- 1Department of Spine and Osteopathy Ward, The First Affiliated Hospital of Guangxi Medical University, Nanning, China
- 2Department of Operating Room, Taixing People’s Hospital, Taixing, China
- 3Reproductive Medicine Center, The First Afliated Hospital of Guangxi Medical University, Nanning, China
Objective: The relationship between different autoimmune diseases and bone mineral density (BMD) and fractures has been reported in epidemiological studies. This study aimed to explore the causal relationship between autoimmune diseases and BMD, falls, and fractures using Mendelian randomization (MR).
Methods: The instrumental variables were selected from the aggregated statistical data of these diseases from the largest genome-wide association study in Europe. Specifically, 12 common autoimmune diseases were selected as exposure. Outcome variables included BMD, falls, and fractures. Multiple analysis methods were utilized to comprehensively evaluate the causal relationship between autoimmune diseases and BMD, falls, and fractures. Additionally, sensitivity analyses, including Cochran’s Q test, MR-Egger intercept test, and one analysis, were conducted to verify the result’s reliability.
Results: Strong evidence was provided in the results of the negatively association of ulcerative colitis (UC) with forearm BMD. UC also had a negatively association with the total body BMD, while inflammatory bowel disease (IBD) depicted a negatively association with the total body BMD at the age of 45–60 years. Horizontal pleiotropy or heterogeneity was not detected through sensitivity analysis, indicating that the causal estimation was reliable.
Conclusion: This study shows a negative causal relationship between UC and forearm and total body BMD, and between IBD and total body BMD at the age of 45–60 years. These results should be considered in future research and when public health measures and osteoporosis prevention strategies are formulated.
Introduction
Osteoporosis, a common systemic bone disease, is mainly characterized by reduced bone mineral density (BMD), bone tissue deterioration and structure destruction, and increased risk of fractures (1, 2). Osteoporosis is an increasingly critical public health challenge worldwide. There is a significant increase in the incidence rate with age, and leads to hip fracture, which leads to an increase in mortality (3, 4). However, the pathogenesis and risk factors of osteoporosis are still poorly comprehended; therefore, prevention and treatment remain a huge challenge. Currently, an effective method for diagnosing osteoporosis and evaluating the risk of brittle fractures is through BMD measurement (5). BMD is a feature with highly polygenic influence, as depicted by the Genome-wide association study (GWAS), and some of the genetic determinants may cause low BMD, leading to fractures (6, 7).
Autoimmune diseases have been reported to have a significant impact on bone health, leading to an enhanced osteoporosis and fracture risk; however, the exact mechanism is ambiguous (8). Chronic inflammation associated with autoimmune diseases can damage bone and reduce bone density, making it more prone to fractures (9). Additionally, autoimmunity can lead to an overall decrease in calcium levels through a decrease in calcium absorption and an increase in calcium excretion (10). Although epidemiological studies have shown some association between autoimmune diseases and osteoporosis, whether these associations are causal should be further explored. Unmeasurable confounding factors and reverse causal relationships limit traditional epidemiological research, possibly leading to deviations, thus, hindering the possibility of inferring causal relationships (11). Nevertheless, the determination of whether a causal relationship exists between autoimmune diseases and osteoporosis can point out specific biological pathways and provide information for prevention strategies.
A new method of epidemiological etiology inference is Mendelian randomization (MR), which can effectively offset the bias caused by confounding factors and reverse causality by using genetic variation as an instrumental variable (IV), assist in identifying the causal relationship between exposure and results, and modify the genetic mechanism of human genetics and complex diseases (12, 13). Additionally, GWAS provides a robust and reliable IV for MR research using a genetic variation. Alleles follow the law of independent classification and can reduce the confounding effect of environmental factors, which mimics the design of randomized controlled trials (RCT) (14). In recent years, the causal relationship between multiple complex diseases, including autoimmune diseases, has been assessed widely using MR methods (15, 16).
Thus, we used summary statistics from the largest available genome-wide datasets in this study and applied the MR method to determine the evidence of causal relationships between autoimmune diseases (celiac disease (CeD), rheumatoid arthritis (RA), Crohn’s disease (CD), psoriasis (PsO), primary sclerosing cholangitis (PSC), asthma, inflammatory bowel disease (IBD), primary biliary cirrhosis (PBC), ulcerative colitis (UC), type 1 diabetes (T1D), systemic lupus erythematosus (SLE), ankylosing spondylitis (AS)) and BMD, falls, and fractures. The term “autoimmune” will be used to describe both immune and inflammatory disorders (such as asthma).
Materials and methods
Research design
This dual-sample MR study aimed to explore the potential causal relationship between autoimmune diseases and the risk of BMD, falls, and fractures. The single nucleotide polymorphism (SNP) used as a tool must fulfill the following assumptions to obtain convincing conclusions: 1.The relevance assumption, IVs must be strongly associated with exposure; 2. The exclusion restriction assumption, IVs should only affect the outcomes through exposure; 3. The independence assumption, IVs cannot be associated with any possible confounding factors (17). These three key assumptions are illustrated in Figure 1. MR analysis strictly follows the Strengthening Epidemiological Observation Research Report (STROBE) guidelines (18). All original studies obtained ethical recognition and informed consent.
Data resources
Summary-level data from the largest publicly available GWAS for MR analysis of each trait was used for the exposure. The IEU OpenGWAS project provided the summary statistical data of 12 common autoimmune diseases, including CeD, RA, CD, PsO, PSC, asthma, IBD, PBC, UC, T1D, SLE and AS (19). All study participants were of European origin. The diagnostic criteria of the disease are presented in the original publication.
For the outcome, BMD loss in the forearm, femoral neck, lumbar spine, and heel enhances the risk of osteoporosis and fracture more than the other body parts (20). Therefore, the summary statistical data (21) of BMD of the forearm, femoral neck, and lumbar spine were obtained from the GEnetic Factors for OSteoporosis Consortium (GEFOS), including 8143, 32735, and 28498 participants. The GWAS dataset (22) of the UK Biobank provided the summary statistics of heel BMD, including 426,824 participants. The BMD measurement method of the study participants is described in detail in the original publication. Age has been considered a risk factor for osteoporosis (23). Therefore, a large GWAS meta-analysis was used for obtaining the summary statistical data (24) of the whole body BMD of these five age groups, that is, under 15, 15–30, 30–45, 45–60, and over 60 years, including a total of 66,628 participants. Osteoporosis results in fractures of the fragile bones (1). Therefore, the summary statistical data of fractures of various parts such as the arm, ankle, leg, spine, wrist, and other bones were obtained from the MRC IEU OpenGWAS database, including 460,340 and 306,379 participants with fractures of the heel (left and right heels). Additionally, the summary statistical data of falls from the MRC IEU OpenGWAS database was also obtained, including a total of 461,725 participants (25, 26).
Genetic tool variable selection
After obtaining GWAS summary data of various autoimmune diseases, a series of quality control steps were carried out to ensure that SNPs identified as IV must be closely related to exposure. First, SNPs with genome-wide significance were extracted (P<5×10-8). Secondly, to avoid bias, we prune SNPs with r2<0.001 threshold with a 10,000 kb window to exclude SNPs in strong linkage disequilibrium (27, 28). Additionally, SNPs corresponding to exposure-related phenotypes were removed through Phenoscanner V2 to eliminate potential pleiotropic effects. Finally, the variance proportion of the exposure is calculated using the R2 value of each SNP, and instrument bias is avoided by using F-statistics to estimate the instrument strength (29).
Statistical analysis
In this study, MR-Egger, weighted median (WM), inverse variance weighted (IVW), simple mode, and weighted mode were used to conduct MR analysis to better evaluate the causal relationship between autoimmune diseases and BMD, falls, and fractures. When the level of pleiotropy of IVs does not exist, IVW has the highest statistical power; therefore, it is used as the main analysis method (30, 31). Different types of genetic pleiotropy are considered by other MR methods and are based on potentially different assumptions to check the robustness of the results. For example, WM can still provide unbiased causal estimation, when more than 50% of the information comes from invalid IVs (32). the MR-Egger method can also provide an unbiased estimation of causality when all IVs do not meet the core MR hypothesis (33). The effect of exposure on outcome was expressed using an odds ratio (OR) and 95% confidence interval (95% CI).
Sensitivity analysis
A series of sensitivity analyses, including heterogeneity tests (IVW and MR-Egger), horizontal pleiotropy (MR-Egger intercept and MR-PRESSO), and retention analysis, were conducted to rule out the possible violation of the MR hypothesis (Figure 1). The IV and size of heterogeneity are tested using the p-value of Cochran’s Q statistics evaluated by I2 statistics (34). The outliers are detected using MR-PRESSO analysis (35). The level of pleiotropy is identified from the deviation between intercept and zero in MR-Egger analysis (33). Additionally, an analysis is left to evaluate whether the causal effect estimation may be biased or interfered with by a single SNP (30). The results are visualized using funnel and forest plots. All MR analyses were performed using the “TwoSampleMR” package in R version 4.2.1. P<0.05 is considered statistically significant.
