- 1Department of Endocrinology, Hainan Affiliated Hospital of Hainan Medical University, Hainan General Hospital, Haikou, China
- 2Department of Endocrinology, Hainan General Hospital, Haikou, China
Background: Dyslipidemia is highly prevalent among individuals with prediabetes, further exacerbating their cardiovascular risk. However, the genetic determinants underlying diabetic dyslipidemia in Southern Han Chinese remain largely unexplored.
Methods: We performed a genome-wide association study (GWAS) of blood lipid traits in 451 Southern Han Chinese adults with prediabetes. Fasting plasma lipids, including triglycerides (TG), total cholesterol (TC), high-density lipoprotein cholesterol (HDL-C) and low-density lipoprotein cholesterol (LDL-C) were assayed. Genotyping was conducted using the Precision Medicine Diversity Array and Gene Titan platform, followed by genotype imputation using IMPUTE2 with the 1000 Genomes Project (Phase 3, Southern Han Chinese) as reference. Single nucleotide polymorphisms (SNPs) associated with lipid levels were identified using mixed linear regression, with adjustment for covariates.
Results: We identified 58, 215, 74 and 81 novel SNPs associated with TG, TC, HDL-C and LDL-C levels, respectively (P < 5×10-5). Several implicated loci were located in or near genes involved in lipid metabolism, including SRD5A2, PCSK7, PITPNC1, IRX3, BPI, and LBP. Pathway enrichment analysis highlighted lipid metabolism and insulin secretion.
Conclusion: This first GWAS of dyslipidemia in Southern Han Chinese with prediabetes identified novel genetic variants associated with lipid traits. Our findings provide new insights into genetic mechanisms underlying heightened cardiovascular risk in the prediabetic stage. Functional characterization of implicated loci is warranted.
Introduction
Diabetes mellitus is a prevalent chronic metabolic disease characterized by elevated levels of blood glucose (1). In 2021, there were 529 million people living with diabetes mellitus worldwide, which remains a substantial public health issue (2). The global burden of prediabetes is also substantial and growing (3). Prediabetes confers not only heightened risk for diabetes mellitus development, but also independent risk for cardiovascular disease (4, 5). Compared to healthy normoglycemic individuals, patients with prediabetes exhibit a greater propensity for atherogenic dyslipidemia, marked by elevated triglycerides (TG), total cholesterol (TC) and low-density lipoprotein cholesterol (LDL-C), alongside decreased high-density lipoprotein cholesterol (HDL-C) (6). This lipid profile significantly promotes atherosclerotic cardiovascular disease in the prediabetic population (7). Elucidating the pathogenic mechanisms underlying dyslipidemia in prediabetes is therefore imperative to inform strategies for complication prevention and management.
Lipid metabolism is a complex physiological process under polygenic regulation. While genome-wide association studies (GWASs) have uncovered several lipid-related loci in normoglycemic (8–11) and diabetes mellitus populations (12), focused genetic analyses in prediabetic cohorts remain scarce. Given the obvious dyslipidemia in prediabetes, studying the genetic characteristics of blood lipids in this population can reveal specific genetic variations. This can provide insights into disease susceptibility, aid risk stratification, and guide targeted interventions to mitigate cardiovascular risk.
In this study, we performed GWAS of lipid levels in 451 Han Chinese adults with prediabetes using the Precision Medicine Diversity Array. We aim to identify novel loci associated with TG, TC, HDL-C and LDL-C that may be specific to the prediabetic state. Significant variants will also undergo functional annotation. Our findings can facilitate personalized dyslipidemia management and cardiovascular risk assessment in this high-risk population.
Materials and methods
Study design and participants
To identify genetic loci associated with lipid traits, including TG, TC, HDL-C, and LDL-C, in the context of prediabetes among Southern Han Chinese, we conducted a GWAS (Figure 1). A total of 451 patients with prediabetes were recruited from the National Diabetes Prevalence Survey conducted by the Chinese Medical Association in 2017. Prediabetes was defined according to standard diagnostic criteria: 5.6mmol/L ≤ fasting plasma glucose (FPG) < 7.0mmol/L, or 7.8mmol/L ≤ 2-hours postprandial glucose (2hPG) < 11.1mmol/L, or 5.7% ≤ hemoglobin A1C (HbA1C) ≤ 6.4% (13, 14). All participants were at least 18 years old and had resided in the selected community for at least five years. Exclusion criteria were pregnancy, severe illness (e.g., cancer, kidney disease, acute infections), and cognitive impairment. Blood lipid levels were measured in a central laboratory using a Roche Modular autoanalyzer (Roche Diagnostics, Indianapolis, IN, USA).
This study was approved by the Ethical Committee of the Hainan Affiliated Hospital of Hainan Medical University (Med-Eth-Re (2019) 18) and adhered to ethical standards set forth by the committee and the Declaration of Helsinki. All participants provided written informed consent after being fully informed of the study’s purpose.
DNA extraction and genotyping
Fasting peripheral blood (5mL) was collected from each participant in Ethylene diamine tetraacetic acid (EDTA) tubes and stored at −20°C. Genomic DNA was extracted from whole blood using the GoldMag-Mini Whole Blood Genomic DNA Purification Kit (GoldMag Co. Ltd., Xi’an, China) per manufacturer’s protocol. DNA concentration and purity were evaluated using the Nanodrop 2000 spectrophotometer (Thermo Fisher Scientific, Waltham, MA, USA). Samples were genotyped on the Applied Biosystems Axiom Precision Medicine Diversity Array (Thermo Fisher Scientific) using the GeneTitan platform. Genotyping results were managed and analyzed with Axiom Analysis Suite software (version 6.0). Of 108,825 genotyped markers, copy number variants, sex chromosome and mitochondrial SNPs, deletion and duplicated loci, and genotype calling rate < 95% and SNPs with HHardy-Weinberg equilibrium < 5×10−6 were excluded.
