Drivers of Spatiotemporal Eukaryote Plankton Distribution in a Trans-Basin Water Transfer Canal in China
- 1International Joint Laboratory of Watershed Ecological Security and Collaborative Innovation Center of Water Security for Water Source Region of Middle Route Project of South-North Water Diversion in Henan Province, College of Water Resource and Environment Engineering, Nanyang Normal University, Nanyang, China
- 2Qushou Branch Bureau of Construction and Administration Bureau of Middle Route Project of South-North Water Division, Nanyang, China
- 3Department of Environmental Science and Management, Portland State University, Portland, OR, United States
- 4College of Life Sciences, Hainan Normal University, Haikou, China
Planktonic eukaryotes are important components of aquatic ecosystems, and analyses of the whole eukaryotic planktonic community composition and function have far-reaching significance for water resource management. We aimed to understand the spatiotemporal variation and drivers of eukaryotic plankton distribution in the Middle Route Project of the South-to-North Water Diversion in Henan Province, China. Specifically, we examined planktonic assemblages and water quality at five stations along the canal and another one located before the dam in March, June, September, and December 2019. High-throughput sequencing revealed that the eukaryotic plankton community was primarily composed of 53 phyla, 200 genera, and 277 species, with Cryptophyta, Ciliophora, and norank_k_Cryptophyta being the dominant phyla. Redundancy analysis of the eukaryotic community and environmental factors showed that five vital factors affecting eukaryotic plankton distribution were oxidation-reduction potential, nitrate nitrogen, pH, total phosphorus, and water flow velocity. Furthermore, the geographical distribution of eukaryotic communities was consistent with the distance decay model. Importantly, environmental selection dominantly shaped the geographical distribution of the eukaryotic community. In summary, our study elucidates the ecological response of planktonic eukaryotes by identifying the diversity and ecological distribution of planktonic eukaryotes in trans-basin diversion channels.
Introduction
The Middle Route Project (MRP) of the South-to-North Water Diversion (SNWD) in China is the world’s longest inter-basin water diversion project, with a total length of 1,432 km. Since water diversion started in 2014, more than 44 billion m3 of water has been diverted to northern China through open channels, directly benefiting 140 million people and greatly alleviating the water resource shortage in northern China. Since the MRP canal, which is a concrete-bottom channel, does not intersect with other rivers, it is considered as a new closed artificial aquatic system. The community structure of canal planktonic eukaryotes is currently undergoing dynamic evolution and is dependent on the hydrological, hydrodynamic, and nutrient factors. Moreover, the rapid proliferation of biomass of specific groups occurs during specific periods and affects water quality, thereby leading to potential ecological problems (Liang et al., 2017). Therefore, safe and high-quality water in the MRP of the SNWD is a priority and should be ensured by studying the distribution of the planktonic eukaryote community in the MRP canal.
Planktonic eukaryotes primarily include phytoplankton and zooplankton; both are important links that play central roles in the energy flow and material cycle functional in aquatic ecosystems (Yang et al., 2014; Yan et al., 2020). Phytoplankton and zooplankton participate in the biogeochemical processes of aquatic ecosystems, such as the material cycles, by releasing pollutants through catabolism and synthesis. Considering that distribution characteristics and ecological functions provide crucial insights for the management and maintenance of an ecosystem (Bunse and Pinhassi, 2017), studying the community composition and distribution of planktonic eukaryotes is a high-priority area of aquatic ecosystem research.
Aquatic microorganisms are sensitive to water quality and are important indicators for biological monitoring and water quality evaluation. Studies have shown that the community structure of planktonic fungi is affected by water type, pH, temperature, electrical conductivity, and physical-chemical properties of organic matter, including nitrogen and phosphorus levels. Previous research involving cloning and sequencing data from GenBank has confirmed that variations in the nutritional status of the aquatic body altered the community composition of planktonic eukaryotes (Lefranc et al., 2005). This indicated that planktonic eukaryotes can be used to infer the nutrient status of the water body.
Planktonic eukaryote classification has traditionally been performed by optical microscopy and is influenced by multiple factors including sampling conditions, preservation techniques, and the substantial inherent variation and divergence in these organisms, especially at the species level. With the development of DNA sequencing technology (Philippe et al., 2015; Goodwin et al., 2016), high-throughput sequencing has been widely applied in biological evaluation, including the assessment of freshwater microflora and fauna (Stein et al., 2014; Visco et al., 2015; Su et al., 2017; Needham et al., 2018). Compared to traditional taxonomic methods, high-throughput sequencing technology can identify specific DNA fragments efficiently and quickly, providing more reliable and richer biological information (Keck et al., 2017; Vasselon et al., 2017; Zhang et al., 2017).
Few studies have been conducted on the MRP channel (Nong et al., 2020). Previous studies primarily focused on the water source area and the upstream tributaries (Chen et al., 2018; Han et al., 2021). Due to the unique channel structure, low nutrient levels, and continuously disturbed hydrological characteristics, the water conditions of the main MRP canal differ from those of the Danjiangkou Reservoir. Therefore, this study explored the community characteristics and succession rules of planktonic eukaryotes during trans-basin long-distance water diversion; here, we aimed to reveal the key factors driving their evolution to facilitate the prediction of water quality in the MRP of the SNWD. Specifically, we used high-throughput sequencing technology to survey the planktonic eukaryote community structure and to analyze the environmental factors affecting community succession in the MRP in Henan Province, China. These results will provide empirical support for scientific operations and management in similar aquatic conservation projects.
Materials and Methods
Study Area
This study was conducted on the MRP of the SNWD, which starts from Taocha (TC) of Danjiangkou Reservoir (32.67°N–39.98°N, 111.71°E–116.27°E), with an average width of 54 m and an average water depth of 6.95 m. The MRP flows northward for 1,276 km through four provinces to Tuancheng Lake in Beijing, the capital of China. On average, 9.5 billion m3 of water is diverted annually (Zhang et al., 2021). The MRP of the SNWD spans the subtropical humid climate zone and the temperate monsoon climate zone, with distinct seasonal temperature variations and annual average precipitation ranging between 542.7 and 1173.4 mm. Six sampling stations were set up along the MRP of the SNWD to collect and analyze water samples in the canal in Henan Province every 3 months (Table 1 and Figure 1). In 2019, four samples were collected in March (spring), June (summer), September (autumn), and December (winter). Hydrological data were obtained from the management offices located along with the MRP.
Determination of Physical and Chemical Factors of Water Samples
The water samples in this study were collected in accordance with surface water sampling standards under sunny or cloudy weather conditions to minimize the impact of rainfall or other extreme weather on water quality. Physical and chemical variables were determined according to China Surface Water Environmental Quality Standard (GB3838-2002). water temperature (WT), pH, dissolved oxygen (DO), electrical conductivity (Cond), and oxidation-reduction potential (ORP) were measured in the field with a YSI 6920 (YSI Inc., Yellow Springs, OH, United States). Two-liter water samples were collected using a sterilized column water extractor and sent to the laboratory for chemical analysis within 24 h. Permanganate index (CODMn) was determined using the potassium permanganate digestion titration method. Chlorophyll a (Chl a) concentration was spectrophotometrically measured after extraction in 90% ethanol. Total phosphorus (TP) was determined with acidified molybdate to form reduced phosphomolybdenum blue, which was spectrophotometrically measured. Total nitrogen (TN) was assayed using alkaline persulfate digestion and UV spectrophotometry, while ammonia nitrogen (NH4+-N) and nitrate-nitrogen (NO3–-N) were measured by the spectrophotometric method using Nessler’s reagent and thymol spectrophotometry, respectively. Total organic carbon (TOC) was determined using a TOC analyzer (Multi N/C 3100, Analytik Jena Ltd., Germany), concerning the environmental protection standard “Determination of water quality of total organic carbon combustion oxidation—non-dispersive infrared absorption method” (HJ501-2009).