Results
The causal relationship between autoimmune diseases and bone mineral density
The different body regions have different causal effects of autoimmune diseases on BMD. Based on the IVW results, UC was negatively associated with BMD of the forearm (OR = 0.949, 95% CI = 0.917–0.981, P = 0.002) (Figure 2). In the analysis of the heterogeneity test, neither IVW nor Cochran’s Q test of the MR-Egger method found evidence of heterogeneity. Directional pleiotropy was not demonstrated using the MR-Egger intercept test results. Additionally, the remaining analysis also proved that the robustness of MR affected estimation (Figure 3) (Table 1). However, the IVW method did not demonstrate any statistically significant relationship between other autoimmune diseases and BMD in different body regions, and the robustness of MR affecting estimation was proved by the sensitivity analysis (Supplementary Material).
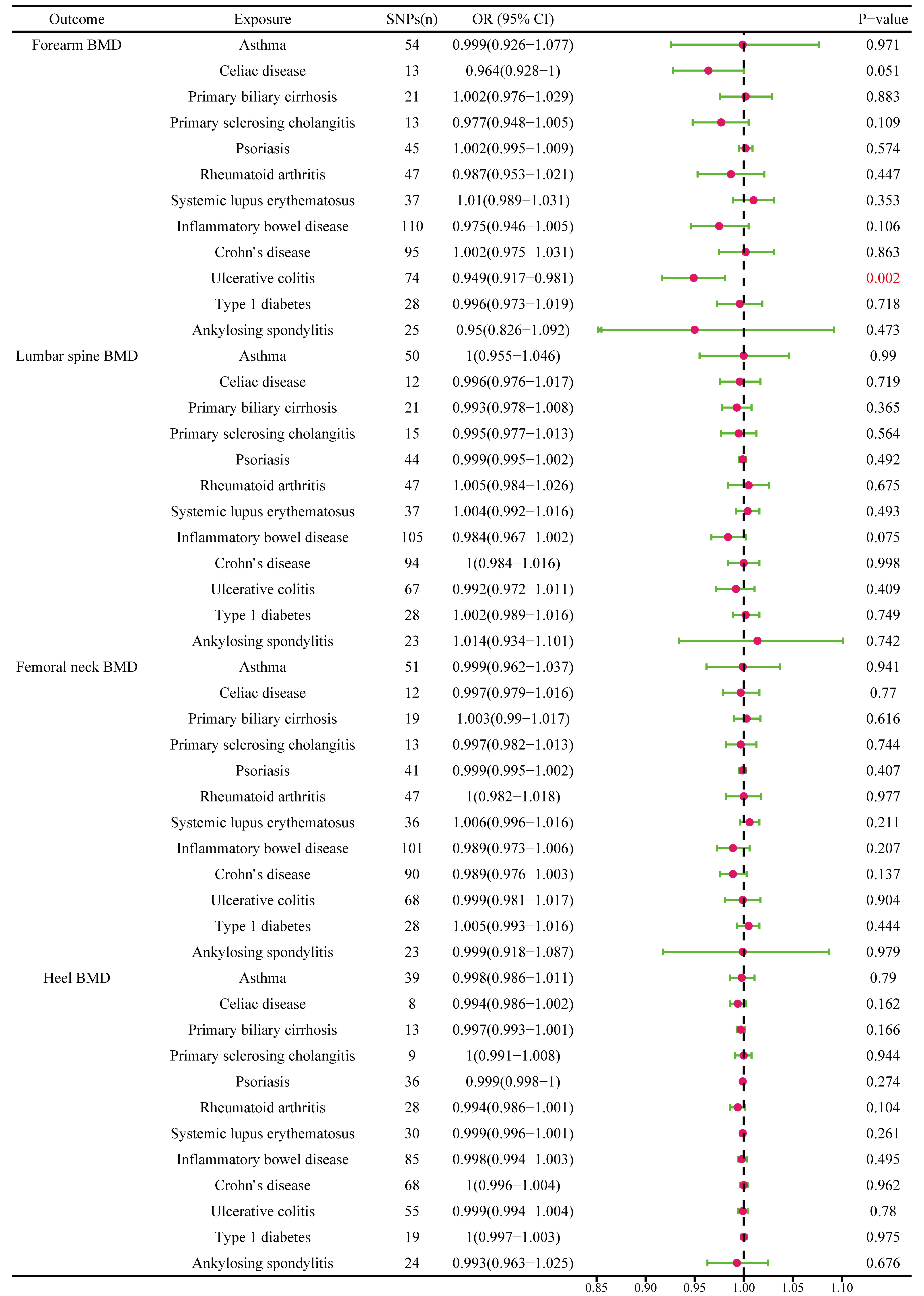
Figure 2 The causal effect of autoimmune diseases on different parts of the body BMD. IVW, inverse variance weighted; CI, confidence interval; BMD, bone mineral density; SNP, single nucleotide polymorphism.
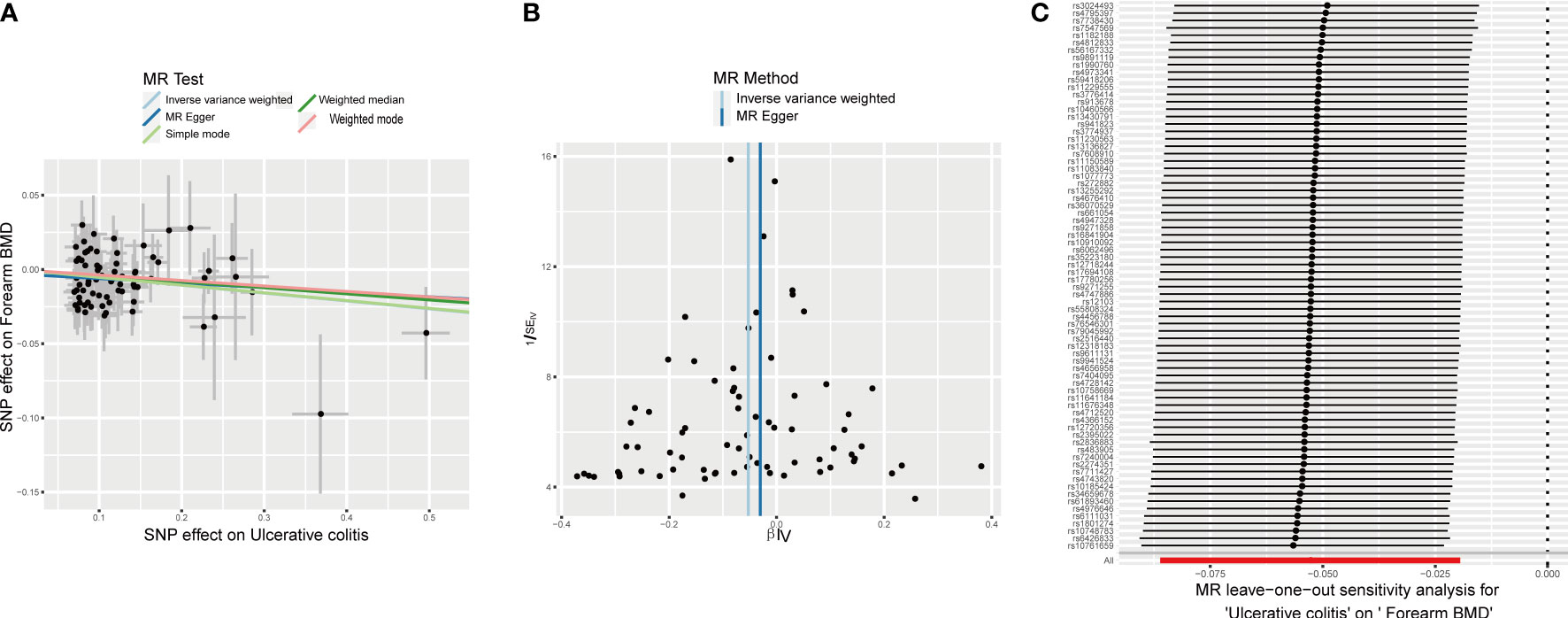
Figure 3 Effects of UC on forearm BMD. (A) Scatter plot. The slopes of each line represent the causal association for each method. (B) Funnel plot. (C) Leave-one-out analysis.