Genotype imputation and quality control
Additional SNPs were imputed using IMPUTE2 software and the 1000 Genomes Project Phase III (Southern Han Chinese) reference panel, increasing genomic coverage to 9,378,219 SNPs. Post-imputation quality control removed non-biallelic variants, SNPs with imputation quality score ≤ 0.4, call rate < 90%, and HWE p-value < 5×10−6. The final imputed dataset contained 1,752,717 SNPs.
Statistical analysis
Extreme outlier values for lipid traits were removed based on 3σ principle. Lipid indicators were then standardized using the RNOmni package in R software. Mixed linear regression analysis was performed to identify genetic variants associated with lipid traits (TG, TC, HDL-C, LDL-C) under an additive model with adjustment for age, gender, and smoking using Gold Helix SNP & Variation Suite software (version 8.7). Genome-wide significance was defined as P < 5 × 10−5. Pearson correlation analysis was conducted in SPSS 20.0 (IBM Corp, Armonk, NY) to assess associations between lipid indicators, with p < 0.05 considered statistically significant.
Bioinformatics analysis
To explore the biological activities and functions of candidate lipid-related genes, the STRING database (https://cn.string-db.org/) was utilized to analyze protein-protein functional interactions. KEGG and Gene Ontology (GO) enrichment analyses were performed using SangerBox 3.0 (http://sangerbox.com/home.html) and g:Profiler databases (https://biit.cs.ut.ee/gprofiler/orth), respectively, to elucidate pathway and functional enrichments.
Results
The baseline characteristics of the study participants are presented in Table 1. The mean age of prediabetic participants was 51.78 ± 14.19 years. The cohort comprised 235 females (52.1%) and 216 males (47.9%). Most were non-smokers (n = 341, 75.6%), with 28 occasional smokers (6.2%) and 82 regular smokers (18.2%). Mean lipid levels were as follows: TG 1.999 ± 1.075 mmol/L; TC 5.525 ± 1.096 mmol/L; HDL-C 1.438 ± 0.305 mmol/L; and LDL-C 3.052 ± 0.862 mmol/L. Significant positive correlations were observed between TC, LDL-C, and TG (Supplementary Figure 1).
This genome-wide association study identified 58, 215, 74 and 81 novel SNPs surpassing genome-wide significance (P < 5×10-5) for association with TG, TC, HDL-C and LDL-C, respectively (Supplementary Tables 1–4; Figure 2). Lead SNPs were selected based on statistical significance and regional association patterns, resulting in 17 TG loci, 10 TC loci, 17 HDL-C loci, and 22 LDL-C loci (Table 2). Of these, 13 SNPs achieved P < 5×10-6, including rs12750886 near TAL1 (P = 4.33×10-6), rs7598882 in LINC01494 (P = 1.40 × 10-6), rs632148 (P = 4.59 × 