DNA Extraction and Sequencing of Planktonic Eukaryote Samples
First, 1,500 ml of each water sample was filtered through 0.22-μm acetate fiber sterile microporous membrane, and each sample was filtered three times. After filtration, the filter membrane was cut into blocks and placed in a 50-ml sterile centrifugal tube for eukaryotic plankton collection. The total DNA in the sample was extracted using an Omega Water DNA Kit (Omega Bio-Tek, Norcross, GA, United States). The concentration and purity of extracted DNA were determined using a micro-ultraviolet spectrophotometer. To obtain target DNA sequences of different samples, the V4 region of 18S rDNA was obtained from the floating eukaryotes using the universal primers SSU0817F (5′-TTAGCATGGAATAATRRAATAGGA-3′) and 1196R (5′-TCTGGACCTGGTGAGTTTCC-3′). PCR was carried out using TransStart FastPfu DNA Polymerase (TransGen AP221-02; TransGen Biotech) in a 20-μl reaction volume. The reaction conditions for PCR amplification were 95°C for 3 min; 35 cycles of 95°C for 30 s, 55°C for 30 s, and 72°C for 45 s; 72°C for 10 min; and 10°C until stopped. The PCR mixture included 5 × FastPfu Buffer (4 μl), 2.5 mM dNTPs (2 μl), 5 μM forward primer (0.8 μl), 5 μM reverse primer (0.8 μl), FastPfu Polymerase (0.4 μl), BSA (0.2 μl), and DNA template (10 ng), with ddH2O added to attain a final volume of 20 μl. The amplification products of different samples were homogenized to a final concentration of 10 nmol/L and then mixed in the same volume. The sequencing was performed by Shanghai Meiji Biomedical Technology Co., Ltd. (Shanghai, China).
Analysis of Planktonic Eukaryote High-Throughput Data
The extracted DNA samples were sent to Shanghai Meiji Biomedical Technology Co., Ltd., for purification and MiSeq amplification. Flash 1.2.11 was used for paired-end sequence splicing and Fastp 0.19.6 was used for quality control; chimeras were removed to obtain the optimized sequences. The optimized sequences were clustered using Uparse in the SILVA 128/18S eukaryotic database by operational taxonomic unit (out) clustering; an OTU abundance table was established for subsequent analysis, and quantitative insights for microbial ecology (QIIME) was used for bioinformatics analysis. According to the sequence similarity, the ribosomal database project (RDP) classification algorithm was used to classify OTUs with 97% similarity, and the community composition of each sample was evaluated at each classification level. In addition, mothur was used to calculate the Chao, Ace, Simpson, and Shannon index values for alpha diversity analysis. STAMP was used to compare and analyze the differences among eukaryotic community structures of different samples (P < 0.05).
Data Analysis
All data were processed using Excel 2019 (Microsoft Corporation, Redmond, United States). SPSS 25 (IBM SPSS Inc., Chicago, IL, United States) was used to calculate the mean and SD of environmental factors. Detrended correspondence analysis of MiSeq sequencing OTU data showed that the longest gradient length was less than 3; thus, redundancy analysis (RDA) was performed next. Mantel analysis was used to analyze the relationship between eukaryotic community diversity and water physicochemical parameters. Non-metric multidimensional scale analysis (NMDS) and correlation analysis were used to evaluate the community distribution of planktonic eukaryotes. Both NMDS and Mantel analyses were conducted in R 4.0.4 (R Programming Language, University of Auckland, New Zealand). Using the “vegan” package in R, variance partitioning analysis (VPA) and variance decomposition analysis were used to calculate the interpretation rate for geographical distance and environmental selection of the planktonic eukaryote community structure in the canal in Henan Province. The geographic distance based on the principal coordinates of neighbor matrices (PCNM) variables was obtained from the longitude and latitude of each sampling point calculated using the PCNM function of the “vegan” package in R. The Bray–Curtis (community similarity) distance matrix was calculated using the vegdist function of the “vegan” package in R to determine relative species abundance and environmental factors at each sampling site. ArcGIS 10.7 (ESRI, Redlands, CA, United States) was used as a spatial analysis tool to visualize the sampling stations of the canal in Henan Province.
Results
Analysis of Physical and Chemical Properties
During the investigation period, all variables except TN were consistent with the classes I–II water quality of the Surface Water Environmental Quality Standard (GB38382-2002). The mean and standard deviation (SD) of the environmental factors at the six sampling stations are shown in Table 2. WT, Cond, ORP, DO, TP, and NH4+-N greatly fluctuated by season throughout the year, whereas other variables showed no significant fluctuation between seasons (Table 2).
Community Structure Analysis of Planktonic Eukaryotes
A total of 3,504,894 optimized sequences with an average length of 378 bp were obtained from 72 samples collected from six stations in this study (Figure 2).
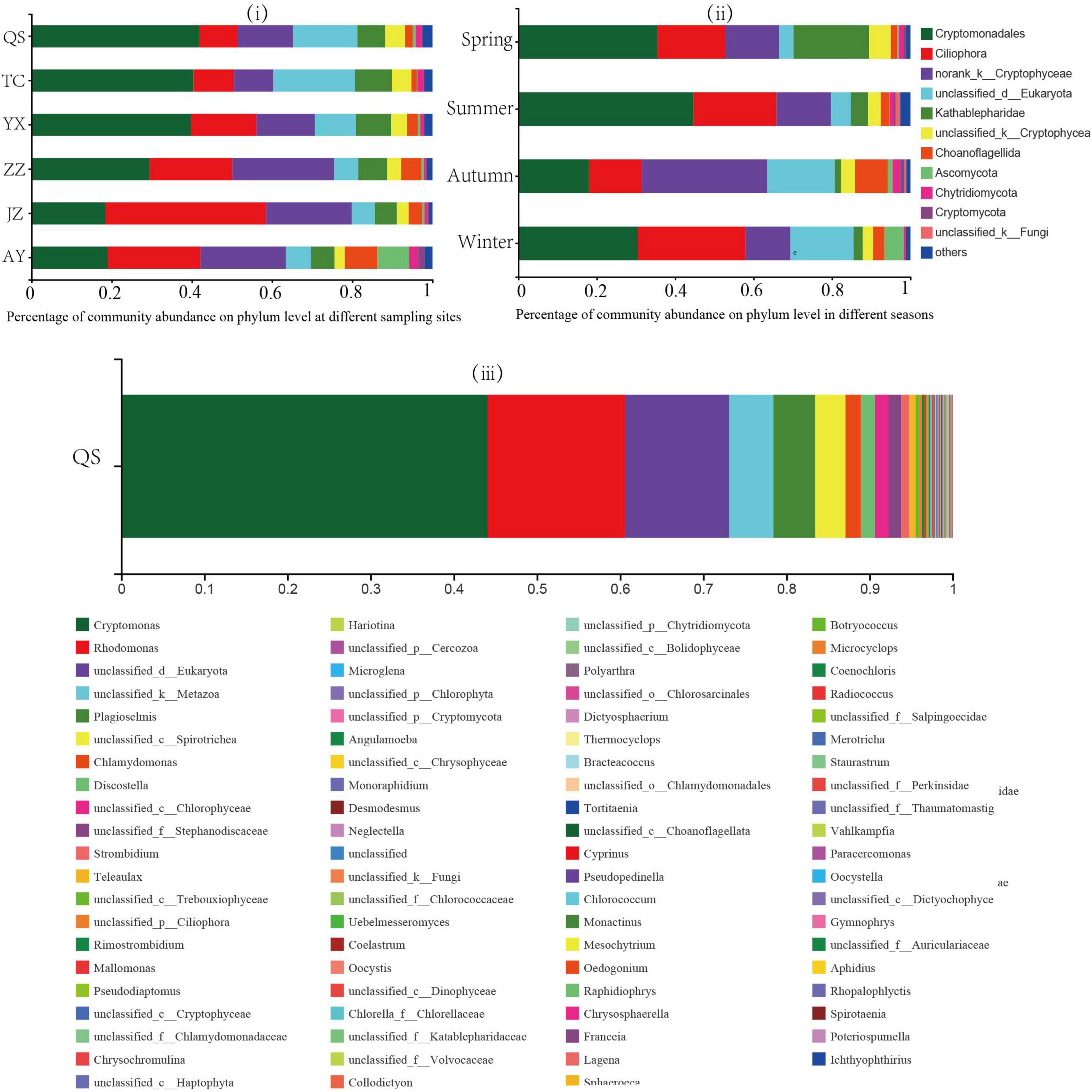
Figure 2. Percent (%) of community abundance at the phylum level. Each color represents a plankton eukaryote species. Community structure of planktonic eukaryotes (i) at each sampling station and (ii) in each season, and (iii) community abundance at QS sampling points.