The causal effect of autoimmune diseases on total body BMD and that at different ages. Based on the IVW results, UC was negatively associated with the total body BMD (OR = 0.981, 95% CI = 0.969–0.994, P = 0.003), while IBD was negatively associated with the total body BMD at the age of 45–60 years (OR = 0.977, 95% CI = 0.957–0.998, P = 0.033) (Figure 4). No evidence of heterogeneity was found using Cochran’s Q test of IVW and MR-Egger methods. Directional pleiotropy was not demonstrated by the MR-Egger intercept test results. Additionally, the robustness of MR effect estimation was also proved by the remaining analysis (Figure 5) (Table 1). However, no significant relationship existed between other autoimmune diseases and systemic BMD and that of different age groups observed in the IVW method. Sensitivity analysis also proved the robustness of MR effect estimation (Supplementary Material).
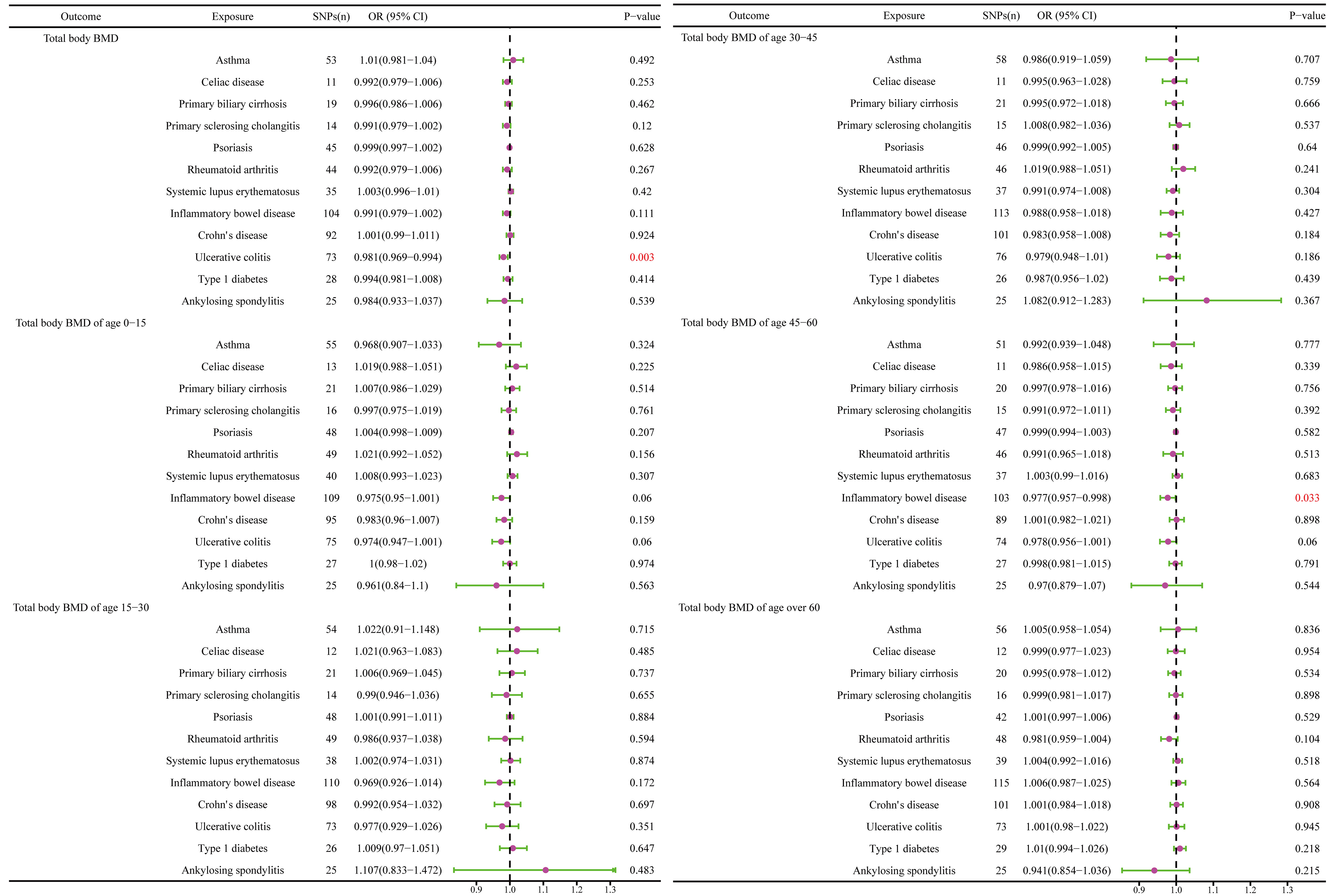
Figure 4 The causal effect of autoimmune diseases on total body BMD and that at different ages. IVW, inverse variance weighted; CI, confidence interval; BMD, bone mineral density; SNP, single nucleotide polymorphism.
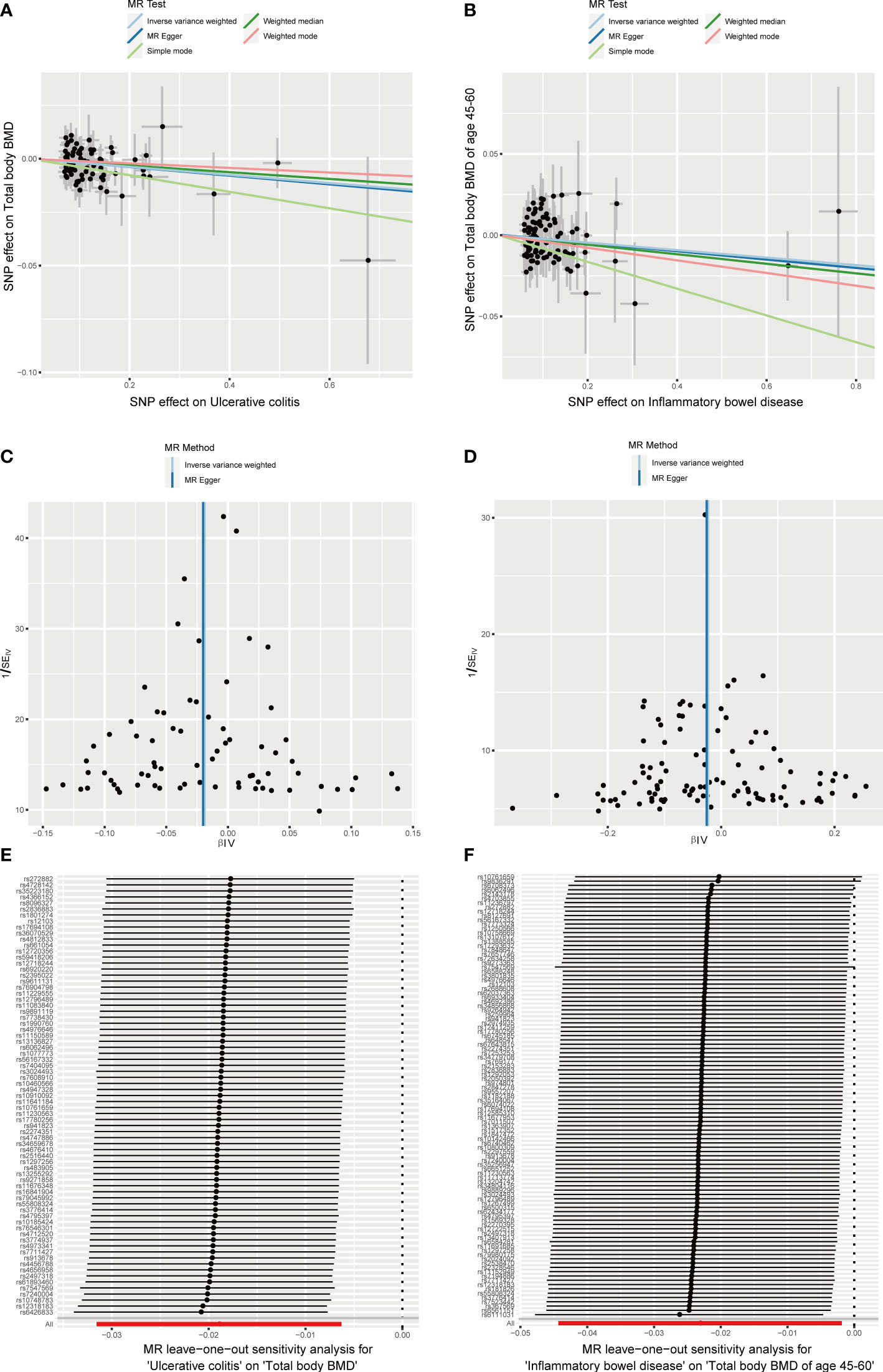
Figure 5 Effects of UC and IBD on total body BMD and that at different ages. (A, B) Scatter plot. The slopes of each line represent the causal association for each method. (C, D) Funnel plot. (E, F) Leave-one-out analysis.