10-6), rs534999 (P = 4.75 × 10-6), and rs558803 (P = 4.75 × 10-6) in SRD5A2 associated with TG and rs62277650 (P = 3.86 × 10-6), rs76927959 (P = 3.86 × 10-6), rs62277655 (P = 5.85 × 10-7), rs7667521 (P = 5.85 × 10-7), rs7667528 (P = 5.85 × 10-7), and rs7697698 (P = 5.85 × 10-7) in SORCS2, rs914653 in C9orf92/BNC2 (P = 1.69× 10-6), and rs56094199 in LOC400499 (P = 3.88× 10-6) associated with HDL-C. Full lead SNP results are presented in Table 2.
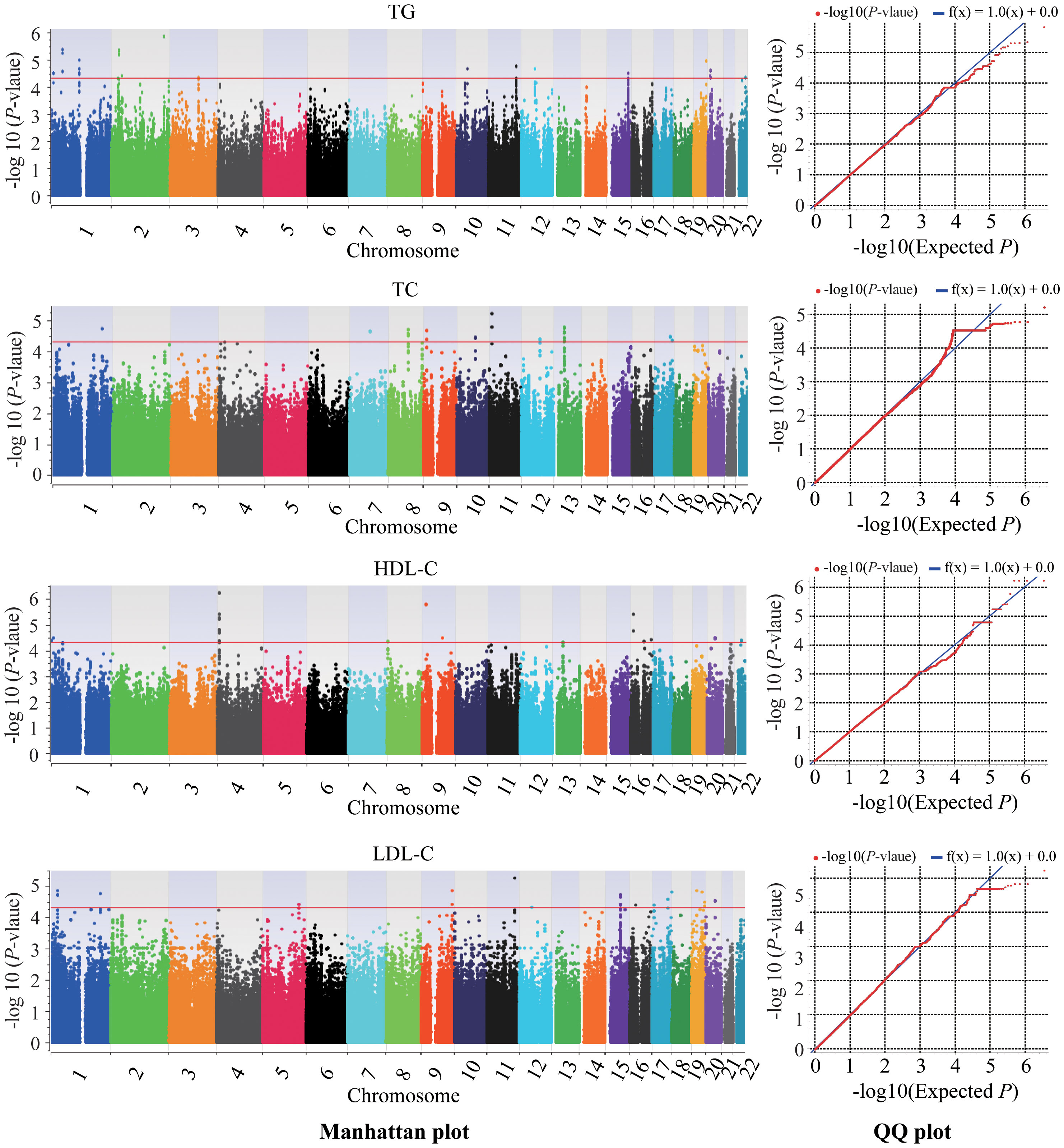
Figure 2 Manhattan plots and quantile-quantile plots of association of lipid traits in the GWAS. Chromosomes are shown on the x-axis, and the −log10 of the p-value on the y-axis. The red line represents the genome-wide significance cut off of 5 × 10−5. TC, total cholesterol; TG, triglyceride; HDL-C, high-density lipoprotein-cholesterol; LDL-C, low density lipoprotein cholesterol; QQ, quantile-quantile.
The lead SNPs tagged distinct association signals in or near genes with known roles in lipid metabolism and transport, such as SRD5A2, PCSK7, SNAP25, PITPNC1, IRX3, BPI, and LBP. Meanwhile, KEGG enrichment analysis revealed that the genes mapped by the lead SNPs were enriched in several key pathways, including ther elipid metabolism, insulin secretion, Wnt signaling pathway, cardiac muscle contraction, MAPK signaling pathway, fatty acid metabolism, nitrogen metabolism, and metabolic pathways (Figure 3). In addition, we conducted interaction analysis and Gene Ontology (GO) enrichment analysis on the genes (SRD5A2, MICAL2, SORCS2, and MATN1) significantly associated with lipid traits in our GWAS results. The interaction analysis using the STRING database identified several potential protein-protein interactions among the lipid-associated genes (Figure 4). The GO enrichment analysis results revealed significant enrichment of several GO terms, includin gregulation of hormone levels, exocytosis, nerve growth factor signaling pathway, and extracellular matrix organization (Figure 5; Supplementary Table 5). In the next stage, we will follow up these SNPs in larger cohorts and perform functional characterization to elucidate the mechanisms underlying the genetic associations with lipid levels.
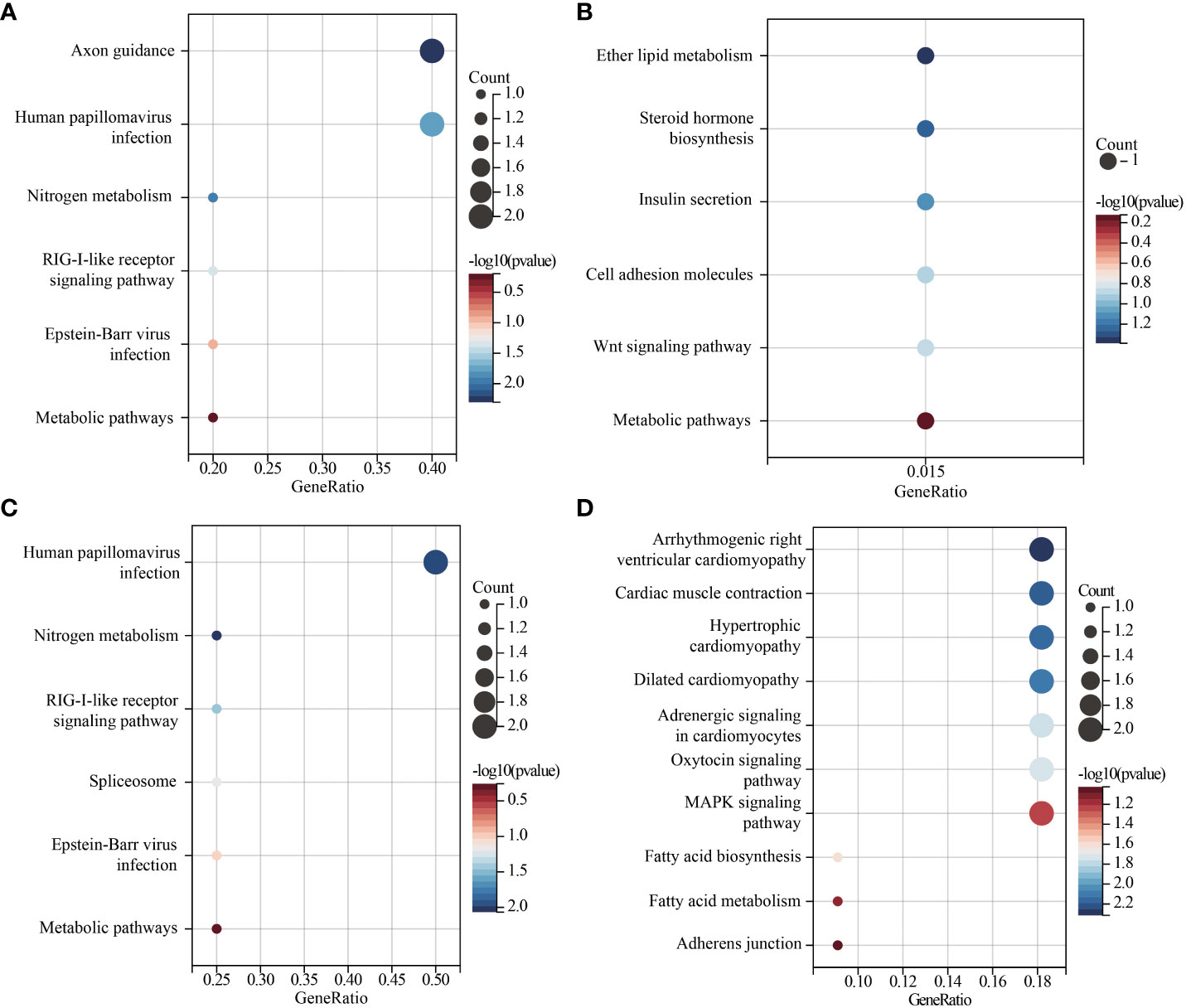
Figure 3 Scatter plot of KEGG enrichment of lead SNPs located or near genes. (A). lead SNPs located or near genes associated with TG levels. (B). lead SNPs located or near genes associated with TC levels. (C). lead SNPs located or near genes associated with HDL-C levels. (D). lead SNPs located or near genes associated with LDL-C levels. GeneRatio is the number of genes enriched in the KEGG entry divided by the total number of given genes.
