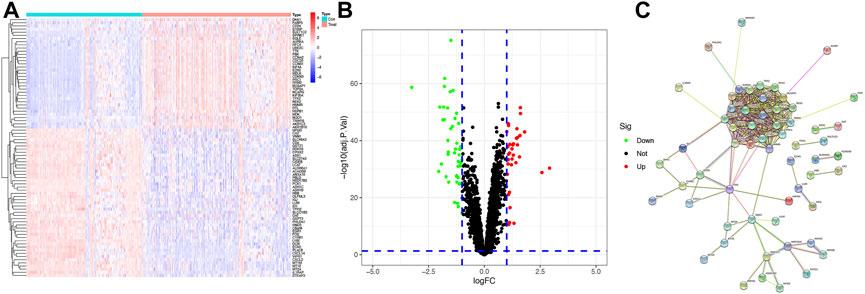
FIGURE1. Identification of candidate genes (A) Heatmap of differential gene expression between normal and HCC samples in the training cohort, log2FC = 1. (B) Volcano map of differential gene expression between normal and HCC samples in the training cohort. (C) PPI network between these genes (cutoff = 0.4).
Functional enrichment and pathway analyses
KEGG and GO function enrichment analyses were performed here. As for GO function analysis, three GO terms were selected: molecular function (MF), cellular component (CC), and biological process (BP). Expression analysis showed that DEGs had the most uniquely enriched terms for organelle fission, (mitotic) nuclear division, and spindle, which were related to cell proliferation, cell division, and cell cycling. The processes mentioned were substantially over-represented during cancer transformation. Also, “oxidoreductase activity, acting on the CH–CH group of donors” was enriched in the list (Figure 2A). In Figures 2B, C, several up-regulated DEGs were mainly enriched in processes of “nuclear division,” “organelle fission,” etc., while down-regulated ones were mainly gathered in “response to toxic substance”.
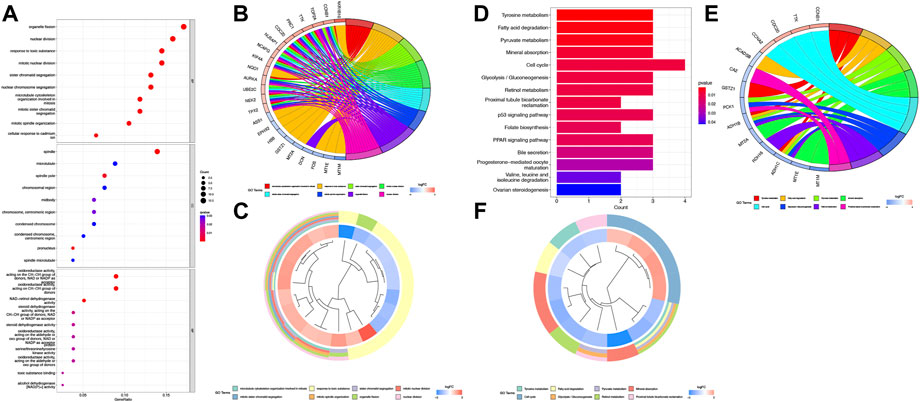
FIGURE 2. Function analysis based on the DEGs (A) Heatmap of GO analysis. (B) Circos plot of GO terms based on DEGs. (C) Cluster profiler analysis of the GO based on DEGs. (D) Barplot of KEGG terms based on DEGs. (E) Circos plot of KEGG terms based on DEGs. (F) Cluster profiler analysis of the KEGG based on DEGs.
Furthermore, KEGG pathway enrichment analysis indicated that DEGs were significantly enriched in “Cell cycle”, “Tyrosine metabolism”, etc (Figure 2D). In Figures 2E and F, upregulated DEGs were mainly enriched in the “Cell cycle” while downregulated DEGs were gathered in several cancer-related metabolism pathways.
To further validate and organize the results of KEGG and GO function, the DEGs were functionally annotated using Metascape. Metascape analysis showed the top 20 clusters of enriched biological processes like “mitotic cell cycle process,” “response to toxic substance,” etc (Figures 3A and B).
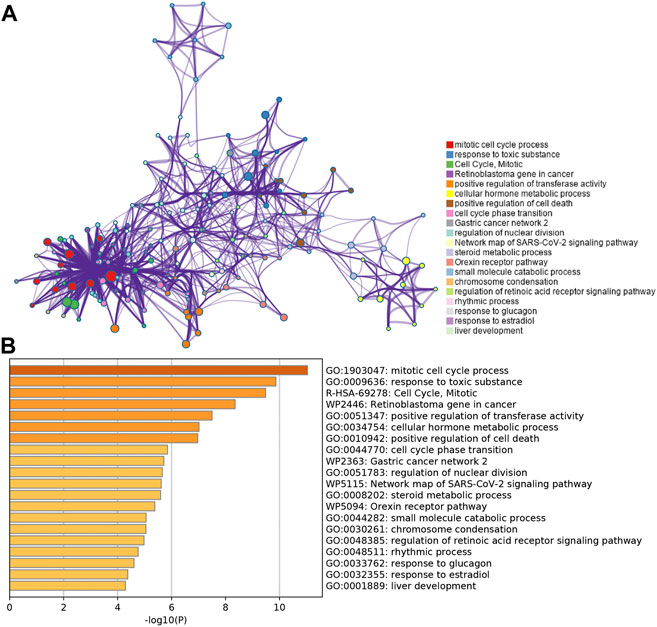
FIGURE 3. Functional pathway analysis using Metascape. (A) Network of enriched terms colored by the cluster identified in DEGs using the Metascape tool. (B) Top 20 clusters of enriched biological processes identified in DEGs using the Metascape tool.
Identification and validation of diagnostic-related genes
Further comparison analysis between liver tissues from HCC and normal samples was identified by improving the screening criteria of logFC into |log2FC|>2, and we got four genes as candidate genes (Supplementary Figure S1). Next, a random forest analysis was performed, and four diagnostic-related genes (MT1M, SPINK1, AKR1B10, and SLCO1B3) were ensured, which showed that two genes were upregulated and two genes were downregulated (Figures 4A, B). The mean decrease in the Gini coefficient was a measure of how each variable contributed to the homogeneity of the nodes in the resulting random forest. The values were all over 40, which meant the four genes were of great importance in the development of the ANN model (Figure 4B). The diagnostic-related genes identified were shown in heatmap and could divide the training cohort into two groups (Figure 4C). Further validation of the diagnostic-related genes was performed by QRT-PCR (Figure 4D).