The plankton eukaryotes of the canal included 53 phyla, 158 families, 200 genera, 277 species, and 754 OTU, among which Cryptophyta, Ciliophora, and norank_k_Cryptophyta were the dominant phyla (Figure 2). Among the OTUs observed from QS to YX, those from YX to ZZ gradually increased, whereas those from YX to ZZ gradually decreased. Additionally, the greatest number of OTUs was detected in summer and the fewest in autumn. Of the various eukaryotes, the relative abundance of Cryptophyta was the highest in QS (41.83%) and the lowest in JZ (18.54%, Figure 2i). The relative abundance of unclassified_d_Eukaryota was the highest in TC (20.37%) and the lowest in JZ (18.54%). The relative abundance of Cryptophyta and unclassified_d_Eukaryota decreased with increased water transport distance. The relative abundance of Ciliophora was the lowest in QS (9.65%) and the highest in JZ (40.02%). The relative abundance of norank_k_Cryptophyta was the lowest in TC (9.72%) and the highest in ZZ (25.37%). The relative abundance of Ciliophora and norank_k_Cryptophyta gradually increased with the distance of water transfer (Figure 2i). The relative abundances of Ascomycota and Choanoflagellida at the AY sampling station were the highest (7.94 and 8.16%, respectively) and were significantly higher than those at the other sampling stations.
Regarding seasonal changes, the relative abundance of Cryptophyta was higher in summer (44.57%, Figure 2ii). The relative abundance of Ciliophora was lowest in autumn (13.57%), whereas those of Choanoflagellida and norank_k_Cryptophyta were the highest in autumn (8.31 and 31.89%, respectively). Other seasonal variations were not significant. Among them, the relative abundance of Kathablepharidae peaked in spring (19.25%), which was significantly higher than that in other seasons. The relative abundance of Ascomycota was 0.24% in summer and 4.82% in winter.
Among these sampling sites, we detected the percentage of community abundance at the genus level at the QS sampling site (Figure 2iii). Among them, Cryptomonas accounted for a large proportion (44.11%), followed by Rhodomonas, accounting for 16.50%.
Analysis of Planktonic Eukaryote Alpha Diversity
The abundance and diversity of planktonic eukaryotes of the canal in Henan Province differed among seasons (Figure 3). Ace and Chao indices of AY were the lowest in winter, Shannon and Simpson indices of ZZ were the lowest in autumn, and Ace, Chao, Shannon, and Simpson indices of QS and TC were the highest in summer (Figures 3iii,iiii). The Shannon and Simpson diversity indices of TC and QS were higher in summer than those of other stations and seasons, which indicated that compared with the changes in hydrological conditions, the eukaryotic community of TC was more significantly affected by the Danjiangkou Reservoir. As water transport distance increased, the number of species in the planktonic eukaryote community gradually decreased (Figures 3i,ii).
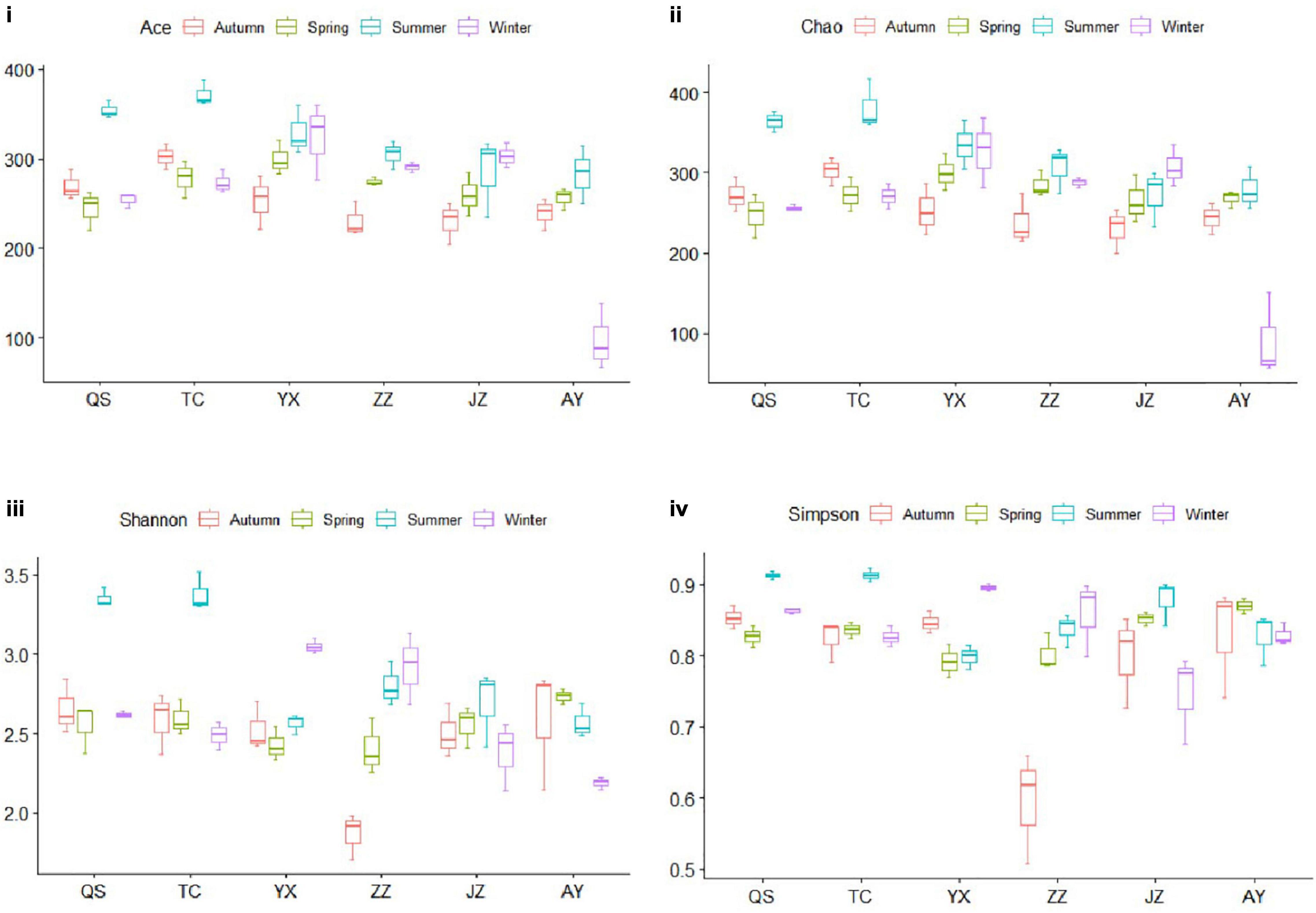
Figure 3. Evaluation of alpha diversity. The Ace index (i) represented richness and evenness, the Chao index (ii) represented the number of OTUs in the samples, and the Shannon and Simpson indices represented community diversity (iii,iv, respectively). The boxes with a horizontal line in the figure panels represent the median value, and the upper and lower lines of each box indicate the maximum and minimum values, respectively.
Non-metric Multidimensional Scale Analysis of Planktonic Eukaryotes in the Canal in Henan Province
Adonis analysis showed that there were significant differences among eukaryotic communities in different seasons (R2 = 0.41, P < 0.001). The NMDS results of the planktonic eukaryote OTUs at the six sampling stations showed that there were seasonal changes in the planktonic eukaryote community of the canal in Henan Province (Figure 4). The planktonic eukaryote community gathered in spring, which differed from other seasons; in spring, the dispersion degree of eukaryotic communities was higher, and the community composition varied greatly among the samples.
At the spatial scale, the eukaryotic communities of AY and JZ were quite different from those of other stations. The eukaryotic communities in TC and QS had low similarity in autumn, but high similarity in other seasons. The eukaryotic communities of YX and AY had the highest similarity in spring and the lowest similarity in autumn. Overall, the community structure of planktonic eukaryotes greatly differed across both temporal and spatial scales.
Redundancy Analysis of the Planktonic Eukaryote Community and Environmental Factors
Variance inflation factors were used to analyze the collinearity of environmental factors. The environmental factors with a variance inflation factor of > 10 were removed, and the factors with relatively low collinearity were retained. Detrended correspondence analysis showed that the longest gradient length for the four axes was less than 3; therefore, the RDA linear model was selected. Simultaneously, we eliminated environmental factors that were not significantly related to species (P > 0.05). Our RDA results are shown in Figure 5. ORP, WT, Vel, NO3–-N, and pH had significant effects on the eukaryotic community of the canal. The total explanatory variance of the first two RDA axes was 75.98%. ORP, Cond, and DO were the main drivers of spring and summer communities, and ORP had a higher impact on the eukaryotic community in spring and summer than in other seasons. The community in autumn and winter significantly differed from other seasons, and the community in autumn was influenced by multiple environmental factors, such as Vel. The winter community was significantly affected by DO, TP, and WT. At the spatial scale, TC and QS were significantly affected by ORP in spring and summer and DO in winter. The eukaryotic communities at other sampling stations were also affected by different environmental factors in different seasons. Overall, the driving factors of the planktonic eukaryote community in the canal had significant temporal and spatial differences.
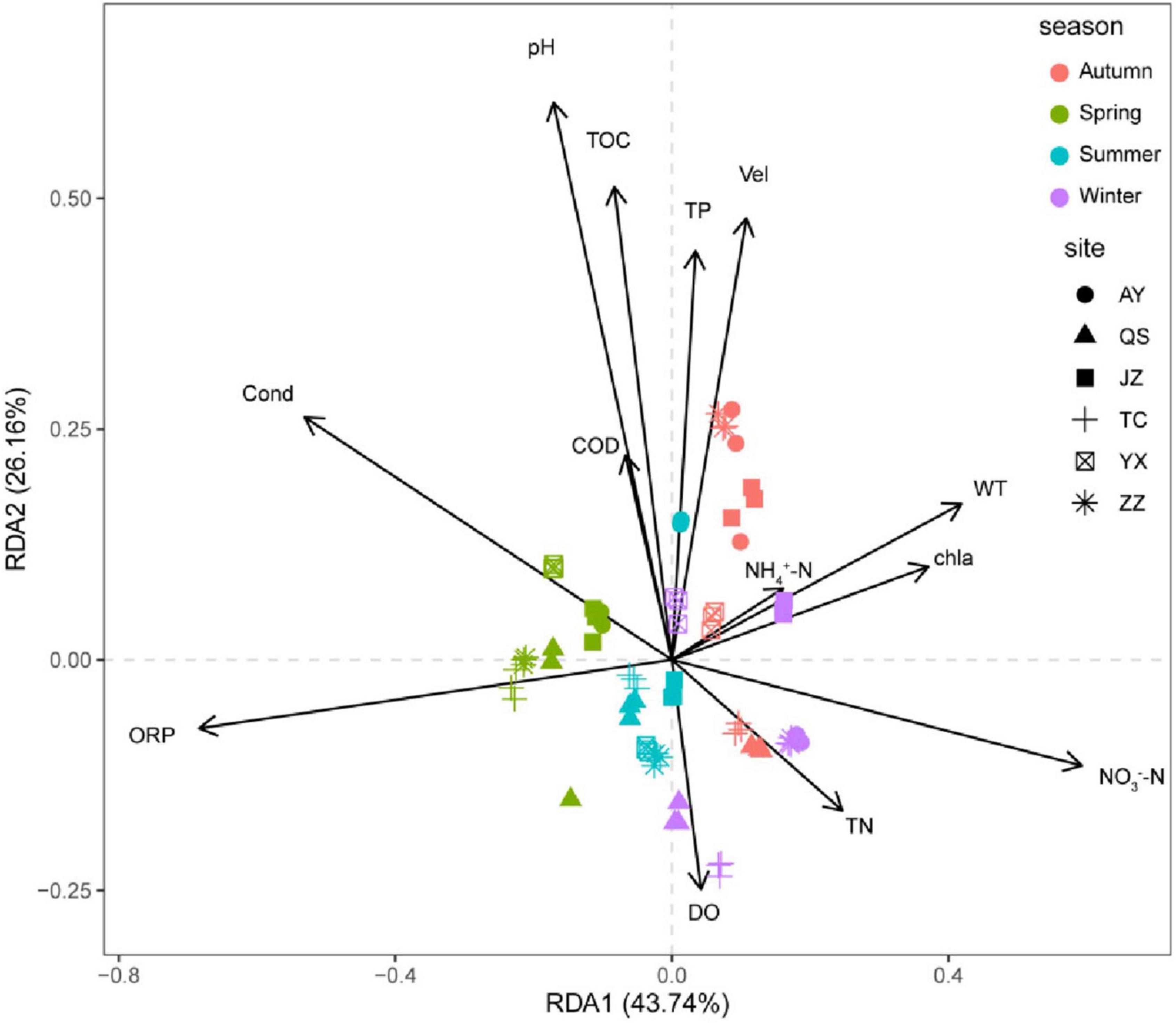
Figure 5. Redundancy analysis (RDA) ranking of planktonic eukaryote community and physicochemical factors of the canal in Henan Province.
Mantel Analysis of the Planktonic Eukaryote Community and Environmental Factors
The Mantel test was used to determine the relationship between environmental factors and species. At the phylum level, ORP, pH, Cond, TP, PCNM2, and distance were the main correlation parameters of eukaryotic community diversity and abundance (Figure 6). The pH, Cond, ORP, TP, PCNM2, and distance had significant effects on community abundance (P < 0.05). The pH, Cond, ORP, PCNM2, and distance had significant effects on Shannon diversity (P < 0.05), and TP had significant effects on Simpson diversity (P < 0.05).
Geographical Distribution Patterns of Planktonic Eukaryotes
To further explore the effects of geographical distance and environmental heterogeneity on the eukaryotic community structure of the canal, the linear relationship between the community similarity of plankton and geographical distance and the environmental difference was established and analyzed using distance decay (Figure 7). The results showed that eukaryotic community similarity was significantly negatively correlated with both geographic distances (Figure 7i, P < 0.05) and environmental heterogeneity (Figure 7ii, P < 0.05), which indicated that the geographical distribution of the planktonic eukaryote community followed the distance decay model, where community similarity gradually decreased with increased geographic distance and environmental heterogeneity. The results of this study also indicated that the distribution of the eukaryotic community was influenced by both dispersal limitation and environmental selection (Figure 7).
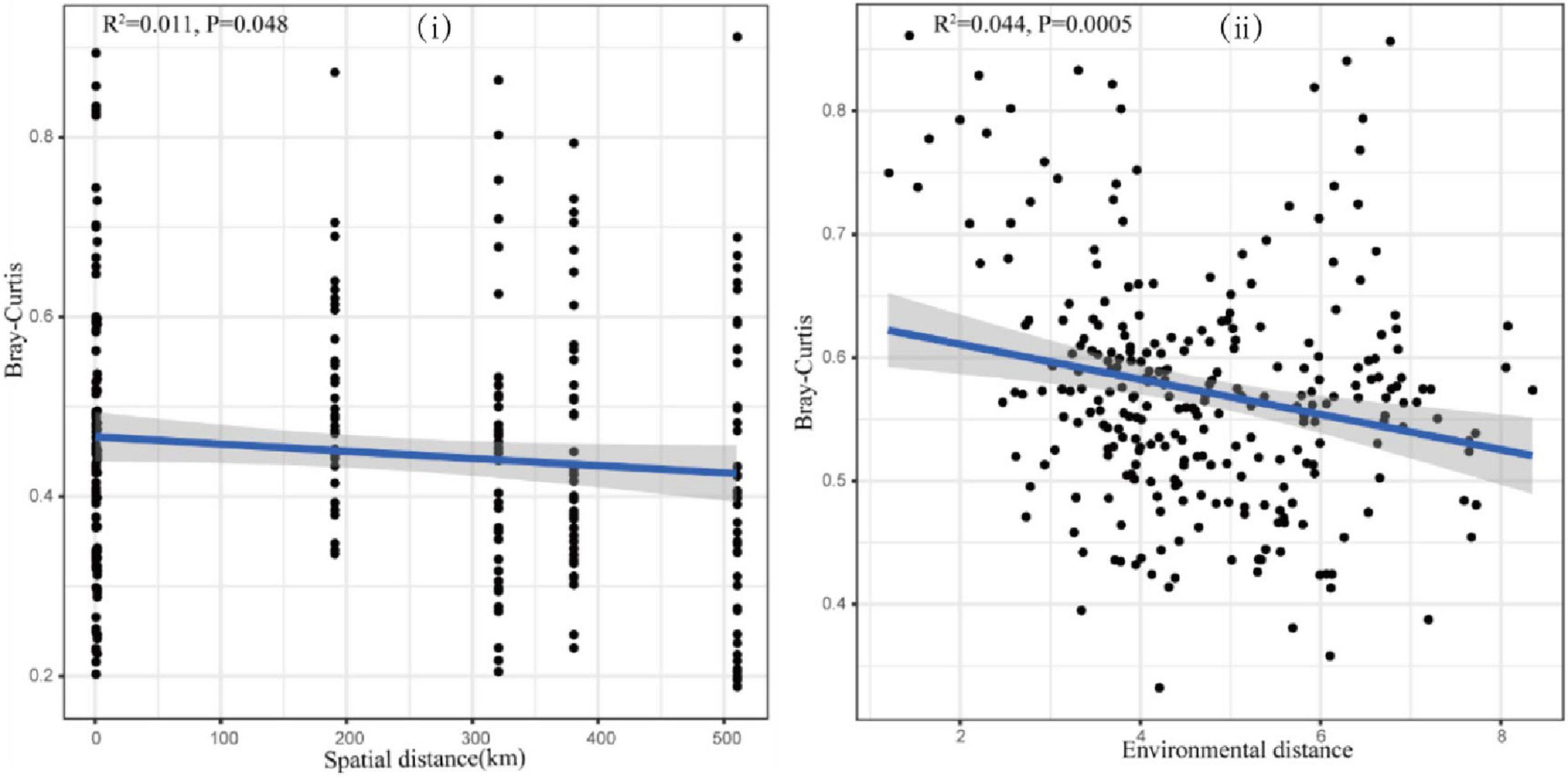
Figure 7. Distance–decay relationship between (i) eukaryotes and geographical distance and (ii) environmental difference.
To clarify the relative effects of season, geographical distance, and environmental heterogeneity on planktonic eukaryote community diversity, VPA was used to calculate the explanation rate of eukaryotic community diversity (Figure 8). The results showed that environmental heterogeneity, geographical distance, and seasonality accounted for 46, 8, and 13%, respectively, of planktonic eukaryote community diversity, and 21% of diversity was unexplained. These results indicated that environmental screening contributed more to eukaryotic community diversity than dispersal limitation. Environmental factors and spatial factors accounted for 1% of the eukaryotic community diversity, which was less than environmental factors and season (6%). This finding indicated that the interaction between environmental factors and seasons was greater than that between environmental factors and spatial factors in the process of community construction.
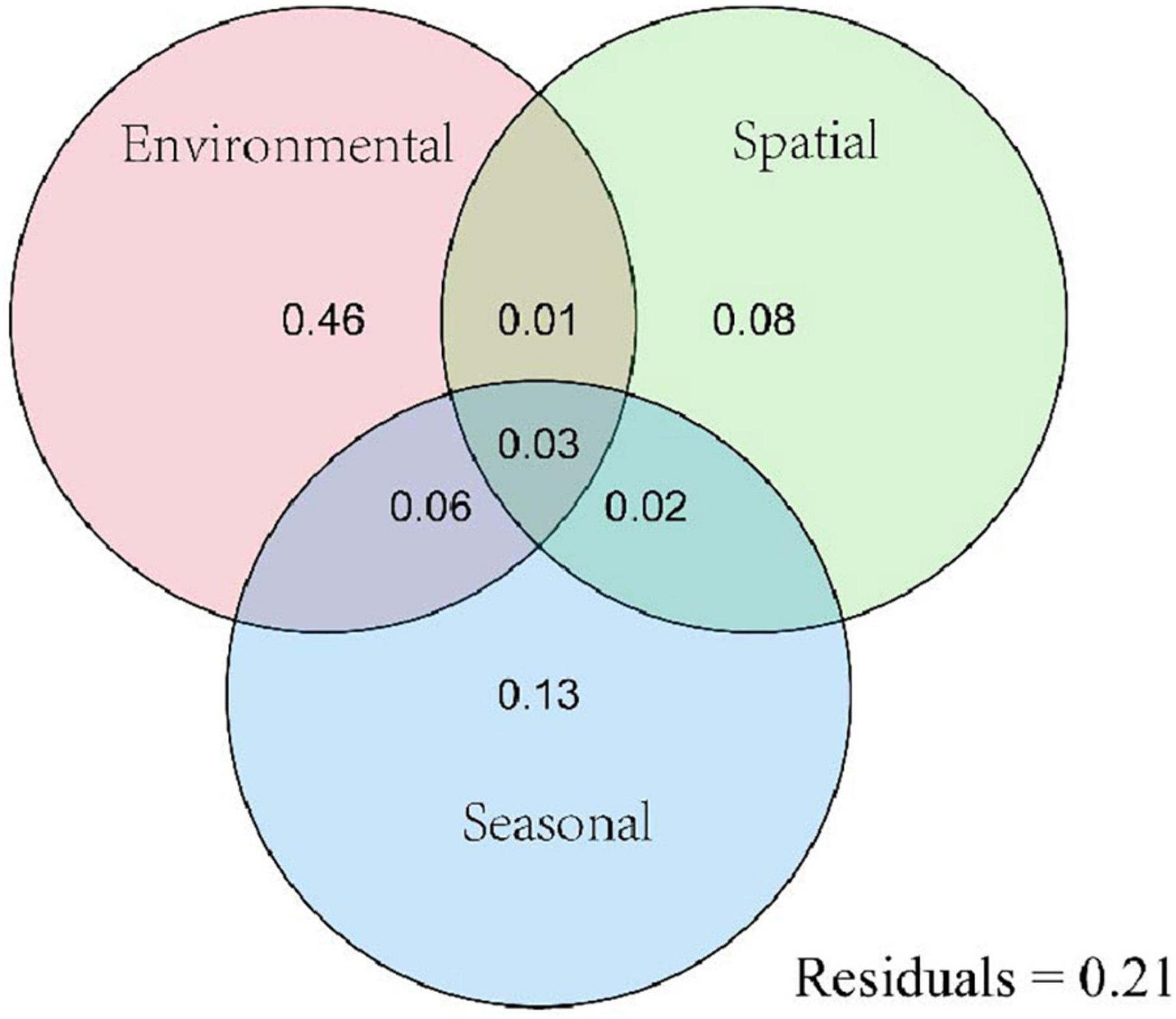
Figure 8. Variance partitioning analysis (VPA) showing the effects of environmental factors, seasons, and geographical distance on eukaryotic communities.
Discussion
In this study, we described the spatio-temporal dynamics of planktonic eukaryotes in the MRP of the SNWD in Henan Province by performing high-throughput sequencing of the SSU rRNA.
As it contains both highly conserved regions needed for primer annealing and variable regions to allow detailed taxonomic classification, and therefore, is routinely used for molecular identification of eukaryotic microorganism species (Goodwin et al., 2016), 18S rDNA gene was chosen as a target here. In addition, the DNA can be easily amplified because of its high copy number (Guillou et al., 2013). High-throughput sequencing can provide more information related to the classification of planktonic eukaryotes than traditional identification methods, such as isolation culture identification and PCR-denaturation gradient gel electrophoresis (Kagami et al., 2012). Therefore, using high-throughput sequencing technology, community structure information of phytoplankton, zooplankton, and other eukaryotes can be obtained quickly and accurately, and the connection between different eukaryotic species can be rapidly integrated. Compared with the study of specific eukaryotes alone (such as phytoplankton and zooplankton), it is more beneficial to understand the integrity of the community structure of planktonic eukaryotes.
Community Composition of Planktonic Eukaryotes
There were 53 phyla and 277 species of planktonic eukaryotes in the Henan Province canal, which comprehensively represented the community composition structure and diversity; this included Cryptophyta, Ciliophora, and norank_k_Cryptophyta as the dominant phyla. The eukaryotic plankton communities displayed a wide variety of growth characteristics that accounted for their adaptability to the canal environment. Medium-trophic water bodies are reportedly dominated by Cryptophyta, Bacillariophyta, and Pyrroptata, whereas eutrophic water bodies displayed abundant Chlorophyta and Cyanophyta (Tao et al., 2016). After establishing the community structure of plankton in the canal, we next determined the water quality and found it to be moderate. A previous study reported that the trophic degree increased with water transport distance (Chen et al., 2017). Our results concur with this study as we found that with increasing water transport distance along the canal, the relative abundances of Ciliophora, Choanoflagellida, and norank_k_Cryptophyta also increased. However, the relative abundances of Cryptophyta and unclassified_Eukaryota gradually decreased with increased water transport distance. Ciliophora is considered a predator of bacteria but can have a symbiotic relationship with algae (Stoecker et al., 2017; Grujcic et al., 2018; Stoecker and Lavrentyev, 2018). In addition, a previous study reported that the presence of free-living eukaryotes, such as ciliates and microalgae, changed with nutrient status (Lefranc et al., 2005). In agreement, we noticed that as water transport distance increased along the canal, the trophic degree also increased.
Additionally, the eukaryotes present in the canal were closely associated with the nutrient status of the canal. Temporally, eukaryotic communities had higher similarity in spring, whereas it was the lowest in autumn (Figure 4). We also observed that the canal WT was lowest in spring. Thus, we hypothesized that low temperatures likely remove some species that thrive at high temperatures, making communities more similar. This was consistent with the lower Shannon and Simpson indices of the eukaryotic community in spring. Cryptophyta was reported to have a higher population density at lower WT (Moreau et al., 2007). A previous study on the phytoplankton niche in Chaohu Lake reported that Cryptophyta displayed a wide range of ecological adaptations and could make full use of the habitat resources (Wang et al., 2010). Our results of lower WT in winter and spring, which made Cryptophyta the dominant species of the canal, are consistent with the results of these past studies. Noticeably, the diversity of samples was the lowest in autumn and the highest in summer (Figure 4). Furthermore, our results showed that Cryptophyta significantly and negatively correlated with water velocity (P < 0.001, Figure 6), and the relative abundance of Cryptophyta was the lowest at higher flow rates in autumn. Moreover, there was a significant negative correlation between Kathablepharidae and WT (P < 0.001, Figure 6), and the relative abundance of Kathablepharidae was the highest when WT was the lowest in spring. Thus, both WT and Vel exerted important effects on the planktonic eukaryote species in a season-dependent manner.
Effects of Environmental Factors on the Planktonic Eukaryote Community
Previous research indicates that WT is a vital environmental factor affecting the growth and development, community structure, abundance, and horizontal and vertical distribution of microorganisms in aquatic ecosystems (Shi et al., 2020). Furthermore, increased WT accelerates the growth and proliferation of the plankton population, and plankton species exhibit differences in adaptability to WT. It is reported that WT drives the change in dominant species by regulating cell activity and, thus, controls the plankton community structure (Stoecker et al., 2017). Stoecker et al. (2017) noticed that the evenness and diversity of samples in autumn were lower than those in other seasons, but WT was the highest in autumn compared to other seasons. A study evaluating the dynamics and functional roles of algal and bacterial communities in winter in two seasonal snow-covered lakes in Central Europe revealed that the algal biomass of the two lakes in winter was lower than the long-term summer average (Bullerjahn et al., 2020). This observation was in contrast with our results.
Phytoplankton biomass and spatial distribution largely depend on flow conditions. For example, a study of Zhongxin Lake in Shanghai Chongming Island reported that turbulence was negatively correlated with phytoplankton biomass, and flow velocity also showed a strong inverse correlation with Chl a concentration (Li et al., 2013). An inhibitory effect of turbulence on phytoplankton growth was also reported by another study that used ecological models and water quality data (Zhao et al., 2020). This study also found that the effect of nutrients on phytoplankton growth was the opposite of that of turbulence. Strong turbulence increases shear stress and may lead to mechanical damage or lysis of cells, which results in cell rupture or death (Zhou et al., 2016). In addition, turbulent systems enhance sediment re-suspension, leading to attenuated light penetration and decreased nutrient absorption, which may negatively affect phytoplankton survival (Li et al., 2019). In our study, the amount of water in the canal was not significantly affected by summer rainfall as the water distribution was controlled by upstream reservoirs, and, thus, the inhibition of the eukaryotic community by turbulence was weakened. Consequently, WT became the main factor affecting microbial growth in the water. Therefore, it is likely that the higher flow and velocity of the canal in autumn resulted in decreased community diversity and evenness, and turbulence had a greater effect on the eukaryotic community than WT.
Nutrient status and nutrient concentrations differently affect microbial species (Marc et al., 2014). Nitrogen and phosphorus are usually the limiting factors during the growth stage of microorganisms (Carlsson et al., 2012). It is reported that nutrient sufficiency affects the growth rate and productivity of plankton and bacterial community composition (Jong-Geol et al., 2019). Furthermore, a study revealed that the rapid absorption and utilization of nitrogen was the main cause of algal proliferation (Wu et al., 2009). Moreover, in Germany’s Kielstau Catchment, the contribution of nutrient regimes was higher than that of flow regimes to both trait- and taxonomy-based temporal beta diversity and its components (Wu et al., 2022). The results of this study showed that NH4+-N and NO3–-N significantly and negatively correlated with eukaryotic community diversity and abundance, which suggested that community growth consumed a large amount of nitrogen. The Cond and ORP values were determined by the type and concentration of dissolved ions and WT. The change of Cond likely the degree of eutrophication in the reservoir (Yan et al., 2021). Both Cond and ORP were significantly correlated with the eukaryotic community diversity and abundance (Figure 6), which indicated that the degree of eutrophication had a direct impact on the community. Additionally, pH, plankton growth, and reproduction displayed interdependence. In water bodies with low pH, plankton growth is limited and the number of individuals decreases, whereas high pH (alkaline) water bodies are more conducive to photosynthesis by plankton to form organic matter and increase plankton reproduction (Wang et al., 2016). Furthermore, the community composition of microbial eukaryotes also relates to the change in pH and DO concentration. The growth, reproduction, and other physiological processes of microorganisms, including respiration and death, consume a large amount of DO (Ouyang and Wei, 2013) leading to a significant negative correlation between eukaryotic community diversity and abundance and DO. Moreover, a positive correlation was noticed between pH and the eukaryotic community composition.
Previous research has confirmed the distance–decay relationship of bacterial communities with spatial similarity in lakes (Logares et al., 2013), reservoirs (Liu et al., 2015), and rivers (Isabwe et al., 2018). Earlier studies have shown that changes in ecological processes, such as biological diffusion and species sorting, are correlated with water dispersal time rather than river network density (Read et al., 2015), and these changes play an important role in bacterial community composition. Although dispersal limitation and environmental screening are considered important drivers that control microbial geographic distribution (Hanson et al., 2012; Zhang et al., 2018), it is not yet clear whether this effect is only due to dispersal limitation and/or environmental screening (Huszar et al., 2015). Furthermore, a study on the Guadiana River in Spain reported that environmental screening had a greater impact on the benthic diatom community than dispersal limitation (Urrea and Sabater, 2009). Moreover, a study on the Lancang River of China revealed that benthic diatoms were primarily affected by dispersal limitation (Sun et al., 2020). This study showed that the geographical distribution of eukaryotic communities in the canal followed a distance–decay pattern, that is, community similarity decreased with an increase in both geographical distance and environmental heterogeneity. The VPA results indicated that environmental screening had a greater influence on the geographical distribution of eukaryotic communities than dispersal limitation. Under the dynamic water transfer mode, the flow was stable, and the planktonic eukaryotes overcame most of the diffusion barriers, and the migration rate was higher. Therefore, the effect of dispersal limitation on the geographical distribution of eukaryotes in this canal was less than that of environmental screening.
Conclusion
This study investigated the community composition of the planktonic eukaryotes in the MRP of the SNWD using MiSeq sequencing technology and identified factors influencing the community composition. Our results showed that the eukaryotic communities were diverse, as there were 277 species in 53 phyla, including Cryptophyta, Ciliophora, norank_k_Cryptophyta, and Choanoflagellida. The community structure of eukaryotes and the relative abundance of dominant phyla significantly varied among different channel sections. Although the eukaryotic community was affected by environmental and spatial variables, seasonal variation was the most significant factor. The influence of environmental screening on the geographical distribution of eukaryotic communities was greater than the diffusion limitation of the MRP canal. The analysis of eukaryotic diversity indices and water quality showed that the eukaryotic community in the MRP of the SNWD was sensitive to physicochemical parameters. In addition, a large proportion of planktonic eukaryotes of the canal could not be annotated, as the public DNA database of eukaryotes are yet to register this information. Therefore, our study advocates the construction of an aquatic DNA database that will enrich species information and improve the proportion of species annotated in subsequent studies.
Data Availability Statement
The datasets presented in this study can be found in online repositories. The names of the repository/repositories and accession number(s) can be found below: MG-RAST (accession: mgp102285). Further inquiries about the datasets of this study should be directed to the corresponding author.
Author Contributions
YL and YZ conceived the study. FK, YZ, JW, YL, and YP analyzed the data. FK and YL wrote the manuscript. YP helped to revise the manuscript. All authors collected the data, provided the important inputs on the manuscript, contributed to the article, and approved the submitted version.
Funding
This study was funded by the National Natural Science Foundation of China (No. 51879130) and the higher discipline innovation and talent introduction base of Henan Province (No. CXJD2019001).
Conflict of Interest
The authors declare that the research was conducted in the absence of any commercial or financial relationships that could be construed as a potential conflict of interest.
Publisher’s Note
All claims expressed in this article are solely those of the authors and do not necessarily represent those of their affiliated organizations, or those of the publisher, the editors and the reviewers. Any product that may be evaluated in this article, or claim that may be made by its manufacturer, is not guaranteed or endorsed by the publisher.
Supplementary Material
The Supplementary Material for this article can be found online at: https://www.frontiersin.org/articles/10.3389/fevo.2022.899993/full#supplementary-material
References
Bullerjahn, G. S., McKay, R. M. L., Bernát, G., Prášil, O., Vörös, L., Pálffy, K., et al. (2020). Community dynamics and function of algae and bacteria during winter in central European great lakes. J. Great Lakes Res. 46, 732–40. doi: 10.1016/j.jglr.2019.07.002
Bunse, C., and Pinhassi, J. (2017). Marine bacterioplankton seasonal succession dynamics. Trends Microbiol. 25, 494–505. doi: 10.1016/j.tim.2016.12.013
Carlsson, P., Granéli, E., Granéli, W., Rodriguez, E. G., de Carvalho, W. F., Brutemark, A., et al. (2012). Bacterial and phytoplankton nutrient limitation in tropical marine waters, and a coastal lake in Brazil. J. Exp. Mar. Biol. Ecol. 418-419, 37–45. doi: 10.1016/j.jembe.2012.03.012
Chen, Z., Chen, H., Li, Y., Huang, J., Lu, K., Zhao, H., et al. (2017). Community structure and influencing factors of bacterioplankton in the main cancel of the mid-line project of South-to-North water division in sections of Henan Province. J. Chin. Environ. Sci. 37, 1505–13. doi: 10.13227/j.hjkx.201707164
Chen, Z., Yuan, J., Sun, F., Zhang, F., Chen, Y., Ding, C., et al. (2018). Planktonic fungal community structures and their relationship to water quality in the Danjiangkou Reservoir, China. Sci. Rep. 8:10596. doi: 10.1038/s41598-018-28903-y
Goodwin, S., McPherson, J. D., and McCombie, W. R. (2016). Coming of age: ten years of next-generation sequencing technologies. Nat. Rev. Genet. 17, 333–51. doi: 10.1038/nrg.2016.49
Grujcic, V., Nuy, J. K., Salcher, M. M., Shabarova, T., Kasalicky, V., Boenigk, J., et al. (2018). Cryptophyta as major bacterivores in freshwater summer plankton. ISME J. 12, 1668–81. doi: 10.1038/s41396-018-0057-5
Guillou, L., Bachar, D., Audic, S., Bass, D., Berney, C., Bittner, L., et al. (2013). The protist ribosomal reference database (PR2): a catalog of unicellular eukaryote small sub-unit rRNA sequences with curated taxonomy. Nucleic Acids Res. 41, D597–604. doi: 10.1093/nar/gks1160
Han, X. M., Gong, Z. L., Yang, X. M., Li, Y. Y., Chen, Z. J., Zhu, H. H., et al. (2021). Diversity and function prediction of bacterioplankton under human disturbance in the main stream of the Laoguan River before and after the flood season. Huan Jing Ke Xue 42, 831–41. doi: 10.13227/j.hjkx.202007082
Hanson, C. A., Fuhrman, J. A., Horner-Devine, M. C., and Martiny, J. B. H. (2012). Beyond biogeographic patterns: processes shaping the microbial landscape. Nat. Rev. Microbiol. 10, 497–506. doi: 10.1038/nrmicro2795
Huszar, V. L. M., Nabout, J. C., Appel, M. O., Santos, J. B. O., Abe, D. S., and Silva, L. H. S. (2015). Environmental and not spatial processes (directional and non-directional) shape the phytoplankton composition and functional groups in a large subtropical river basin. J. Plankton Res. 2015, 1–11. doi: 10.1093/plankt/fbv084
Isabwe, A., Yang, J. R., Wang, Y., Liu, L., Chen, H., and Yang, J. (2018). Community assembly processes underlying phytoplankton and bacterioplankton across a hydrologic change in a human-impacted river. Sci. Total Environ. 630, 658–67. doi: 10.1016/j.scitotenv.2018.02.210
Jong-Geol, K., Joo-Han, G., Man-Young, J., Sung-Uk, A., Jung-Ho, H., Sanghoon, K., et al. (2019). Distinct temporal dynamics of planktonic archaeal and bacterial assemblages in the bays of the Yellow Sea. PLoS One 14:e0221408. doi: 10.1371/journal.pone.0221408
Kagami, M., Amano, Y., and Ishii, N. (2012). Community structure of planktonic fungi and the impact of parasitic chytrids on phytoplankton in Lake Inba, Japan. Microb. Ecol. 63, 358–68. doi: 10.1007/s00248-011-9913-9
Keck, F., Vasselon, V., Tapolczai, K., Rimet, F., and Bouchez, A. (2017). Freshwater biomonitoring in the information age. Front. Ecol. Environ. 15:266–74. doi: 10.1002/fee.1490
Lefranc, M., Thenot, A., Lepere, C., and Debroas, D. (2005). Genetic diversity of small eukaryotes in lakes differing by their trophic status. Appl. Environ. Microbiol. 71, 5935–42. doi: 10.1128/AEM.71.10.5935-5942.2005
Li, F., Zhang, H., Zhu, Y., Xiao, Y., and Chen, L. (2013). Effect of flow velocity on phytoplankton biomass and composition in a freshwater lake. Sci. Total Environ. 447, 64–71. doi: 10.1016/j.scitotenv.2012.12.066
Li, K., He, Y., Dai, L., He, Q., Ai, H., Yang, G., et al. (2019). Interactions between suspended particulate matter and algal cells contributed to the reconstruction of phytoplankton communities in turbulent waters. Water Res. 149, 251–62. doi: 10.1016/j.watres.2018.11.003
Liang, J., Xin, X., Lu, L., Hu, S., Zhu, D., and Tang, J. (2017). Analysis of water quality variation and potential pollution sources in main canal of Middle Route Project of South-to-North Water Diversion. People Yangtze River 48, 6–9. doi: 10.16232/j.cnki.1001-4179.2017.15.002
Liu, L., Yang, J., Yu, Z., and David, M. W. (2015). The biogeography of abundant and rare bacterioplankton in the lakes and reservoirs of China. ISME J. 9, 2068–77. doi: 10.1038/ismej.2015.29
Logares, R., Lindström, E. S., Langenheder, S., Logue, J. B., Paterson, H., Laybourn-Parry, J., et al. (2013). Biogeography of bacterial communities exposed to progressive long-term environmental change. ISME J. 7, 937–48. doi: 10.1038/ismej.2012.168
Marc, L., Özgül, I., Tamara, G., Adriana, A., Bruno, L., Lise-Marie, P., et al. (2014). Bacterial community composition in three freshwater reservoirs of different alkalinity and trophic status. PLoS One 9:e116145. doi: 10.1371/journal.pone.0116145
Moreau, M. F., Surico-Bennett, J., Vicario-Fisher, M., Crane, D., Gersberg, R. M., and Hurlbert, S. H. (2007). Contaminants in tilapia (Oreochromis mossambicus) from the Salton Sea, California, in relation to human health, piscivorous birds and fish meal production. Hydrobiologia 576, 127–65. doi: 10.1007/s10750-006-0299-5
Needham, D. M., Fichot, E. B., Wang, E., Berdjeb, L., Cram, J. A., Fichot, C. D. G., et al. (2018). Dynamics and interactions of highly resolved marine plankton via automated high-frequency sampling. ISME J. 12, 2417–32. doi: 10.1038/s41396-018-0169-y
Nong, X., Shao, D., Zhong, H., and Liang, J. (2020). Evaluation of water quality in the South-to-North Water Diversion Project of China using the water quality index (WQI) method. Water Res. 178:115781. doi: 10.1016/j.watres.2020.115781
Ouyang, X. R., and Wei, Y. (2013). A preliminary exploration of dissolved oxygen based on FVCOM in Meiliang Bay, Lake Taihu and its influence mechanism. J. Lake Sci. 25, 478–88. doi: 10.18307/2013.0404
Philippe, E., Franck, L., and Jan, P. (2015). Accurate multiplexing and filtering for high-throughput amplicon-sequencing. Nucleic Acids Res. 5, 2513–24. doi: 10.1093/nar/gkv107
Read, D. S., Gweon, H. S., Bowes, M. J., Newbold, L. K., Field, D., Bailey, M. J., et al. (2015). Catchment-scale biogeography of riverine bacterioplankton. ISME J. 9, 516–26. doi: 10.1038/ismej.2014.166
Shi, X., Li, S., Zhang, M., Liu, C., Wu, Q., Li, S., et al. (2020). Temperature mainly determines the temporal succession of the photosynthetic picoeukaryote community in Lake Chaohu, a highly eutrophic shallow lake. Sci. Total Environ. 702:134803. doi: 10.1016/j.scitotenv.2019.134803
Stein, E. D., Martinez, M. C., Stiles, S., Miller, P. E., and Zakharov, E. V. (2014). Is DNA barcoding actually cheaper and faster than traditional morphological methods: results from a survey of freshwater bioassessment efforts in the United States? PLoS One 9:e95525. doi: 10.1371/journal.pone.0095525
Stoecker, D. K., and Lavrentyev, P. J. (2018). Mixotrophic plankton in the Polar Seas: a pan-arctic review. Front. Mar. Sci. 5:292. doi: 10.3389/fmars.2018.00292
Stoecker, D. K., Hansen, P. J., Caron, D. A., and Mitra, A. (2017). Mixotrophy in the marine plankton. Annu. Rev. Mar. Sci. 9, 311–35. doi: 10.1146/annurev-marine-010816-060617
Su, X., Steinman, A. D., Xue, Q., Zhao, Y., Tang, X., and Xie, L. (2017). Temporal patterns of phyto- and bacterioplankton and their relationships with environmental factors in Lake Taihu, China. Chemosphere 184, 299–308. doi: 10.1016/j.chemosphere.2017.06.003
Sun, S. H., Chen, J., Wang, P. F., Wang, C., and Yuan, Q. S. (2020). Biogeographic distribution patterns of diatoms in Lancang River and their key drivers. Environ. Sci. 41, 5458–69. doi: 10.13227/j.hjkx.202005258
Tao, M., Xie, B. W., Ze-Min, Q. I., Yang, P. H., and Bin, L. I. (2016). Phytoplankton community structure and water quality assessment in Tuojiang River. Oceanol. Limnol. Sin. 47, 854–61. doi: 10.11693/hyhz20160500108
Urrea, G., and Sabater, S. (2009). Epilithic diatom assemblages and their relationship to environmental characteristics in an agricultural watershed (Guadiana River, SW Spain). Ecol. Indicat. 9, 693–703. doi: 10.1016/j.ecolind.2008.09.002
Vasselon, V., Rimet, F., Tapolczai, K., and Bouchez, A. (2017). Assessing ecological status with diatoms DNA metabarcoding: scaling-up on a WFD monitoring network (Mayotte island, France). Ecol. Indicat. 82, 1–12. doi: 10.1016/j.ecolind.2017.06.024
Visco, J. A., Apothéloz-Perret-Gentil, L., Cordonier, A., Esling, P., Pillet, L., and Pawlowski, J. (2015). Environmental monitoring: inferring the diatom index from next-generation sequencing data. Environ. Sci. Technol. 49, 7597–605. doi: 10.1021/es506158m
Wang, Y., Chen, L., Niu, Y., and Luo, M. (2016). Spatio-temporal variation in phytoplankton community and its influencing factors in Dan Jiangkou Reservoir. J. Lake Sci. 28, 1057–65. doi: 10.18307/2016.0516
Wang, Z., Wu, W., Zuo, M., and Li, D. (2010). Niche analysis of phytoplankton community in Lake Chaohu. Resour. Environ. Yangtze River Basin 19, 685–91. doi: 10.3724/SP.J.1088.2010.00432
Wu, L., Yu, Y., Zhang, T., Feng, W., Zhang, X., and Li, W. (2009). PCR-DGGE fingerprinting analysis of plankton communities and its relationship to lake trophic status. Int. Rev. Hydrobiol. 94, 528–41. doi: 10.1002/iroh.200911129
Wu, N., Wang, Y., Wang, Y., Sun, X., Faber, C., and Fohrer, N. (2022). Environment regimes play an important role in structuring trait- and taxonomy-based temporal beta diversity of riverine diatoms. J. Ecol. 2022, 1–13. doi: 10.1111/1365-2745.13859
Yan, G., Yin, X., Wang, X., Wang, L., Li, D., and Li, H. (2021). Relationship of chlorophyll a concentration and environmental factors in Dongting Lake based on GAM model. J. Chin. Environ. Sci. 42, 313–22. doi: 10.19674/j.cnki.issn1000-6923.20210709.004
Yan, M., Chen, S., Huang, T., Li, B., Li, N., Liu, K., et al. (2020). Community compositions of phytoplankton and eukaryotes during the mixing periods of a drinking water reservoir: dynamics and interactions. Int. J. Environ. Res. Public Health 17:1128. doi: 10.3390/ijerph17041128
Yang, S., Han, X., Zhang, C., Sun, B., Wang, X., and Shi, X. (2014). Seasonal changes in phytoplankton biomass and dominant species in the Changjiang River estuary and adjacent seas:general trends based on field durvey data 1959-2009. J. Ocean Univ. China 13, 926–34. doi: 10.1007/s11802-014-2515-7
Zhang, C., Zhu, Y., Song, G., Mi, W., Bi, Y., Wang, S., et al. (2021). Spatiotemporal pattern of phytoplankton community structure and its determining factors in the channel of the middle route of South-to-North Water Diversion Project. J. Lake Sci. 33, 675–86.
Zhang, J., Zhu, C., Guan, R., Xiong, Z., Zhang, W., Shi, J., et al. (2017). Microbial profiles of a drinking water resource based on different 16S rRNA V regions during a heavy cyanobacterial bloom in Lake Taihu, China. Environ. Sci. Pollut. Res. Int. 24, 12796–808. doi: 10.1007/s11356-017-8693-2
Zhang, W., Pan, Y., Yang, J., Chen, H., Holohan, B., Vaudrey, J., et al. (2018). The diversity and biogeography of abundant and rare intertidal marine microeukaryotes explained by environment and dispersal limitation. Environ. Microbiol. 20, 462–76. doi: 10.1111/1462-2920.13916
Zhao, G., Gao, X., Zhang, C., and Sang, G. (2020). The effects of turbulence on phytoplankton and implications for energy transfer with an integrated water quality-ecosystem model in a shallow lake. J. Environ. Manage. 256:109954. doi: 10.1016/j.jenvman.2019.109954
Keywords: eukaryotic plankton, high-throughput sequencing technology, the Middle Route Project of South-to-North Water Diversion, redundancy analysis, distance-attenuation analysis
Citation: Li Y, Khan FH, Wu J, Zhang Y, Jiang Y, Chen X, Yao Y, Pan Y and Han X (2022) Drivers of Spatiotemporal Eukaryote Plankton Distribution in a Trans-Basin Water Transfer Canal in China. Front. Ecol. Evol. 10:899993. doi: 10.3389/fevo.2022.899993
Received: 19 March 2022; Accepted: 17 June 2022;
Published: 15 July 2022.
Edited by:
Naicheng Wu, Ningbo University, ChinaCopyright © 2022 Li, Khan, Wu, Zhang, Jiang, Chen, Yao, Pan and Han. This is an open-access article distributed under the terms of the Creative Commons Attribution License (CC BY). The use, distribution or reproduction in other forums is permitted, provided the original author(s) and the copyright owner(s) are credited and that the original publication in this journal is cited, in accordance with accepted academic practice. No use, distribution or reproduction is permitted which does not comply with these terms.
*Correspondence: Yuying Li, lyying200508@163.com