The causal relationship between autoimmune diseases and fall risk
RA was positively associated with the falls (OR = 1.003, 95% CI = 1.000–1.005, P = 0.04), which was supported by the weighted median analysis (OR = 1.003, 95% CI = 1.000–1.007, P = 0.049). Additionally, T1D was weakly positively associated with falls (OR = 1.002, 95% CI = 1.000–1.003, P = 0.016) (Figure 6). No evidence of heterogeneity was detected using Cochran’s Q test of IVW and MR-Egger methods. Directional pleiotropy was not demonstrated using MR-Egger intercept test results. Additionally, the remaining analysis also proved the robustness of MR effect estimation (Figure 7) (Table 1). However, no significant relationship existed between other autoimmune diseases and systemic BMD and that of different age groups observed in the IVW method. Sensitivity analysis also proved the robustness of MR effect estimation (Supplementary Material).
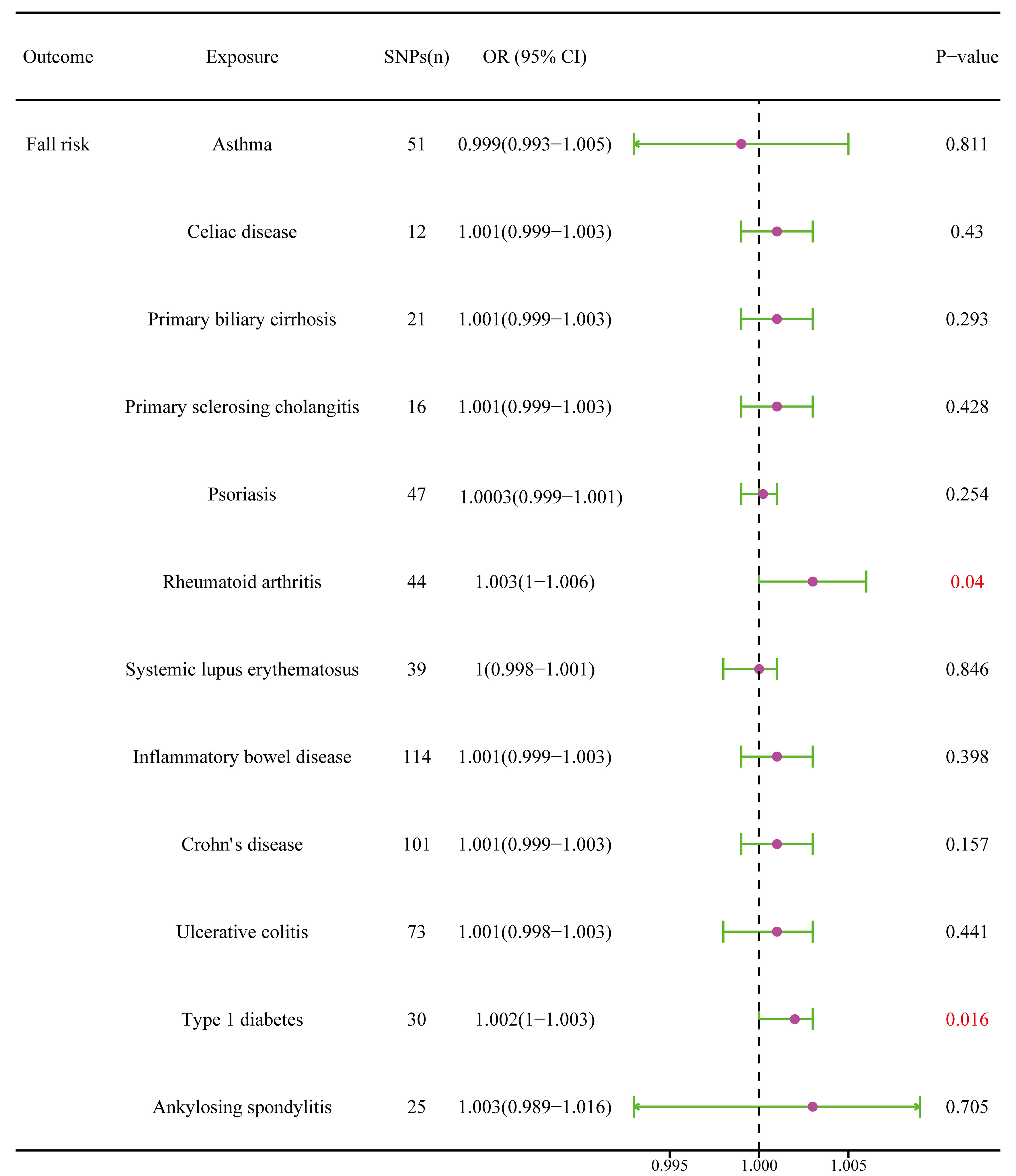
Figure 6 The causal effect of autoimmune diseases on fall risk. IVW, inverse variance weighted; CI, confidence interval; BMD, bone mineral density; SNP, single nucleotide polymorphism.
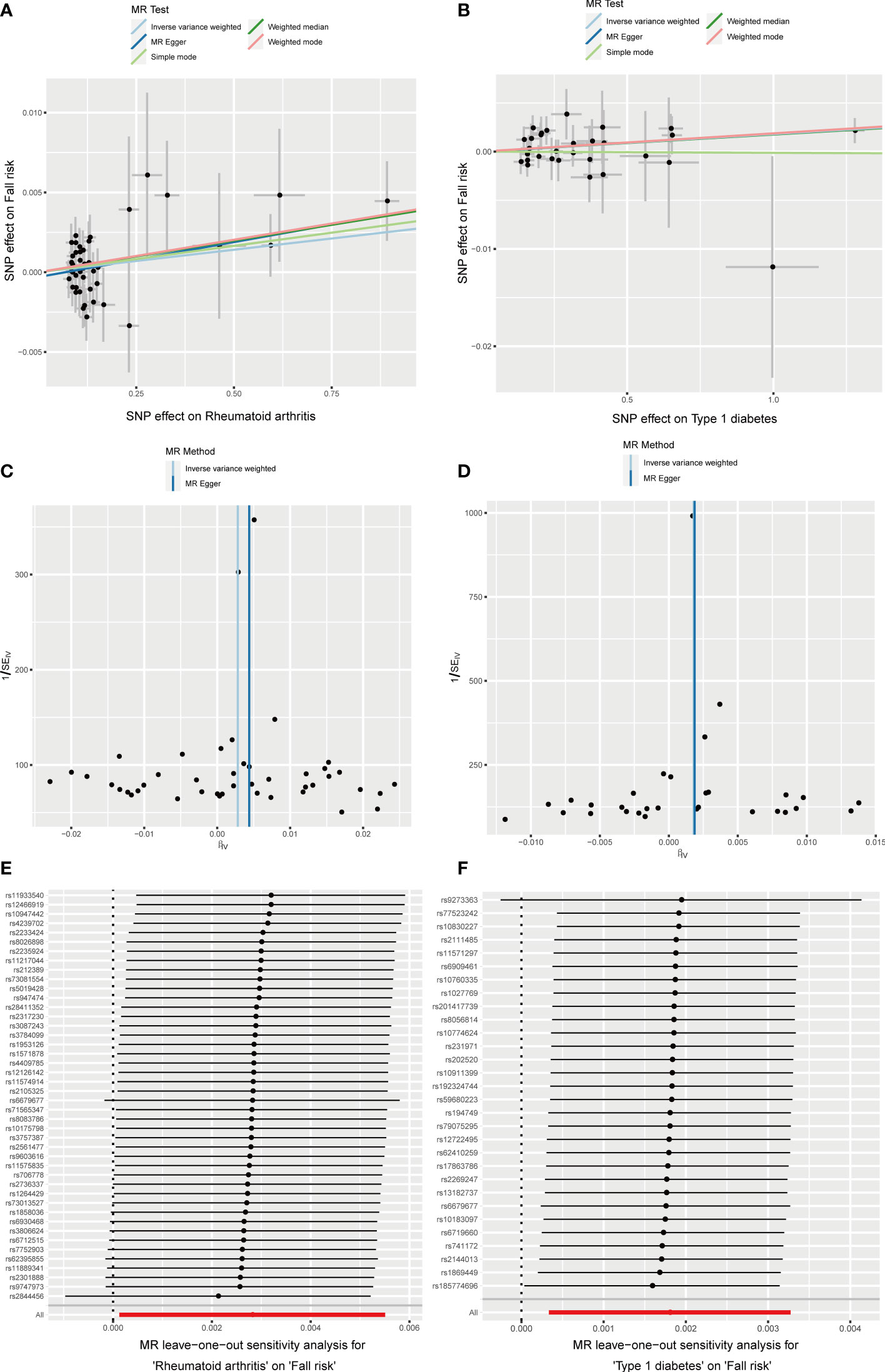
Figure 7 Effects of RA and T1D on fall risk. (A, B) Scatter plot. The slopes of each line represent the causal association for each method. (C, D) Funnel plot. (E, F) Leave-one-out analysis.
The causal relationship between autoimmune diseases and fracture risk
The MR results between autoimmune disease and fracture risks are shown in the table. Based on the IVW results, PSC was weakly positively associated with the risk of wrist fractures (OR = 1.0007, 95% CI = 1.0001–1.0012, P = 0.014), and several immune diseases were weakly positively associated with the increased risk of left heel fractures, including IBD (OR = 1.0009, 95% CI = 1.0003–1.0016, P = 0.006); CD (OR = 1.0006, 95% CI = 1.0001–1.0012, P = 0.033); and UC (OR = 1.0008, 95% CI = 1.0001–1.0015, P = 0.025) (Figure 8). No evidence of heterogeneity was found using Cochran’s Q test of IVW and MR-Egger methods. MR-Egger intercept test results did not show directional pleiotropy. Additionally, the remaining analysis also proved the robustness of MR effect estimation (Figure 9) (Table 1).
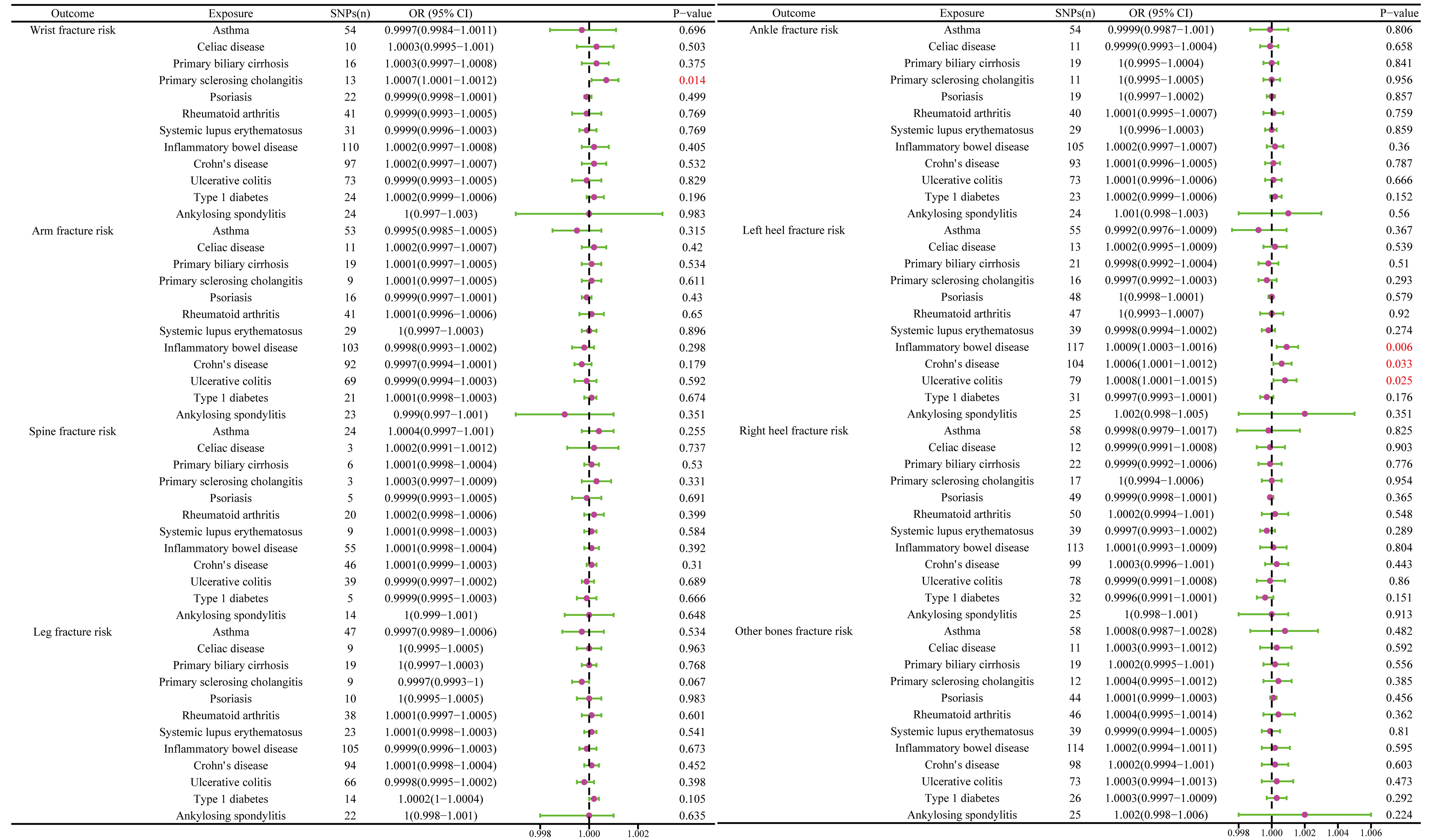
Figure 8 The causal effect of autoimmune diseases on fracture risk. IVW, inverse variance weighted; CI, confidence interval; BMD, bone mineral density; SNP, single nucleotide polymorphism.
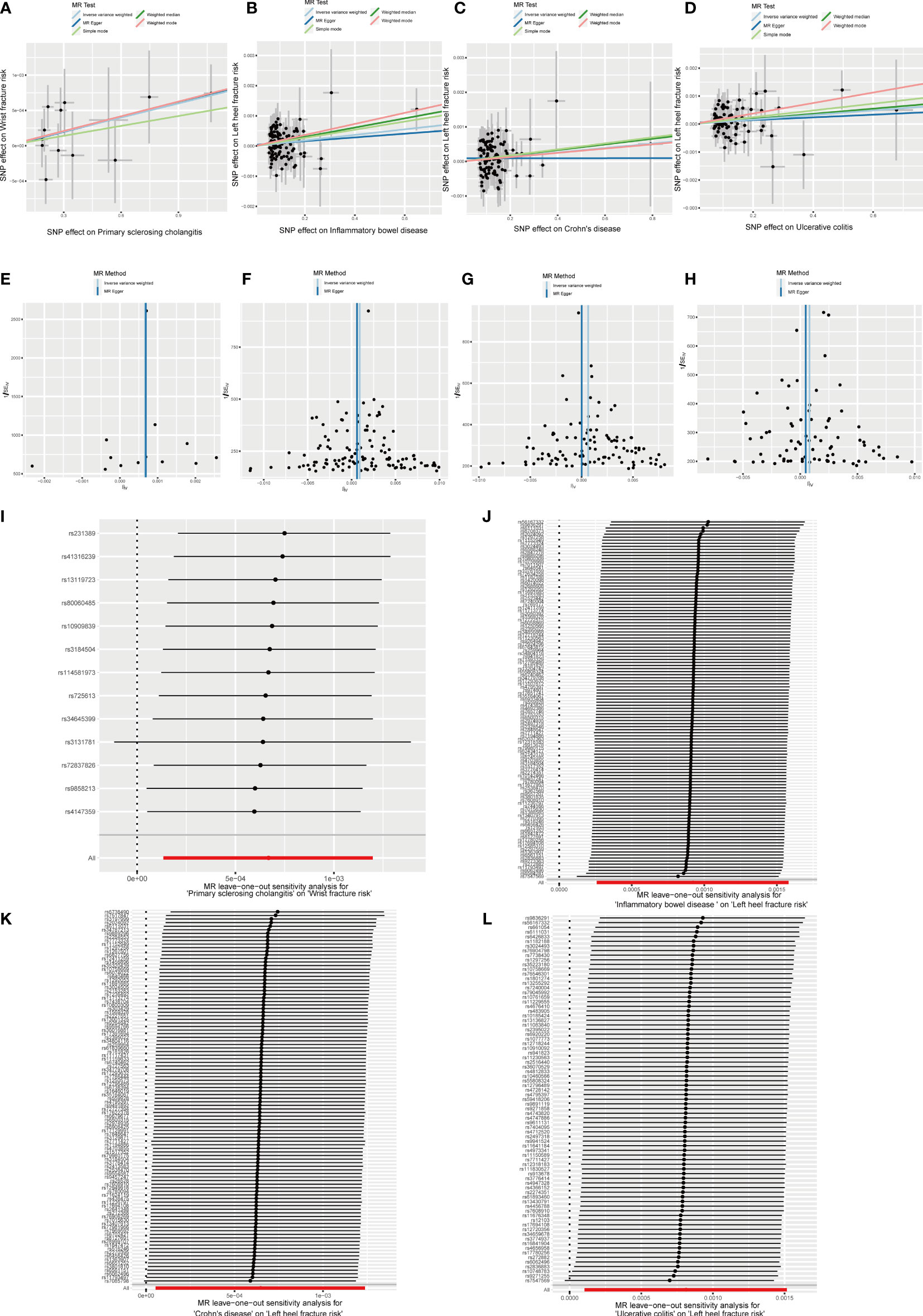
Figure 9 Effects of PSC, IBD, CD and UC on fracture risk. (A–D) Scatter plot. The slopes of each line represent the causal association for each method. (E–H) Funnel plot. (I–L) Leave-one-out analysis.
Discussion
This study aimed to explore the causal relationship between autoimmune diseases and BMD, falls, and fractures. The causal relationship between different immune diseases and BMD in different body regions, different age levels, and falls and fractures in different body parts was assessed using GWAS data, and the two-sample Mendelian randomization analysis was conducted. The study results demonstrated a negative causal relationship between UC and forearm and body BMD, and that between IBD and body BMD at the age of 45–60 years. A weakly positive causal relationship existed between RA and T1D and falls and between PSC and the risk of wrist fractures. There was a positive causal relationship with the risk of left heel fractures. However, there was no evidence to support the causal relationship between other immune diseases and outcomes.
Osteoporosis is mainly characterized by reduced BMD and increased fracture risk. Research shows that many autoimmune rheumatic diseases have been proven to be complicated by systemic and local bone loss (36–38). However, this pathogenic process has multifactorial physiology and pathology, including treatment, fixation, and reduction of physical activity caused by musculoskeletal symptoms (39). BMD reduction in autoimmune diseases is determined by high disease activity, long disease duration, and joint injury (40). Some patients may develop focal or systemic bone loss before the diagnosis of autoimmune diseases. In RA patients without disease-modifying antirheumatic drugs or corticosteroids, BMD is mainly related to demographic factors such as age and gender (40, 41). Thus, these findings suggest that other factors, such as antirheumatic treatment, may partly mediate the reduction of BMD in some patients with autoimmune diseases (42–44). Therefore, whether autoimmune diseases have a direct impact on osteoporosis is still ambiguous.
IBD encompassing both UC and CD, is a chronic, recurrent, inflammatory disorder of the gastrointestinal tract (45). In recent years, the association between IBD and BMD has been widely explored. A prospective study showed BMD reduction in both CD and UC patients (46). Another study found that BMD values of young male patients with IBD not using corticosteroids were lower than those of healthy controls (47). Conversely, Bernstein et al. demonstrated that the decrease in BMD in patients with IBD was associated with the use of corticosteroids but not with the disease itself (48). The main determinants of BMD in patients with CD are sex, age, and body weight (49). This may be the key factor contributing to the discrepancy between the conclusions owing to the presence of many confounders in traditional observational studies. MR analysis based on the random distribution of genetic variation can effectively overcome the bias caused by hybrid and reverse causal problems and provide more reliable results compared with observational research (12). Recently, Wu et al. also used MR to analyze the causal relationship between IBD and BMD. These results support the causal effect of UC on bone BMD reduction in the whole body and forearm BMD. The CD has a clear causal relationship with the reduction of bone BMD in the femoral neck (50). However, this study was limited owing to its small sample size, and only the causal relationship between IBD and BMD in different body regions was explored, without exploring the causal relationship between IBD and BMD at different age levels and the risk of falling and fractures. Therefore, a larger sample size was used, and a more comprehensive assessment was conducted in this study, which not only proved a negative causal relationship between UC and forearms BMD and body BMD but also found a negative causal relationship between IBD and body BMD at the age of 45–60 years. Osteoporosis is an important risk factor for accidents, disability, and death (51). Therefore, this finding is of great significance to public health. Fractures reduce quality of life and independence and increase the risk of mortality. The result is a significant economic and social burden, which will increase even more with an aging population (52). With approximately 18 million wrist and hand fractures worldwide, wrist fractures are the most common type, constituting a huge medical burden (53). Heel fractures account for 1-2% of all fractures, whereas talar fractures account for approximately 60% and have a higher incidence and disability rate, making it a significant public health concern (54–56). Research has found that risk factors for heel fractures include osteoporosis, diabetes, and others (55, 57). In this study, we found a positive causal relationship between PSC and the risk of wrist fractures. Additionally, a positive causal relationship was found between IBD, including UC and CD, and the risk of left heel fractures. However, we noticed that the OR values of these results are very close to 1, and the confidence interval is also very close to 1, indicating that these associations are very weak and may not have clinical significance. Therefore, more research is needed to verify these associations.
Prevention of falls is a major public health challenge with significant associations with immune-mediated diseases. Studies have found that the fall rate among patients with multiple sclerosis is always >50%, leading to a 2–3 fold higher rate of injuries and fractures compared to age-matched controls (58). Kensuke et al.’s study also found that the risk of falls was increased in patients with RA, with reported incidence rates of 30–60% (59, 60) and a higher risk of falls-related fractures than the general population (61). Therefore, the causal relationship between the risk of falls in patients with immune-mediated diseases must be explored to prevent the consequences of falls and treating osteoporosis. Our study found a positive causal relationship between falls and RA and T1D among many immune-mediated diseases, indicating that early interventions are essential to reduce the risk of injury and costly falls.
However, this study has several limitations. First, in the two-sample MR analysis, no overlap of participants should exist between the exposure and outcome samples. However, in this study, we were unable to estimate the degree of overlap. For larger consortia summary statistics with sample overlap to the second dataset used, the direction and extent of bias are unknown, but in very large alliances, the bias of weak tools may not be significant, but in medium-sized alliances, potential biases and exaggerated Type 1 error rates should be investigated (62). Therefore, by calculation, for larger consortia summary statistics with sample overlap to the second dataset used, the bias is 0.001 and the Type 1 error rate is 0.05. In addition, we conducted sensitivity analyses to assess the robustness of our results to different assumptions about the strength of the instruments and the potential for sample overlap (33). Secondly, using summary data from large GWAS datasets cannot analyze stratified risk factors related to disease duration, severity, treatment, and complications. Additionally, most of the participants included in this MR analysis were Europeans; therefore, our conclusions should be validated in other populations. Finally, although measures were taken to exclude outlier variants, the effect of pleiotropic effects on the results could not be excluded; thus, larger sample MR studies or RCTs are needed to obtain more accurate results, and confounding factors may still act through the second hypothesis of MR.
Conclusion
In conclusion, a negative causal relationship exists between UC and forearm and body BMD, and between IBD and body BMD at the age of 45–60 years. However, no evidence exists to support the causal relationship between other immune diseases and outcomes. These results should be considered in future research and when formulating public health measures and osteoporosis prevention strategies.
Data availability statement
The datasets presented in this study can be found in online repositories. The names of the repository/repositories and accession number(s) can be found in the article/Supplementary Material.
Ethics statement
All original studies obtained ethical recognition and informed consent. Written informed consent to participate in this study was provided by the participants’ legal guardian/next of kin. Written informed consent was obtained from the individual(s) for the publication of any potentially identifiable images or data included in this article. Ethical review and approval was not required for the study on human participants in accordance with the local legislation and institutional requirements. Written informed consent for participation was not required for this study in accordance with the national legislation and the institutional requirements.
Author contributions
SFW, CL, and XZ designed the study. LC and JZ analyzed the data. YYan, ZY, CZ, and BF processed the digital visualization. SFW wrote and revised the manuscript. CL, LR and XZ revised the manuscript. All co-authors participated in the laboratory operation. All authors contributed to the article and approved the submitted version.
Funding
This work was supported by the “Medical Excellence Award” Funded by the Creative Research Development Grant from the First Affiliated Hospital of Guangxi Medical University and the Guangxi Young and Middle aged Teacher’s Basic Ability Promoting Project. (Grant/Award Number: 2020KY03024).
Acknowledgments
We are grateful to XZ (Spine and Osteopathy Ward, The First Affiliated Hospital of Guangxi Medical University) for his kindly assistance in all stages of the present study.
Conflict of interest
The authors declare that the research was conducted in the absence of any commercial or financial relationships that could be construed as a potential conflict of interest.
Publisher’s note
All claims expressed in this article are solely those of the authors and do not necessarily represent those of their affiliated organizations, or those of the publisher, the editors and the reviewers. Any product that may be evaluated in this article, or claim that may be made by its manufacturer, is not guaranteed or endorsed by the publisher.
Supplementary material
The Supplementary Material for this article can be found online at: https://www.frontiersin.org/articles/10.3389/fendo.2023.1196269/full#supplementary-material
References
1. Wright NC, Saag KG, Dawson-Hughes B, Khosla S, Siris ES. The impact of the new National Bone Health Alliance (NBHA) diagnostic criteria on the prevalence of osteoporosis in the USA. Osteoporos Int (2017) 28(4):1225–32. doi: 10.1007/s00198-016-3865-3
2. Clynes MA, Westbury LD, Dennison EM, Kanis JA, Javaid MK, Harvey NC, et al. Bone densitometry worldwide: a global survey by the ISCD and IOF. Osteoporos Int (2020) 31(9):1779–86. doi: 10.1007/s00198-020-05435-8
3. Guzon-Illescas O, Perez Fernandez E, Crespi Villarias N, Quiros Donate FJ, Pena M, Alonso-Blas C, et al. Mortality after osteoporotic hip fracture: incidence, trends, and associated factors. J Orthop Surg Res (2019) 14(1):203. doi: 10.1186/s13018-019-1226-6
4. Yu F, Xia W. The epidemiology of osteoporosis, associated fragility fractures, and management gap in China. Arch Osteoporos (2019) 14(1):32. doi: 10.1007/s11657-018-0549-y
5. Lochmuller EM, Muller R, Kuhn V, Lill CA, Eckstein F. Can novel clinical densitometric techniques replace or improve DXA in predicting bone strength in osteoporosis at the hip and other skeletal sites? J Bone Miner Res (2003) 18(5):906–12. doi: 10.1359/jbmr.2003.18.5.906
6. Trajanoska K, Rivadeneira F. The genetic architecture of osteoporosis and fracture risk. Bone (2019) 126:2–10. doi: 10.1016/j.bone.2019.04.005
7. Yang TL, Shen H, Liu A, Dong SS, Zhang L, Deng FY, et al. A road map for understanding molecular and genetic determinants of osteoporosis. Nat Rev Endocrinol (2020) 16(2):91–103. doi: 10.1038/s41574-019-0282-7
8. Pietschmann P, Butylina M, Kerschan-Schindl K, Sipos W. Mechanisms of systemic osteoporosis in rheumatoid arthritis. Int J Mol Sci (2022) 23(15):8407. doi: 10.3390/ijms23158740
9. Schett G, David JP. The multiple faces of autoimmune-mediated bone loss. Nat Rev Endocrinol (2010) 6(12):698–706. doi: 10.1038/nrendo.2010.190
10. Park YJ, Yoo SA, Kim M, Kim WU. The role of calcium-calcineurin-NFAT signaling pathway in health and autoimmune diseases. Front Immunol (2020) 11:195. doi: 10.3389/fimmu.2020.00195
11. Trajanoska K, Rivadeneira F. Using mendelian randomization to decipher mechanisms of bone disease. Curr Osteoporos Rep (2018) 16(5):531–40. doi: 10.1007/s11914-018-0467-3
12. Burgess S, Dudbridge F, Thompson SG. Combining information on multiple instrumental variables in Mendelian randomization: comparison of allele score and summarized data methods. Stat Med (2016) 35(11):1880–906. doi: 10.1002/sim.6835
13. Dalbeth N, Topless R, Flynn T, Cadzow M, Bolland MJ, Merriman TR. Mendelian randomization analysis to examine for a causal effect of urate on bone mineral density. J Bone Miner Res (2015) 30(6):985–91. doi: 10.1002/jbmr.2434
14. Evans DM, Davey Smith G. Mendelian randomization: new applications in the coming age of hypothesis-free causality. Annu Rev Genomics Hum Genet (2015) 16:327–50. doi: 10.1146/annurev-genom-090314-050016
15. Xu Q, Ni JJ, Han BX, Yan SS, Wei XT, Feng GJ, et al. Causal relationship between gut microbiota and autoimmune diseases: A two-sample mendelian randomization study. Front Immunol (2021) 12:746998. doi: 10.3389/fimmu.2021.746998
16. Alipour P, Senkevich K, Ross JP, Spiegelman D, Manousaki D, Dion PA, et al. Investigation of the causal relationship between ALS and autoimmune disorders: a Mendelian randomization study. BMC Med (2022) 20(1):382. doi: 10.1186/s12916-022-02578-9
17. VanderWeele TJ, Tchetgen Tchetgen EJ, Cornelis M, Kraft P. Methodological challenges in mendelian randomization. Epidemiology (2014) 25(3):427–35. doi: 10.1097/EDE.0000000000000081
18. Skrivankova VW, Richmond RC, Woolf BAR, Yarmolinsky J, Davies NM, Swanson SA, et al. Strengthening the reporting of observational studies in epidemiology using mendelian randomization: the STROBE-MR statement. JAMA (2021) 326(16):1614–21. doi: 10.1001/jama.2021.18236
19. Garcia-Rodriguez JF, Alvarez-Diaz H, Lorenzo-Garcia MV, Marino-Callejo A, Fernandez-Rial A, Sesma-Sanchez P. Extrapulmonary tuberculosis: epidemiology and risk factors. Enferm Infecc Microbiol Clin (2011) 29(7):502–9. doi: 10.1016/j.eimc.2011.03.005
20. Medina-Gomez C, Kemp JP, Trajanoska K, Luan J, Chesi A, Ahluwalia TS, et al. Life-course genome-wide association study meta-analysis of total body BMD and assessment of age-specific effects. Am J Hum Genet (2018) 102(1):88–102. doi: 10.1016/j.ajhg.2017.12.005
21. Zheng HF, Forgetta V, Hsu YH, Estrada K, Rosello-Diez A, Leo PJ, et al. Whole-genome sequencing identifies EN1 as a determinant of bone density and fracture. Nature (2015) 526(7571):112–7. doi: 10.1038/nature14878
22. Kemp JP, Morris JA, Medina-Gomez C, Forgetta V, Warrington NM, Youlten SE, et al. Identification of 153 new loci associated with heel bone mineral density and functional involvement of GPC6 in osteoporosis. Nat Genet (2017) 49(10):1468–75. doi: 10.1038/ng.3949
23. Hadji P, Colli E, Regidor PA. Bone health in estrogen-free contraception. Osteoporos Int (2019) 30(12):2391–400. doi: 10.1007/s00198-019-05103-6
24. Khanna K, Sabharwal S. Spinal tuberculosis: a comprehensive review for the modern spine surgeon. Spine J (2019) 19(11):1858–70. doi: 10.1016/j.spinee.2019.05.002
25. Weiner J 3rd, Maertzdorf J, Sutherland JS, Duffy FJ, Thompson E, Suliman S, et al. Metabolite changes in blood predict the onset of tuberculosis. Nat Commun (2018) 9(1):5208. doi: 10.1038/s41467-018-07635-7
26. Garg RK, Raut T, Malhotra HS, Parihar A, Goel M, Jain A, et al. Evaluation of prognostic factors in medically treated patients of spinal tuberculosis. Rheumatol Int (2013) 33(12):3009–15. doi: 10.1007/s00296-013-2841-x
27. Hemani G, Zheng J, Elsworth B, Wade KH, Haberland V, Baird D, et al. The MR-Base platform supports systematic causal inference across the human phenome. Elife (2018) 7:e34408. doi: 10.7554/eLife.34408
28. Genomes Project C, Auton A, Brooks LD, Durbin RM, Garrison EP, Kang HM, et al. A global reference for human genetic variation. Nature (2015) 526(7571):68–74. doi: 10.1038/nature15393
29. Mensah-Kane J, Schmidt AF, Hingorani AD, Finan C, Chen Y, van Duijvenboden S, et al. No clinically relevant effect of heart rate increase and heart rate recovery during exercise on cardiovascular Disease: A Mendelian Randomization Analysis. Front Genet (2021) 12:569323. doi: 10.3389/fgene.2021.569323
30. Burgess S, Davey Smith G, Davies NM, Dudbridge F, Gill D, Glymour MM, et al. Guidelines for performing Mendelian randomization investigations. Wellcome Open Res (2019) 4:186. doi: 10.12688/wellcomeopenres.15555.1
31. Burgess S, Butterworth A, Thompson SG. Mendelian randomization analysis with multiple genetic variants using summarized data. Genet Epidemiol (2013) 37(7):658–65. doi: 10.1002/gepi.21758
32. Bowden J, Davey Smith G, Haycock PC, Burgess S. Consistent Estimation in Mendelian Randomization with Some Invalid Instruments Using a Weighted Median Estimator. Genet Epidemiol (2016) 40(4):304–14. doi: 10.1002/gepi.21965
33. Bowden J, Davey Smith G, Burgess S. Mendelian randomization with invalid instruments: effect estimation and bias detection through Egger regression. Int J Epidemiol (2015) 44(2):512–25. doi: 10.1093/ije/dyv080
34. Greco MF, Minelli C, Sheehan NA, Thompson JR. Detecting pleiotropy in Mendelian randomisation studies with summary data and a continuous outcome. Stat Med (2015) 34(21):2926–40. doi: 10.1002/sim.6522
35. Verbanck M, Chen CY, Neale B, Do R. Detection of widespread horizontal pleiotropy in causal relationships inferred from Mendelian randomization between complex traits and diseases. Nat Genet (2018) 50(5):693–8. doi: 10.1038/s41588-018-0099-7
36. Maas F, Spoorenberg A, van der Slik BPG, van der Veer E, Brouwer E, Bootsma H, et al. Clinical Risk Factors for the Presence and Development of Vertebral fractures in patients with ankylosing spondylitis. Arthritis Care Res (Hoboken) (2017) 69(5):694–702. doi: 10.1002/acr.22980
37. Rees F, Doherty M, Grainge MJ, Lanyon P, Zhang W. The worldwide incidence and prevalence of systemic lupus erythematosus: a systematic review of epidemiological studies. Rheumatol (Oxford) (2017) 56(11):1945–61. doi: 10.1093/rheumatology/kex260
38. Adami G, Fassio A, Rossini M, Caimmi C, Giollo A, Orsolini G, et al. Osteoporosis in rheumatic diseases. Int J Mol Sci (2019) 20(23):5867. doi: 10.3390/ijms20235867
39. Coury F, Peyruchaud O, Machuca-Gayet I. Osteoimmunology of bone loss in inflammatory rheumatic diseases. Front Immunol (2019) 10:679. doi: 10.3389/fimmu.2019.00679
40. Guler-Yuksel M, Bijsterbosch J, Goekoop-Ruiterman YP, de Vries-Bouwstra JK, Hulsmans HM, de Beus WM, et al. Changes in bone mineral density in patients with recent onset, active rheumatoid arthritis. Ann Rheum Dis (2008) 67(6):823–8. doi: 10.1136/ard.2007.073817
41. Guler-Yuksel M, Allaart CF, Goekoop-Ruiterman YP, de Vries-Bouwstra JK, van Groenendael JH, Mallee C, et al. Changes in hand and generalised bone mineral density in patients with recent-onset rheumatoid arthritis. Ann Rheum Dis (2009) 68(3):330–6. doi: 10.1136/ard.2007.086348
42. Habib GS, Haj S. Bone mineral density in patients with early rheumatoid arthritis treated with corticosteroids. Clin Rheumatol (2005) 24(2):129–33. doi: 10.1007/s10067-004-0989-1
43. Napoli N, Chandran M, Pierroz DD, Abrahamsen B, Schwartz AV, Ferrari SL, et al. Mechanisms of diabetes mellitus-induced bone fragility. Nat Rev Endocrinol (2017) 13(4):208–19. doi: 10.1038/nrendo.2016.153
44. Ashai S, Harvey NC. Rheumatoid arthritis and bone health. Clin Med (Lond) (2020) 20(6):565–7. doi: 10.7861/clinmed.20.6.rabh
45. Khor B, Gardet A, Xavier RJ. Genetics and pathogenesis of inflammatory bowel disease. Nature (2011) 474(7351):307–17. doi: 10.1038/nature10209
46. Jahnsen J, Falch JA, Mowinckel P, Aadland E. Bone mineral density in patients with inflammatory bowel disease: a population-based prospective two-year follow-up study. Scand J Gastroenterol (2004) 39(2):145–53. doi: 10.1080/00365520310007873
47. Sakellariou GT, Moschos J, Berberidis C, Mpoumponaris A, Kadis S, Molyvas E, et al. Bone density in young males with recently diagnosed inflammatory bowel disease. Joint Bone Spine (2006) 73(6):725–8. doi: 10.1016/j.jbspin.2006.01.017
48. Bernstein CN, Seeger LL, Sayre JW, Anton PA, Artinian L, Shanahan F. Decreased bone density in inflammatory bowel disease is related to corticosteroid use and not disease diagnosis. J Bone Miner Res (1995) 10(2):250–6. doi: 10.1002/jbmr.5650100211
49. Szafors P, Che H, Barnetche T, Morel J, Gaujoux-Viala C, Combe B, et al. Risk of fracture and low bone mineral density in adults with inflammatory bowel diseases. A systematic literature review with meta-analysis. Osteoporos Int (2018) 29(11):2389–97. doi: 10.1007/s00198-018-4586-6
50. Wu F, Huang Y, Hu J, Shao Z. Mendelian randomization study of inflammatory bowel disease and bone mineral density. BMC Med (2020) 18(1):312. doi: 10.1186/s12916-020-01778-5
51. Gebre AK, Prince RL, Schousboe JT, Kiel DP, Thompson PL, Zhu K, et al. Calcaneal quantitative ultrasound is associated with all-cause and cardiovascular disease mortality independent of hip bone mineral density. Osteoporos Int (2022) 33(7):1557–67. doi: 10.1007/s00198-022-06317-x
52. Blank RD. Practical management of fracture risk among peri- and postmenopausal women. Fertil Steril (2019) 112(5):782–90. doi: 10.1016/j.fertnstert.2019.09.038
53. Crowe CS, Massenburg BB, Morrison SD, Chang J, Friedrich JB, Abady GG, et al. Global trends of hand and wrist trauma: a systematic analysis of fracture and digit amputation using the Global Burden of Disease 2017 Study. Inj Prev (2020) 26(Supp 1):i115–i24. doi: 10.1136/injuryprev-2019-043495
54. Griffin D, Parsons N, Shaw E, Kulikov Y, Hutchinson C, Thorogood M, et al. Operative versus non-operative treatment for closed, displaced, intra-articular fractures of the calcaneus: randomised controlled trial. BMJ (2014) 349:g4483. doi: 10.1136/bmj.g4483
55. Galluzzo M, Greco F, Pietragalla M, De Renzis A, Carbone M, Zappia M, et al. Calcaneal fractures: radiological and CT evaluation and classification systems. Acta BioMed (2018) 89(1-S):138–50. doi: 10.23750/abm.v89i1-S.7017
56. Bridgman SA, Dunn KM, McBride DJ, Richards PJ. Interventions for treating calcaneal fractures. Cochrane Database Syst Rev (2000) 2):CD001161. doi: 10.1002/14651858.CD001161
57. Hedlund LJ, Maki DD, Griffiths HJ. Calcaneal fractures in diabetic patients. J Diabetes Complications (1998) 12(2):81–7. doi: 10.1016/S1056-8727(97)00052-4
58. Fritz NE, Eloyan A, Baynes M, Newsome SD, Calabresi PA, Zackowski KM. Distinguishing among multiple sclerosis fallers, near-fallers and non-fallers. Mult Scler Relat Disord (2018) 19:99–104. doi: 10.1016/j.msard.2017.11.019
59. Kawabata K, Matsumoto T, Kasai T, Chang SH, Hirose J, Tanaka S. Association between fall history and performance-based physical function and postural sway in patients with rheumatoid arthritis. Mod Rheumatol (2021) 31(2):373–9. doi: 10.1080/14397595.2020.1731134
60. Brenton-Rule A, Dalbeth N, Menz HB, Bassett S, Rome K. Foot and ankle characteristics associated with falls in adults with established rheumatoid arthritis: a cross-sectional study. BMC Musculoskelet Disord (2016) 17:22. doi: 10.1186/s12891-016-0888-z
61. Jin S, Hsieh E, Peng L, Yu C, Wang Y, Wu C, et al. Incidence of fractures among patients with rheumatoid arthritis: a systematic review and meta-analysis. Osteoporos Int (2018) 29(6):1263–75. doi: 10.1007/s00198-018-4473-1
Keywords: two-sample Mendelian randomization, osteoporosis, bone mineral density, fall risk, fracture risk
Citation: Wu S, Ye Z, Yan Y, Zhan X, Ren L, Zhou C, Chen T, Yao Y, Zhu J, Wu S, Ma F, Liu L, Fan B and Liu C (2023) The causal relationship between autoimmune diseases and osteoporosis: a study based on Mendelian randomization. Front. Endocrinol. 14:1196269. doi: 10.3389/fendo.2023.1196269
Received: 29 March 2023; Accepted: 17 July 2023;
Published: 29 August 2023.
Edited by:
Daniela Merlotti, University of Siena, ItalyReviewed by:
Mischa Lundberg, Region Hovedstad Psychiatry, DenmarkNurhasan Agung Prabowo, Sebelas Maret University, Indonesia
Copyright © 2023 Wu, Ye, Yan, Zhan, Ren, Zhou, Chen, Yao, Zhu, Wu, Ma, Liu, Fan and Liu. This is an open-access article distributed under the terms of the Creative Commons Attribution License (CC BY). The use, distribution or reproduction in other forums is permitted, provided the original author(s) and the copyright owner(s) are credited and that the original publication in this journal is cited, in accordance with accepted academic practice. No use, distribution or reproduction is permitted which does not comply with these terms.
*Correspondence: Chong Liu, liuchong@stu.gxmu.edu.cn
†These authors have contributed equally to this work