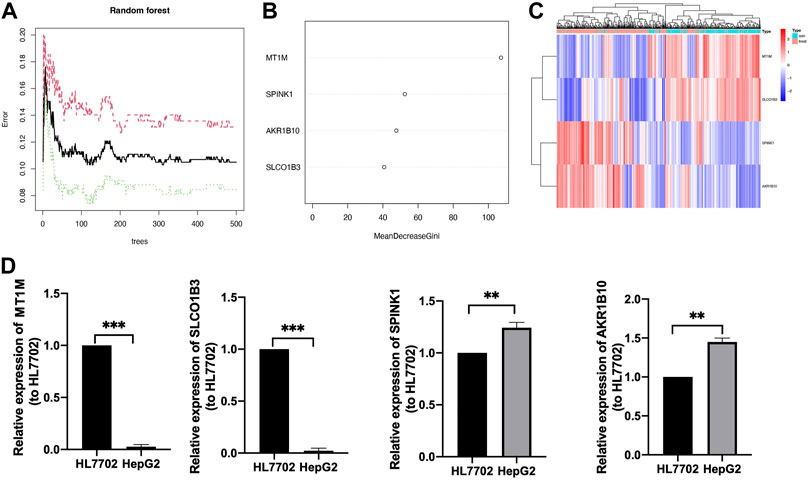
FIGURE 4. Identification of target genes. (A) Decision tree random forest tree. (B) Mean decrease in Gini coefficient of four target genes. (C) Heatmap of diagnostic candidate DEGs (D) QRT-PCR of four target genes, **p < 0.01, ***p < 0.001.
Construction and validation of the diagnostic model built on an artificial neural network
We calculated the risk gene scores of the four genes in each sample to get the median cutoff value and defined the upregulated gene as “1” and the downregulated gene as “0” in each sample in the training and testing cohorts (Supplementary Table S2). The approximate ratio of the sample number in the training cohort (Normal:221; HCC:284) and the testing cohort (Normal:41; HCC:93) is 4 to 1. Then, the ANN method was performed to construct a diagnostic model based on gene scores of MT1M, SPINK1, AKR1B10, and SLCO1B3 in each sample. The ANN model included three layers (input, hidden, and output) in which the number of nodes in the input layer and output layer was equal to 4 (number of input genes) and 2 (control and treatment), respectively (Figure 5A). An ROC curve was performed to detect whether the model could distinguish the HCC sample from the normal sample, and the area under the curve (AUC) was 0.910 (Figure 5B). In addition, the model worked well in the testing cohort as the AUC of ROC was 0.953 (Figure 5C).
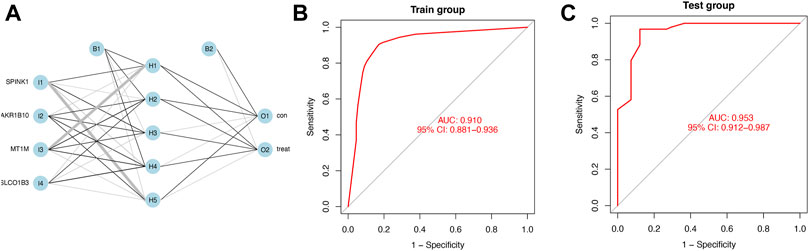
FIGURE 5. Diagnostic model constructed by the ANN (A) Schematic representation of the ANN model developed to predict the risk of HCC and normal samples. Thin lines represented synaptic weight <0; the thicker lines represented synaptic weight >0. (B) AUC of ROC curves verified the diagnostic performance of the ANN model in the training cohort. (C) AUC of ROC curves verified the diagnostic performance of the ANN model in the testing cohort.
Infiltrating immune cell analysis
The relative percent of 22 representative immune cells in the normal and HCC samples is presented in Figure 6A to show the approximate change in the proportion of immune cells in the training cohort. In Figure 6B, only “T cells CD4 naive”, “Macrophages M0”, “Macrophages M1”, and “Macrophages M2” displayed a substantial difference between the two groups (Figure 6B). “Macrophages M1” was the most significant one (p < 0.001) compared to “T cells CD4 naïve” (p = 0.021), “Macrophages M0” (p = 0.038), and “Macrophages M2” (p = 0.017). Furthermore, Macrophages M2 showed a significant negative correlation with Macrophages M1 and M0, while Macrophages M0 and M2 were both upregulated in the HCC group (Figures 6B, C).
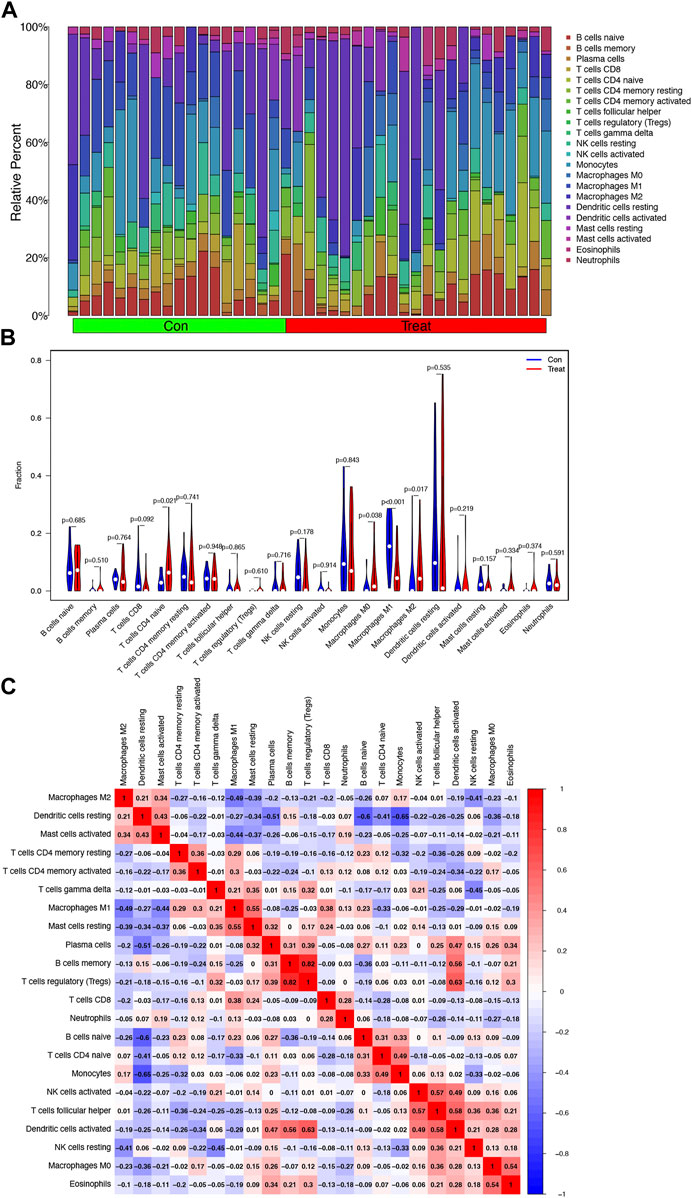
FIGURE 6. Analysis of infiltrating immune cells in the training cohort. (A) Heatmap of relative fraction of 22 representative immune cell population in the Con (normal) and Treat (HCC) cohorts was displayed. (B) Boxplots showed the cores of 22 immune cells between the Con (normal) and Treat (tumor) cohorts. (C) Heatmap of correlation between 22 immune cells.
