- 1Institute of Rheumatology, Prague, Czechia
- 2Department of Rheumatology, First Faculty of Medicine, Charles University in Prague, Prague, Czechia
- 3Department of Obstetrics and Gynecology, First Faculty of Medicine, Charles University in Prague, Prague, Czechia
- 4General University Hospital in Prague, Prague, Czechia
Objective: Osteoporosis is associated with an impaired balance between bone resorption and formation, which in turn leads to bone loss and fractures. Many recent studies have underlined the regulatory role of microRNAs (miRNAs) in bone remodeling processes and their potential as biomarkers of osteoporosis. The purpose of this study was to prospectively examine the association of circulating miRNAs and bone biomarkers with estrogen status in women before and after oophorectomy, as well as in oophorectomized women on estrogen therapy.
Methods: In this prospective study, we included 11 women before oophorectomy and hysterectomy and at 201 ± 24 days after the surgery. Another 11 women were evaluated 508 ± 127 days after oophorectomy and hysterectomy and after an additional 203 ± 71 days of estradiol treatment. Serum miRNAs were profiled by sequencing. Estrogen status and biomarkers of bone metabolism were quantified. Bone mineral density was assessed in the lumbar spine.
Results: Our analysis revealed 17 miRNAs associated with estrogen levels. Of those miRNAs that were upregulated with estrogen deficiency and downregulated after estrogen therapy, miR-422a correlated with serum beta-carboxy-terminal type I collagen crosslinks (β-CTX) and procollagen 1 N-terminal propeptide (P1NP); and miR-1278 correlated with serum β-CTX, P1NP, osteocalcin, sclerostin, and Dickkopf-1(Dkk1). In contrast, we found an inverse association of miR-24-1-5p with estrogen status and a negative correlation with serum β-CTX, P1NP, osteoprotegerin, and sclerostin levels.
Conclusion: The reported miRNAs associated with estrogen status and bone metabolism could be potential biomarkers of bone pathophysiology and would facilitate studies on the prevention of postmenopausal osteoporosis. Our findings require validation in an extended cohort.
Introduction
Osteoporosis is a skeletal disease characterized by low bone mass and microarchitectural deterioration, which are related to unbalanced bone resorption and bone formation (1), leading to bone fragility and susceptibility to fracture (2). The consequences of osteoporosis substantially increase the consumption of medical and economic resources worldwide (3). Until now, many expression profiling studies have revealed that bone remodeling processes are well-tuned at the transcriptional level and controlled by noncoding RNAs, especially long non-coding RNAs and microRNAs (miRNAs) (4–6).
MiRNAs are short RNAs of typically 18-22 nucleotides that operate as post-transcriptional regulators of protein-coding genes and the non-coding genome (7). They regulate many developmental and functional pathways, and their aberrant expression has been associated with various disorders (8), including osteoporosis (7, 9). In particular, several miRNAs with distinguishable expression profiles have been identified in bone samples from patients with osteoporosis and those with low impact fractures in most studies (10–15). Generally, during the bone remodeling process, miRNAs regulate differentiation of osteoblast and osteoclast and bone formation by targeting the regulators of osteogenesis or osteoclastogenesis, namely, transcription factors and signaling pathways (7, 16, 17). MiRNAs can escape from tissues into the bloodstream and become circulating miRNAs, providing additional information related to bone metabolism (7). The existing literature has mainly assessed the profiles of circulating miRNAs in patients with established osteoporosis and low-impact fractures. Recent research has drawn attention to the association of circulating miRNAs with bone remodeling in menstrual cessation (18). In the early postmenopausal period, the bone mineral density is not decreased but the bone resorption rate excessively exceeds new bone formation, posing a possible osteoporosis risk (19). The purpose of this study was to prospectively examine the association of circulating miRNAs and bone biomarkers with estrogen status in women before and after oophorectomy, as well as in oophorectomized women on estrogen therapy.
Patients and Methods
Patients
Between July 2018 and May 2019, 22 women who had been consecutively referred for bone status assessment through the Department of Obstetrics and Gynecology, General University Hospital in Prague, and had hysterectomy and bilateral oophorectomy before menopause were included in the present study. Surgery was indicated for benign gynecological diseases, including leiomyoma (14), metrorrhagia (3), breast cancer gene mutation (3), dysplasia of the cervix (1), and benign ovarian cyst (1). Of these subjects, 11 women were evaluated 18 ± 10 days before oophorectomy and hysterectomy and 201 ± 24 days after the surgery. None of these women received hormone therapy after surgery. The other 11 women who had previously undergone oophorectomy and hysterectomy were evaluated at baseline 508 ± 127 days after the surgery. Hormone treatment was initiated by their gynecologist. A check-up was performed after 203 ± 71 days of oral hormonal treatment, corresponding to 1 mg of estradiol per day.
Complete medical histories and dietary questionnaires were obtained from all subjects. No subject had bone- or calcium-related metabolic disease or received medications that are known to affect bone or calcium metabolism. No woman had a history of alcohol abuse, diabetes mellitus, active neoplastic disease, liver disease, known endocrine and rheumatologic disease, immunosuppressive treatment, treatment with corticosteroids, aromatase inhibitors, anti-osteoporotic drugs, anticonvulsants, or a history of fragility fractures or had previously received hormone replacement therapy. Subjects were advised to maintain their usual physical activity and dietary pattern throughout the study.
The study was approved by the Institutional Review Board at the Institute of Rheumatology, Prague, Czech Republic. All participants signed written informed consent forms to participate and agreed to DXA and blood tests. All study procedures were carried out in compliance with the laws and regulations governing the use of human subjects (Declaration of Helsinki) (20).