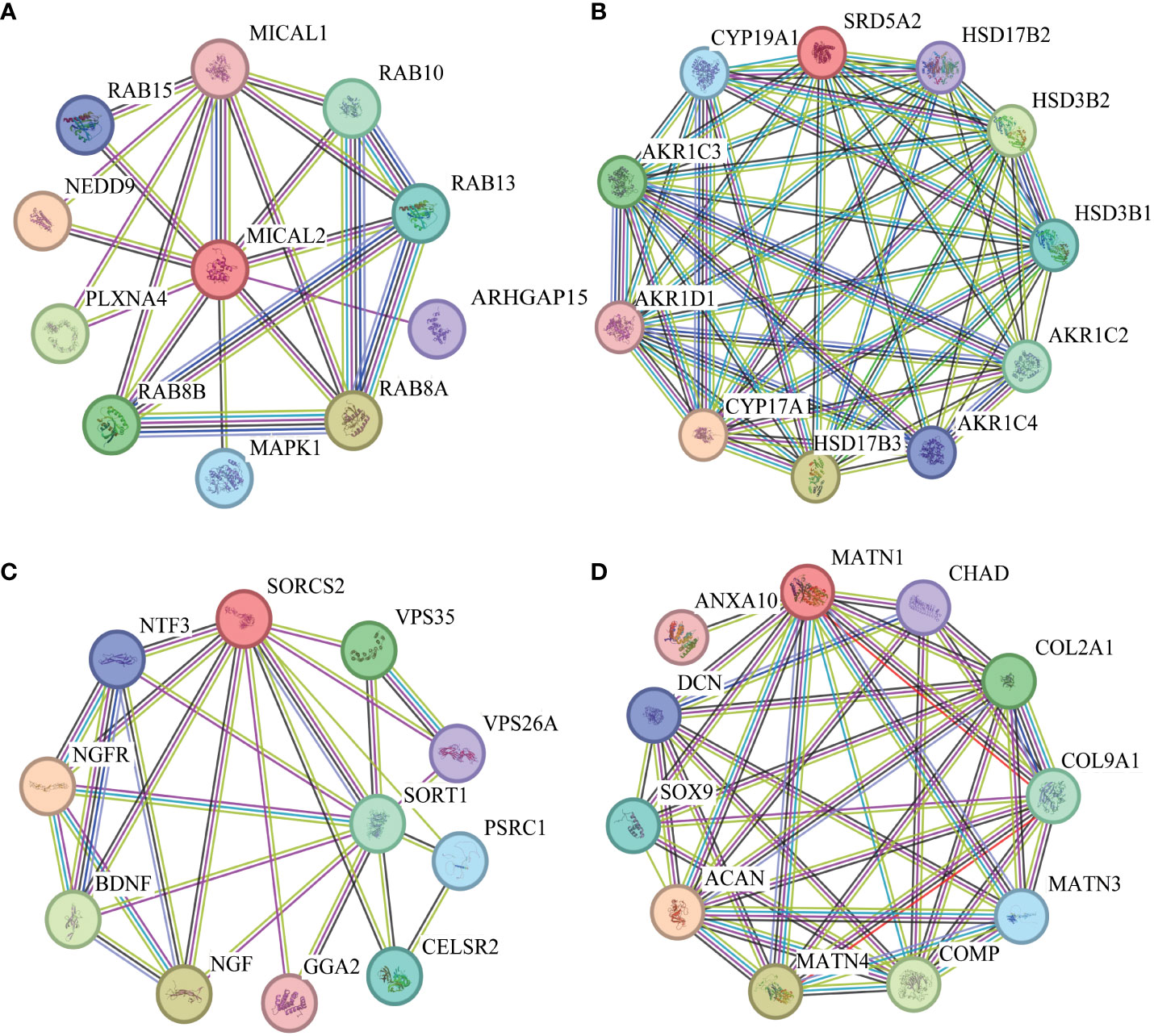
Figure 4 Protein-protein interaction (PPI) networks. (A). PPI network diagram of interaction with MICAL2 protein. (B). PPI network diagram of interaction with SRD5A2 protein. (C). PPI network diagram of interaction with SORCS2 protein. (D). PPI network diagram of interaction with MATN1 protein.
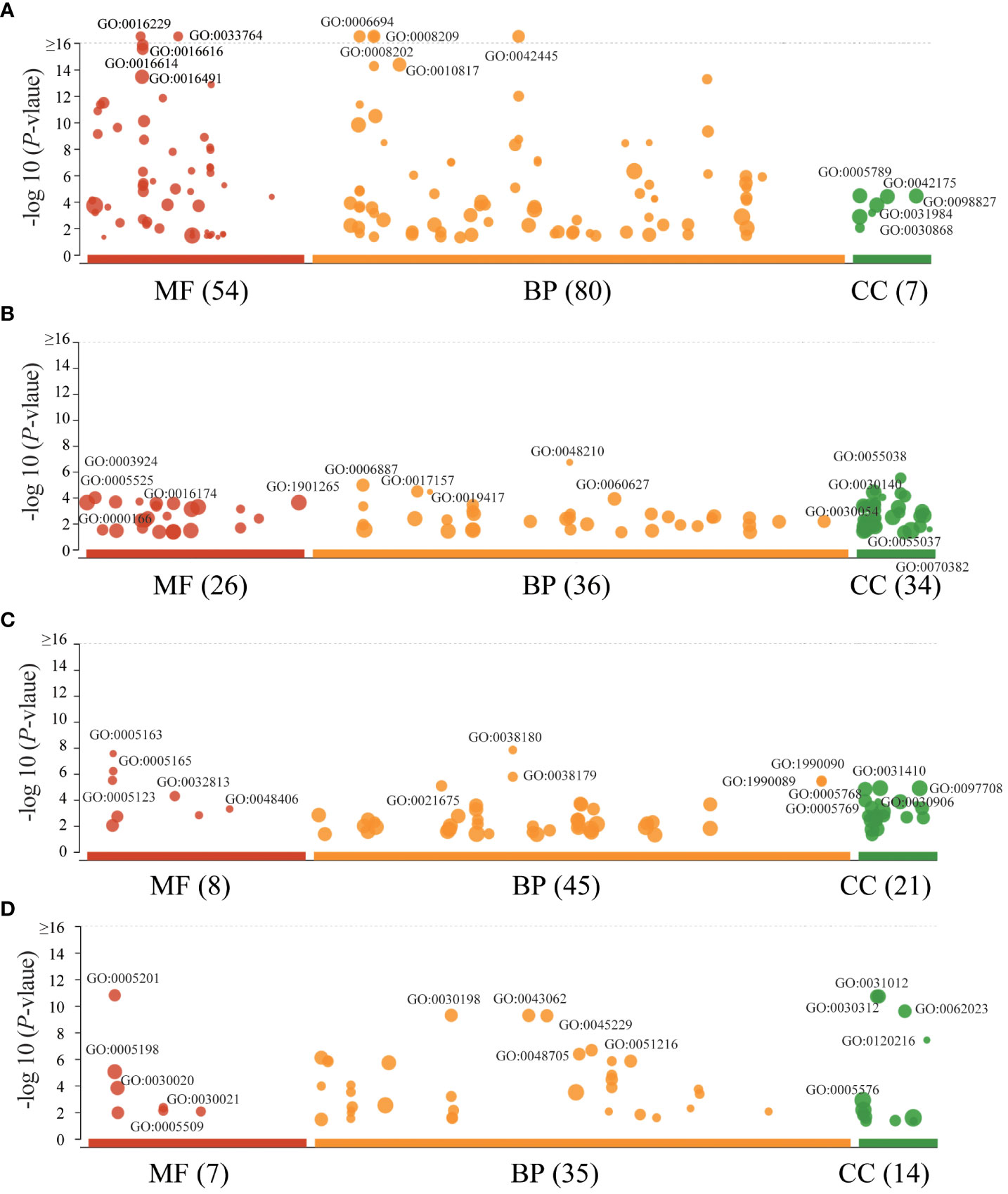
Figure 5 GO enrichment of interaction genes. (A). GO enrichment of MICAL2 interaction genes. (B). GO enrichment of SRD5A2 interaction genes. (C). GO enrichment of SORCS2 interaction genes. (D). GO enrichment of MATN1 interaction genes. The size of data points represents the number of enriched genes. BP, biological process; CC, cellular component; MF, molecular function.
Discussion
Our GWAS results identified numerous novel SNPs significantly associated with lipid traits, including 58 SNPs for TG, 215 SNPs for TC, 74 SNPs for HDL-C, and 81 SNPs for LDL-C, in a Chinese Han prediabetic poputation. Previous studies have shown that several lead SNPs located in or nearby genes, such as SRD5A2, PCSK7, PITPNC1, IRX3, BPI, and LBP, play a role in lipid metabolism (15–19). Meanwhile, enrichment analysis revealed that the lead SNPs located in or nearby genes were significantly enriched in elipid metabolism, insulin secretion pathways, and includin gregulation of hormone levels, exocytosis process.
Most individuals go through a prediabetes stage before progressing to full-blown diabetes. Recent research indicates that the long-term complications of diabetes manifest in individuals with prediabetes. These complications include cardiovascular disease, which is a leading cause of death in diabetes patients (20). It is well-established that dyslipidemia, including elevated TG levels and altered cholesterol profiles, plays a significant role in the development of cardiovascular disease. For instance, plasma TG levels of ≥2 mmol/L have been found to be associated with a two-fold increase in mortality from cardiovascular disease (21). In diabetic patients, elevated TC levels have been linked to an increased risk of cardiovascular disease (22). Increases in LDL-C levels have also been associated with a higher risk of myocardial infarction (23). Additionally, there is a U-shaped association between HDL-C levels and the risk of cardiovascular disease mortality, with a potential interaction between HDL-C levels and glycemic status (24). Therefore, by conducting a GWAS to identify the genetic variations associated with lipid levels in individuals with prediabetes, we can gain insights into the genetic basis of lipid metabolism in prediabetes patients.
Notably, our study identified six SNPs (rs10207755, rs522638, rs523349, rs632148, rs534999, and rs558803) in SRD5A2 that showedassociations with TG levels in individuals with prediabetes. The SRD5A2 gene encodes steroid 5-alpha reductase 2 and has been shown to suppress lipogenesis through inhibiting cortisol effects (15). The SRD5A2 variant rs523349 was previously linked with a higher risk of the progression and death of patients with metastatic prostate cancer (25). Additionally, SNPs in the SIK3-PAFAH1B2 intergenic region and PCSK7, along with rs6032829 in SNAP25, were associated with TG. These genes have demonstrated associations with TG levels in prior genome-wide studies in Korean population (26). Meanwhile, significant associations are detected between SNAP25 polymprphism rs363050 and increasing fasting glucose, HbA1c, and lower insulin in type 2 diabetes mellitus (T2DM) patients (27). WWOX r517797882 was found to be significantly assciated with increased level of TG in T2DM in the Han Chinese population (28). However, it’s important to note that there is no overlap in the genomic locus examined between our study and the earlier investigation. This discrepancy may be attributed to the distinct focus of our study on prediabetes, whereas the previous research specifically addressed T2DM. The differences in the studied populations and conditions highlight the need for targeted investigations tailored to the specific stages and conditions within the spectrum of diabetes-related disorders.
We observed that the PITPNC1 SNP rs2011941 was associated with TC levels in individuals with prediabetes. PITPNC1, a member of the phosphatidylinositol transfer protein family, promotes the thermogenes is of brown adipose tissue (17). In our study, we also identified an intergenic SNP (rs9929651) between IRX3 and CRNDE, as well as multiple SORCS2 SNPs, that showed significant associations with HDL-C levels in individuals with prediabetes. Previous studies have reported a negative correlation between IRX3 expression levels of and serum TC, TG, and LDL-C levels (18). Additionally, SORCS2 has been found to facilitate the release of endostatin from astrocytes and regulate post-stroke angiogenesis (29). Previous studuy also has observed that PSMD6 rs831571 had a significant association with decreased HDL-C, while WWOX r517797882 significantly increased level of HDL-C in T2DM in the Han Chinese population (28). These findings highlight the importance of considering specific genes and pathways in the context of prediabetes and T2DM. However, it is important to note that additional studies are needed to validate these associations and elucidate the underlying mechanisms involved.
Futhermore, several SNPs were significantly associated with LDL-C levels, including rs7254892 in NECTIN2, rs1126757 in IL11, rs547687426 in BPI, rs186778434 in LBP, and rs13045621 near MAFB. These genes have established functional roles in regulating vascular inflammation, fibrosis, and lipid metabolism based on findings from recent studies. For example, recent studies have revealed pro-angiogenic, anti-apoptotic and anti-inflammatory properties of NTN1 as well as preventing vascular dysfunction in diabetes (30). The NECTIN2 cell adhesion molecule has been shown to participate in lipid metabolism and serves as a potential biomarker for disease progression in carotid artery stenosis (31). Additionally, the cytokines IL6 and IL11 are upregulated under glucotoxic conditions, and IL6/IL11-mediated islet fibrosis may contribute to dysfunction in T2DM (32). Furthermore, the BPI-like proteins LBP and BPI are involved in lipid homeostasis and have been implicated in cardiovascular pathogenesis (19). However, the functional effects of many other lead SNPs located in or nearby novel candidate genes still need to be explored and validated experimentally. Elucidating the mechanisms by which these uncharacterized genes influence lipid traits will provide new insights into dyslipidemia in the prediabetic state.
In our study, we found that genes significantly linked to lipid levels in prediabetes are involved in regulating various pathways, including ether lipid metabolism, steroid hormone biosynthesis, cell adhesion molecules, Wnt signaling pathway, fatty acid biosynthesis, MAPK signaling pathway, fatty acid metabolism, and metabolic pathways. Additionally, our GO enrichment analysis showed that proteins interacting with SRD5A2, MICAL1, and MATN1 proteins may be engaged in hormone and steroid metabolism, regulation of exocytosis and vesicle-mediated transport, and extracellular structure organization, respectively. These discoveries strongly indicate that the genes associated with these pathways play a critical role in regulating lipid levels in individuals with prediabetes. Delving deeper into the intricate mechanisms governing these pathways will help uncover potential therapeutic targets and elucidate the molecular mechanisms underlying disease development. This provides valuable insights for further research into the molecular regulation of prediabetes, enriching our overall understanding of the associated biological processes.
This study has several limitations. First, the sample size was small with only 400 patients, which reduces power to detect SNPs with minor effects. Expanding the cohort would improve statistical power. Second, only Southern Han Chinese were included, limiting generalizability to all Chinese prediabetics. Multi-center studies across diverse populations are needed. Third, environmental and lifestyle factors were not well controlled, which could influence blood lipids. Detailed lifestyle data should be collected in future studies and considering gene-gene and gene-environment interactions. Fourth, as discovery research, only preliminary SNP screening was done without functional validation of novel hits. Experimental studies are needed to verify biological roles. Fifth, this study did not identify SNPs related to dyslipidemia in prediabetes. Further research will consider stratified patients in prediabetes according to lipid levels to identify SNPs related to dyslipidemia in prediabetes. Finally, comparison with healthy controls was lacking, thus it remains unclear if identified SNPs are specific to prediabetes. Comparisons to normoglycemic cohorts would aid in elucidating pathogenic mechanisms underlying dyslipidemia. Addressing these limitations in follow-up studies will provide more robust insights into the genetic architecture of lipid abnormalities in prediabetes.
Conclusions
This GWAS provides confirmatory evidence for associations of multiple lipid loci (58 SNPs for TG, 215 SNPs for TC, 74 SNPs for HDL-C, and 81 SNPs for LDL-C) in a prediabetic Southern Chinese Han population, while also uncovering novel SNP associations warranting further functional characterization. The findings yield insights into dyslipidemia genetic mechanisms in prediabetes.
Data availability statement
The data presented in this study are deposited in the Zenodo repository, accessible via the DOI number 10.5281/zenodo.10586090.
Ethics statement
The studies involving humans were approved by Hainan Affiliated Hospital of Hainan Medical University. The studies were conducted in accordance with the local legislation and institutional requirements. The participants provided their written informed consent to participate in this study. Written informed consent was obtained from the individual(s) for the publication of any potentially identifiable images or data included in this article.
Author contributions
HQ: Conceptualization, Project administration, Writing – original draft. ZG: Investigation, Writing – original draft. CP: Data curation, Writing – review & editing. LL: Formal analysis, Methodology, Writing – review & editing. QO: Conceptualization, Methodology, Project administration, Writing – review & editing.
Funding
The author(s) declare financial support was received for the research, authorship, and/or publication of this article. This research was supported by the Hainan Provincial Key Research and Development Project (ZDYF2021SHFZ078 and ZDYF2022SHFZ316), project supported by Hainan Province Clinical Medical Center.
Acknowledgments
Thanks to all participants for their great support of this research.
Conflict of interest
The authors declare that the research was conducted in the absence of any commercial or financial relationships that could be construed as a potential conflict of interest.
Publisher’s note
All claims expressed in this article are solely those of the authors and do not necessarily represent those of their affiliated organizations, or those of the publisher, the editors and the reviewers. Any product that may be evaluated in this article, or claim that may be made by its manufacturer, is not guaranteed or endorsed by the publisher.
Supplementary material
The Supplementary Material for this article can be found online at: https://www.frontiersin.org/articles/10.3389/fendo.2023.1334893/full#supplementary-material
References
1. Cloete L. Diabetes mellitus: an overview of the types, symptoms, complications and management. Nurs standard (Royal Coll Nurs (Great Britain) 1987) (2022) 37(1):61–6. doi: 10.7748/ns.2021.e11709
2. Ong K, Stafford LK, McLaughlin SA, Boyko EJ, Vollset S, Smith AE, et al. Global, regional, and national burden of diabetes from 1990 to 2021, with projections of prevalence to 2050: a systematic analysis for the Global Burden of Disease Study 2021. Lancet (London England) (2023) 402(10397):203–34.
3. Rooney MR, Fang M, Ogurtsova K, Ozkan B, Echouffo-Tcheugui JB, Boyko EJ, et al. Global prevalence of prediabetes. Diabetes Care (2023) 46(7):1388–94. doi: 10.2337/dc22-2376
4. Schlesinger S, Neuenschwander M, Barbaresko J, Lang A, Maalmi H, Rathmann W, et al. Prediabetes and risk of mortality, diabetes-related complications and comorbidities: umbrella review of meta-analyses of prospective studies. Diabetologia (2022) 65(2):275–85. doi: 10.1007/s00125-021-05592-3
7. Neves JS, Newman C, Bostrom JA, Buysschaert M, Newman JD, Medina JL, et al. Management of dyslipidemia and atherosclerotic cardiovascular risk in prediabetes. Diabetes Res Clin Pract (2022) 190:109980. doi: 10.1016/j.diabres.2022.109980
8. Hoffmann TJ, Theusch E, Haldar T, Ranatunga DK, Jorgenson E, Medina MW, et al. A large electronic-health-record-based genome-wide study of serum lipids. Nat Genet (2018) 50(3):401–13. doi: 10.1038/s41588-018-0064-5
9. Deek R, Nasser J, Ghanem A, Mardelli M, Khazen G, Salloum AK, et al. Genome-wide association analysis of HDL-C in a Lebanese cohort. PloS One (2019) 14(6):e0218443. doi: 10.1371/journal.pone.0218443
10. Selvaraj MS, Li X, Li Z, Pampana A, Zhang DY, Park J, et al. Whole genome sequence analysis of blood lipid levels in >66,000 individuals. Nat Commun (2022) 13(1):5995. doi: 10.1038/s41467-022-33510-7
11. Kanai M, Akiyama M, Takahashi A, Matoba N, Momozawa Y, Ikeda M, et al. Genetic analysis of quantitative traits in the Japanese population links cell types to complex human diseases. Nat Genet (2018) 50(3):390–400. doi: 10.1038/s41588-018-0047-6
12. Santoro N, Chen L, Todd J, Divers J, Shah AS, Gidding SS, et al. Genome-wide association study of lipid traits in youth with type 2 diabetes. J Endocrine Soc (2021) 5(11):bvab139. doi: 10.1210/jendso/bvab139
13. Echouffo-Tcheugui JB, Perreault L, Ji L, Dagogo-Jack S. Diagnosis and management of prediabetes: A review. Jama (2023) 329(14):1206–16. doi: 10.1001/jama.2023.4063
14. Khan RMM, Chua ZJY, Tan JC, Yang Y, Liao Z, Zhao Y. From pre-diabetes to diabetes: diagnosis, treatments and translational research. Medicina (Kaunas Lithuania) (2019) 55(9):546. doi: 10.3390/medicina55090546
15. Nasiri M, Nikolaou N, Parajes S, Krone NP, Valsamakis G, Mastorakos G, et al. 5α-reductase type 2 regulates glucocorticoid action and metabolic phenotype in human hepatocytes. Endocrinology (2015) 156(8):2863–71. doi: 10.1210/en.2015-1149
16. Vargas-Alarcón G, Pérez-Méndez O, González-Pacheco H, Ramírez-Bello J, Posadas-Sánchez R, Escobedo G, et al. The rs508487, rs236911, and rs236918 Genetic Variants of the Proprotein Convertase Subtilisin-Kexin Type 7 (PCSK7) Gene Are Associated with Acute Coronary Syndrome and with Plasma Concentrations of HDL-Cholesterol and Triglycerides. Cells (2021) 10(6):1444. doi: 10.3390/cells10061444
17. Tang G, Ma C, Li L, Zhang S, Li F, Wu J, et al. PITPNC1 promotes the thermogenesis of brown adipose tissue under acute cold exposure. Sci China Life Sci (2022) 65(11):2287–300. doi: 10.1007/s11427-022-2157-y
18. Ma Y, Chen G, Yi J, Li Q, Tan Z, Fan W, et al. IRX3 plays an important role in the pathogenesis of metabolic-associated fatty liver disease by regulating hepatic lipid metabolism. Front Endocrinol (2022) 13:895593. doi: 10.3389/fendo.2022.895593
19. Yu Y, Song G. Lipopolysaccharide-binding protein and bactericidal/permeability-increasing protein in lipid metabolism and cardiovascular diseases. Adv Exp Med Biol (2020) 1276:27–35. doi: 10.1007/978-981-15-6082-8_3
20. Brannick B, Dagogo-Jack S. Prediabetes and cardiovascular disease: pathophysiology and interventions for prevention and risk reduction. Endocrinol Metab Clinics North America (2018) 47(1):33–50. doi: 10.1016/j.ecl.2017.10.001
21. Wadström BN, Pedersen KM, Wulff AB, Nordestgaard BG. Elevated remnant cholesterol, plasma triglycerides, and cardiovascular and non-cardiovascular mortality. Eur Heart J (2023) 44(16):1432–45. doi: 10.1093/eurheartj/ehac822
22. Khil J, Kim SM, Chang J, Choi S, Lee G, Son JS, et al. Changes in total cholesterol level and cardiovascular disease risk among type 2 diabetes patients. Sci Rep (2023) 13(1):8342. doi: 10.1038/s41598-023-33743-6
23. Johannesen CDL, Langsted A, Mortensen MB, Nordestgaard BG. Association between low density lipoprotein and all cause and cause specific mortality in Denmark: prospective cohort study. BMJ (Clinical Res ed) (2020) 371:m4266. doi: 10.1136/bmj.m4266
24. Ishibashi T, Kaneko H, Matsuoka S, Suzuki Y, Ueno K, Ohno R, et al. HDL cholesterol and clinical outcomes in diabetes mellitus. Eur J Prev Cardiol (2023) 30(8):646–53. doi: 10.1093/eurjpc/zwad029
25. Shiota M, Fujimoto N, Yokomizo A, Takeuchi A, Itsumi M, Inokuchi J, et al. SRD5A gene polymorphism in Japanese men predicts prognosis of metastatic prostate cancer with androgen-deprivation therapy. Eur J Cancer (Oxford Engl 1990) (2015) 51(14):1962–9. doi: 10.1016/j.ejca.2015.06.122
26. Gombojav B, Lee SJ, Kho M, Song YM, Lee K, Sung J. Multiple susceptibility loci at chromosome 11q23.3 are associated with plasma triglyceride in East Asians. J Lipid Res (2016) 57(2):318–24.
27. Al-Daghri NM, Costa AS, Alokail MS, Zanzottera M, Alenad AM, Mohammed AK, et al. Synaptosomal protein of 25 kDa (Snap25) polymorphisms associated with glycemic parameters in type 2 diabetes patients. J Diabetes Res (2016) 2016:8943092. doi: 10.1155/2016/8943092
28. Permana S, Lukman H, Norahmawati E, Eka Puspita O, Faisal Moh Al Zein D, Kawamoto Y, et al. East Asian Genome-wide association study derived loci in relation to type 2 diabetes in the Han Chinese population. Acta Biochim Polonica (2019) 66(2):679–86.
29. Malik AR, Lips J, Gorniak-Walas M, Broekaart DWM, Asaro A, Kuffner MTC, et al. SorCS2 facilitates release of endostatin from astrocytes and controls post-stroke angiogenesis. Glia (2020) 68(6):1304–16. doi: 10.1002/glia.23778
30. Toque HA, Fernandez-Flores A, Mohamed R, Caldwell RB, Ramesh G, Caldwell RW. Netrin-1 is a novel regulator of vascular endothelial function in diabetes. PloS One (2017) 12(10):e0186734. doi: 10.1371/journal.pone.0186734
31. Li S, Gao Y, Ma K, Li Y, Liu C, Yan Y, et al. Lipid-related protein NECTIN2 is an important marker in the progression of carotid atherosclerosis: An intersection of clinical and basic studies. J Trans Internal Med (2021) 9(4):294–306. doi: 10.2478/jtim-2021-0044
Keywords: GWAS, prediabetes, HDL-C, TC, TG, LDL-C
Citation: Gao Z, Pu C, Lin L, Ou Q and Quan H (2024) Genome-wide association study of blood lipid levels in Southern Han Chinese adults with prediabetes. Front. Endocrinol. 14:1334893. doi: 10.3389/fendo.2023.1334893
Received: 08 November 2023; Accepted: 22 December 2023;
Published: 02 February 2024.
Edited by:
Kai Guo, University of Michigan, United StatesReviewed by:
Yue Liu, The University of Texas at Austin, United StatesDa Zhang, Benitec Biopharma, Inc., United States
Copyright © 2024 Gao, Pu, Lin, Ou and Quan. This is an open-access article distributed under the terms of the Creative Commons Attribution License (CC BY). The use, distribution or reproduction in other forums is permitted, provided the original author(s) and the copyright owner(s) are credited and that the original publication in this journal is cited, in accordance with accepted academic practice. No use, distribution or reproduction is permitted which does not comply with these terms.
*Correspondence: Huibiao Quan, qhb13876078153@hainmc.edu.cn
†These authors have contributed equally to this work