Projecting the future redistribution of Pinus koraiensis (Pinaceae: Pinoideae: Pinus) in China using machine learning
- 1Hubei Key Laboratory of Biological Resources Protection and Utilization, Hubei Minzu University, Enshi City, Hubei, China
- 2College of Forestry and Horticulture, Hubei Minzu University, Enshi City, Hubei, China
- 3State Key Laboratory for Conservation and Utilization of Subtropical Agro-Bioresources, Guangdong Key Laboratory for Innovative Development and Utilization of Forest Plant Germplasm, College of Forestry and Landscape Architecture, South China Agricultural University, Guangzhou, China
Introduction: As an important coniferous tree in northeast China, Pinus koraiensis not only maintains the stability of the forest ecosystem at high latitudes but also plays a crucial role in regional socioeconomic development. With the intensification of climate change in recent years, the stability of P. koraiensis habitats is constantly disturbed by external uncertain environmental factors, which greatly affects the geographical distribution of P. koraiensis. However, its geographical distribution is still unclear, which greatly hinders further understanding of the ecological process of P. koraiensis. Consequently, it is particularly important to explore the potential distribution and migration of P. koraiensis during several critical periods.
Methods: Random forest (RF) was used to establish the redistribution of P. koraiensis.
Results: The results showed that temperature seasonality and precipitation in the coldest quarter were the key factors limiting the current distribution of P. koraiensis. Currently, P. koraiensis is mainly distributed in the Lesser Khingan Mountains and Changbai Mountains, with a total suitable area of ~4.59 × 105 km2. In the past, the historical distribution of P. koraiensis during the LIG period was basically consistent with the current distribution range, but its distribution range was more complete. In the LGM period, the suitable distribution of P. koraiensis became fragmented, especially at the connection between the Lesser Khingan Mountains and the Changbai Mountains. Under future climate scenarios, the suitable distribution of P. koraiensis is projected to increase, while the highly suitable distribution will be reduced. The dramatically worrying change is that the suitable habitats of P. koraiensis are gradually breaking and separating in the junction zone between the Lesser Khingan Mountains and Changbai Mountains, which will cause the ecological corridor to break. The shifts in the distribution centroid indicated that the P. koraiensis population will migrate northward.
Discussion: However, it remains to be verified whether long-distance migration can be achieved without human assistance. Our results can provide some solutions for protection and management strategies for P. koraiensis populations and the impact of climate change, shedding light on the effectiveness of management responses.
1 Introduction
The sign of global warming has been remarkable over the past century. It is expected that global temperatures will rise by 1.1–6.4°C in the 21st century (Sanjay et al., 2017). An increasing number of climate warming events are first affecting environmentally sensitive areas, especially in high-latitude and high-altitude regions (IPCC, 2014). Vegetation, due to its rapid responses to changing environmental conditions, was the first area to receive attention from researchers to investigate climate change (Tinner et al., 2013). In the Northern Hemisphere, vegetation was projected to expand toward polar and high altitudes with climate warming (Ernakovich et al., 2014). In high-latitude regions, the possible distribution range and optimal distribution range of Khingan larch in the Greater Khingan Mountains and spruce, fir, and redwood in the eastern mountains of the Lesser Khingan Mountains from 1961 to 2010 shifted northward (Zhao et al., 2013; Ma et al., 2014). The distribution range of evergreen broad-leaved forests in eastern China has expanded to the north (Fan et al., 2022). The forests in Northeast China are the largest forest distribution area in the high-latitude area of China and the central distribution area of broad-leaved Korean pine (Pinus koraiensis Siebold and Zucc.) forest (Ji, 2010; Lyu et al., 2017). As an excellent timber and economic tree species, P. koraiensis not only maintains and conserves soil and water but also plays an important role in regional socioeconomic development (Aizawa et al., 2012). Indeed, climate change has led to a certain degree of reduction to the distribution range of the P. koraiensis population in isolated patches. In addition, the migration ability of P. koraiensis is limited due to its phenotypic plasticity, seed dispersal ability, reproductive capacity, and degree of range fragmentation, making it unable to occupy new ecological niches, resulting in a serious decline in these population. Consequently, the populations in these isolated patches are difficult to recover through natural regeneration (Liang et al., 2018; Liu et al., 2023). In addition, climate-induced changes in the location of suitable habitats may cause the distribution of P. koraiensis to deviate from existing protected areas, and the reduction in the P. koraiensis population caused by human destruction in non-protected areas must also be carefully considered (Araújo et al., 2004; Heller and Zavaleta, 2009). Therefore, it is particularly important to explore the potential distribution of P. koraiensis under climate change and formulate relevant protection strategies.
Species distribution models (SDMs) have been widely applied to select the key environmental variables correlated with species distribution and quantitatively determine the effects of climate change on species habitats (Austin, 2007). Currently, commonly used models include the maximum entropy model (MaxEnt), random forest (RF), surface range envelope (SRE; usually called BIOCLIM), generalized additive model (GAM), Bayesian models (BA), genetic algorithm for rule-set prediction (GARP) and ecological niche factor analysis (ENFA) (Hirzel et al., 2002; Beaumont et al., 2005; Elith et al., 2006; Sánchez-Flores, 2007; Rong et al., 2019). Among them, RF with machine learning performance are being broadly used to predict the potential distribution of species because of their advantages, such as human-computer interaction, effective development of intelligent systems, and reduction of human burden (Janiesch et al., 2021). RF is based on decision trees and incorporates random attribute selection during training through bagging technology, generating a large number of classification and regression trees, and then performing regression analysis on selected subsamples. It is an integrated machine learning method that circumvents the previously common pitfall of overfitting in machine learning models, obtaining a more accurate and stable prediction (Breiman, 2001). Besides, the RF is very effective in processing large amounts of data, can deal well with missing data, can effectively solve nonlinear, interaction, collinearity and other problems, can directly measure the importance of variables, and thereby has received widespread attention in the prediction of species distribution (Bi et al., 2013; Bradter et al., 2013). Benefiting from its broad application prospects, increasing research has also proven the reliability and accuracy of RF in predicting species distributions (Sahragard et al., 2018; Zhang et al., 2020). Kaky et al. (2020) employed eight different models, including support-vector machine (SVM), classification and regression trees (CART), MaxEnt, RF, boosted regression trees (BRT), flexible discriminant analysis (FDA), maximum likelihood estimation (MLE) and generalized linear model (GLM), to simulate and compare the potential geographic distributions of medicinal plants in Egypt, with their results indicating that RF has the highest predictive performance. Amiri et al. (2020) compared five species distribution models, covering classification tree analysis (CTA), multivariate adaptive regression splines (MARS), GLM, RF and MaxEnt, to predict the distribution of Artemisia species in central Iran, finding that the projected results of RF had the highest accuracy among these models. Stock et al. (2020) used GAM and RF models to predict the distribution of six fish species, concluding that the RF exhibited superior predictive performance. Therefore, RF was used as predictive models for the potential distribution of target species in climate change scenarios in this study.
In China, P. koraiensis was included in the “International Union for Conservation of Nature (IUCN) red list” in 2013, with a protection level of Vulnerable (VU). According to historical investigation, the natural conditions of the Lesser Khingan Mountains are the most suitable for the growth of P. koraiensis, andwere preliminarily collected according to the field sampling more than half of the world's P. koraiensis population is distributed here (Li et al., 2020). However, in 1948, the natural P. koraiensis forest located in the Lesser Khingan Mountains started to decreased from 1.2 million hectares to <5 × 104 hectares after 56 years of logging, with only ~3 million mature trees remaining (Guo et al., 2012). As a constructive tree species in the original broad-leaved P. koraiensis forest in Northeast China, the number of P. koraiensis in the original forest has decreased significantly due to long-term destructive interference, and many broad-leaved P. koraiensis forests have degenerated into secondary forests (Lin et al., 2020; Wang et al., 2021). In addition, there is a lack of P. koraiensis seed sources around a large area of secondary forests, or even if there are P. koraiensis seed sources (with a small amount of artificial P. koraiensis forests around the secondary forest), interference from pine fruit picking and other activities can create obstacles to the natural regeneration of P. koraiensis due to insufficient seed sources (Li et al., 2012; Wang et al., 2022). After the cones of P. koraiensis mature and fall off, the trees must rely on rodents to spread the seeds (large-seeded pine species) and complete the subsequent regeneration process, which is also one of the reasons for the obstacles in P. koraiensis regeneration. In the context of global warming, the natural regeneration and regeneration capacity of P. koraiensis forests located in high latitude areas will inevitably continue to decrease. Therefore, it is crucial to determine whether the P. koraiensis population can track to climate change. To address this question, this study will employ RF to project the redistribution and migration of P. koraiensis under future climate scenarios. This research has three objectives: (1) identify the critical environmental variables limiting the current distribution range of the P. koraiensis population, (2) project the future redistribution of P. koraiensis in response to varying climate change scenarios and track the shifts in the potential distributions of this species, and (3) formulate protection and management strategies based on the above conclusions and the current situation of P. koraiensis.
2 Materials and methods
2.1 P. koraiensis presence records
We gathered the presence data of P. koraiensis through three methods. First, field investigations of P. koraiensis were implemented from 2021 to 2023 with the assistance of the Department of Forestry. The distance between the investigated points was >20 km. For each point of presence, the storing information with the location's name, longitude, latitude, and altitude was recorded. Second, we downloaded and arranged presence data from the websites of the Global Biodiversity Information1 (GBIF), Chinese Virtual Herbarium2 (CVH), China National Forest Resource Continuous Investigation Platform,3 China's Nature Reserve Specimen Resource Sharing Platform,4 and China's Teaching Specimen Resource Sharing Platform.5 Third, we collected relevant occurrence records from literature. We then eliminated occurrence data with incomplete geographic information or minimal geographic coordinate differences, and initially collected 239 presence points of the P. koraiensis. Furthermore, to eliminate sample error due to records with overfitting and unclear spatial information, we used the “Spatial rarity Occurrence Data” tool in the SDM toolbox of ArcGIS 10.4 (Esri, Environmental Systems Research Institute) to filter distribution points, and only one site per 10 × 10 square kilometers was retained. Finally, 109 filtered records constituted the presence data for P. koraiensis (Figure 1).
2.2 Environmental data
The environmental data consisted of 19 bioclimatic variables and three topographic variables (Table 1). Climate data from the global climate (WorldClim 1.46) were downloaded and used as climate variables, and a resolution of 30 “climate variables was chosen, including three periods of past, present and future (The resolution for all periods has been uniformly calibrated and standardized). The terrain data are derived from the digital elevation model (DEM) data of the geospatial data cloud7 with a spatial resolution of 30 m, including elevation, slope and aspect. We used ArcGIS 10.4 to convert the elevation layer into slope and aspect layers. We accessed the National Fundamental Geographic Information System8 and downloaded the National Map as the analytical base map.
The current climate data (1950–2000) are obtained by using the thin plate smoothing spline function interpolation method on the observed data of meteorological stations all over the world (Hijmans et al., 2005). For past periods, we selected the Last Interglacial period (~120,000–140,000 years ago, LIG, similar to the current climate) and the Last Glacial Maximum period (~22,000 years ago, LGM) in paleoclimatic data to predict the distribution of past critical periods. The original data were derived from the Coupled Model Intercomparison Project (CMIP5), and the climate conditions during the LIG and LGM were reconstructed using similar data from the CCSM4 climate model framework (Hasumi and Emori, 2004). Typically, general circulation models (GCMs) generate future and past climate scenarios, that have been scaled down and calibrated (deviation-corrected) by WorldClim. The future period includes the 2050s (2041–2060) and the 2070s (2061–2080). The data come from the BCC-CSM1.1 climate model of the Beijing Climate Center, China Meteorological Administration, which is among the global climate models (GCMs) released by the fifth Intergovernmental Panel on Climate Change (IPCC AR5) (Stocker, 2014). The Intergovernmental Panel on Climate Change (IPCC) Fifth Assessment Report (AR5) published Representative concentration pathways (RCPs) with four distinct trajectories of greenhouse gas concentrations: a strict mitigation scenario (RCP2.6), two moderate emission scenarios (RCP4.5, RCP6.0), and a high greenhousegas emission scenario (RCP8.5). These pathways are measured by the total radiative forcing (RF) in the year 2100 compared to the values from the year 1750, representing scenarios where the total radiative forcing in the year 2100 is higher by 2.6, 4.5, 6, and 8.5 W/m2 respectively (Uhorakeye and Möller, 2017). We selected RCP2.6, RCP4.5 and RCP8.5 to project the future distributions of P. koraiensis in the 2050s and 2070s in this study.
To eliminate over-fitting of the models due to multi-collinearity between these environmental factors, two methods were chosen to filter the variables in turn. First, we used the Pearson correlation coefficients (r) to calculate the correlation within environmental variables, with only one (r > 0.8) retained in each set of strongly correlated factors. Furthermore, the retained variables were filtered using the nonzero regression coefficients from the least absolute shrinkage and selection (LASSO) regularized method. LASSO is a method of regression analysis that uses selection and regularization of variables to improve the accuracy and reliability of statistical model predictions. The method stabilizes the model parameters by shrinking the regression coefficients, reducing some of these coefficients to zero (Ning et al., 2021a). The feature selection is carried out after the shrinkage, where every non-zero value is selected for the construction of the model. Accuracy is enhanced as the method incorporates coefficient shrinkage, thereby reducing variance and reducing bias. Ultimately, 10 environmental variables, including Bio2, Bio3, Bio4, Bio5, Bio8, Bio9, Bio13, Bio15, Bio18, and Bio19, were selected to participate in the modeling based on the screening results.
2.3 P. koraiensis distribution predicted by RF
The RF contained in the BioMod2 package in R was used to predict the potential distribution of P. koraiensis in this study. To eliminate sample bias caused by the occurrence records from different sources, we randomly generated pseudo-presence points using ArcGIS 10.4. Finally, a total of 500 presence and pseudo-presence datasets form a point-based input dataset. Then, 10-fold cross-validation process was used to train and validate the model. Of the 10 subsets, one subset was kept for model validation and the remaining subsets were used as training data. In each subset, a single model was trained using 70% of the occurrence data and the remaining data was used to evaluate the predictive performance of the model. The model is then run 10 times for cross-validation, resulting in better accuracy, fewer errors, and more realistic predictions. Concurrently, the weights of environmental variables affecting the geographical distribution of P. koraiensis can be filtered out. Environmental variables with a cumulative contribution of more than 80% (in descending order of weight) were selected as pivotal variables. Ultimately, a more accurate and real distribution of P. koraiensis was obtained.
The output layers were imported into ArcGIS 10.4 for further analysis, and the final distribution map was generated. The habitat suitability index (HSI) was applied to classify the suitable distribution of P. koraiensis. This method was first proposed by the United States Fish and Wildlife Service in the Habitat Assessment Program (Habitat Evaluation Procedures, HEP), and the HSI is widely used to quantify the relationship between an organism's habitat preference and habitat factors (Lu et al., 2012; Zhang et al., 2018). Hence, the potential distribution of P. koraiensis was divided into four categories: unsuitable (0–0.3), marginally suitable (0.31–0.5), moderately suitable (0.51–0.7) and highly suitable (0.71–1). Next, we calculated the suitable area for current, past and future climate scenarios by multiplying the number of grid cells present by their spatial resolution.
We used the AUC, TSS and Kappa value as the evaluation indices of the statistical accuracy of the model (Table 2). AUC refers to the area surrounded by the receiver operating characteristic curve (ROC) and coordinate axis. For indicators quantified by the ROC curve, the range value ranges from 0 to 1. The closer the value is to 1, the higher the accuracy of the model is (Wiley et al., 2003). The kappa coefficient is a statistical index to measure classification accuracy, with a range between −1 and 1. True skill statistics (TSS) is an improved test index derived from the kappa coefficient, and it is mainly used to convert continuous predicted values into binary values and provide an accuracy measure, where its value range is the same as that of the kappa coefficient (Allouche et al., 2006; Hipólito et al., 2015). The closer the value is to 1, the higher the accuracy of the model is.
2.4 The centroid changes using SDM tools from current to future climate scenarios
To measure the change trend of the P. koraiensis distribution, the SDM toolkit9 for ArcGIS 10.4 was used to track the current and future distribution changes in suitable habitat (Brown, 2014). Measuring the centroid shifts of species distributions quantifies the core changes within the range of species distribution to a central point (centroid), then creating a vector file that describes the magnitude and direction of change over time, and generating maps of distribution changes under different climate change scenarios.
3 Results
3.1 Screening of important environmental variables and model performance
Based on the RF results, we obtained the ranking of environmental variables affecting the distribution of P. koraiensis, and selected the variables that reached an 80% cumulative contribution rate. Furthermore, we selected the top five environmental variables in terms of average cumulative contribution rate as the important environmental variables (Figures 2A, B). Five dominant variables with contribution rates varying from large to small were Bio4 (temperature seasonality), Bio19 (precipitation coldest quarter), Bio3 (isothermality), Bio15 (precipitation seasonality), and Bio5 (max temperature of warmest month). In addition, the total contribution rate of environmental variables related to temperature is higher than that of rainfall-related environmental variables among the five important variables, indicating that P. koraiensis is more sensitive to temperature than precipitation, which will help us further comprehend the response of P. koraiensis to climate change.
The obtained AUC, kappa and TSS values of each period based on the prediction of the model are shown in Figure 3. The results show that the model established in this study has high reliability, with AUC values >0.95, kappa values >0.8 and TSS values >0.85 in each period, indicating that the model has relatively high prediction accuracy and accurate prediction results.
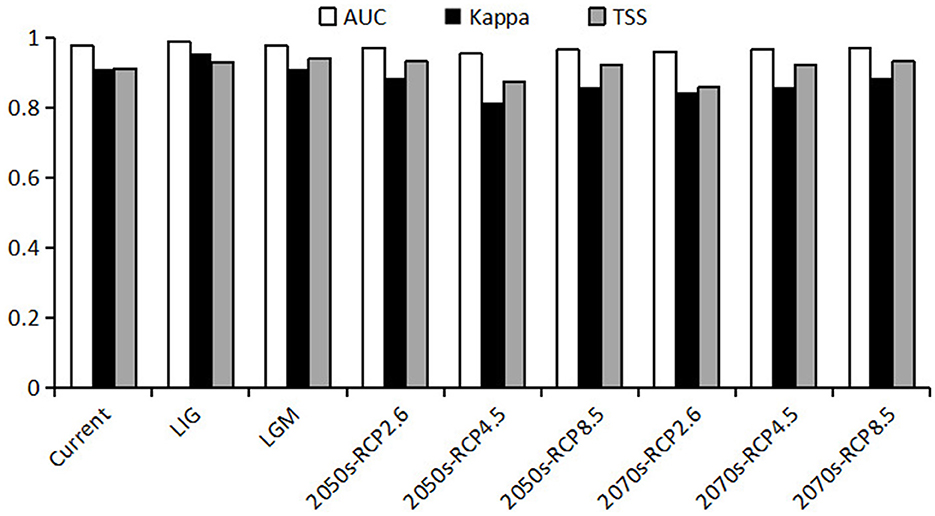
Figure 3. Three indicators for predicting the accuracy of current, past and future climate scenarios for Pinus koraiensis.
3.2 Potential distribution of P. koraiensis in China from the past to the future
3.2.1 Potential distribution of P. koraiensis in the current climate scenarios
The current distribution of P. koraiensis in China is shown in Figure 4, where it is distributed in southeastern Heilongjiang Province, central and eastern Jilin Province, northern Liaoning Province, and northeastern Inner Mongolia. Suitable distribution mainly extends along the Lesser Khingan Mountains to the Changbai Mountains, bordering North Korea. At present, the total suitable area of P. koraiensis is ~4.59 × 105 km2, and the highly suitable area, moderately suitable area, and marginally suitable area are ~3.09 × 105, 6.47 × 104, and 8.52 × 104 km2, respectively (Figure 5).
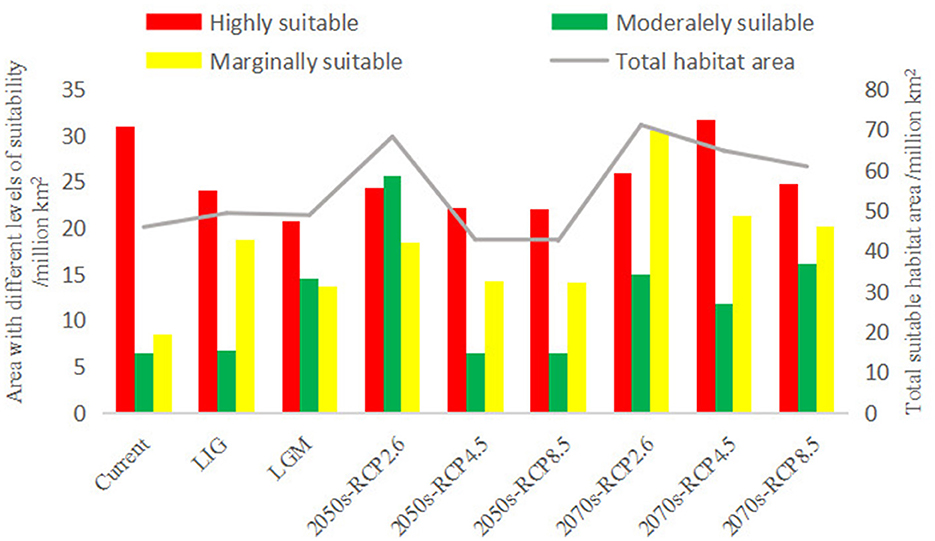
Figure 5. The total suitable area of Pinus koraiensis under different climate scenarios and the suitable area under various categories.
3.2.2 Potential distribution of P. koraiensis in the past
The suitable distribution of P. koraiensis in past climate scenarios is shown in Figure 6. Under the LIG (Figure 6A), the potential distribution of P. koraiensis was mainly distributed in central and eastern Heilongjiang Province, Jilin Province and southeastern Jilin Province, with a total suitable area of ~4.93 × 105 km2. The highly suitable area was ~2.40 × 105 km2, accounting for 48.59% of the total distribution area and showing a continuous and concentrated distribution range.
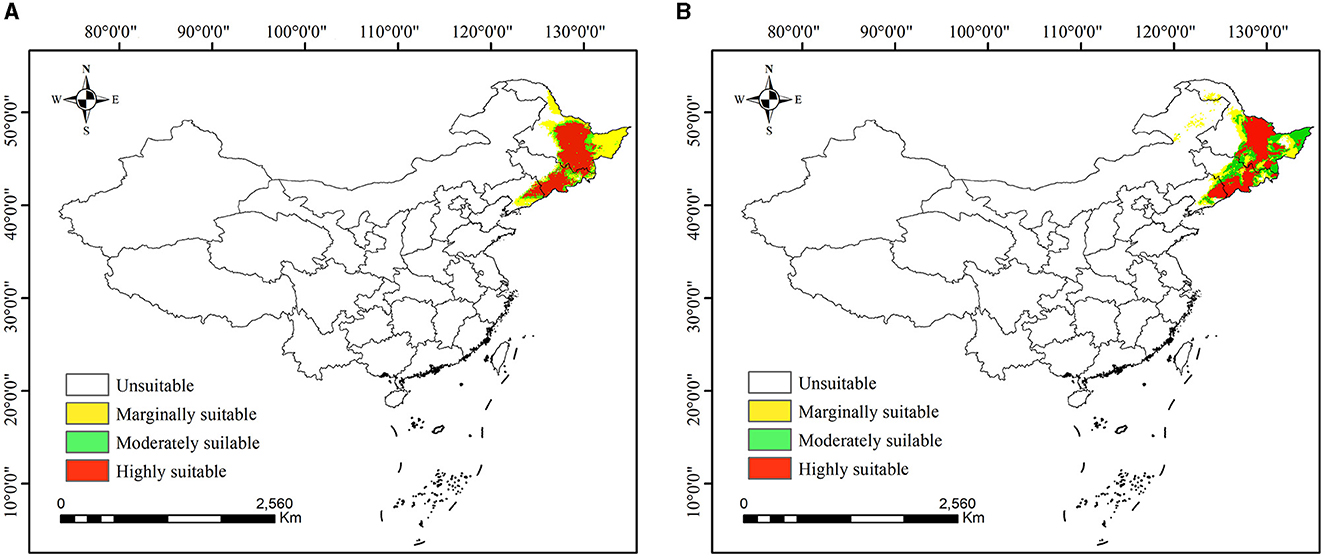
Figure 6. (A, B) Potential distribution of Pinus koraiensis in China under past climate scenarios. (A) In the LIG; (B) In the LGM.
Under the LGM (Figure 6B), the suitable distribution of P. koraiensis gradually showed a fragmented distribution range, with the total area amounting to ~4.89 × 105 km2. The highly, moderately, and marginally suitable areas were ~2.08 × 105, 1.45 × 105, and 1.36 × 105 km2, respectively. Compared with that of the LIG period, the total suitable area decreased by 0.96%, in which the highly suitable area and marginally suitable area decreased by 3.21 × 104 and 5.10 × 104 km2, respectively, while the moderately suitable area increased by 7.84 × 104 km2.
Compared with the current potential distribution, the suitable distribution area of P. koraiensis has decreased since the LIG period. However, the highly suitable distribution increased significantly by an average of 8.54 × 104 km2. From the LIG period to the LGM period, the suitable habitat of P. koraiensis gradually narrowed. In particular, the suitable distribution area at the junction of the Lesser Khingan Mountains and the Changbai Mountains is continuously reduced, forming a fault zone and interrupting the ecological corridor of P. koraiensis migration. Although the suitable distribution has recovered somewhat in the current period, it is generally in a shrinking state, meaning that the suitable distribution of P. koraiensis is facing enormous challenges.
3.2.3 Potential distribution of P. koraiensis in future periods
The future distributions of P. koraiensis are shown in Figure 7. Under most future climate scenarios, the suitable habitat range of P. koraiensis expanded, but the main distribution area in the Lesser Khingan Mountains and Changbai Mountains shrank; in particular, the intersection of the two mountains formed a fault zone, and the distribution expanded in the Greater Khingan Mountains in northern Heilongjiang Province. Overall, the suitable distribution was fragmented, and small patches gradually separated from relatively complete habitats, which is not conducive to the distribution of P. koraiensis.
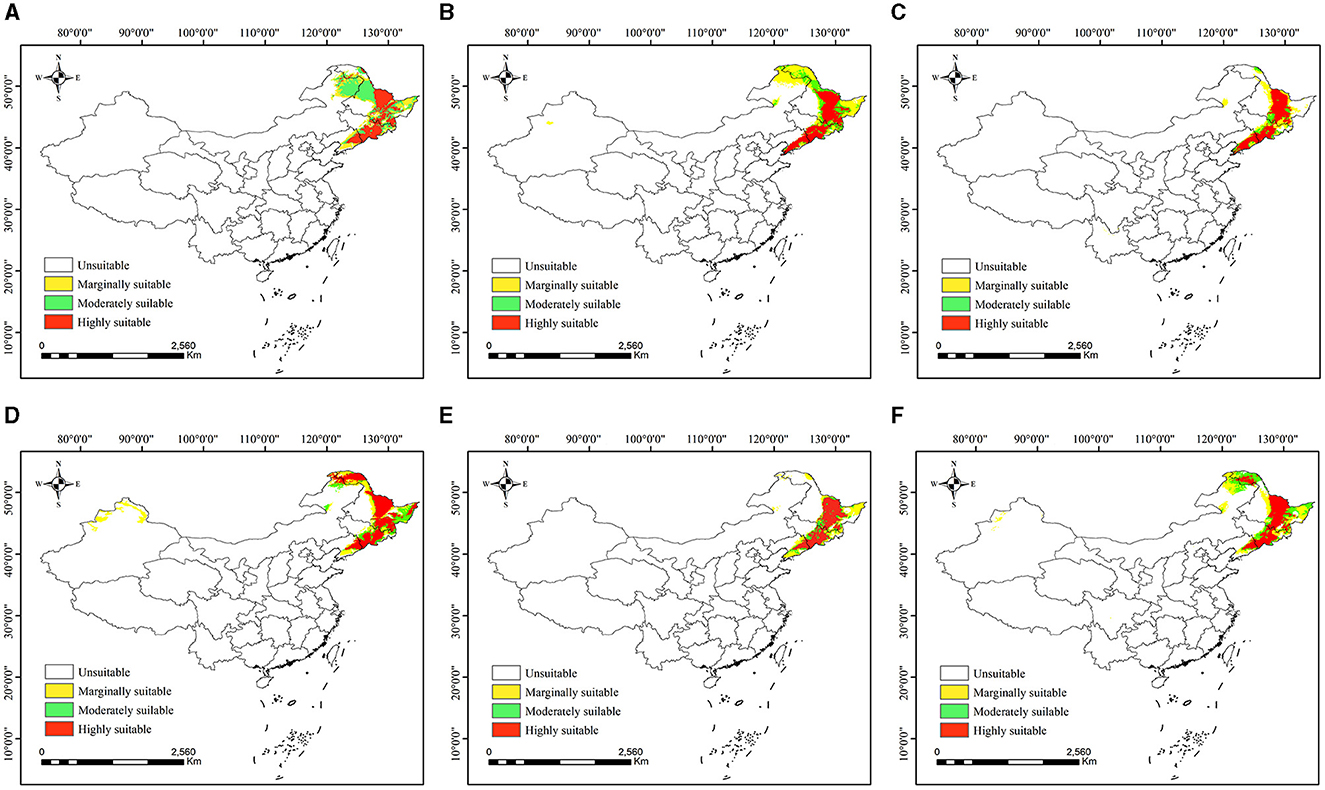
Figure 7. (A–F) Potential distribution of Pinus koraiensis in China under future climate scenarios. (A) RCP2.6-2050s; (B) RCP2.6-2070s (C) RCP4.5-2050s; (D) RCP4.5-2070s (E) RCP8.5-2050s; and (F) RCP8.5-2070s.
Among the three emission paths in the 2050s, under RCP2.6 (Figure 7A), the range of suitable distribution of P. koraiensis spread to the Greater Khingan Mountains in the northern part of Heilongjiang Province. The total suitable area was ~6.83 × 105 km2, in which the areas of high, moderate and marginal suitability were ~2.42 × 105 km2, 2.56 × 105 km2 and 1.85 × 105 km2, respectively. Compared to the current distribution, the overall suitable area has increased by 2.24 × 105 km2. The moderately suitable area and marginally suitable area increased significantly, increasing by 1.91 × 105 km2 and 9.93 × 104 km2, respectively, and the major additions were located in the Lesser Khingan Mountains and Greater Khingan Mountains. However, the highly suitable area decreased by 21.57%.
Under RCP4.5 (Figure 7C), the total suitable area of P. koraiensis was 4.28 × 105 km2, and the areas of highly, moderately and marginally suitable habitats accounted for 51.87%, 14.98% and 33.16%, respectively. In RCP8.5 (Figure 7E), there was no significant difference between the total suitable area of P. koraiensis and RCP4.5, which was ~4.25 × 105 km2, and the highly, moderately and marginally suitable areas accounted for 51.91%, 15.12% and 32.97% of the total suitable areas, respectively. Compared with that under RCP2.6, the total suitable area of P. koraiensis under RCP4.5 and RCP8.5 was greatly reduced, mainly in the Greater Khingan Mountains region. Compared to those under the current climate, the total area and the area of each classification were reduced to varying degrees.
By the 2070s, under the three RCPs, the total suitable area of P. koraiensis increased to different degrees. Under RCP2.6 (Figure 7B), the total suitable area of P. koraiensis reached 7.12 × 105 km2, which is 2.5 × 105 km2 more than that in the current period. The moderately and marginally suitable distributions with areas of 1.5 × 105 and 3.04 × 105 km2 increased, extending to the Greater Khingan Mountains in northwestern Heilongjiang and northeastern Inner Mongolia. However, the highly suitable area was 2.6 × 105 km2, which is 28.66% less than that in the current period, and the main reduction area was located in the Changbai Mountain range at the intersection of Heilongjiang and Jilin Provinces.
Under RCP4.5 (Figure 7D), the total suitable area was 6.48 × 105 km2. Among all climate scenarios, the highly suitable area of RCP4.5 was the largest, reaching 3.16 × 105 km2; it was mainly distributed in northwestern Heilongjiang and in the Greater Khingan Mountains in northeastern Inner Mongolia. The moderately and marginally suitable areas accounted for 18.24 and 32.90% of the total suitable area, respectively, with varying degrees of increase compared with those of the current period, and the new areas were also mainly in the Greater Khingan Mountains region.
Under RCP8.5 (Figure 7F), the highly suitable area was 2.47 × 105 km2, representing a decrease of ~6.24 × 104 km2 compared with the current period. The moderately suitable and marginally suitable areas accounted for 26.34 and 33.14% of the total suitable habitat, respectively. It is worth noting which areas are still in a local expansion state. Different from other climate scenarios, a marginally suitable distribution of fragmented patches appeared in northeastern Xinjiang in the 2070s under the three predicted concentration paths.
3.3 Potential suitable distribution centroid migration of P. koraiensis
The centroid migration map of P. koraiensis distributions is shown Figure 8. At present, the suitable distribution centroid of P. koraiensis is located in the Zhangguangcai-Ridge Mountains, a branch of Changbai Mountains, at the junction of Heilongjiang and Jilin provinces. Under the RCP 2.6 climate scenario, the population centroid will move to southeast Mudanjiang City, Changbai Mountains by the 2050s, and northwest to Yichun City, the Lesser Khingan Mountains by 2070s. The northern boundary will move north by 0.3°-4° in latitude. Under the RCP 4.5 climate scenario, the population centroid will move to southeast Harbin City, south edge of the Lesser Khingan Mountains by the 2050s, and northwest to Yichun City by 2070s. The northern boundary will move north by 1°-3° in latitude. Under the RCP 8.5 climate scenario, the population centroid will move to southeast Harbin City by 2050s, and northwest to Heihe City by 2070s. The northern boundary will move north by 1°-4° in latitude. The direction of centroid migration was different under the future climate change scenarios according to the centroid migration map, while the general trend change is consistent with the transition from low latitude to high latitude.
4 Discussion
P. koraiensis is a dominant species in mixed conifer and broadleaved forests, where in China, it is distributed only along the Changbai Mountains to the Little Khingan Mountains in northeast China, mainly growing on slopes, hills and valleys, typically occurring in mild regions with more than 70% humidity and at altitudes from 600 m to 1,500 m (Li M. et al., 2021). Nearly half of the world's P. koraiensis germplasm resources are located in the Little Khingan Mountains region, Yichun City, China, in which is home to the largest, most primeval forest in Asia, possessing many natural top-tier communities of P. koraiensis (Li X. et al., 2021). Natural P. koraiensis forest absorbs large amounts of CO2 and contributes to climate change regulation, making it a prominent coniferous tree species of great value for the maintenance and protection of the environment in East Asia (Dai et al., 2013; Wang et al., 2023). Therefore, the response of P. koraiensis forests is vital in the face of climate change. The continuous rise in global temperatures may disrupt the existing equilibrium of forests, and without appropriate management and measures, continuous climate change further exacerbates forest degradation.
The prediction results showed that the current suitable range of P. koraiensis is mainly distributed along the Lesser Khingan Mountains to the Changbai Mountains bordering North Korea. According to the field surveys and historical distribution data, the simulated distribution range is basically consistent with the actual distribution area. In the past, the results showed that the historical distribution of P. koraiensis was in a shrinking state compared with the current range, while the fragmentation of the suitable distribution was especially serious in the LGM period. Although the suitable distribution of P. koraiensis has recovered somewhat from the LGM to the present period, it is still in a shrinking state. Under future climate scenarios, the suitable distribution of P. koraiensis will become more fragmented at the connection between the Lesser Khingan Mountains and the Changbai Mountains, causing the ecological corridor to break, which will further block the spread of P. koraiensis. Overall, whether in the past or present, the suitable distribution of P. koraiensis in the ecological corridor between the Lesser Khingan Mountains and the Changbai Mountains is undergoing significant changes. During the Quaternary Glacier, the junction between the Lesser Khingan Mountains and the Changbai Mountains was a corridor for many species to migrate south, and then this hotbed of life gradually returned to the north with climate change (Hewitt, 2000; Lang et al., 2002). There is a gathering of Changbai flora, which is deeply influenced by the flora of North China, and the flora of Mongolia and the Lesser Khingan Mountains are permeated at the same time. Despite the infiltration of southern plants, more than 78% of the seed plants here belong to the temperate distribution of components, occupying an absolute advantage, with typical northern characteristics (Peiyun et al., 1995). Thus, the forest types here are very complex, with a large area of primitive P. koraiensis forests, spruce and fir forests, larch forests, coniferous and broad-leaved mixed forest, white birch forests, poplar-birch forests and other forest types present, but this also intensifies forest competition and succession (Wang X. et al., 2006; Deng et al., 2023). P. koraiensis forest is the top-level community of this forest, which means that the forest succession here will eventually become a P. koraiensis forest without human interference (Yu et al., 2022). However, it is often difficult to find P. koraiensis seedlings in P. koraiensis forests, which means that P. koraiensis as the dominant tree species will eventually be replaced by other tree species. Over time, the suitable areas for the existing P. koraiensis forest will gradually become patchy, while small patches will gradually disappear.
Results from the variable filtering revealed temperature and precipitation as primary limiting factors determining the current distribution of P. koraiensis. An increasing number of studies have found that the distribution of P. koraiensis is closely related to its growth and development, namely, whether it migrates with latitude or altitude (Liu et al., 2016; Petrenko et al., 2022). P. koraiensis is a tree species that is distributed in humid temperate regions and that has strong cold resistance, and there is no frost damage to old or young trees during the winter in the Lesser Khingan Mountains at −30°C (Wang X. P. et al., 2006; Li et al., 2023). Therefore, P. koraiensis has a certain degree of plasticity in temperature requirements under humid conditions, with a greater adaptability to temperature (Yuan et al., 2021; Song et al., 2023). Therefore, its potential geographical distribution seems to be larger than its current natural geographical distribution. As global temperatures rise, the high-latitude climate niche of P. koraiensis seems more suitable for its northward migration trend. Xu and Yan (2001) considered that the latitudinal boundary of the potential distribution of P. koraiensis would move northward. Lyu et al. (2017) deemed that the future warming climate may make the suitable distribution range of P. koraiensis move northward because tree species characteristics and location are the key factors affecting tree growth response to climate change. Precipitation is an important factor that cannot be ignored in the growth and development process of P. koraiensis. During seedling development, due to the underdeveloped root system, P. koraiensis requires a humid environment to grow better, especially during the germination and seedling stages (Zhang et al., 2015; Wang et al., 2021). According to research from Yuan et al. (2021), it is believed that the speed of high growth of P. koraiensis in June is closely related to the magnitude of air humidity, and the rainfall from May to June has a significant impact on the annual high growth. P. koraiensis has a high plasticity in soil moisture requirements, ranging from well-drained moist soil to relatively dry and barren steep slopes where it can develop into forests (Kim et al., 2019). However, it is not suitable for growing on waterlogged land with poor drainage and poor ventilation (Choi, 2008). Therefore, in low-lying valleys with poor drainage, P. koraiensis is often replaced by larch and spruce fir (Yu et al., 2006). According to some studies, temperature and precipitation are considered the key climate factors affecting the composition and structure of P. koraiensis mixed forests (Sun et al., 2021). Zhang et al. (2014) thought that P. koraiensis will continue to exist, but all the forests dominated by P. koraiensis in this area will be transferred and become broad-leaved forests due to global warming and increased precipitation in the future. In addition, a warming climate could exacerbate the death of forest trees by heat-induced drought (Wang et al., 2017; Ning et al., 2022). Studies have shown that climate warming will cause tree species to migrate to regions with higher latitudes and elevations to adapt to climate change (Alexander et al., 2018; You et al., 2018). However, there are many obstacles that may prevent species from migrating to higher latitudes and elevations, such as insufficient habitat, encountering more barriers to dispersal, increasing average annual temperature and decreasing total annual precipitation in lowlands compared to alpine regions (Chen et al., 2011; Zu et al., 2021). Undoubtedly, there are many environmental factors that affect the natural geographical distribution of P. koraiensis under future climate change. Similar to other tree species, P. koraiensis may grow as long as it meets the suitable living environment. Notably, some human factors need to be carefully considered in future climate change research.
Despite our predicted results indicated, which potential changes in the potential distribution of P. koraiensis, it did not furnish absolute projections. There are two key points that must be noted. On the one hand, the growth and regeneration processes of P. koraiensis are very interesting phenomena. Its seedlings are usually far away from the parent tree (Miyaki, 1987). However, P. koraiensis seedlings prefer shade and therefore need mature trees to block sunlight (Zhang et al., 2012). Due to the obstruction of sunlight by large trees, as well as the inhibition of seed germination by secondary metabolites secreted by the roots of P. koraiensis, the growth space of P. koraiensis seedlings is severely compressed (Sun et al., 2016). Therefore, P. koraiensis seeds need to be transported to the broad-leaved or deciduous forests surrounding the P. koraiensis forest by rodents and birds (Hayashida, 1989). The seeds stored by these animals usually germinate together, with four or five seedlings squeezed together to grow (Li et al., 2019). It is not until P. koraiensis individuals reach four or five meters tall that they stand out compared to other trees. Most broad-leaved trees can grow to just over 20 m, while P. koraiensis can grow to over 40 m (Qian et al., 2003). Usually, P. koraiensis and A. nephrolepis are dominant tree species in these forests, forming a unique mixed forest of needled and broad-leaved trees (Nakamura and Krestov, 2005). Regarding wooden stakes created by humans or lightning, stakes will slowly loosen and pulverize under the decomposition of microorganisms and insects, becoming a loose and fertile breeding ground (Stokland et al., 2012). Subsequently, A. nephrolepis seeds appear, and almost every aged P. koraiensis stump grows A. nephrolepis saplings, which can provide the most comfortable seedbed for A. nephrolepis. If there were no stakes, A. nephrolepis seedlings would grow on the naturally fallen trunk of P. koraiensis. These fallen trees, also known as nanny trees, support A. nephrolepis seedings that require a soft substrate. Finally, a unique mixed forest of P. koraiensis and A. nephrolepis is formed (Li and Zhu, 1991). On the other hand, we also need to consider that the spread of P. koraiensis seeds is limited by species dispersal capacity and interspecific competition (Liang et al., 2018). In particular, P. koraiensis is a large-seeded pine, and its main mode of transmission is seeds being carried and buried by rodents, resulting in its short propagation distance and its distribution being limited to the area around the mother tree (Wang et al., 2021). According to previous studies, the distance from the mother tree of pine seeds spread by rodents is no more than 50 m, which means that it is almost impossible for P. koraiensis seeds to achieve long-distance propagation (Wang et al., 2019). In addition, except for P. koraiensis seeds that humans gather, most of the remaining seeds will be eaten by rodents, and a small number of seeds will be cut off by rodents during germination, which is also one of the main reasons for the poor natural renewal ability of P. koraiensis, leading to a decrease in population distribution (Wang et al., 2021).
There are some limitations in our study. First, we were able to use only a small sample size because there may be some areas that have not been investigated due to limitations imposed by mountains and rivers (Beniston, 2003). According to relevant historical materials, most P. koraiensis occurrence records appear in the Little Khingan Mountains and the Changbai Mountains (Zhang et al., 2011). However, some studies have shown that P. koraiensis likely exists in the Greater Khingan Mountains (Cheng and Yan, 2007). The Greater Khingan Mountains in northeast China have the largest intact primary forest with a wide distribution range, and its suitable distribution area may not have been excavated or recorded (Zhao et al., 2020). In addition, in real life, large-scale migration is not possible. Being a large-seeded pine species, P. koraiensis has a short dispersal distance that is limited to the area around the parent trees, and it mainly relies on animal dispersal, which are all adverse factors affecting its dispersal (Ning et al., 2021b). Previous studies have shown that the majority of P. koraiensis seeds scatter within a distance of < 50 m (Wang et al., 2019). Put differently, realization of long-distance seed dispersal events is nearly unfeasible with the existing dispersal range, which would result in zero dispersal on the scale of our models. Additionally, geographical barriers such as mountains and rivers nearly impede communication between species, making short-distance dispersal events predominate within local geographical ranges (Ning et al., 2021a). However, our predictive results indicated that the potential distribution area of P. koraiensis will shift northward, exhibiting a pattern of long-distance migration. There may be a mechanism that enables wider dispersal of P. koraiensis seeds within the local geographic range, but it is currently unclear. Moreover, other studies have also not uncovered substantial evidence of long-distance migration. Therefore, it's necessary to further explore distribution expansion studies related to seed dispersal.
Our study analyzed the effects of climate change on the distribution of P. koraiensis, but in reality, we need to consider not only biological factors such as seed dispersal and interspecific competition but also the effects of forest fires and human footprints on the natural regeneration of P. koraiensis forests. Overall, from the perspective of various factors, climate change has threatened the habitat of P. koraiensis, which is very unfavorable to the population development of P. koraiensis. Therefore, the protection of P. koraiensis is urgent. Protected area networks (conservation reserve systems with fixed boundaries) need to be established as soon as possible. In addition, specific and synchronized management strategies need to be put on the agenda, especially because the location of suitable habitat may deviate from the scope of the protected area due to climate change. First, we should focus on protecting the shrinking part of the suitable distribution area of P. koraiensis while also promoting the spread of P. koraiensis into new suitable habitats by modifying the boundaries of protected areas to incorporate unprotected areas into protected areas. Second, given the actions of climate change, we should strengthen and develop forest strength, find places where P. koraiensis may exist as primary forests, establish new protected areas, and adopt measures such as adjusting and reforming the species composition structure to protect P. koraiensis. Then, in situ conservation strategies should be adopted to protect its habitat, ban timber harvesting, implement sustainable management systems such as thinning and artificial plantation combinations, protect and promote the regeneration of P. koraiensis mother trees, and enhance the protection of existing natural forests to maintain the genetic diversity of this species. Finally, ex situ protection of P. koraiensis should be combined with in situ protection. Ex situ protection means establishing protected areas, while ex situ protection means planting P. koraiensis in unnatural areas, such as by cloning seed orchards and other measures to expand the genetic basis and protect the population of P. koraiensis.
5 Conclusion
The RF was used to predict the potential habitat distribution of P. koraiensis in China under various past, current and future climate scenarios. The results showed that the current distribution of P. koraiensis was mainly distributed in the Lesser Khingan Mountains and Changbai Mountains. Temperature and rainfall are important environmental factors limiting the distribution of P. koraiensis. From LIG to LGM, the potential distribution range of P. koraiensis will decrease, and the habitat will gradually become fragmented from the complete distribution. Under the future climate scenario, the shocking change is that the suitable habitat at the junction of the Lesser Khingan Mountains and Changbai Mountains will gradually become patchy until separated, leading to the break of the ecological corridor for the spread of P. koraiensis seeds. Furthermore, this situation will hinder gene exchange between P. koraiensis populations, leading to local extinction of populations. However, it remains to be verified whether long-distance migration can be achieved without human assistance. Therefore, it is necessary to strengthen conservation measures. Based on the predicted results, the boundary of protected areas can be adjusted depending on the migration direction of P. koraiensis, and new protected areas can be established in unprotected districts. In addition, the P. koraiensis population can be protected through a combination of ex situ conservation and in situ conservation. Furthermore, the protection of existing natural forests of P. koraiensis can be strengthened by banning timber harvesting and implementing sustainable management systems to maintain the genetic diversity of this species.
Data availability statement
The raw data supporting the conclusions of this article will be made available by the authors, without undue reservation.
Author contributions
XC: Writing – original draft, Conceptualization, Data curation, Formal analysis, Investigation, Methodology, Software, Validation, Visualization. KX: Data curation, Investigation, Validation, Writing – original draft. RD: Data curation, Investigation, Project administration, Writing – original draft. LW: Funding acquisition, Investigation, Project administration, Supervision, Validation, Writing – review & editing. LC: Investigation, Project administration, Validation, Writing – review & editing. HN: Conceptualization, Data curation, Methodology, Project administration, Resources, Software, Supervision, Validation, Writing – original draft, Writing – review & editing. XA: Conceptualization, Methodology, Supervision, Writing – review & editing. HC: Conceptualization, Methodology, Writing – review & editing.
Funding
The author(s) declare that financial support was received for the research, authorship, and/or publication of this article. This study was supported by National Natural Science Foundation of China (32260303) and Hubei Key Laboratory of Biologic Resources Protection and Utilization Open Fund Projects for 2023 (KYPT012307).
Acknowledgments
We thank our lab colleagues for their help and discussion of the research in this experiment.
Conflict of interest
The authors declare that the research was conducted in the absence of any commercial or financial relationships that could be construed as a potential conflict of interest.
Publisher's note
All claims expressed in this article are solely those of the authors and do not necessarily represent those of their affiliated organizations, or those of the publisher, the editors and the reviewers. Any product that may be evaluated in this article, or claim that may be made by its manufacturer, is not guaranteed or endorsed by the publisher.
Footnotes
References
Aizawa, M., Kim, Z. S., and Yoshimaru, H. (2012). Phylogeography of the Korean pine (Pinus koraiensis) in northeast Asia: inferences from organelle gene sequences. J. Plant Res. 125, 713–723. doi: 10.1007/s10265-012-0488-4
Alexander, J. M., Chalmandrier, L., Lenoir, J., Burgess, T. I., Essl, F., Haider, S., et al. (2018). Lags in the response of mountain plant communities to climate change. Glob. Chang. Biol. 24, 563–579. doi: 10.1111/gcb.13976
Allouche, O., Tsoar, A., and Kadmon, R. (2006). Assessing the accuracy of species distribution models: prevalence, kappa and the true skill statistic (TSS). J. Appl. Ecol. 43, 1223–1232. doi: 10.1111/j.1365-2664.2006.01214.x
Amiri, M., Tarkesh, M., Jafari, R., and Jetschke, G. (2020). Bioclimatic variables from precipitation and temperature records vs. remote sensing-based bioclimatic variables: which side can perform better in species distribution modeling? Ecol. Inform. 57:101060. doi: 10.1016/j.ecoinf.2020.101060
Araújo, M. B., Cabeza, M., Thuiller, W., Hannah, L., and Williams, P. H. (2004). Would climate change drive species out of reserves? An assessment of existing reserve-selection methods. Glob. Chang. Biol. 10, 1618–1626. doi: 10.1111/j.1365-2486.2004.00828.x
Austin, M. (2007). Species distribution models and ecological theory: a critical assessment and some possible new approaches. Ecol. Modell. 200, 1–19. doi: 10.1016/j.ecolmodel.2006.07.005
Beaumont, L. J., Hughes, L., and Poulsen, M. (2005). Predicting species distributions: use of climatic parameters in BIOCLIM and its impact on predictions of species' current and future distributions. Ecol. Modell. 186, 251–270. doi: 10.1016/j.ecolmodel.2005.01.030
Beniston, M. (2003). Climatic change in mountain regions: a review of possible impacts. Clim. Change 59, 5–31. doi: 10.1023/A:1024458411589
Bi, Y., Xu, J., Li, Q., Guisan, A., Thuiller, W., Zimmermann, N., et al. (2013). Applying BioMod for model-ensemble in species distributions: a case study for Tsuga chinensis in China. Plant Divers. Resour. 35, 647–655. doi: 10.7677/ynzwyj201312127
Bradter, U., Kunin, W. E., Altringham, J. D., Thom, T. J., and Benton, T. G. (2013). Identifying appropriate spatial scales of predictors in species distribution models with the random forest algorithm. Methods Ecol. Evol. 4, 167–174. doi: 10.1111/j.2041-210x.2012.00253.x
Brown, J. L. (2014). SDM toolbox: a python-based GIS toolkit for landscape genetic, biogeographic and species distribution model analyses. Methods Ecol. Evol. 5, 694–700. doi: 10.1111/2041-210X.12200
Chen, I. C., Hill, J. K., Ohlemüller, R., Roy, D. B., and Thomas, C. D. (2011). Rapid range shifts of species associated with high levels of climate warming. Science 333, 1024–1026. doi: 10.1126/science.1206432
Cheng, X., and Yan, X. (2007). Effects of global climate change on forest succession in Daxing'anling of Northeast China. Chin. J. Ecol. 26, 1277–1284. doi: 10.13292/j.1000-4890.2007.023
Choi, D. (2008). Ecophysiological study of the growth of conifers in Korea in acidified soil with elevated CO2: the role of ectomycorrhizal infection. Eurasian J. For. Res. 11, 1–39. doi: 10.1046/j.1365-2567.1996.459542.x
Dai, L., Jia, J., Yu, D., Lewis, B. J., Zhou, L., Zhou, W., et al. (2013). Effects of climate change on biomass carbon sequestration in old-growth forest ecosystems on Changbai Mountain in Northeast China. For. Ecol. Manage. 300, 106–116. doi: 10.1016/j.foreco.2012.06.046
Deng, J., Zhou, W., Dai, L., Yuan, Q., Zhou, L., Qi, L., et al. (2023). The effects of shrub removal on soil microbial communities in primary forest, secondary forest and plantation forest on Changbai Mountain. Microb. Ecol. 85, 642–658. doi: 10.1007/s00248-021-01943-0
Elith, J., Graham, H. C., P. Anderson, R., Dudík, M., Ferrier, S., Guisan, A., et al. (2006). Novel methods improve prediction of species' distributions from occurrence data. Ecography 29, 129–151. doi: 10.1111/j.2006.0906-7590.04596.x
Ernakovich, J. G., Hopping, K. A., Berdanier, A. B., Simpson, R. T., Kachergis, E. J., Steltzer, H., et al. (2014). Predicted responses of arctic and alpine ecosystems to altered seasonality under climate change. Glob. Chang. Biol. 20, 3256–3269. doi: 10.1111/gcb.12568
Fan, D., Lei, S., Liang, H., Yao, Q., Kou, Y., Cheng, S., et al. (2022). More opportunities more species: Pleistocene differentiation and northward expansion of an evergreen broad-leaved tree species Machilus thunbergii (Lauraceae) in Southeast China. BMC Plant Biol. 22:35. doi: 10.1186/s12870-021-03420-9
Guo, X., Zhang, W., and Ren, G. M. (2012). Research on genetic diversity of original Pinus koraiensisin Xiaoxinganling Mountain. For. Eng. 28, 14–16. doi: 10.16270/j.cnki.slgc.2012.01.023
Hasumi, H., and Emori, S. (2004). K-1 coupled GCM (MIROC) description: Center for climate system research. Tokyo: University of Tokyo.
Hayashida, M. (1989). Seed dispersal by red squirrels and subsequent establishment of Korean pine. For. Ecol. Manage. 28, 115–129. doi: 10.1016/0378-1127(89)90064-9
Heller, N. E., and Zavaleta, E. S. (2009). Biodiversity management in the face of climate change: a review of 22 years of recommendations. Biol. Conserv. 142, 14–32. doi: 10.1016/j.biocon.2008.10.006
Hewitt, G. (2000). The genetic legacy of the Quaternary ice ages. Nature 405, 907–913. doi: 10.1038/35016000
Hijmans, R. J., Cameron, S. E., Parra, J. L., Jones, P. G., and Jarvis, A. (2005). Very high resolution interpolated climate surfaces for global land areas. Int. J. Climatol. 25, 1965–1978. doi: 10.1002/joc.1276
Hipólito, J., Hasui, É., and Viana, B. F. (2015). Solving problems involving the distribution of a species of unknown distribution via ecological niche modeling. Nat. Conserv. 13, 15–23. doi: 10.1016/j.ncon.2015.03.001
Hirzel, A. H., Hausser, J., Chessel, D., and Perrin, N. (2002). Ecological-niche factor analysis: how to compute habitat-suitability maps without absence data? Ecology 83, 2027–2036. doi: 10.1890/0012-9658(2002)083[2027:ENFAHT]2.0.CO;2
Janiesch, C., Zschech, P., and Heinrich, K. (2021). Machine learning and deep learning. Electron. Mark. 31, 685–695. doi: 10.1007/s12525-021-00475-2
Ji, Y. (2010). Climate-growth relationships of Korean pine in Heilongjiang and their potential for global warming. Harbin: Northeast Forestry University.
Kaky, E., Nolan, V., Alatawi, A., and Gilbert, F. (2020). A comparison between Ensemble and MaxEnt species distribution modelling approaches for conservation: a case study with Egyptian medicinal plants. Ecol. Inform. 60:101150. doi: 10.1016/j.ecoinf.2020.101150
Kim, J. W., Kim, S. Y., and Choi, B. K. (2019). Alpine vegetation on the Paekdu-San (Changbaishan) summit of the North-east China. J. Plant Biol. 62, 436–450. doi: 10.1007/s12374-019-0305-x
Lang, S., Xiaoyong, C., and Yuanyuan, L. (2002). Glacial refugia and postglacial recolonization patterns of organisms. Acta Ecol. Sin. 22, 1983–1990. doi: 10.3321/j.issn:1000-0933.2002.11.026
Li, D., Jin, Z., Yang, C., Yang, C., and Zhang, M. (2019). Scatter-hoarding the seeds of sympatric forest trees by Apodemus peninsulae in a temperate forest in northeast China. Polish J. Ecol. 66, 382–394. doi: 10.3161/15052249PJE2018.66.4.006
Li, J. Q., and Zhu, N. (1991). Structure and process of Korean pine population in the natural forest. For. Ecol. Manage. 43, 125–135. doi: 10.1016/0378-1127(91)90080-F
Li, M., Deng, G., Shao, X., and Yin, Z. Y. (2021). Precipitation variation since 1748 CE in the central Lesser Khingan Mountains, Northeast China. Ecol. Indic. 129:107969. doi: 10.1016/j.ecolind.2021.107969
Li, X., Liu, X. T., Wei, J. T., Li, Y., Tigabu, M., Zhao, X. Y., et al. (2020). Genetic improvement of Pinus koraiensis in China: current situation and future prospects. Forests 11:148. doi: 10.3390/f11020148
Li, X., Zhao, M., Xu, Y., Li, Y., Tigabu, M., Zhao, X., et al. (2021). Genetic diversity and population differentiation of Pinus koraiensis in China. Horticulturae 7:104. doi: 10.3390/horticulturae7050104
Li, Y. B., Mou, P., Wang, T. M., and Ge, J. (2012). Evaluation of regeneration potential of Pinus koraiensis in mixed pine-hardwood forests in the Xiao Xing'an Mountains, China. J. For. Res. 23, 543–551. doi: 10.1007/s11676-012-0294-9
Li, Z., Wang, C., Luo, D., Hou, E., and Ibrahim, M. M. (2023). Leaf-branch vulnerability segmentation occurs all year round for three temperate evergreen tree species. Plant Physiol. Biochem. 197:107658. doi: 10.1016/j.plaphy.2023.107658
Liang, Y., Duveneck, M. J., Gustafson, E. J., Serra-Diaz, J. M., and Thompson, J. R. (2018). How disturbance, competition, and dispersal interact to prevent tree range boundaries from keeping pace with climate change. Glob. Chang. Biol. 24, e335–e351. doi: 10.1111/gcb.13847
Lin, L., He, J., Xie, L., and Cui, G. (2020). Prediction of the suitable area of the Chinese white pines (Pinus subsect. strobus) under climate changes and implications for their conservation. Forests 11:996. doi: 10.3390/f11090996
Liu, K., Sun, H., He, H. S., and Guan, X. (2023). Seed harvesting and climate change interact to affect the natural regeneration of Pinus koraiensis. Forests 14:829. doi: 10.3390/f14040829
Liu, M., Mao, Z. J., Li, Y., Sun, T., Li, X. H., Huang, W., et al. (2016). Response of radial growth of Pinus koraiensis in broad-leaved Korean pine forests with different latitudes to climatical factors. J. Appl. Ecol. 27, 1341–1352. doi: 10.13287/j.1001-9332.201605.020
Lu, C. Y., Gu, W., Dai, A. H., and Wei, H. Y. (2012). Assessing habitat suitability based on geographic information system (GIS) and fuzzy: a case study of Schisandra sphenanthera Rehd. et Wils. in Qinling Mountains, China. Ecol. Modell. 242, 105–115. doi: 10.1016/j.ecolmodel.2012.06.002
Lyu, S., Wang, X., Zhang, Y., and Li, Z. (2017). Different responses of Korean pine (Pinus koraiensis) and Mongolia oak (Quercus mongolica) growth to recent climate warming in northeast China. Dendrochronologia 45, 113–122. doi: 10.1016/j.dendro.2017.08.002
Ma, J., Hu, Y., Bu, R., Chang, Y., Deng, H., Qin, Q., et al. (2014). Predicting impacts of climate change on the aboveground carbon sequestration rate of a temperate forest in northeastern China. PLoS ONE 9:e96157. doi: 10.1371/journal.pone.0096157
Miyaki, M. (1987). Seed dispersal of the Korean pine, Pinus koraiensis, by the red squirrel, Sciurus vulgaris. Ecol. Res. 2, 147–157. doi: 10.1007/BF02346923
Nakamura, Y., and Krestov, P. V. (2005). “Coniferous forests of the temperate zone of Asia,” in Coniferous Forests, ed. F. A. Andersson (Amsterdam: Elsevier), 163–220.
Ning, H., Ling, L., Sun, X., Kang, X., and Chen, H. (2021a). Predicting the future redistribution of Chinese white pine Pinus armandii Franch. Under climate change scenarios in China using species distribution models. Glob. Ecol. Conserv. 25:e01420. doi: 10.1016/j.gecco.2020.e01420
Ning, H., Tang, M., and Chen, H. (2021b). Impact of climate change on potential distribution of chinese white pine beetle Dendroctonus armandi in China. Forests 12:544. doi: 10.3390/f12050544
Ning, Q. R., Gong, X. W., Li, M. Y., and Hao, G. Y. (2022). Differences in growth pattern and response to climate warming between Larix olgensis and Pinus koraiensis in Northeast China are related to their distinctions in xylem hydraulics. Agricu. For. Meteorol. 312:108724. doi: 10.1016/j.agrformet.2021.108724
Peiyun, F., Jiyun, L., Wei, C., Xinghua, Y., and Chaixia, Z. (1995). Studies on the flora of seed plants from Changbai Mountain. Bull. Bot. Res. 15, 491–500.
Petrenko, T. Y., Korznikov, K. A., Kislov, D. E., Belyaeva, N. G., and Krestov, P. V. (2022). Modeling of cold-temperate tree Pinus koraiensis (Pinaceae) distribution in the Asia-Pacific region: climate change impact. For. Ecosyst. 9:100015. doi: 10.1016/j.fecs.2022.100015
Qian, H., Yuan, X. Y., and Chou, Y. L. (2003). “Forest vegetation of northeast China,” in Forest Vegetation of Northeast Asia, eds. J. Kolbek, M. Šrutek, and E. O. Box (Dordrecht: Springer), 181–230. doi: 10.1007/978-94-017-0143-3_6
Rong, Z., Zhao, C., Liu, J., Gao, Y., Zang, F., Guo, Z., et al. (2019). Modeling the effect of climate change on the potential distribution of Qinghai spruce (Picea crassifolia Kom.) in Qilian Mountains. Forests 10:62. doi: 10.3390/f10010062
Sahragard, H. P., Ajorlo, M., and Karami, P. (2018). Modeling habitat suitability of range plant species using random forest method in arid mountainous rangelands. J. Mt. Sci. 15, 2159–2171. doi: 10.1007/s11629-018-4898-1
Sánchez-Flores, E. (2007). GARP modeling of natural and human factors affecting the potential distribution of the invasives Schismus arabicus and Brassica tournefortii in ‘El Pinacate y Gran Desierto de Altar'Biosphere Reserve. Ecol. Modell. 204, 457–474. doi: 10.1016/j.ecolmodel.2007.02.002
Sanjay, J., Krishnan, R., Shrestha, A. B., Rajbhandari, R., and Ren, G. Y. (2017). Downscaled climate change projections for the Hindu Kush Himalayan region using CORDEX South Asia regional climate models. Adv. Clim. Change Res. 8, 185–198. doi: 10.1016/j.accre.2017.08.003
Song, Y., Li, X., Zhang, M., Xia, G., and Xiong, C. (2023). Effect of cold stratification on the temperature range for germination of Pinus koraiensis. J. For. Res. 34, 221–231. doi: 10.1007/s11676-022-01540-y
Stock, B. C., Ward, E. J., Eguchi, T., Jannot, J. E., Thorson, J. T., Feist, B. E., et al. (2020). Comparing predictions of fisheries bycatch using multiple spatiotemporal species distribution model frameworks. Canad. J. Fish. Aquat. Sci. 77, 146–163. doi: 10.1139/cjfas-2018-0281
Stocker, T. (2014). Climate change 2013: the physical science basis: working group I contribution to the fifth assessment report of the intergovernmental panel on climate change. Cambridge, MA: Cambridge University Press.
Stokland, J. N., Siitonen, J., and Jonsson, B. G. (2012). Biodiversity in Dead Wood. Cambridge, MA: Cambridge University Press. doi: 10.1017/CBO9781139025843
Sun, Y., Henderson, M., Liu, B., and Yan, H. (2021). Directional variability in response of Pinus koraiensis radial growth to climate change. Forests 12:1684. doi: 10.3390/f12121684
Sun, Y., Zhu, J., Sun, O. J., and Yan, Q. (2016). Photosynthetic and growth responses of Pinus koraiensis seedlings to canopy openness: implications for the restoration of mixed-broadleaved Korean pine forests. Environ. Exp. Bot. 129, 118–126. doi: 10.1016/j.envexpbot.2016.02.005
Tinner, W., Colombaroli, D., Heiri, O., Henne, P. D., Steinacher, M., Untenecker, J., et al. (2013). The past ecology of Abies alba provides new perspectives on future responses of Silver Fir forests to global warming. Ecol. Monogr. 83, 419–439. doi: 10.1890/12-2231.1
Uhorakeye, T., and Möller, B. (2017). Impacts of expected climate change on hydropower generation in Rwanda. Afr. J. Eng. Res. 5, 83–96. doi: 10.30918/AJER.53.17.021
Wang, J., Geoff Wang, G., Li, R., Sun, Y., Yu, L., Zhu, J., et al. (2022). Cascading effect of source limitation on the granivore-mediated seed dispersal of Korean pine (Pinus koraiensis) in secondary forest ecosystems. Ecol. Process. 11, 1–13. doi: 10.1186/s13717-021-00352-y
Wang, J., Wang, G. G., Zhang, T., Yuan, J., Yu, L., Zhu, J., et al. (2021). Use of direct seeding and seedling planting to restore Korean pine (Pinus koraiensis Sieb. Et Zucc.) in secondary forests of Northeast China. For. Ecol. Manag. 493:119243. doi: 10.1016/j.foreco.2021.119243
Wang, J., Yan, Q., Lu, D., Diao, M., Yan, T., Sun, Y., et al. (2019). Effects of microhabitat on rodent-mediated seed dispersal in monocultures with thinning treatment. Agric. For. Meteorol. 275, 91–99. doi: 10.1016/j.agrformet.2019.05.017
Wang, X., Fang, J., Tang, Z., and Zhu, B. (2006). Climatic control of primary forest structure and DBH-height allometry in Northeast China. For. Ecol. Manage. 234, 264–274. doi: 10.1016/j.foreco.2006.07.007
Wang, X., Zhang, M., Ji, Y., Li, Z., Li, M., Zhang, Y., et al. (2017). Temperature signals in tree-ring width and divergent growth of Korean pine response to recent climate warming in northeast Asia. Trees 31, 415–427. doi: 10.1007/s00468-015-1341-x
Wang, X. P., Tang, Z. Y., and Fang, J. Y. (2006). Climatic control on forests and tree species distribution in the forest region of Northeast China. J. Integr. Plant Biol. 48, 778–789. doi: 10.1111/j.1744-7909.2006.00294.x
Wang, Y., Qu, L., Tamai, Y., Watanabe, T., and Koike, T. (2023). “Essential role of symbiotic microorganisms supporting forests in east asia under changing environment,” in Chapter: 9. Symbiosis in Nature, ed. E. C. Rigobelo (Croatia: IntechOpen). doi: 10.5772/intechopen.110645
Wiley, E. O., McNyset, K. M., Peterson, A. T., Robins, C. R., and Stewart, A. M. (2003). Niche modeling perspective on geographic range predictions in the marine environment using a machine-learning algorithm. Oceanography 16, 120–127. doi: 10.5670/oceanog.2003.42
Xu, D., and Yan, H. (2001). A study of the impacts of climate change on the geographic distribution of Pinus koraiensis in China. Environ. Int. 27, 201–205. doi: 10.1016/S0160-4120(01)00083-6
You, J., Qin, X., Ranjitkar, S., Lougheed, S. C., Wang, M., Zhou, W., et al. (2018). Response to climate change of montane herbaceous plants in the genus Rhodiola predicted by ecological niche modelling. Sci. Rep. 8:5879. doi: 10.1038/s41598-018-24360-9
Yu, D. P., Zhai, L. J., Wang, Q. L., and Dai, L. M. (2006). Dynamics of dominant tree species in a forest ecotone on the northern slop of Changbai Mountain. J. For. Res. 17, 216–220. doi: 10.1007/s11676-006-0050-0
Yu, P., Zhang, X., Gu, H., Pan, J., and Chen, X. (2022). Soil phosphorus fractions and their availability over natural succession from clear-cut of a mixed broadleaved and Korean pine forest in northeast China. J. For. Res. 33, 1–8. doi: 10.1007/s11676-020-01285-6
Yuan, D., Zhu, L., Cherubini, P., Li, Z., Zhang, Y., Wang, X., et al. (2021). Species-specific indication of 13 tree species growth on climate warming in temperate forest community of northeast China. Ecol. Indic. 133:108389. doi: 10.1016/j.ecolind.2021.108389
Zhang, J., Zhou, Y., Zhou, G., and Xiao, C. (2014). Composition and structure of Pinus koraiensis mixed forest respond to spatial climatic changes. PLoS ONE 9:e97192. doi: 10.1371/journal.pone.0097192
Zhang, M., Yan, Q., and Zhu, J. (2015). Optimum light transmittance for seed germination and early seedling recruitment of Pinus koraiensis: implications for natural regeneration. iForest 8, 853–859. doi: 10.3832/ifor1397-008
Zhang, M., Zhu, J., and Yan, Q. (2012). Seed germination of Pinus koraiensis in response to light regimes caused by shading and seed positions. For. Syst. 21, 426–438. doi: 10.5424/fs/2012213-02721
Zhang, Q., Wei, H., Zhao, Z., Liu, J., Ran, Q., Yu, J., et al. (2018). Optimization of the fuzzy matter element method for predicting species suitability distribution based on environmental data. Sustainability 10:3444. doi: 10.3390/su10103444
Zhang, X., Wang, W. C., Fang, X., Ye, Y., and Zheng, J. (2011). Vegetation of Northeast China during the late seventeenth to early twentieth century as revealed by historical documents. Reg. Environ. Change 11, 869–882. doi: 10.1007/s10113-011-0224-y
Zhang, X., Wei, H., Zhang, X., Liu, J., Zhang, Q., Gu, W., et al. (2020). Non-pessimistic predictions of the distributions and suitability of Metasequoia glyptostroboides under climate change using a random forest model. Forests 11:62. doi: 10.3390/f11010062
Zhao, C. Y., Ying, W., Xiao-Yu, Z., Yan, C., Yu-Lian, L., Da-Ming, S., et al. (2013). Changes in climatic factors and extreme climate events in Northeast China during 1961-2010. Adv. Clim. Change Res. 4, 92–102. doi: 10.3724/SP.J.1248.2013.092
Zhao, F., Liu, Y., and Shu, L. (2020). Change in the fire season pattern from bimodal to unimodal under climate change: the case of Daxing'anling in Northeast China. Agric. For. Meteorol. 291:108075. doi: 10.1016/j.agrformet.2020.108075
Keywords: Pinus koraiensis, climate change, random forest, potential distribution, habitat shifts
Citation: Chen X, Xiao K, Deng R, Wu L, Cui L, Ning H, Ai X and Chen H (2024) Projecting the future redistribution of Pinus koraiensis (Pinaceae: Pinoideae: Pinus) in China using machine learning. Front. For. Glob. Change 7:1326319. doi: 10.3389/ffgc.2024.1326319
Received: 23 October 2023; Accepted: 22 April 2024;
Published: 03 May 2024.
Edited by:
Zuoqiang Yuan, Northernwest Polytechnical University, ChinaReviewed by:
Jianfeng Liu, Chinese Academy of Forestry, ChinaBehlül Güler, Dokuz Eylül University, Türkiye
Copyright © 2024 Chen, Xiao, Deng, Wu, Cui, Ning, Ai and Chen. This is an open-access article distributed under the terms of the Creative Commons Attribution License (CC BY). The use, distribution or reproduction in other forums is permitted, provided the original author(s) and the copyright owner(s) are credited and that the original publication in this journal is cited, in accordance with accepted academic practice. No use, distribution or reproduction is permitted which does not comply with these terms.
*Correspondence: Hang Ning, ninghanghb@163.com; Xunru Ai, hbmyaxru@163.com; Hui Chen, chenhuiyl@163.com