Blood Collection
Venous blood samples were obtained after an overnight fast from each subject at baseline and follow-up visits for laboratory analyses. To separate the serum, whole blood was collected into commercially available collection tubes. After one hour of clotting, the blood was centrifuged at 2000xg for 20 minutes. Serum (supernatant) was transferred into a new tube and frozen at -70°C until further processing.
MiRNA Isolation
MiRNAs were extracted from 200 µL of blood serum using NucleoSpin miRNA Plasma (Macherey-Nagel) according to the manufacturer’s protocol. Isolated miRNAs were not quantified since concentrations were below the detection limits of the NanoDrop 2000. Follow-up procedures were conducted with undiluted samples.
Massively Parallel Sequencing
Library Preparation
Libraries for massively parallel sequencing (MPS) were prepared from 10.5 µL of isolated miRNAs using Kit v3 (Bioo Scientific) according to the manufacturer’s protocol. Fragments after the amplification step were analyzed using a Fragment Analyzer (Advanced Analytical), and fragments of the miRNA library (145 bp) were quantified. Samples were pooled in equal concentrations of miRNA library fragments, which were isolated using the Pippin Prep system with 3% agarose (Sage Science) before sequencing. The isolated fragments were quantified using a Qubit 2.0 fluorometer (Thermo Fisher Scientific) and were used for sequencing on a NextSeq 500 (Illumina) according to the manufacturer’s protocol.
Bioinformatics Analyses
Adaptor sequences in MPS data were identified and removed with Cutadapt (v2.5) software (21). Only high-quality reads with a length between 16 and 28 bp after adapter trimming were retained as potential miRNA reads. The quality of both raw and processed reads was evaluated using FastQC software (22).
Count-based miRNA expression data were generated by the Chimira tool (23) from FASTQ files. All sequences were adapter trimmed and mapped against miRBase v22 (24), allowing up to two mismatches per sequence. All samples were evaluated for differential expression using DESeq2 (25). Further analyses were performed using R/Bioconductor packages. Raw data and annotated sequences of the small RNA libraries were uploaded to the GEO database.
DESeq2 computed the normalized miRNA-read count, dispersion, and base mean (the average of the normalized count values, divided by the size factors, taken over all samples). The dispersion can be understood as a squared coefficient of variation and the mean dispersion value of 26.04 represents the coefficient of variation of 4.49. miRNAs with a base mean < 10 were indistinguishable from the sampling noise and were filtered out of the dataset.
Bone Densitometry
The areal bone mineral density (aBMD) of the lumbar spine (LS-BMD) was evaluated using dual-energy X-ray absorptiometry (DXA) (GE Healthcare Lunar software version 14.1) and expressed in grams/cm2 and T-score. The T-score was calculated using the National Health and Nutrition Examination Survey (NHANES) of young women as a reference. Quality control assurance measurements were performed following the manufacturer’s recommendations. The short-term in vivo precision error for the lumbar spine (L1–L4) was 0.7%; the long-term in vivo precision error was 0.31%. Trained examiners with extensive experience conducted the measurements.
Biochemical Analysis
The concentrations of total serum calcium, phosphate, glucose, total alkaline phosphatase, γ-glutamyltransferase (GGT), thyroid-stimulating hormone (TSH), 1-84 amino acid fragment of parathyroid hormone (intact PTH), 25(OH)D, beta-carboxy-terminal type I collagen crosslinks (β-CTX), procollagen 1 N-terminal propeptide (P1NP), osteocalcin, estradiol, and FSH were determined using the Beckman Coulter AU 680 (Beckman Coulter, USA), Roche cobas e601 (Roche, Switzerland), and Liaison XL (Diasorin, Italy) analytical systems. The plasma estradiol measuring range was 18.4-11,000 pmol/l with intra- and interassay CV < 12%. The plasma FSH measuring range was 0.1-200 IU/l with intra- and interassay CV < 6%. The serum β-CTX measuring range was 0.01-6 µg/l with intra- and interassay CV < 6%. The serum PINP measuring range was 5-1200 µg/l with intra- and interassay CV < 6%. The estimated glomerular filtration rate (eGFR) was calculated (26). Serum sclerostin was assessed using the Bioactive Sclerostin ELISA (Biomedica, Austria), and the measuring range was 10-320 pmol/l with intra- and interassay CV < 5%. Serum Dickkopf-1 (DKK1) was analyzed using the DKK-1 ELISA (Biomedica, Austria), and the measuring range was 10-160 pmol/l with intra- and interassay CV < 3%. Serum osteoprotegerin was measured using the Human Osteoprotegerin ELISA (Biovendor, Czech Republic), and the measuring range was 1.5-60 pmol/l with intra- and interassay CV < 7%.
Biostatistical Analyses
Characteristics of the population were computed using SigmaPlot version 14.0, Systat Software, San Jose, CA, USA. The Kolmogorov–Smirnov test was applied to assess the normality of the data. Data with a normal distribution are presented as the mean ± SD, while nonparametric data are presented as the median and quartiles. The paired t-test was used to compare biochemical variables, depending on the normality of the variables. Univariate analysis with Pearson correlation was used. Statistical significance was defined as a p-value < 0.05.
DESeq2 algorithm provided a matrix of normalized read counts, which were analyzed with RStudio software and relevant packages (27, 28). Since miRNAs had normalized read count equal to zero in several samples, we employed glmmTMB R package (28), in which we can specify fixed and random effects models for the conditional and zero-inflated components of the model with negative binomial assumption. Using this R extension, we fitted a GLMM-NB, using the normalized read count of miRNAs as the outcome variable, with fixed effects of the level of estrogen (or the biomarker of bone metabolism) and follicle-stimulating hormone (FSH) as a confounding variable. We also fitted a random-effects structure, which includes a random intercept for patient ID. To test differential miRNA expression between patients with optimal and low estrogen levels (estrogen status) or the association with the biomarkers of bone remodelling (adjusted with FSH level and a random intercept for patient ID), the following model was used:
where i - the miRNA; j – patients ID; β – regression coefficient; γ – random parameter; and ϵ – error. The effect of estrogen level was represented as the incidence rate ratio (IRR), which means that the number of expected observations on the miRNAs’ count changes by the value of IRR if the estrogen level changes from optimal to low. The association of the bone modelling biomarkers is represented as the slope, which is the change of normalized read counts if the value of the biomarkers changes by one unit. The adjusted p-value was calculated using Benjamini-Hochberg (BH) method (29) and indicated in Supplementary Tables.
Results
Demographic and Clinical Characteristics
At baseline, no differences were observed in age, body mass index (BMI), serum 1-84 amino acid fragment of parathyroid hormone (intact PTH), creatinine, glucose, thyroid stimulating hormone (TSH), or creatinine clearance between women before and after oophorectomy (Groups A and C, Table 1). At follow-up 201 days after oophorectomy (Group B), lumbar spine bone mineral density (LS BMD) and serum estradiol decreased significantly, while serum follicle-stimulating hormone (FSH), type 1 collagen crosslinked C-telopeptide (β-CTX), intact amino-terminal propeptide of type I procollagen (P1NP), alkaline phosphatase, osteocalcin, sclerostin, calcium, and phosphate significantly increased compared to values before oophorectomy (Group A). Similar differences were observed between the variables before oophorectomy (Group A) and 508 days after oophorectomy (Group C), except for sclerostin. In women on oral hormonal therapy for 203 days (from 508 days, Group C, to 711 days after oophorectomy, Group D), LS-BMD and serum estradiol increased significantly, while serum FSH, β-CTX, P1NP, alkaline phosphatase, osteocalcin, sclerostin, osteoprotegerin, calcium, phosphate, and PTH decreased. Dkk1and OPG serum levels did not correlate with bone remodeling markers (β-CTX and P1NP), while levels of β-CTX and P1NP were highly correlated (Table 2; Figure 1).
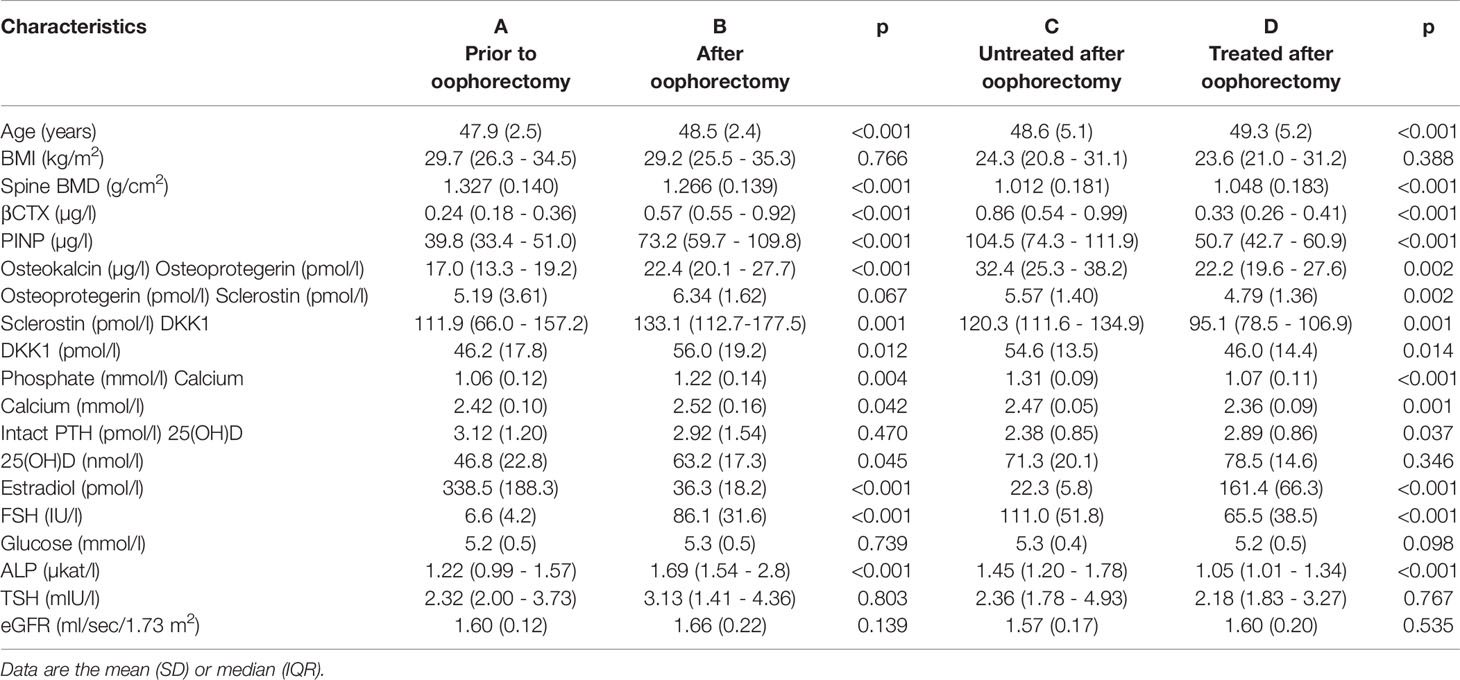
Table 1 Characteristics of 11 untreated women prior to oophorectomy (A) and 201 days after oophorectomy (B) and 11 untreated women 508 days after oophorectomy (C) and then after 203 days of estradiol treatment (D).
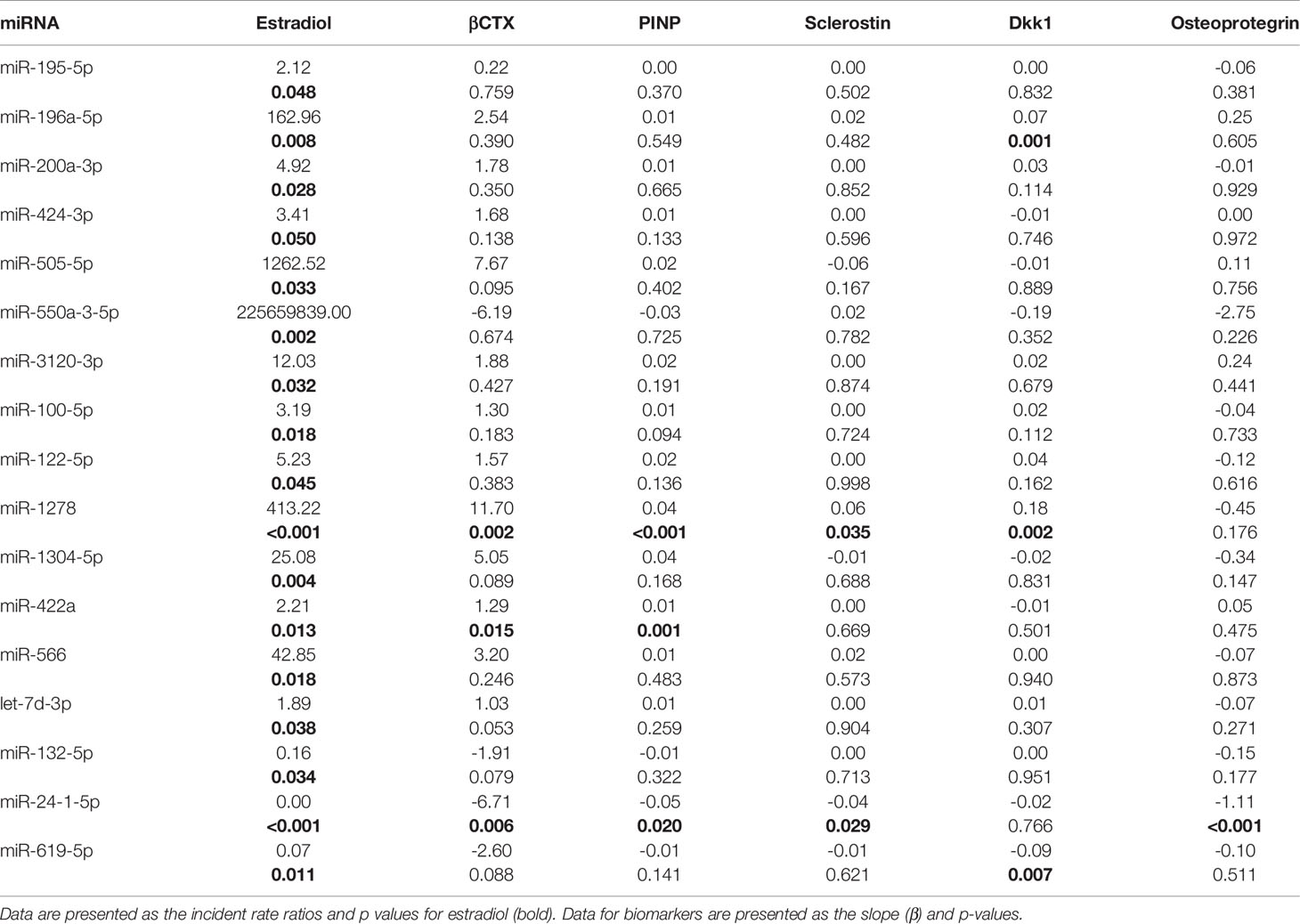
Table 2 Association of changes in 17 miRNAs with disparate concentrations between sufficient and low estrogen levels in serum with biomarkers in 11 untreated women before oophorectomy and 201 days after, and in 11 women initially untreated for 508 days and then treated with estradiol for 203 days.
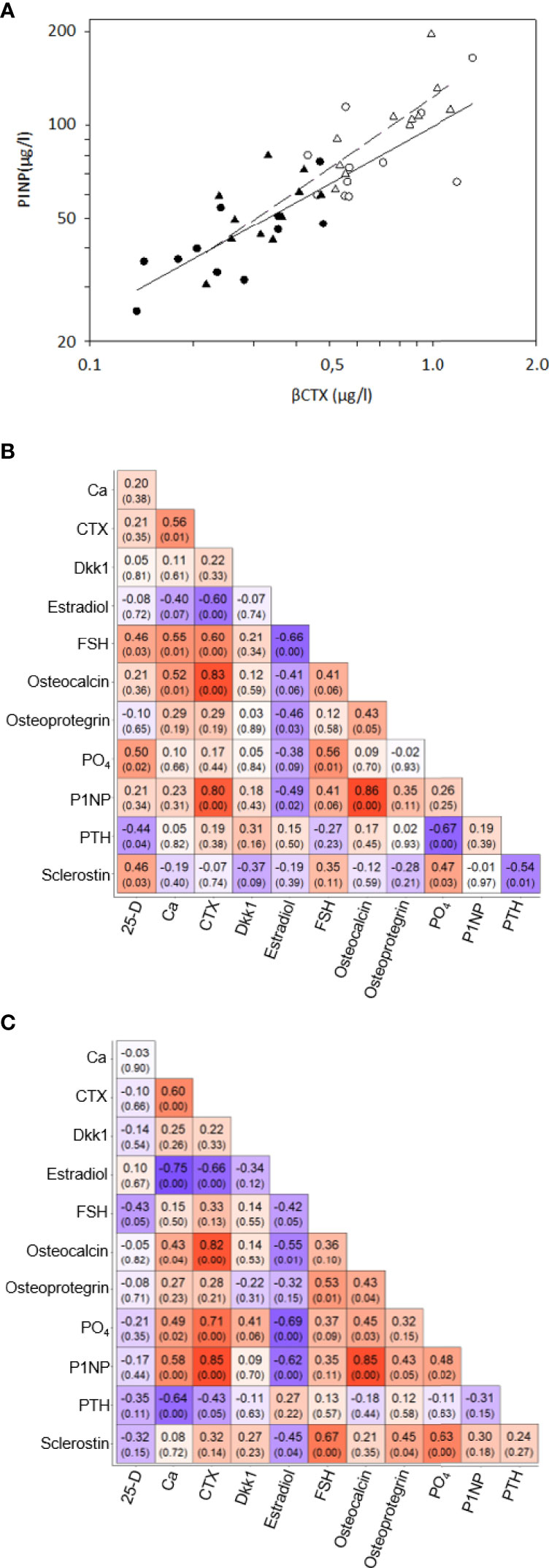
Figure 1 Correlations of bone remodeling biomarkers – (A) Correlations between serum βCTX and P1NP in 11 women before oophorectomy, Group A (•) and untreated after surgery, Group B (o) (solid line), and in 11 oophorectomized women untreated, Group C (Δ) and treated with estradiol, Group D (▲). (B) Correlation matrix of selected the bone remodelling biomarkers in the Group A and B. The matrix included Pearson’s correlation coefficients and p-value in the bracket below. The color indicates the strength and direction of the coefficient. (C) Correlation matrix of selected the bone remodelling biomarkers in the Group C and D. The matrix included Pearson’s correlation coefficients and p-value in the bracket below. The color indicates the strength and direction of the coefficient.
Of the 48 sequenced samples, 22 samples were paired up as having sufficient and low levels of estrogen. The four remaining samples were additional measurements to four paired samples and were thus discarded.
MiRNome Profiling in Patient Serum – Sequencing Study
Sequencing revealed a median coverage of over 10 million unprocessed reads per sample, but after trimming and aligning to miRBase v22, we found that the median was 4.9 million reads per sample with a balanced distribution (SD = 0.043).
After alignment, we found 1305 unique miRNA sequences. Since low-read count miRNAs with high dispersion might be indistinguishable from sampling noise and increase the false-positive rate, we filtered out all miRNAs with a base mean of > 10 and found 439 miRNAs.
Since patients were paired up, we employed generalized linear mixed-effects modeling with the negative binomial assumption (GLMM-NB) and patients as the random effect. The first analysis focused on changes in miRNA concentrations in serum with sufficient and low levels of estrogen, and we found 17 miRNAs with p < 0.05 and a difference in fold change of > 50%. To affirm their connection to osteoporosis, we examined their relationship to lumbar spine BMD, biomarkers and hormones associated with bone metabolism, and biomarkers of low-grade inflammation. Significant associations are given in Table 2 and Tables S1, S2 in detail.
The concentrations of 14 miRNAs showed an inverse association with changes in estrogen status (Table 2). Serum concentrations of miR-422a were positively associated with serum β-CTX and P1NP levels (Figure 2). The amount of miR-1278 was positively associated with serum β-CTX, P1NP, sclerostin, osteocalcin, and Dkk1 levels. Increased miR-422a levels were associated with increased serum β-CTX and P1NP levels (Table 2).
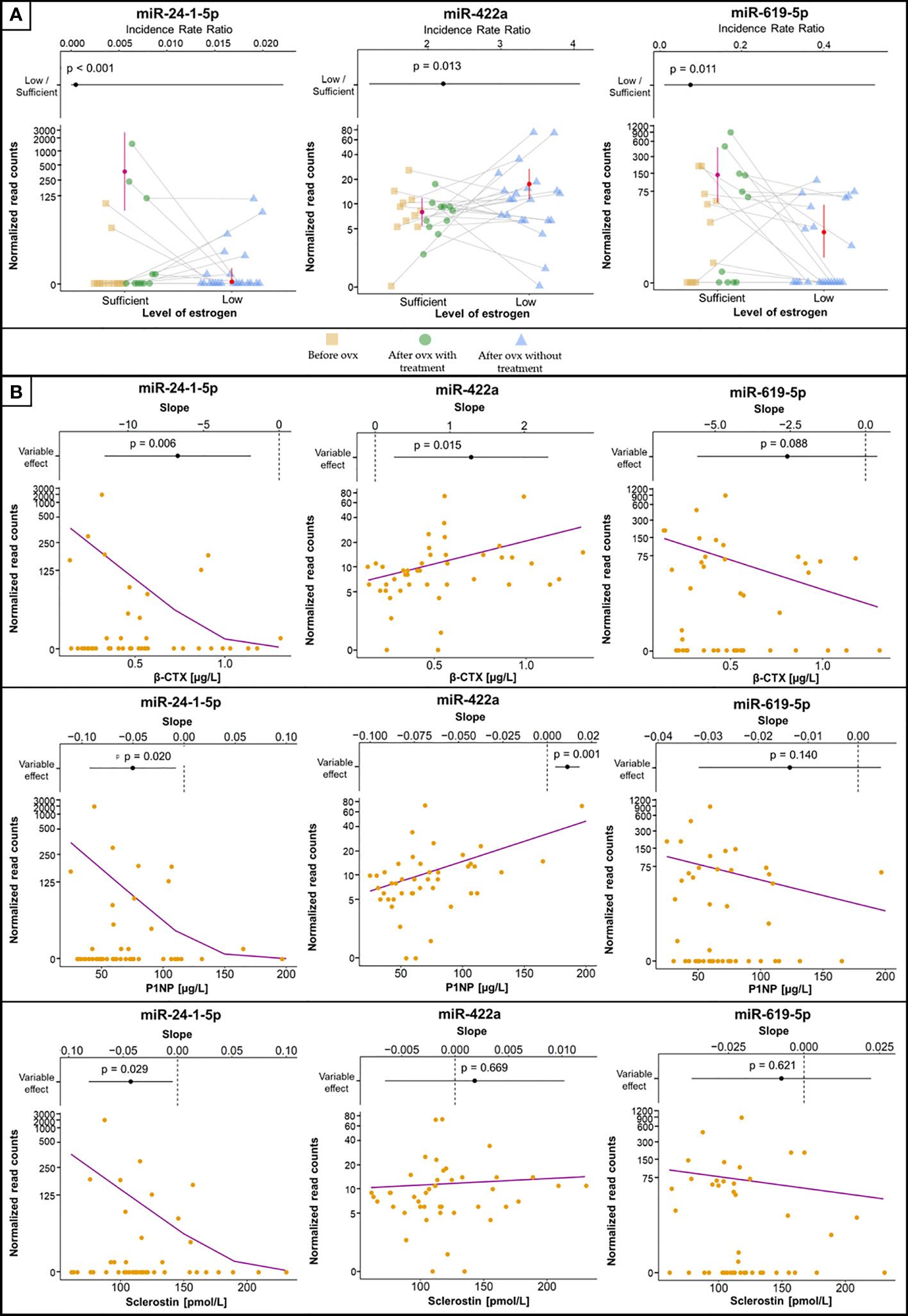
Figure 2 Selected miRNAs associated with estrogen status and biomarkers of bone metabolism or inflammation. (A) MiRNA concentrations between groups, with error bars representing 95% confidence intervals and estimated means (filled circles). Samples from the same patient are connected with grey lines. The plots at the top are visualizations of the 95% confidence intervals with IRR (filled circles), where values > 1 indicate increased concentrations in samples with low estrogen levels and vice versa. The vertical dashed line indicates IRR equal to 1 (= no difference). p values denoting the statistical significance between the groups are specified above the slope and were computed using GLMM-NB. (B) The association of selected miRNAs with levels of biomarkers. The violet line is the regression line computed using GLMM-NB. The plots at the top are visualizations of 95% confidence intervals with the slope (β) (filled circles), where a positive value indicates a positive association of the miRNA with the biomarker and vice versa. The vertical dashed line indicates a slope equal to 0 (= no difference). p values denoting the statistical significance of the association are specified above the slope and were computed using GLMM-NB.
In contrast, the levels of miR-132-5p, miR-24-1-5p, and miR-619-5p showed a positive association with estrogen status (Figure 2). Of these, miR-24-1-5p was negatively associated with β-CTX, PINP, osteoprotegerin, and sclerostin levels. The remaining miRNAs were not associated with any biomarker of bone metabolism or inflammation. Furthermore, the associations between miRNA concentrations and lumbar spine BMD were not significant.
MiRNome Profiling in Patient Serum – osteomiR®
An association between osteoporosis and circulating miRNA profiles can be assessed using commercially available kits, such as osteomiR® (TAmiRNA, Austria). This complete kit includes materials for miRNA isolation, reverse transcription, and qRT–PCR with LNA probes. In addition to quality control (QC) probes, this kit screened ten miRNAs connected to osteoporosis. However, in most of our samples, these miRNAs were below the detection limit of qRT–PCR (CT > 30), and the QC probes indicated low concentrations after isolation. We verified these results by additional miRNA isolation from serum and quantification by TaqMan Advanced miRNA assays (Thermo).
All ten miRNAs included in the osteomiR® kit were detected in our miRNA-Seq analysis, and their relation to estrogen levels and biomarkers was assessed using GLMM-NB with patients as a random effect and FSH as a confounding variable. Nonetheless, no miRNA was significantly different between samples with sufficient and low estrogen levels (Table S3), but we found an association of miR-375 with β-CTX and P1NP (Table S4).
Discussion
In this study, we screened the profile of circulating miRNAs in women before and after oophorectomy, as well as in oophorectomized women on estrogen therapy. We identified 17 miRNAs, which were different in sera with either sufficient or low estrogen levels. Of the 14 miRNAs upregulated after oophorectomy and downregulated with estrogen therapy, miR-1278, miR-24-1-5p, and miR-422awere associated with biomarkers of bone metabolism, suggesting their role in the pathogenesis of osteoporosis (11, 30–33).
The expression of miR-422a was previously detected in monocytes and was considered a potential biomarker for postmenopausal osteoporosis (34). We observed a decrease in miR-422a levels after estradiol treatment, and a positive correlation of miR-422a levels with β-CTX and PINP but not with sclerostin levels. These findings support the role of miR-422a expression in bone remodeling associated with estrogen status (35–39).
The linkage of miR-1278 to bone metabolism was not explored previously, but we found associations of miR-1278 with serum levels of bone remodeling biomarkers (β-CTX, P1NP, and osteocalcin). In addition, miR-1278 was positively associated with sclerostin and Dkk1, which are inhibitors of the WNT signaling pathway. These associations are in concordance with previous studies on the association between inhibitors of the WNT signaling pathway and estrogen status (40–44). The Wnt/β-catenin pathway activation enhances bone mass not only by stimulating osteoblastogenesis but, at least to some extent, also by inhibiting osteoclastogenesis as well (44).
In contrast, estrogen deficiency in our patients was associated with decreased serum levels of miR-24-1-5p and increased serum levels of β-CTX, P1NP, and sclerostin compared to those of women with sufficient estrogen status. Similarly, postmenopausal women treated with teriparatide had a significant and inverse correlation of miR-24-3p at 3 months with P1NP at 3 and 12 months and with β-CTX at 12 months (45). However, Seeliger et al. observed an upregulated miR-24-3p concentration in sera and bone tissue of osteoporotic patients (11), while another study did not find any differences in the relative expression of miR-24-3p between healthy, osteoporotic, and sarcopenic postmenopausal women (14). The discrepancy observed among these studies might arise from the quantification of different isoforms of this miRNA.
In this study, levels of β-CTX and P1NP were highly correlated, reflecting the coupling of bone resorption and bone formation (46). The early phase after oophorectomy is characterized by the prevalence of bone resorption over bone formation; following initiation of estrogen treatment, a decrease in markers of bone resorption is later followed by a decrease in markers of bone formation (47). Compared with women before oophorectomy and women treated with estrogen, untreated oophorectomized women showed higher serum β-CTX, PINP, and osteocalcin, but also higher sclerostin, Dkk1, and OPG, and levels. Our sclerostin data correspond with a negative correlation of sclerostin levels with the free estradiol index in postmenopausal women (48), with significantly higher serum sclerostin levels in postmenopausal women than premenopausal women (49), and with a decrease in serum (42, 50) and bone mRNA sclerostin levels (43) after the administration of estrogen. DKK1 expression in bone was enhanced after ovariectomy in mice, and DKK1 antisense oligonucleotide treatment reduced the promoting effect of estrogen deficiency on DKK1 (51). Postmenopausal women with significantly increased serum DKK1 had more significant osteoporosis (51). Osteoprotegerin levels are higher in postmenopausal osteoporotic women, compared with controls (52), and the inverse relationship between serum OPG and serum oestradiol levels was demonstrated in females (53). Serum OPG levels measured after 3 months and 1 year of HT decreased significantly compared to baseline (54).
In this study, while miR-1278, miR-24-1-5p, and miR-422a correlated with markers of bone remodeling (β-CTX and P1NP), only miR-1278 and miR-24-1-5p correlated with serum sclerostin levels. Both in our untreated and treated women, serum levels of inhibitors of bone formation (sclerostin and Dkk1), and bone resorption (OPG), were not correlated with markers of bone remodeling. Interestingly, circulating sclerostin levels do not decrease in postmenopausal women on antiresorptive therapy with bisphosphonates (55). Taken together, in agreement with previous data (49, 54), our results indicate that changes in serum sclerostin, Dkk1 and OPG levels may represent a compensatory response of the osteocyte functional activity reflecting acute estrogen deficiency and/or estrogen replete state, rather than changes in remodeling of bone.
Above all, the amount of miR-200a-3p was elevated in our patients with low estrogen levels and downregulated in women with high estrogen. Physiologically, miR-200s are overexpressed in several clinical conditions related to estrogen status, such as in the mammary glands during mammary gland development, pregnancy, and lactation (56), as well as in estrogen-dependent cancers (57). In humans, miR-200a-3p was associated with osteoporosis (4), albeit not significantly expressed in patients with osteoporotic bone fractures (11, 13), whereas our GLMM-NB analysis did not reveal any pertinence of miR-200a-3p to lumbar spine BMD or biomarkers. This particular miRNA is notable due to its substantial increase in our patients with low estrogen levels and its apparent relevance to osteoporosis.
Our study did not aim to establish an association of miRNAs with the probability of osteoporosis and low impact fractures. To assess osteoporosis in our cohort, we used the commercially available kit osteomiR® (TamiRNA) (58). However, the screening failed due to an insufficient amount of miRNAs after isolation, and we speculate that our serum samples contained insufficient levels of miRNA for qRT–PCR. Fortunately, our well-designed MPS could detect miRNAs at low concentrations, so we were able to quantify all miRNAs included in osteomiR®. This underlines the importance of sample processing and highlights the advantage of MPS.
Although our study is informative, there are several limitations. First, several lifestyle factors that can influence estrogen levels, such as nutritional status and exercise, were not addressed. Second, the miRNAs of interest had zero counts in several samples; however, we employed a statistical approach, which should resolve this problem. Third, the cohort of 22 pairs of samples might be insufficient for a proper statistical analysis, but recruitment of such a uniform cohort is complicated and time-consuming. Fourth, the duration of our study was relatively short, as it was an exploratory study. Given the short duration of the study and the low number of cases, changes in BMD should be interpreted with caution. Fifth, the ovariectomized women in this study were treated with 1 mg of estradiol. Bone remodeling is dose-dependently regulated by estrogen (59, 60). Estradiol at follicular to periovulatory levels is needed to suppress a mildly activated immune system responsible for increased postmenopausal bone resorption (59). However, women in this study were referred for bone status assessment, and the estrogen therapy was prescribed by their gynecologists. Sixth, the MPS results were not validated by qRT–PCR due to the low miRNA concentration in sera. Seventh, as smoking was reported by only one woman treated with estrogen after oophorectomy, the effects of smoking on the liver metabolism of sex hormones were not taken into consideration in the analyses. Further significant limitations include seasonal variability, vitamin D status, and the effects of PTH on osteocytic sclerostin production (61, 62). In our patients treated with estrogen, no significant correlation was observed between serum sclerostin, serum vitamin D and PTH. Six out of 11 women before oophorectomy were vitamin D insufficient. They were supplemented with 800 IU vitamin D. Previously, such a supplementation did not significantly change sclerostin levels in women (63). In patients with vitamin D severe deficiency (25- hydroxyvitamin D level ≤ 20 ng/mL) receiving a monthly intramuscular injection of 300,000 IU of cholecalciferol, serum sclerostin levels decreased (64). In our untreated patients, after oophorectomy, an increase in serum 25- hydroxyvitamin D was correlated with the increase in sclerostin levels. Finally, this study is descriptive, and our results warrant further validation by additional research.
To our knowledge, this is the first prospective study to compare the associations between changes in estrogen status and relative serum levels of circulating miRNAs with BMD and biomarkers of both bone metabolism in women before and after ovariectomy as well as in ovariectomized women after estrogen treatment. The design of this study eliminates the confounding effects of age on the association between the levels of circulating miRNAs, estradiol, follicle-stimulating hormone, and bone variables (65, 66). The present findings corroborate a few previous studies on the expression of miRNAs during treatment with anti-osteoporotic agents (45), as well as studies comparing profiles in postmenopausal osteoporotic and healthy premenopausal women (66, 67). Although this study reported substantial changes in several miRNAs (let-7d-3p, miR-1278, miR-24-1-5p, miR-422a, and miR-619-5p) that regulate osteoblast and osteoclast differentiation in association with estrogen status, these results require further research and validation in an extended cohort.
In summary, of the 14 miRNAs that showed upregulation with estrogen deficiency and downregulation after estrogen therapy, miR-422a correlated with serum β-CTX and P1NP and miR-1278 correlated with serum β-CTX, P1NP, osteocalcin, sclerostin, and Dkk1. Of the 3 miRNAs showing downregulation with estrogen deficiency and upregulation after estrogen therapy, miR-24-1-5p showed a negative correlation with serum β-CTX, P1NP, osteoprotegerin, and sclerostin levels. These miRNAs represent promising biomarkers in bone pathophysiology in studies on the prevention of postmenopausal osteoporosis and the mechanism of antiresorptive therapies. Future prospective studies are required to validate the potential clinical application of our findings.
Data Availability Statement
The original contributions presented in the study are publicly available. This data can be found in GEO database (accession number GSE194086).
Ethics Statement
The studies involving human participants were reviewed and approved by The Institutional Review Board of the Institute of Rheumatology, Prague, Czechia. The patients/participants provided their written informed consent to participate in this study.
Author Contributions
All authors were involved in drafting the article or in revising it critically for important intellectual content. All authors take responsibility for the integrity of the data and the accuracy of the data analysis JB was responsible for serum miRNA profiling, biostatistical analyses, data interpretation and manuscript preparation. AP was responsible for miRNA isolation and preparation of MPS libraries. LW was responsible for biochemical analyses and data interpretation. HH performed the clinical diagnoses. KP and LŠ performed the critical revision of the manuscript. XS was responsible for data interpretation. JS designed the study, was responsible for blood collection, patient follow-up, bone densitometry, immunoassays and manuscript preparation. All authors had access to the data, and have read and accepted the final version of the manuscript for submission.
Funding
This research was funded by the Ministry of Health of the Czech Republic under grant NV18-05-00394 and by RVO 00023728 (Institute of Rheumatology), Charles University project SVV 260 523.
Conflict of Interest
The authors declare that the research was conducted in the absence of any commercial or financial relationships that could be construed as a potential conflict of interest.
Publisher’s Note
All claims expressed in this article are solely those of the authors and do not necessarily represent those of their affiliated organizations, or those of the publisher, the editors and the reviewers. Any product that may be evaluated in this article, or claim that may be made by its manufacturer, is not guaranteed or endorsed by the publisher.
Acknowledgments
We thank Alena Adamová Ludmila Hauptvoglová, and Tereza Malá, for their excellent technical assistance in this project. We acknowledge the Core Facility Genomics supported by the NCMG research infrastructure (LM2015091 funded by MEYS CR) for their support with obtaining scientific data presented in this paper.
Supplementary Material
The Supplementary Material for this article can be found online at: https://www.frontiersin.org/articles/10.3389/fendo.2022.864299/full#supplementary-material
References
1. Khosla S, Oursler MJ, Monroe DG. Estrogen and the Skeleton. Trends Endocrinol Metab (2012) 23(11):576–81. doi: 10.1016/j.tem.2012.03.008
2. Klibanski A, Adams-Campbell L, Bassford T, Blair SN, Boden SD. Osteoporosis Prevention, Diagnosis, and Therapy. JAMA (2001) 285(6):785–95. doi: 10.1001/jama.285.6.785
3. Hernlund E, Svedbom A, Ivergard M, Compston J. Osteoporosis in the European Union: Medical Management, Epidemiology and Economic Burden. Arch Osteo (2013) 8:136. doi: 10.1007/s11657-013-0136-1
4. Huai Y, Zhang W, Chen Z, Zhao F, Wang W, Dang K, et al. A Comprehensive Analysis of MicroRNAs in Human Osteoporosis. Front Endocrinol (2020) 11:516213. doi: 10.3389/fendo.2020.516213
5. An JH, Ohn JH, Song JA, Yang JY, Park H, Choi HJ, et al. Changes of MicroRNA Profile and MicroRNA-MRNA Regulatory Network in Bones of Ovariectomized Mice. J Bone Mine Res (2014) 29(3):644–56. doi: 10.1002/jbmr.2060
6. Yavropoulou MP, Yovos JG. The "Dark Matter" of DNA and the Regulation of Bone Metabolism: The Role of Non-Coding RNAs. J Musculos Neuro Interact (2018) 18(1):18–31.
7. Jones TL, Esa MS, Li KHC, Krishnan SRG, Elgallab GM, Pearce MS, et al. Osteoporosis, Fracture, Osteoarthritis & Sarcopenia: A Systematic Review of Circulating MicroRNA Association. Bone (2021) 152:116068. doi: 10.1016/j.bone.2021.116068
8. Condrat CE, Thompson DC, Barbu MG, Bugnar OL, Boboc A, Cretoiu D, et al. MiRNAs as Biomarkers in Disease: Latest Findings Regarding Their Role in Diagnosis and Prognosis. Cells (2020) 9(2):276. doi: 10.3390/cells9020276
9. Grillari J, