- 1Genomics and Molecular Medicine Unit, Institute of Genomics and Integrative Biology (IGIB), Council of Scientific and Industrial Research (CSIR), Delhi, India
- 2Institute of Human Behaviour and Allied Sciences (IHBAS), Delhi, India
- 3Academy of Scientific and Innovative Research (AcSIR), Council of Scientific and Industrial Research (CSIR), Delhi, India
- 4Genomic Medicine Institute, Lerner Research Institute, Cleveland Clinic Foundation, Cleveland, OH, United States
- 5Department of Pharmacology, Faculty of Pharmacy, Jamia Hamdard, Delhi, India
- 6AceProbe Technologies (India) Pvt. Ltd, Delhi, India
- 7Department of Clinical and Experimental Medicine, University of Foggia, Foggia, Italy
- 8Department of Physiology and Pharmacology “Vittorio Erspamer” Sapienza University, Rome, Italy
Previously, we demonstrated an integrated genomic convergence and network analysis approach to identify the candidate genes associated with the complex neurodegenerative disorder, Alzheimer’s disease (AD). Here, we performed a pilot study to validate the in silico approach by studying the association of genetic variants from three identified critical genes, APOE, EGFR, and ACTB, with AD. A total of 103 patients with AD and 146 healthy controls were recruited. A total of 46 single-nucleotide polymorphisms (SNPs) spanning the three genes were genotyped, of which only 19 SNPs were included in the final analyses after excluding non-polymorphic and Hardy–Weinberg equilibrium-violating SNPs. Apart from our previously reported APOE ε4, four other SNPs in APOE (rs405509, rs7259620, −rs769449, and rs7256173), one in EGFR (rs6970262), and one in ACTB (rs852423) showed a significant association with AD (p < 0.05). Our results validate the reliability of genomic convergence and network analysis approach in identifying the AD-associated candidate genes.
Introduction
Alzheimer’s disease (AD), the most common cause of dementia, is becoming a major health burden worldwide among the elderly due to the aging population, absence of early diagnostic markers, and the lack of disease-modifying treatment (Rajasekhar and Govindaraju, 2018). Globally, 46.8 million were affected by AD in 2015, which is estimated to reach 131.5 million by 2050. As per the Alzheimer’s’ Disease International (ADI) report, Asia Pacific region would witness rise in patients with AD (PwAD) from 23 million in 2015 to 71 million with India having 12 million cases (Prince, 2015).
AD is heritable, but genetically complex. The disease, genetically, is segregated into two forms: familial AD and sporadic, which are clinically indistinguishable. The major genetic signatures identified for familial AD are present in the three genes, namely, the amyloid precursor protein (APP) and the presenilins (PSEN1 and PSEN2), while sporadic AD involves the contribution of both genetic and environmental factors (Bekris et al., 2010). However, despite several decades of genetic research, only a small fraction of the genetic variants associated with AD risk have been identified (Wightman et al., 2021). Currently, at least 29 major risk loci for late onset or sporadic AD risk have been uncovered by various large-scale meta-analysis studies (Jansen et al., 2019; Wightman et al., 2021). A major issue with the findings of the genome level studies is the identification of signatures mostly in the non-coding regions with unknown significance. Furthermore, the common variants fail to fully explain the underlying pathophysiology probably due to small effect size (Van Cauwenberghe et al., 2016; Visscher et al., 2021).
As the vast literature is available on genomic studies involving different methodologies such as genome-wide studies including linkage, association, and expression (Talwar et al., 2016), previously, we employed an integrative approach combining multiple data sources along with network modeling of protein–protein interactions to identify the candidate genes associated with AD which revealed APOE, EFGR, and ACTB as the hub genes (Talwar et al., 2014). The aim of the present cross-sectional study was to validate the findings of our genomic convergence and network analysis approach by investigating the association of genetic variants from the three genes with AD in North Indian population.
Methods
Study Participants and Phenotyping for Case–Control Study
Individuals of age >50 years and North Indian ancestry attending outpatient neurobehavioral clinic at the Institute of Human Behavior and Allied Sciences (IHBAS) from 2010 to 2012 were enrolled in this cross-sectional study. Family members gave written informed consent, and the study was approved by the Institutional Ethics Committee. The other details related to diagnosis, assessment, investigations, and recruitment of the patients with AD (PwAD) and healthy controls are provided in Supplementary File S1, Methods. The demographic and clinical characteristics of the recruited PwAD as well as healthy controls were reported previously (Talwar et al., 2017).
Genotyping
Genomic DNA was isolated from the peripheral blood leukocytes using a modification of a salting out procedure (Miller et al., 1988). The functionally important single-nucleotide polymorphisms (SNPs) in APOE, EGFR, and ACTB genes were screened from the literature, and then the functional significance of the SNPs was determined by screening databases including HaploReg, RegulomeDb, rVarBase, and Braineac (in build in vitro and in vivo evidence). Please refer to Supplementary File S2 for more details. The selected SNPs were genotyped by AceProbe Technologies (India) Pvt. Ltd. by using the Sequenom MassARRAY iPLEX platform (Sequenom Inc., San Diego, United States). The list of primers used is provided in Supplementary File S1, Supplementary Table S1.
Statistical Analysis
The SNP association analysis was conducted in PLINK version 1.09 (Purcell et al., 2007) and gPLINK (https://zzz.bwh.harvard.edu/plink/gplink.shtml). The imputation, quality control (QC), and association analysis procedure are described in Supplementary File S1, Methods. In brief, after imputation and QC, univariate SNP association analysis was carried out by 2×2 contingency table of χ2 test or Fisher’s exact test. The Benjamini–Hochberg (BH) method was used for multiple testing corrections based on the false discovery rate (FDR) (Benjamini and Hochberg, 1995; Clarke et al., 2011).
Logistic regression analysis was conducted to assess the differences of genotype frequencies between AD and non-demented control groups. The additive model, the dominant model and the recessive model were used in the logistic regression analysis for SNP association with disease phenotype adjusting for age, gender, and education status as covariates. In the additive model, homozygotes for the major allele, and heterozygotes and homozygotes for the minor allele were coded to a quantitative numeric variable for genotypes (0, 1, and 2), implying the additive effects of allele dosage. The dominant and recessive models assume the full dominance [genotype coding (0, 1, and 1)] or recessive [genotype coding (0, 0, and 1)] for the minor allele (Purcell et al., 2007; Laird and Lange, 2011). The linkage disequilibrium (LD) pattern was analyzed using Haploview software (Barrett, 2009). The level of significance was set to 0.05.
Results
Basic Characteristics of Study Subjects
A total of 108 PwAD (60.2% men) fulfilling the selection criteria along with 159 healthy controls (51.6% men) of similar ethnicity were enrolled (Talwar et al., 2017). A significant difference was observed in the mean age and education status between the cases and controls (p < 0.05) (Talwar et al., 2017), requiring an adjustment of the two potential confounders during the association analysis.
Frequency Distribution of Genetic Variants
A total of 46 functional SNPs, 10 from APOE, 24 from EGFR, and 12 from ACTB were selected for the case–control association analysis. Out of the total 267 individuals, 12 were excluded either due to the lack of phenotypic data or insufficient DNA and 6 due to the failure of genotyping, resulting in data from 249 total individuals (103 PwAD and 146 controls). Of the 46 SNPs, 12 were found to be non-polymorphic in individuals from the two groups, while 15 failed the Hardy–Weinberg equilibrium (HWE) test in the controls (Supplementary Files S3a,b). The remaining 19 SNPs were included in the final analyses. Among these 19 SNPs, such as APOE rs429358 and rs7412, resulted in ε2 (rs429358, T; rs7412, T), ε3 (rs429358, T; rs7412, C), and ε4 (rs429358, C; rs7412, C) haplotypes. We previously reported ε3 to be the most common in both cases and controls, while the presence of the ε4 allele increased the risk of having AD (Talwar et al., 2017).
Allelic and Genotypic Associations
The allelic and genotypic distribution of 19 SNPs from the three genes in the genotyped 249 samples was evaluated for the association with AD (Supplementary File S4a). Apart from the previously reported APOE ε alleles, three other SNPs, namely, rs405509, rs7259620, and rs769449, from APOE, and rs6970262 from EGFR showed a significant association in genotypic, Cochran–Armitage trend and allelic analysis (p < 0.05) (Table 1). None of the genotyped SNPs from the ACTB gene showed a significant allelic association. The data for significant associations are provided in Table 1 and for all 19 SNPs in Supplementary Table 4a.
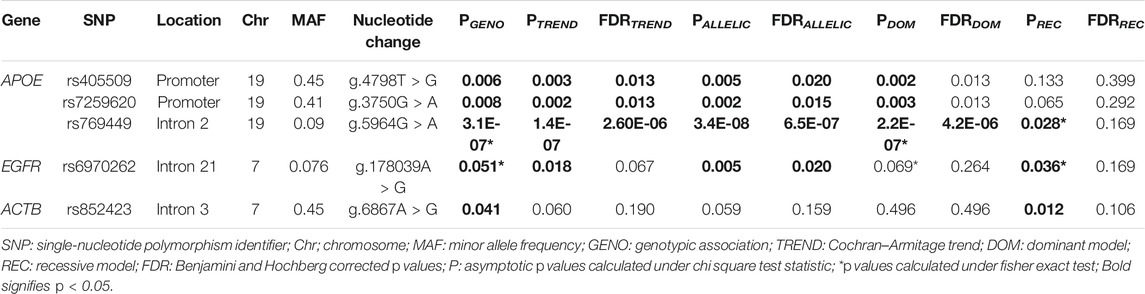
TABLE 1. Association analysis of APOE, EGFR, and ACTB SNPs with AD risk among PwAD (N = 103) and non-demented controls (N = 146).
Logistic regression analysis performed for genotypic association analysis after adjustment for age, gender, and education status showed a significant association of rs405509 (Padditive = 0.003, OR = 0.51, 95% CI = 0.33–0.79 and Pdom = 0.003, and OR = 0.39, 95% CI = 0.21–0.73), rs7259620 (Padditive = 0.007, OR = 0.56, 95% CI = 0.37–0.85 and Pdom = 0.005, OR = 0.44, 95% CI = 0.25–0.79), rs769449 (Padditive = 2.9E-06, OR = 9.75, 95% CI = 3.76–25.30 and Pdom = 1.2E-06, OR = 10.48, 95% CI = 3.98–27.59), and rs7256173 (Padditive = 0.021, OR = 5.54, 95% CI = 1.30–23.69 and Pdom = 0.021, OR = 5.54, 95% CI = 1.30–23.69) from APOE. In EGFR, rs6970262 showed significant association only in the additive model (Padditive = 0.030, OR = 2.18, 95% CI = 1.08–4.41), and in case of ACTB, rs852423 showed significant association only in the recessive model (Precessive = 0.032, OR = 2.18, 95% CI = 1.07–4.46) with AD risk after adjustment for age, gender, and education status. The data for significant associations are provided in Table 2 and for all 19 SNPs in Supplementary Table S4b. Furthermore, rs769449 in APOE was found to be in a strong degree of LD with rs429358 (R2 = 0.96) and rs7259620 was in LD with rs405509 (R2 = 0.88). Despite this, we did not observe the formation of any haplotype block in the study.
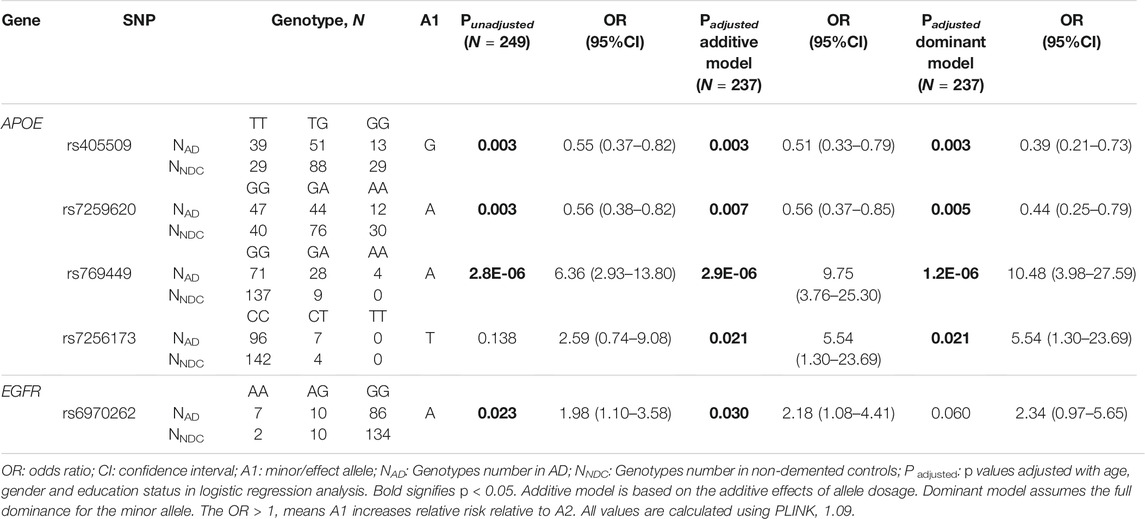
TABLE 2. Association of APOE, EGFR, and ACTB SNPs with Alzheimer’s disease under logistic regression analysis.
Discussion
In the present study, we conducted a pilot genetic association analysis comprising 249 study subjects to validate the findings of our genomic convergence and network analysis using the in silico approach. A total of 46 SNPs in the three identified hub genes, namely, APOE, EGFR, and ACTB, were screened for functional genetic variants to perform the association study. The study observed a significant association of six SNPs with AD: rs405509, rs7259620, rs769449, and rs7256173 from APOE, rs6970262 from EGFR, and rs852423 from ACTB. Despite strong LD between the SNPs of the APOE gene, we did not observe a haplotype block in the studied cohort. This could potentially be due to the presence of multiple SNPs in LD, where uneven distance makes it difficult to determine the exact boundaries of the haplotype block (Takeuchi et al., 2005).
APOE is the most widely studied gene with respect to AD genetics due to its high abundance in different brain cells (Lefterov et al., 2019). Published reports indicate that APOE polymorphisms are associated with AD pathophysiology involving the plaque deposition of intraneuronal amyloid-β (Aβ) aggregates, and hyperphosphorylated tau-mediated neurodegeneration (Yamazaki et al., 2019). In addition, peripheral ApoE also plays a potential role in lipid homeostasis in the brain (Martinez-Morillo et al., 2014). We previously reported the APOE ε4 allele to be associated with increased susceptibility for AD (Talwar et al., 2017). In the current study, apart from the epsilon alleles, we reported other APOE promoters and intronic SNPs including rs405509, rs7259620, rs769449, and rs7256173 to be associated with AD. The rs405509 TT genotype was observed to be over-represented in PwAD. In a previous case–control study, the rs405509 “T” homozygote was associated with the increased risk for developing AD (Lambert et al., 2002). The same group also reported that the patients with the rs405509 TT genotype had higher levels of total Aβ in the Brodmann area of the cerebral cortex than those with GT/GG genotypes (Lambert et al., 2001), indicating the involvement of rs405509 in the processing of β amyloid. Individuals homozygous for the “T” allele were also reported to have higher number of senile plaque in hippocampus CA1 and the subiculum (Berr et al., 2001), and the elderlies displayed cognitive impairment and low gray matter volume (Ma et al., 2016). Such effects could be due to lower brain ApoE levels in individuals with the TT genotype at rs405509. Substitution of rs405509 “T” to the minor allele “G” significantly increases the APOE promoter activity (Artiga et al., 1998), suggesting a protective effect of the rs405509 “G” allele in AD, as observed in our study. These findings provide evidence that rs405509 may be involved in the APOE gene regulation and, therefore, may serve as a potential biomarker in AD.
The association of another APOE promoter SNP, rs7259620, in the present study was supported by similar findings from a case–control study involving Japanese population where the minor allele “A” was observed to have a protective role in LOAD (Takei et al., 2009); however, its role in APOE regulation is still unexplored. We also found the intronic APOE SNP, rs769449, to be highly associated with AD, where the presence of “A” allele showed an increased risk. The SNP was previously reported to be associated with a cognitive decline among European-Americans and African-Americans (Zhang and Pierce, 2014). Cruchaga et al. (2013) observed rs769449 to be associated with the CSF levels of tau and ptau proteins, two key biochemical markers of axonal degeneration, neuronal loss, and a cognitive decline in PwAD, suggesting a role of this intronic SNP in AD pathophysiology. Nevertheless, the effect of “G” to “A” substitution in this SNP has not been investigated yet. Last, the APOE genetic variant, rs7256173, has not been previously associated with AD.
Another gene, EGFR, encodes the epidermal growth factor receptor, a transmembrane protein that binds to the epidermal growth factor. EGFR knock-out mice were reported to develop neurodegeneration leading to early death due to defects in cortical neurogenesis, indicating that EGFR signaling plays a role in neurogenesis (Wong and Guillaud, 2004). Our study identified “A” allele/AA genotype of the EGFR intronic variant rs6970262 to be significantly associated with increased AD risk.
Although rs6970262 has not been previously associated with AD, previous investigations from our group reported EGFR as a potential candidate for assessing AD risk (Talwar et al., 2014; Talwar et al., 2017). The EGFR was indicated as a factor that mediates beta amyloid (Aβ) in animal models (Wang et al., 2012). Moreover, poor olfactory discrimination associated with AD may be due to the reduced EGF-dependent olfactory neurogenesis (Enwere et al., 2004). The olfactory system is a relevant effector in different animal models of neurodegenerative diseases (Loseva et al., 2009). Moreover, the olfactory bulbectomy is a known animal model of depression (Song and Leonard, 2005), and the neuroimaging studies found reduced olfactory bulb volume in depressive patients (Negoias et al., 2010). Depression is a risk factor for AD (Modrego and Ferrández, 2004; Ownby et al., 2006; Sun et al., 2008) and frequently observed in preclinical AD (Geerlings et al., 2000; Visser et al., 2000). It has been reported that soluble Aβ induces a depressive-like phenotype in rats (Colaianna et al., 2010). Hence, considering that a pilot study has found a possible relationship between depression and EGFR mutation status in patients with non–small-cell lung cancer (Jacobs et al., 2017), the EGFR intronic variant rs6970262 could be useful to characterize depressed patients with high risk to develop AD.
The presence of immunoreactive EGFR was also observed in neuritic plaques from PwAD (Birecree et al., 1988). Similar findings were reported in the brain vasculature of demented elderly patients where the increased EGFR expression was related to proliferative or regenerative activities in the vascular architecture of PwAD, suggesting that it might be used as a potential biomarker for early diagnosis of dementia using skin biopsy (Styren et al., 1990; Styren et al., 1993).
Our study also involved important SNPs from the gene ACTB, which encodes the non-muscle cytoskeletal actin, β-actin. Mutations in this gene cause Baraitser–Winter syndrome 1 and juvenile-onset dystonia (https://www.genecards.org/cgi-bin/carddisp.pl?gene=ACTB, last update: 24 May 2021). Due to the highly conserved sequence and ubiquitous expression of ACTB, the gene is used as a reference for the normalization of target gene expression in mRNA and protein studies. However, Leduc et al. (2011) found the gene to be an unsuitable reference gene due to lower stability of mRNA in the frontal cortex of PwAD, suggesting dysregulation of the gene in AD. In addition, an integrated in silico analysis involving four different gene expression datasets also found ACTB to be a central gene in AD pathophysiology (Hu et al., 2015). Our study observed a significant genotypic association of the ACTB variant rs852423 with increased susceptibility to AD. The association of this SNP with AD also appears in the signaling network presented in the database NeuroMMSig (Domingo-Fernández et al., 2017).
The current knowledge is limited, but a connection from this gene and glucose metabolism in AD pathophysiology is worth to be explored in the future studies. In fact, in vitro experiment that generated insulin-secreting beta cells from human pluripotent stem cells has shown the fundamental role of actin in pancreatic progenitor gene expression and endocrine function (Hogrebe et al., 2020; Siehler et al., 2020). The pancreas has a central role in the glucose metabolism, and the glycolytic dysfunction has been associated with the dysfunction of Alzheimer’s brains, such as synaptic impairment, brain atrophy, mitochondrial impairment, and Aβ deposition (Zhang et al., 2021). Moreover, diabetes has been classified as a risk factor for AD (Profenno et al., 2010), especially when associated with the APOE ε4 genotype. The subjects carrying an APOE ε4 allele without dementia have shown a reduction in the cerebral metabolic rate of glucose (Mosconi et al., 2008).
The present study has some inherent limitations such as the low sample size. However, our study was a pilot investigation to validate the findings of our in silico approach (Talwar et al., 2014), and therefore, a small sample size may yield a greater scientific value relative to the expense of the genotyping required to perform the study (Bacchetti et al., 2011). In addition, our study performed the association analysis with very few genes, in which the role of potentially actionable novel targets may get overlooked. Therefore, to establish the robustness of our in silico findings, future studies with larger sample sizes using an expanded gene list need to be performed.
In summary, this study provides the first evidence of the association of the EGFR genetic variant rs6970262. Our previous in silico analysis (Talwar et al., 2014) and its validation in clinical samples in the current study provide evidence of the association of regulatory variants present in the promoter and intronic regions of APOE, EGFR, and ACTB, highlighting the importance of integration of experimental and computational approaches to reveal the clinical significance of genetic variants in a disease phenotype. However, as multiple SNPs are implicated in complex disorders such as AD, a combination of genetics with biochemical serum markers would be crucial to uncover the pathophysiological cascade (Talwar et al., 2014; Talwar et al., 2017). Our results may help to reveal the functional role of these variants in the pathogenesis of AD. Further validation studies are required to confirm our findings and elucidate the mechanistic role of these polymorphisms in AD pathophysiology.
Data Availability Statement
The raw data supporting the conclusion of this article will be made available by the authors, without undue reservation.
Ethics Statement
The studies involving human participants were reviewed and approved by the Ethics Committee, the Institute of Human Behaviour and Allied Sciences. The patients/participants provided their written informed consent to participate in this study.
Author Contributions
PuT conceived the idea. SK contributed in diagnosis and phenotyping of the patients. SK, RA, and RK contributed in formulating the study design and critical evaluation of the study. PaT and LS performed critical evaluation of the study and helped in manuscript writing. PC contributed in genotyping of the crucial SNPs. PuT and CR wrote the manuscript. PuT, CR, AS, and HK analyzed the data. RK conceived, interpreted, and supervised the study design. All the authors read and approved the final manuscript.
Funding
This work was funded by the Council of Scientific and Industrial Research (BSC0123 and OLP1154).
Conflict of Interest
PC was employed by AceProbe Technologies (India) Pvt. Ltd.
The remaining authors declare that the research was conducted in the absence of any commercial or financial relationships that could be construed as a potential conflict of interest.
Publisher’s Note
All claims expressed in this article are solely those of the authors and do not necessarily represent those of their affiliated organizations, or those of the publisher, the editors, and the reviewers. Any product that may be evaluated in this article, or claim that may be made by its manufacturer, is not guaranteed or endorsed by the publisher.
Acknowledgments
The authors express their thanks to all the patients and their family members as well as healthy subjects for participating in the study. We thank the Director, Dr. Anurag Agarwal, CSIR-IGIB, for the motivation and unconditional support. The authors gratefully acknowledge valuable scientific discussions and suggestions by Prof. Samir K. Brahmachari (CSIR-IGIB) and Dr. Mitali Mukerji (CSIR-IGIB). PT acknowledges CSIR for providing postdoctoral research associate fellowship till September 2017 and the Department of Science and Technology (DST) for providing postdoctoral fellowship (CSRI-PDF) after that. AS acknowledges the CSIR for providing fellowship.
Supplementary Material
The Supplementary Material for this article can be found online at: https://www.frontiersin.org/articles/10.3389/fgene.2021.722221/full#supplementary-material
References
Artiga, M. J., Bullido, M. J., Sastre, I., Recuero, M., Garcı́a, M. A., Aldudo, J., et al. (1998). Allelic Polymorphisms in the Transcriptional Regulatory Region of Apolipoprotein E Gene. FEBS Lett. 421, 105–108. doi:10.1016/s0014-5793(97)01543-3
Bacchetti, P., Deeks, S. G., and Mccune, J. M. (2011). Breaking Free of Sample Size Dogma to Perform Innovative Translational Research. Sci. Transl Med. 3, 87ps24. doi:10.1126/scitranslmed.3001628
Barrett, J. C. (2009). Haploview: Visualization and Analysis of SNP Genotype Data. Cold Spring Harb Protoc. 2009, pdb ip71. doi:10.1101/pdb.ip71
Bekris, L. M., Yu, C.-E., Bird, T. D., and Tsuang, D. W. (2010). Review Article: Genetics of Alzheimer Disease. J. Geriatr. Psychiatry Neurol. 23, 213–227. doi:10.1177/0891988710383571
Benjamini, Y., and Hochberg, Y. (1995). Controlling the False Discovery Rate: a Practical and Powerful Approach to Multiple Testing. J. R. Stat. Soc. Ser. B (Methodological) 57, 289–300. doi:10.1111/j.2517-6161.1995.tb02031.x
Berr, C., Lambert, J.-C., Sazdovitch, V., Amouyel, P., Chartier-Harlin, M.-C., Mohr, M., et al. (2001). Neuropathological Epidemiology of Cerebral Aging: a Study of Two Genetic Polymorphisms. Neurobiol. Aging 22, 227–235. doi:10.1016/s0197-4580(00)00227-x
Birecree, E., Whetsell, W. O., Stoscheck, C., King, L. E., and Nanney, L. B. (1988). Immunoreactive Epidermal Growth Factor Receptors in Neuritic Plaques from Patients with Alzheimer's Disease. J. Neuropathol. Exp. Neurol. 47, 549–560. doi:10.1097/00005072-198809000-00006
Clarke, G. M., Anderson, C. A., Pettersson, F. H., Cardon, L. R., Morris, A. P., and Zondervan, K. T. (2011). Basic Statistical Analysis in Genetic Case-Control Studies. Nat. Protoc. 6, 121–133. doi:10.1038/nprot.2010.182
Colaianna, M., Tucci, P., Zotti, M., Morgese, M., Schiavone, S., Govoni, S., et al. (2010). Soluble βamyloid1-42: a Critical Player in Producing Behavioural and Biochemical Changes Evoking Depressive-Related State? Br. J. Pharmacol. 159, 1704–1715. doi:10.1111/j.1476-5381.2010.00669.x
Cruchaga, C., Kauwe, J. S. K., Harari, O., Jin, S. C., Cai, Y., Karch, C. M., et al. (2013). GWAS of Cerebrospinal Fluid Tau Levels Identifies Risk Variants for Alzheimer's Disease. Neuron 78, 256–268. doi:10.1016/j.neuron.2013.02.026
Domingo-Fernández, D., Kodamullil, A. T., Iyappan, A., Naz, M., Emon, M. A., Raschka, T., et al. (2017). Multimodal Mechanistic Signatures for Neurodegenerative Diseases (NeuroMMSig): a Web Server for Mechanism Enrichment. Bioinformatics 33, 3679–3681. doi:10.1093/bioinformatics/btx399
Enwere, E., Shingo, T., Gregg, C., Fujikawa, H., Ohta, S., and Weiss, S. (2004). Aging Results in Reduced Epidermal Growth Factor Receptor Signaling, Diminished Olfactory Neurogenesis, and Deficits in fine Olfactory Discrimination. J. Neurosci. 24, 8354–8365. doi:10.1523/jneurosci.2751-04.2004
Geerlings, M. I., Bouter, L. M., Schoevers, R. A., Beekman, A. T. F., Jonker, C., Deeg, D. J. H., et al. (2000). Depression and Risk of Cognitive Decline and Alzheimer's Disease. Br. J. Psychiatry 176, 568–575. doi:10.1192/bjp.176.6.568
Hogrebe, N. J., Augsornworawat, P., Maxwell, K. G., Velazco-Cruz, L., and Millman, J. R. (2020). Targeting the Cytoskeleton to Direct Pancreatic Differentiation of Human Pluripotent Stem Cells. Nat. Biotechnol. 38, 460–470. doi:10.1038/s41587-020-0430-6
Hu, W., Lin, X., and Chen, K. (2015). Integrated Analysis of Differential Gene Expression Profiles in Hippocampi to Identify Candidate Genes Involved in Alzheimer's Disease. Mol. Med. Rep. 12, 6679–6687. doi:10.3892/mmr.2015.4271
Jacobs, J. M., Traeger, L., Eusebio, J., Simon, N. M., Sequist, L. V., Greer, J. A., et al. (2017). Depression, Inflammation, and Epidermal Growth Factor Receptor (EGFR) Status in Metastatic Non-small Cell Lung Cancer: A Pilot Study. J. Psychosomatic Res. 99, 28–33. doi:10.1016/j.jpsychores.2017.05.009
Jansen, I. E., Savage, J. E., Watanabe, K., Bryois, J., Williams, D. M., Steinberg, S., et al. (2019). Genome-wide Meta-Analysis Identifies New Loci and Functional Pathways Influencing Alzheimer's Disease Risk. Nat. Genet. 51, 404–413. doi:10.1038/s41588-018-0311-9
Lambert, J.-C., Mann, D., Goumidi, L., Harris, J., Amouyel, P., Iwatsubo, T., et al. (2001). Effect of the APOE Promoter Polymorphisms on Cerebral Amyloid Peptide Deposition in Alzheimer's Disease. The Lancet 357, 608–609. doi:10.1016/s0140-6736(00)04063-0
Lambert, J. C., Araria-Goumidi, L., Myllykangas, L., Ellis, C., Wang, J. C., Bullido, M. J., et al. (2002). Contribution of APOE Promoter Polymorphisms to Alzheimer's Disease Risk. Neurology 59, 59–66. doi:10.1212/wnl.59.1.59
Leduc, V., Legault, V., Dea, D., and Poirier, J. (2011). Normalization of Gene Expression Using SYBR green qPCR: a Case for Paraoxonase 1 and 2 in Alzheimer's Disease Brains. J. Neurosci. Methods 200, 14–19. doi:10.1016/j.jneumeth.2011.05.026
Lefterov, I., Wolfe, C. M., Fitz, N. F., Nam, K. N., Letronne, F., Biedrzycki, R. J., et al. (2019). APOE2 Orchestrated Differences in Transcriptomic and Lipidomic Profiles of Postmortem AD Brain. Alz Res. Ther. 11, 113. doi:10.1186/s13195-019-0558-0
Loseva, E., Yuan, T.-F., and Karnup, S. (2009). Neurogliogenesis in the Mature Olfactory System: a Possible Protective Role against Infection and Toxic Dust. Brain Res. Rev. 59, 374–387. doi:10.1016/j.brainresrev.2008.10.004
Ma, C., Zhang, Y., Li, X., Zhang, J., Chen, K., Liang, Y., et al. (2016). Is There a Significant Interaction Effect between Apolipoprotein E Rs405509 T/T and ε4 Genotypes on Cognitive Impairment and gray Matter Volume? Eur. J. Neurol. 23, 1415–1425. doi:10.1111/ene.13052
Martínez-Morillo, E., Hansson, O., Atagi, Y., Bu, G., Minthon, L., Diamandis, E. P., et al. (2014). Total Apolipoprotein E Levels and Specific Isoform Composition in Cerebrospinal Fluid and Plasma from Alzheimer's Disease Patients and Controls. Acta Neuropathol. 127, 633–643. doi:10.1007/s00401-014-1266-2
Miller, S. A., Dykes, D. D., and Polesky, H. F. (1988). A Simple Salting Out Procedure for Extracting DNA from Human Nucleated Cells. Nucl. Acids Res. 16, 1215. doi:10.1093/nar/16.3.1215
Modrego, P. J., and Ferrández, J. (2004). Depression in Patients with Mild Cognitive Impairment Increases the Risk of Developing Dementia of Alzheimer Type. Arch. Neurol. 61, 1290–1293. doi:10.1001/archneur.61.8.1290
Mosconi, L., De Santi, S., Brys, M., Tsui, W. H., Pirraglia, E., Glodzik-Sobanska, L., et al. (2008). Hypometabolism and Altered Cerebrospinal Fluid Markers in normal Apolipoprotein E E4 Carriers with Subjective Memory Complaints. Biol. Psychiatry 63, 609–618. doi:10.1016/j.biopsych.2007.05.030
Negoias, S., Croy, I., Gerber, J., Puschmann, S., Petrowski, K., Joraschky, P., et al. (2010). Reduced Olfactory Bulb Volume and Olfactory Sensitivity in Patients with Acute Major Depression. Neuroscience 169, 415–421. doi:10.1016/j.neuroscience.2010.05.012
Ownby, R. L., Crocco, E., Acevedo, A., John, V., and Loewenstein, D. (2006). Depression and Risk for Alzheimer Disease. Arch. Gen. Psychiatry 63, 530–538. doi:10.1001/archpsyc.63.5.530
Prince, M. J. (2015). World Alzheimer Report 2015: The Global Impact of Dementia: an Analysis of Prevalence, Incidence, Cost and Trends.
Profenno, L. A., Porsteinsson, A. P., and Faraone, S. V. (2010). Meta-analysis of Alzheimer's Disease Risk with Obesity, Diabetes, and Related Disorders. Biol. Psychiatry 67, 505–512. doi:10.1016/j.biopsych.2009.02.013
Purcell, S., Neale, B., Todd-Brown, K., Thomas, L., Ferreira, M. A. R., Bender, D., et al. (2007). PLINK: a Tool Set for Whole-Genome Association and Population-Based Linkage Analyses. Am. J. Hum. Genet. 81, 559–575. doi:10.1086/519795
Rajasekhar, K., and Govindaraju, T. (2018). Current Progress, Challenges and Future Prospects of Diagnostic and Therapeutic Interventions in Alzheimer's Disease. RSC Adv. 8, 23780–23804. doi:10.1039/c8ra03620a
Siehler, J., Blöchinger, A. K., and Lickert, H. (2020). Pharmacological Targeting of the Actin Cytoskeleton to Drive Endocrinogenesis. Trends Pharmacol. Sci. 41, 384–386. doi:10.1016/j.tips.2020.04.002
Song, C., and Leonard, B. E. (2005). The Olfactory Bulbectomised Rat as a Model of Depression. Neurosci. Biobehavioral Rev. 29, 627–647. doi:10.1016/j.neubiorev.2005.03.010
Styren, S. D., Dekosky, S. T., Rogers, J., and Mufson, E. J. (1993). Epidermal Growth Factor Receptor Expression in Demented Elderly: Localization to Vascular Endothelial Cells of Brain, Pituitary and Skin. Brain Res. 615, 181–190. doi:10.1016/0006-8993(93)90028-l
Styren, S. D., Mufson, E. J., Styren, G. C., Harold Civin, W., and Rogers, J. (1990). Epidermal Growth Factor Receptor Expression in Demented and Aged Human Brain. Brain Res. 512, 347–352. doi:10.1016/0006-8993(90)90647-t
Sun, X., Steffens, D. C., Au, R., Folstein, M., Summergrad, P., Yee, J., et al. (2008). Amyloid-Associated Depression. Arch. Gen. Psychiatry 65, 542–550. doi:10.1001/archpsyc.65.5.542
Takei, N., Miyashita, A., Tsukie, T., Arai, H., Asada, T., Imagawa, M., et al. (2009). Genetic Association Study on in and Around the APOE in Late-Onset Alzheimer Disease in Japanese. Genomics 93, 441–448. doi:10.1016/j.ygeno.2009.01.003
Takeuchi, F., Yanai, K., Morii, T., Ishinaga, Y., Taniguchi-Yanai, K., Nagano, S., et al. (2005). Linkage Disequilibrium Grouping of Single Nucleotide Polymorphisms (SNPs) Reflecting Haplotype Phylogeny for Efficient Selection of Tag SNPs. Genetics 170, 291–304. doi:10.1534/genetics.104.038232
Talwar, P., Grover, S., Sinha, J., Chandna, P., Agarwal, R., Kushwaha, S., et al. (2017). Multifactorial Analysis of a Biomarker Pool for Alzheimer Disease Risk in a North Indian Population. Dement Geriatr. Cogn. Disord. 44, 25–34. doi:10.1159/000477206
Talwar, P., Silla, Y., Grover, S., Gupta, M., Agarwal, R., Kushwaha, S., et al. (2014). Genomic Convergence and Network Analysis Approach to Identify Candidate Genes in Alzheimer's Disease. BMC Genomics 15, 199. doi:10.1186/1471-2164-15-199
Talwar, P., Sinha, J., Grover, S., Rawat, C., Kushwaha, S., Agarwal, R., et al. (2016). Dissecting Complex and Multifactorial Nature of Alzheimer's Disease Pathogenesis: a Clinical, Genomic, and Systems Biology Perspective. Mol. Neurobiol. 53, 4833–4864. doi:10.1007/s12035-015-9390-0
Van Cauwenberghe, C., Van Broeckhoven, C., and Sleegers, K. (2016). The Genetic Landscape of Alzheimer Disease: Clinical Implications and Perspectives. Genet. Med. 18, 421–430. doi:10.1038/gim.2015.117
Visscher, P. M., Yengo, L., Cox, N. J., and Wray, N. R. (2021). Discovery and Implications of Polygenicity of Common Diseases. Science 373, 1468–1473. doi:10.1126/science.abi8206
Visser, P. J., Verhey, F. R. J., Ponds, R. W. H. M., Kester, A., and Jolles, J. (2000). Distinction between Preclinical Alzheimer's Disease and Depression. J. Am. Geriatr. Soc. 48, 479–484. doi:10.1111/j.1532-5415.2000.tb04992.x
Wang, L., Chiang, H.-C., Wu, W., Liang, B., Xie, Z., Yao, X., et al. (2012). Epidermal Growth Factor Receptor Is a Preferred Target for Treating Amyloid- -induced Memory Loss. Proc. Natl. Acad. Sci. 109, 16743–16748. doi:10.1073/pnas.1208011109
Wightman, D. P., Jansen, I. E., Savage, J. E., Shadrin, A. A., Bahrami, S., Holland, D., et al. (2021). A Genome-wide Association Study with 1,126,563 Individuals Identifies New Risk Loci for Alzheimer's Disease. Nat. Genet. 53, 1276–1282. doi:10.1038/s41588-021-00921-z
Wong, R. W. C., and Guillaud, L. (2004). The Role of Epidermal Growth Factor and its Receptors in Mammalian CNS. Cytokine Growth Factor. Rev. 15, 147–156. doi:10.1016/j.cytogfr.2004.01.004
Yamazaki, Y., Zhao, N., Caulfield, T. R., Liu, C.-C., and Bu, G. (2019). Apolipoprotein E and Alzheimer Disease: Pathobiology and Targeting Strategies. Nat. Rev. Neurol. 15, 501–518. doi:10.1038/s41582-019-0228-7
Zhang, C., and Pierce, B. L. (2014). Genetic Susceptibility to Accelerated Cognitive Decline in the US Health and Retirement Study. Neurobiol. Aging 35, 1512 e1511–8. doi:10.1016/j.neurobiolaging.2013.12.021-
Keywords: Alzheimer’s disease, genetics, single-nucleotide polymorphism (SNP), APOE, EGFR, ACTB
Citation: Talwar P, Kushwaha S, Rawat C, Kaur H, Srivastava A, Agarwal R, Chandna P, Tucci P, Saso L and Kukreti R (2021) Validating a Genomic Convergence and Network Analysis Approach Using Association Analysis of Identified Candidate Genes in Alzheimer’s Disease. Front. Genet. 12:722221. doi: 10.3389/fgene.2021.722221
Received: 18 June 2021; Accepted: 10 November 2021;
Published: 09 December 2021.
Edited by:
Son Tung Ngo, Ton Duc Thang University, VietnamReviewed by:
Jan Graffelman, Universitat Politecnica de Catalunya, SpainBoon Pin Kee, University of Malaya, Malaysia
Copyright © 2021 Talwar, Kushwaha, Rawat, Kaur, Srivastava, Agarwal, Chandna, Tucci, Saso and Kukreti. This is an open-access article distributed under the terms of the Creative Commons Attribution License (CC BY). The use, distribution or reproduction in other forums is permitted, provided the original author(s) and the copyright owner(s) are credited and that the original publication in this journal is cited, in accordance with accepted academic practice. No use, distribution or reproduction is permitted which does not comply with these terms.
*Correspondence: Ritushree Kukreti, ritus@igib.in
†These authors have contributed equally to this work