The sensitivity of diagnostic-related gene expression to presentative chemotherapy drug
With the help of NCI-60, a public database of human cancer cell lines, we determined the relationship between these diagnostic genes and drug sensitivity and showed the top 16 correlation analyses according to the p-value. Figure 7 demonstrated that SPINK1 was sensitive to vemurafenib, dabrafenib, selumetinib, ARRY-162 (binimetinib) (p < 0.001), and SLCO1B3 was sensitive to LOXO-101(larotrectinib) (p < 0.001) and NMS-E628 (p = 0.002), while it was insensitive to arsenic trioxide. In addition, the expression of MT1M was insensitive to vinblastine, paclitaxel, and tyrothricin (p < 0.001). Moreover, AKR1B10 was insensitive to arsenic trioxide (p = 0.001).
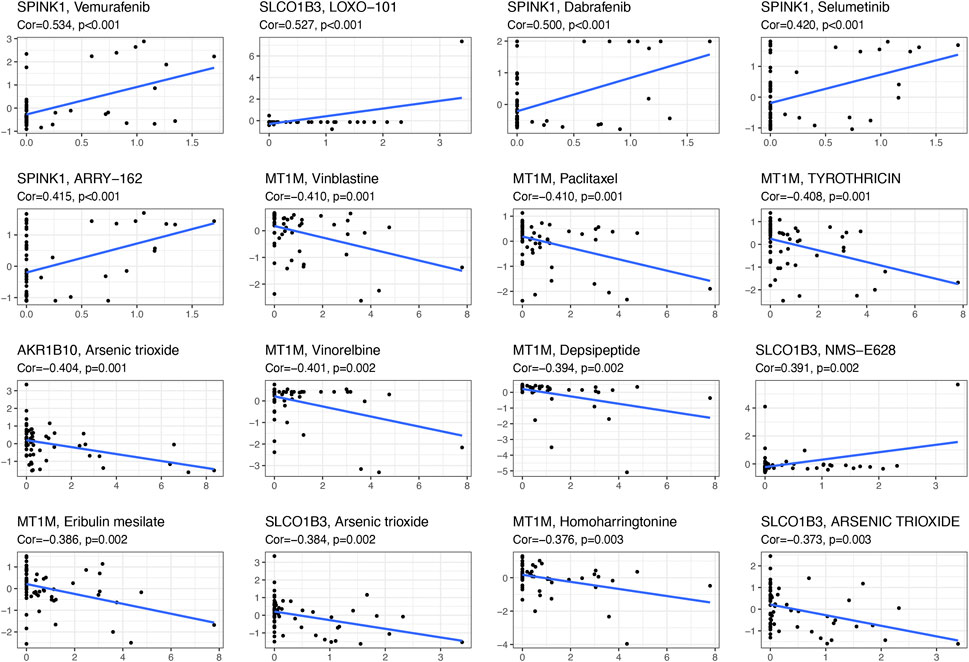
FIGURE 7. Scatter plot of the relationship between diagnostic-related gene expression and drug sensitivity.
The cell viability under various presentative chemotherapy agents
Here, we further validate the HepG2 under various presentative chemotherapy drugs, including vemurafenib, dabrafenib, selumetinib, binimetinib, and larotrectinib, for they were sensitive to SPINK1 and SLCO1B3. We found that the former three kinds of drugs could significantly inhibit the cell viability of HepG2, while the latter two could also work slightly without significant differences observed (Figure 8).
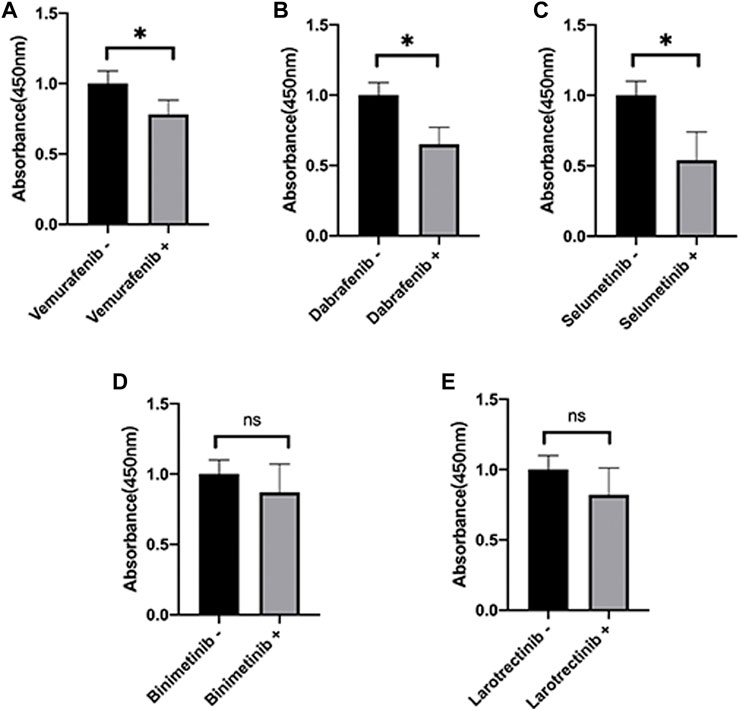
FIGURE 8. Cell viability of HepG2 under various presentative chemotherapy agent treatment in 24 h. The absorbance of HepG2 under treatment of (A) vemurafenib (5 μM), (B) dabrafenib (5 μM), (C) selumetinib (5 μM), (D) binimetinib (5 μM), and (E) larotrectinib (5 μM). *p < 0.05, ns = not significant.
Discussion
HCC is one of the most widespread problems facing by society today, which still has a high mortality rate in China (Xie et al., 20192020). Although comprehensive treatments have been adopted, HCC is associated with poor OS due to late diagnosis and high metastasis rate (Liu et al., 2020; Zhang et al., 2020). Early diagnosis, reasonable assessment of prognosis, and timely intervention are important for HCC patients, which encouraged us to explore better relevant biomarkers and diagnostic models (Beumer et al., 2021). Advancing molecular biology research methods brought diagnostic evaluation based on new types of biomarkers into reality. In this study, we observed 82 DEGs between HCC and normal samples. Based on DEGs, we explored the functional enrichment and pathway analyses and found that they were likely to be involved in mitosis and oxidative stress, which is consistent with the current latest research about cancer proliferation, metastasis, and treatment resistance. Moreover, in the infiltrating immune cell analysis, the unbalance of Macrophage M1/M2 was observed in this study. Macrophage M1 exerted cytotoxic function and eliminated early HCC, while macrophage M2 exerted anti-inflammatory activities and promoted cancer cell proliferation and invasion (Tian et al., 2019). However, Macrophage M0 and Naive T cell amounts were upregulated in the HCC cohort, while not all of them would differentiate to maturity and interfere with HCC proliferation or immigration. Thus, increasing the ratio of M1/M2 and the number of mature T cells might be a potential treatment for HCC (Dou et al., 2019; Yan et al., 2021).
To better select the candidate gene from DEGs for diagnostic model construction, we employed a random forest algorithm and found MT1M, SLCO1B3, SPINK1, and AKR1B10 were the chosen ones. The mean decrease in the Gini coefficient of the four target genes was all above 40, which meant they had obvious specificity in DEGs. MT1M, SLCO1B3, SPINK1, and AKR1B10 were cancer-related genes that were associated with different human diseases, especially in HCC. The specific biological functions of the four diagnostic-related genes (MT1M, SLCO1B3, SPINK1, and AKR1B10) in HCC in the recent 10 years are presented in Table 1.
In this study, we found the four target genes could divide the training cohort into two groups and have the same trend as in previous research. We also confirmed the expression trend of the four genes in HepG2 and HL7702 cell lines by QRT-PCR. Based on the four diagnostic-related genes, the ANN diagnostic model was developed and validated in GEO datasets. The ANN model obtained the highest prediction performance and has been widely used in various diseases to predict the population with high risk (Kourou et al., 2015; Zhong et al., 2019; Li et al., 2020). Based on the four diagnostic candidate genes, we successfully established a diagnostic model as for AUC of ROC was 0.910 and 0.953 in the training and testing cohorts, respectively, which meant it served as a reliable prediction model in our study.
According to the four diagnostic-related genes, we screened the potential drug that has a connection with diagnostic genes by NCI-60. We found that SPINK1 was sensitive to vemurafenib, dabrafenib, selumetinib, and ARRY-162, and SLCO1B3 was sensitive to LOXO-101 and NMS-E628. In addition, we further validated the cell viability of HepG2 under various presentative chemotherapy drugs, including vemurafenib, dabrafenib, selumetinib, binimetinib, and larotrectinib and observed vemurafenib, dabrafenib, and selumetinib might have a broad application prospect in HCC. Vemurafenib was a small-molecule inhibitor of the oncogenic v-raf murine sarcoma viral oncogene homolog B (BRAF) kinase that was used for treatment of melanoma (Hyman et al., 2015). However, increased SPINK1 secretion was reported to be related to vemurafenib resistance in BRAF V600E-mutant colorectal cancers, indicating the need to target different gene variant subtypes of HCC during chemotherapy (29193645). Vemurafenib was also noticed to be the substrate of SLCO1B3, which might influence the absorption and elimination of the HCC chemotherapy drug (23340295). Nevertheless, BRAF gene polymorphisms were associated with capsule formation in HCC (Sun et al., 2021). BRAF-mutation-mediated MAPK pathway downstream was often constitutively activated and led to cancer cell differentiation, proliferation, angiogenesis, and anti-apoptosis, suggesting targeting the BRAF pathway might inhibit HCC progression in the future (Gnoni et al., 2019). Dabrafenib was also a selective inhibitor of BRAF kinase for patients suffering from BRAF-mutated melanoma, advanced non-small cell lung cancer, and anaplastic thyroid cancer harboring the BRAFV600E mutation (Puszkiel et al., 2019). Until now, no association between dabrafenib and expression of SPINK1 was reported in cancer treatment. Also, dabrafenib was found to inhibit the activation of OATP1B3 (SLCO1B3), which might contribute to increasing OATP1B3-substrate-sensitive drug during the absorption phase (Nebot et al., 2021). Considering the pharmacological mechanisms of dabrafenib and vemurafenib were similar, we also expected that dabrafenib might have prospects in the treatment of HCC. Selumetinib was a mitogen-activated protein kinase 1 and 2 (MEK1/2) inhibitor for treatment of neurofibromatosis, pediatric low-grade glioma, non-small cell lung cancer, and melanoma (Campagne et al., 2021). Selumetinib could be delivered by a novel delivery nanosystem in HCC and showed a well-targeted therapeutic strategy for HCC (Farinha et al., 2021). In addition, a combination of sorafenib and selumetinib could inhibit the growth of naïve and sorafenib-resistant HCC tumors via suppression of β-catenin signaling (Huynh et al., 2019). No specific research reported the direct connection among selumetinib, SPINK1, and SLCO1B3. However, SPINK1 promoted HCC metastasis via the MEK/ERK signaling pathway (Ying et al., 2017). Similar research also reported that SPINK1 expression in HCC cells was associated with HCC via activating the c-Raf/MEK/ERK pathway, which suggested that inhibiting the MEK pathway and usage of selumetinib could be a potential treatment strategy for HCC(37). In addition, the potential chemotherapy drug mentioned above mainly participated in cell proliferation, cell division, and cell cycling, which was consistent with our functional analysis and has not been fully used for HCC treatment in clinics (Davies et al., 2002; Yuan et al., 2020). Moreover, combined inhibition of BRAF and CSF-1R, which recruits M2-polarized macrophages in a tumor, resulted in superior antitumor responses (Mok et al., 2015). Although the potential drugs for chemotherapy were with broad application foreground, the diagnostic genes not only could enhance the drug sensitivity but also increased the resistance of chemotherapy drugs approved by the Food and Drug Administration (FDA). Thus, further research is needed for accurate application of these drugs in HCC.
Conclusion
In conclusion, we constructed a novel diagnostic model based on four genes by the ANN model to predict the diagnosis of HCC patients. The model could provide useful insights into the potential prediction of HCC diagnosis. Several potential chemotherapy drugs came into view, although further research is required.
Data availability statement
The original contributions presented in the study are included in the article/Supplementary Material; further inquiries can be directed to the corresponding authors.
Author contributions
MC: writing—original draft. G-BW: writing—reviewing and editing. Z-WX: data curation. D-LS: supervision. ML: funding acquisition.
Funding
This work was supported by The National Natural Science Foundation of China (Nos. 81771564, 81901463, and 81970526).
Conflict of interest
The authors declare that the research was conducted in the absence of any commercial or financial relationships that could be construed as a potential conflict of interest.
Publisher’s note
All claims expressed in this article are solely those of the authors and do not necessarily represent those of their affiliated organizations, or those of the publisher, the editors, and the reviewers. Any product that may be evaluated in this article, or claim that may be made by its manufacturer, is not guaranteed or endorsed by the publisher.
Supplementary material
The Supplementary Material for this article can be found online at: https://www.frontiersin.org/articles/10.3389/fgene.2022.942166/full#supplementary-material
References
Atyah, M., Yin, Y. R., Zhou, C. H., Zhou, Q., Chen, W. Y., Dong, Q. Z., et al. (2018). Integrated analysis of the impact of age on genetic and clinical aspects of hepatocellular carcinoma. Aging (Albany NY) 10 (8), 2079–2097. PubMed PMID: 30125264; PubMed Central PMCID: PMCPMC6128442. doi:10.18632/aging.101531
Ayuso, C., Rimola, J., Vilana, R., Burrel, M., Darnell, A., Garcia-Criado, A., et al. (2018). Diagnosis and staging of hepatocellular carcinoma (HCC): Current guidelines. Eur. J. Radiol. 101, 72–81. PubMed PMID: 29571804. doi:10.1016/j.ejrad.2018.01.025
Beumer, B. R., Buettner, S., Galjart, B., van Vugt, J. L. A., de Man, R. A., Ijzermans, J. N. M., et al. (2021). Systematic review and meta-analysis of validated prognostic models for resected hepatocellular carcinoma patients. Eur. J. Surg. Oncol. 48, 492–499. PubMed PMID: 34602315. doi:10.1016/j.ejso.2021.09.012
Campagne, O., Yeo, K. K., Fangusaro, J., and Stewart, C. F. (2021). Clinical pharmacokinetics and pharmacodynamics of selumetinib. Clin. Pharmacokinet. 60 (3), 283–303. PubMed PMID: 33354735. doi:10.1007/s40262-020-00967-y
Changjun, L., Feizhou, H., Dezhen, P., Zhao, L., and Xianhai, M. (2018). MiR-545-3p/MT1M axis regulates cell proliferation, invasion and migration in hepatocellular carcinoma. Biomed. Pharmacother. 108, 347–354. PubMed PMID: 30227328. doi:10.1016/j.biopha.2018.09.009
Chen, S., Li, K., Jiang, J., Wang, X., Chai, Y., Zhang, C., et al. (2020). Low expression of organic anion-transporting polypeptide 1B3 predicts a poor prognosis in hepatocellular carcinoma. World J. Surg. Oncol. 18 (1), 127. PubMed PMID: 32534581; PubMed Central PMCID: PMCPMC7293789. doi:10.1186/s12957-020-01891-y
Cheng, B. Y., Lau, E. Y., Leung, H. W., Leung, C. O., Ho, N. P., Gurung, S., et al. (2018). IRAK1 augments cancer stemness and drug resistance via the AP-1/AKR1B10 signaling cascade in hepatocellular carcinoma. Cancer Res. 78 (9), 2332–2342. PubMed PMID: 29483095. doi:10.1158/0008-5472.CAN-17-2445
Cui, L-H., Quan, Z-Y., Piao, J-M., Zhang, T-T., Jiang, M-H., Shin, M-H., et al. (2016). Plasma folate and vitamin B12 levels in patients with hepatocellular carcinoma. Int. J. Mol. Sci. 17 (7), E1032. PubMed PMID: 27376276. doi:10.3390/ijms17071032
Davies, H., Bignell, G. R., Cox, C., Stephens, P., Edkins, S., Clegg, S., et al. (2002). Mutations of the BRAF gene in human cancer. Nature 417 (6892), 949–954. PubMed PMID: 12068308. doi:10.1038/nature00766
DiStefano, J. K., and Davis, B. (2019). Diagnostic and prognostic potential of AKR1B10 in human hepatocellular carcinoma. Cancers (Basel) 11 (4), E486. PubMed PMID: 30959792; PubMed Central PMCID: PMCPMC6521254. doi:10.3390/cancers11040486
Dou, L., Shi, X., He, X., and Gao, Y. (2019). Macrophage phenotype and function in liver disorder. Front. Immunol. 10, 3112. PubMed PMID: 32047496. doi:10.3389/fimmu.2019.03112
Edoo, M. I. A., Chutturghoon, V. K., Wusu-Ansah, G. K., Zhu, H., Zhen, T. Y., Xie, H. Y., et al. (2019). Serum biomarkers AFP, CEA and CA19-9 combined detection for early diagnosis of hepatocellular carcinoma. Iran. J. Public Health 48 (2), 314–322. PubMed PMID: 31205886.
Farinha, D., Migawa, M., Sarmento-Ribeiro, A., and Faneca, H. (2021). A combined antitumor strategy mediated by a new targeted nanosystem to hepatocellular carcinoma. Int. J. Nanomedicine 16, 3385–3405. PubMed PMID: 34040370; PubMed Central PMCID: PMCPMC8141275. doi:10.2147/IJN.S302288
Geng, N., Jin, Y., Li, Y., Zhu, S., and Bai, H. (2020). AKR1B10 inhibitor epalrestat facilitates sorafenib-induced apoptosis and autophagy via targeting the mTOR pathway in hepatocellular carcinoma. Int. J. Med. Sci. 17 (9), 1246–1256. PubMed PMID: 32547320; PubMed Central PMCID: PMCPMC7294918. doi:10.7150/ijms.42956
Gnoni, A., Licchetta, A., Memeo, R., Argentiero, A., Solimando, A. G., Longo, V., et al. (2019). Role of BRAF in hepatocellular carcinoma: A rationale for future targeted cancer therapies. Med. Kaunas. 55 (12), E754. PubMed PMID: 31766556; PubMed Central PMCID: PMCPMC6956203. doi:10.3390/medicina55120754
Han, C., Gao, L., Bai, H., and Dou, X. (2018). Identification of a role for serum aldo-keto reductase family 1 member B10 in early detection of hepatocellular carcinoma. Oncol. Lett. 16 (6), 7123–7130. PubMed PMID: 30546447; PubMed Central PMCID: PMCPMC6256343. doi:10.3892/ol.2018.9547
Han, J., Han, M-L., Xing, H., Li, Z-L., Yuan, D-Y., Wu, H., et al. (2020). Tissue and serum metabolomic phenotyping for diagnosis and prognosis of hepatocellular carcinoma. Int. J. Cancer 146 (6), 1741–1753. PubMed PMID: 31361910. doi:10.1002/ijc.32599
Hu, D. G., Marri, S., McKinnon, R. A., Mackenzie, P. I., and Meech, R. (2019). Deregulation of the genes that are involved in drug absorption, distribution, metabolism, and excretion in hepatocellular carcinoma. J. Pharmacol. Exp. Ther. 368 (3), 363–381. PubMed PMID: 30578287. doi:10.1124/jpet.118.255018
Huang, K., Xie, W., Wang, S., Li, Q., Wei, X., Chen, B., et al. (2021). High SPINK1 expression predicts poor prognosis and promotes cell proliferation and metastasis of hepatocellular carcinoma. J. Invest. Surg. 34 (9), 1011–1020. PubMed PMID: 32066292. doi:10.1080/08941939.2020.1728443
Huynh, H., Ong, R., Goh, K. Y., Lee, L. Y., Puehler, F., Scholz, A., et al. (2019). Sorafenib/MEK inhibitor combination inhibits tumor growth and the Wnt/β‑catenin pathway in xenograft models of hepatocellular carcinoma. Int. J. Oncol. 54 (3), 1123–1133. PubMed PMID: 30747223. doi:10.3892/ijo.2019.4693
Hyman, D. M., Puzanov, I., Subbiah, V., Faris, J. E., Chau, I., Blay, J-Y., et al. (2015). Vemurafenib in multiple nonmelanoma cancers with BRAF V600 mutations. N. Engl. J. Med. 373 (8), 726–736. PubMed PMID: 26287849. doi:10.1056/NEJMoa1502309
Ji, X. F., Fan, Y. C., Gao, S., Yang, Y., Zhang, J. J., and Wang, K. (2014). MT1M and MT1G promoter methylation as biomarkers for hepatocellular carcinoma. World J. Gastroenterol. 20 (16), 4723–4729. PubMed PMID: 24782625; PubMed Central PMCID: PMCPMC4000509. doi:10.3748/wjg.v20.i16.4723
Jia, J., Ga, L., Liu, Y., Yang, Z., Wang, Y., Guo, X., et al. (2022). Serine protease inhibitor kazal type 1, A potential biomarker for the early detection, targeting, and prediction of response to immune checkpoint blockade therapies in hepatocellular carcinoma. Front. Immunol. 13, 923031. PubMed PMID: 35924241; PubMed Central PMCID: PMCPMC9341429. doi:10.3389/fimmu.2022.923031
Kitao, A., Matsui, O., Yoneda, N., Kozaka, K., Kobayashi, S., Koda, W., et al. (2020). Gadoxetic acid-enhanced MR imaging for hepatocellular carcinoma: Molecular and genetic background. Eur. Radiol. 30 (6), 3438–3447. PubMed PMID: 32064560. doi:10.1007/s00330-020-06687-y
Kourou, K., Exarchos, T. P., Exarchos, K. P., Karamouzis, M. V., and Fotiadis, D. I. (2015). Machine learning applications in cancer prognosis and prediction. Comput. Struct. Biotechnol. J. 13, 8–17. PubMed PMID: 25750696. doi:10.1016/j.csbj.2014.11.005
Leek, J. T., Johnson, W. E., Parker, H. S., Jaffe, A. E., and Storey, J. D. (2012). The sva package for removing batch effects and other unwanted variation in high-throughput experiments. Bioinformatics 28 (6), 882–883. PubMed PMID: 22257669; PubMed Central PMCID: PMCPMC3307112. doi:10.1093/bioinformatics/bts034
Li, F., Liu, T., Xiao, C. Y., Yu, J. X., Lu, L. G., and Xu, M. Y. (2015). FOXP1 and SPINK1 reflect the risk of cirrhosis progression to HCC with HBV infection. Biomed. Pharmacother. 72, 103–108. PubMed PMID: 26054682. doi:10.1016/j.biopha.2015.04.006
Li, Z., Lin, Y., Cheng, B., Zhang, Q., and Cai, Y. (2021). Identification and analysis of potential key genes associated with hepatocellular carcinoma based on integrated bioinformatics methods. Front. Genet. 12, 571231. PubMed PMID: 33767726. doi:10.3389/fgene.2021.571231
Li, Z., Wu, X., Gao, X., Shan, F., Ying, X., Zhang, Y., et al. (2020). Development and validation of an artificial neural network prognostic model after gastrectomy for gastric carcinoma: An international multicenter cohort study. Cancer Med. 9 (17), 6205–6215. PubMed PMID: 32666682. doi:10.1002/cam4.3245
Lin, S., Lin, Y., Wu, Z., Xia, W., Miao, C., Peng, T., et al. (2021). circRPS16 promotes proliferation and invasion of hepatocellular carcinoma by sponging miR-876-5p to upregulate SPINK1. Front. Oncol. 11, 724415. PubMed PMID: 34595116; PubMed Central PMCID: PMCPMC8476860. doi:10.3389/fonc.2021.724415
Liu, Y., Zhang, J., Liu, H., Guan, G., Zhang, T., Wang, L., et al. (2019). Compensatory upregulation of aldo-keto reductase 1B10 to protect hepatocytes against oxidative stress during hepatocarcinogenesis. Am. J. Cancer Res. 9 (12), 2730–2748. Epub 20191201. PubMed PMID: 31911858; PubMed Central PMCID: PMCPMC6943354.
Liu, Y-C., Yeh, C-T., and Lin, K-H. (2020). Cancer stem cell functions in hepatocellular carcinoma and comprehensive therapeutic strategies. Cells 9 (6), E1331. PubMed PMID: 32466488. doi:10.3390/cells9061331
Mai, R-Y., Zeng, J., Mo, Y-S., Liang, R., Lin, Y., Wu, S-S., et al. (2020). Artificial neural network model for liver cirrhosis diagnosis in patients with hepatitis B virus-related hepatocellular carcinoma. Ther. Clin. Risk Manag. 16, 639–649. PubMed PMID: 32764948. doi:10.2147/TCRM.S257218
Marshall, A., Lukk, M., Kutter, C., Davies, S., Alexander, G., and Odom, D. T. (2013). Global gene expression profiling reveals SPINK1 as a potential hepatocellular carcinoma marker. PLoS One 8 (3), e59459. PubMed PMID: 23527199; PubMed Central PMCID: PMCPMC3601070. doi:10.1371/journal.pone.0059459
Mok, S., Tsoi, J., Koya, R. C., Hu-Lieskovan, S., West, B. L., Bollag, G., et al. (2015). Inhibition of colony stimulating factor-1 receptor improves antitumor efficacy of BRAF inhibition. BMC Cancer 15, 356. PubMed PMID: 25939769. doi:10.1186/s12885-015-1377-8
Nebot, N., Won, C. S., Moreno, V., Munoz-Couselo, E., Lee, D. Y., Gasal, E., et al. (2021). Evaluation of the effects of repeat-dose Dabrafenib on the single-dose pharmacokinetics of rosuvastatin (OATP1B1/1B3 substrate) and midazolam (CYP3A4 substrate). Clin. Pharmacol. Drug Dev. 10 (9), 1054–1063. PubMed PMID: 33932130; PubMed Central PMCID: PMCPMC8453865. doi:10.1002/cpdd.937
Puszkiel, A., Noé, G., Bellesoeur, A., Kramkimel, N., Paludetto, M-N., Thomas-Schoemann, A., et al. (2019). Clinical pharmacokinetics and pharmacodynamics of Dabrafenib. Clin. Pharmacokinet. 58 (4), 451–467. PubMed PMID: 30094711. doi:10.1007/s40262-018-0703-0
Rastogi, A. (2018). Changing role of histopathology in the diagnosis and management of hepatocellular carcinoma. World J. Gastroenterol. 24 (35), 4000–4013. PubMed PMID: 30254404. doi:10.3748/wjg.v24.i35.4000
Shek, F. H., Luo, R., Lam, B. Y. H., Sung, W. K., Lam, T. W., Luk, J. M., et al. (2017). Serine peptidase inhibitor Kazal type 1 (SPINK1) as novel downstream effector of the cadherin-17/β-catenin axis in hepatocellular carcinoma. Cell. Oncol. 40 (5), 443–456. PubMed PMID: 28631187. doi:10.1007/s13402-017-0332-x
Shibasaki, Y., Sakaguchi, T., Hiraide, T., Morita, Y., Suzuki, A., Baba, S., et al. (2015). Expression of indocyanine green-related transporters in hepatocellular carcinoma. J. Surg. Res. 193 (2), 567–576. PubMed PMID: 25173835. doi:10.1016/j.jss.2014.07.055
Sun, W., Zhang, Y., Liu, B., Duan, Y., Li, W., and Chen, J. (2021). Gene polymorphism of MUC15, MMP14, BRAF, and COL1A1 is associated with capsule formation in hepatocellular carcinoma. Can. J. Gastroenterol. Hepatol. 2021, 9990305. PubMed PMID: 34007838; PubMed Central PMCID: PMCPMC8100414. doi:10.1155/2021/9990305
Tian, Z., Hou, X., Liu, W., Han, Z., and Wei, L. (2019). Macrophages and hepatocellular carcinoma. Cell. Biosci. 9, 79. PubMed PMID: 31572568. doi:10.1186/s13578-019-0342-7
Ueno, A., Masugi, Y., Yamazaki, K., Komuta, M., Effendi, K., Tanami, Y., et al. (2014). OATP1B3 expression is strongly associated with Wnt/β-catenin signalling and represents the transporter of gadoxetic acid in hepatocellular carcinoma. J. Hepatol. 61 (5), 1080–1087. PubMed PMID: 24946283. doi:10.1016/j.jhep.2014.06.008
Villanueva, A. (2019). Hepatocellular carcinoma. N. Engl. J. Med. 380 (15), 1450–1462. doi:10.1056/NEJMra1713263
Vogel, A., and Saborowski, A. (2020). Current strategies for the treatment of intermediate and advanced hepatocellular carcinoma. Cancer Treat. Rev. 82, 101946. PubMed PMID: 31830641. doi:10.1016/j.ctrv.2019.101946
Wang, A., Wu, L., Lin, J., Han, L., Bian, J., Wu, Y., et al. (2018). Whole-exome sequencing reveals the origin and evolution of hepato-cholangiocarcinoma. Nat. Commun. 9 (1), 894. PubMed PMID: 29497050. doi:10.1038/s41467-018-03276-y
Wu, T., Ke, Y., Tang, H., Liao, C., Li, J., and Wang, L. (2021). Fidarestat induces glycolysis of NK cells through decreasing AKR1B10 expression to inhibit hepatocellular carcinoma. Mol. Ther. Oncolytics 23, 420–431. PubMed PMID: 34853813; PubMed Central PMCID: PMCPMC8605295. doi:10.1016/j.omto.2021.06.005
Xie, D-Y., Ren, Z-G., Zhou, J., Fan, J., and Gao, Q. (20192020). 2019 Chinese clinical guidelines for the management of hepatocellular carcinoma: Updates and insights. Hepatobiliary Surg. Nutr. 9 (4), 452–463. PubMed PMID: 32832496. doi:10.21037/hbsn-20-480
Yamashita, T., Kitao, A., Matsui, O., Hayashi, T., Nio, K., Kondo, M., et al. (2014). Gd-EOB-DTPA-enhanced magnetic resonance imaging and alpha-fetoprotein predict prognosis of early-stage hepatocellular carcinoma. Hepatology 60 (5), 1674–1685. PubMed PMID: 24700365; PubMed Central PMCID: PMCPMC4142120. doi:10.1002/hep.27093
Yan, P., Pang, P., Hu, X., Wang, A., Zhang, H., Ma, Y., et al. (2021). Specific MiRNAs in naïve T cells associated with hepatitis C virus-induced hepatocellular carcinoma. J. Cancer 12 (1), 1–9. PubMed PMID: 33391397. doi:10.7150/jca.49594
Yang, J. D., and Heimbach, J. K. (2020). New advances in the diagnosis and management of hepatocellular carcinoma. BMJ 371, m3544. PubMed PMID: 33106289. doi:10.1136/bmj.m3544
Ye, X., Li, C., Zu, X., Lin, M., Liu, Q., Liu, J., et al. (2019). A large-scale multicenter study validates aldo-keto reductase family 1 member B10 as a prevalent serum marker for detection of hepatocellular carcinoma. Hepatology 69 (6), 2489–2501. PubMed PMID: 30672601; PubMed Central PMCID: PMCPMC6593451. doi:10.1002/hep.30519
Ying, H. Y., Gong, C. J., Feng, Y., Jing, D. D., and Lu, L. G. (2017). Serine protease inhibitor Kazal type 1 (SPINK1) downregulates E-cadherin and induces EMT of hepatoma cells to promote hepatocellular carcinoma metastasis via the MEK/ERK signaling pathway. J. Dig. Dis. 18 (6), 349–358. PubMed PMID: 28544403. doi:10.1111/1751-2980.12486
Yuan, J., Dong, X., Yap, J., and Hu, J. (2020). The MAPK and AMPK signalings: Interplay and implication in targeted cancer therapy. J. Hematol. Oncol. 13 (1), 113. PubMed PMID: 32807225; PubMed Central PMCID: PMCPMC7433213. doi:10.1186/s13045-020-00949-4
Zhang, Q., Rong, Y., Yi, K., Huang, L., Chen, M., and Wang, F. (2020). Circulating tumor cells in hepatocellular carcinoma: Single-cell based analysis, preclinical models, and clinical applications. Theranostics 10 (26), 12060–12071. PubMed PMID: 33204329. doi:10.7150/thno.48918
Zhang, S., Huang, Z., Zhou, S., Wang, B., Ding, Y., Chu, J. Z., et al. (2018). The effect and mechanism of metallothionein MT1M on hepatocellular carcinoma cell. Eur. Rev. Med. Pharmacol. Sci. 22 (3), 695–701. PubMed PMID: 29461597. doi:10.26355/eurrev_201802_14295
Zhang, T., Guan, G., Zhang, J., Zheng, H., Li, D., Wang, W., et al. (2022). E2F1-mediated AUF1 upregulation promotes HCC development and enhances drug resistance via stabilization of AKR1B10. Cancer Sci. 113 (4), 1154–1167. PubMed PMID: 35178834; PubMed Central PMCID: PMCPMC8990806. doi:10.1111/cas.15272
Zhang, X., Ng, H. L. H., Lu, A., Lin, C., Zhou, L., Lin, G., et al. (2016). Drug delivery system targeting advanced hepatocellular carcinoma: Current and future. Nanomedicine 12 (4), 853–869. PubMed PMID: 26772424. doi:10.1016/j.nano.2015.12.381
Zhong, B-Y., Ni, C-F., Ji, J-S., Yin, G-W., Chen, L., Zhu, H-D., et al. (2019). Nomogram and artificial neural network for prognostic performance on the albumin-bilirubin grade for hepatocellular carcinoma undergoing transarterial chemoembolization. J. Vasc. Interv. Radiol. 30 (3), 330–338. PubMed PMID: 30819473. doi:10.1016/j.jvir.2018.08.026
Keywords: hepatocellular carcinoma, diagnostic model, artificial neural network, MT1M, SPINK1, AKR1B10, SLCO1B3
Citation: Chen M, Wu G-B, Xie Z-W, Shi D-L and Luo M (2022) A novel diagnostic four-gene signature for hepatocellular carcinoma based on artificial neural network: Development, validation, and drug screening. Front. Genet. 13:942166. doi: 10.3389/fgene.2022.942166
Received: 12 May 2022; Accepted: 02 02 September 20222022;
Published: 28 September 2022.
Edited by:
Mukesh Kumar Gupta, National Institute of Technology Rourkela, IndiaReviewed by:
Tung Nguyen-Thanh, Hue University of Medicine and Pharmacy, VietnamKangkang Yu, Fudan University, China
Copyright © 2022 Chen, Wu, Xie, Shi and Luo. This is an open-access article distributed under the terms of the Creative Commons Attribution License (CC BY). The use, distribution or reproduction in other forums is permitted, provided the original author(s) and the copyright owner(s) are credited and that the original publication in this journal is cited, in accordance with accepted academic practice. No use, distribution or reproduction is permitted which does not comply with these terms.
*Correspondence: Dan-Li Shi, jasonmob@sina.com; Meng Luo, luosh9hospital@sina.com
†These authors have contributed equally to this work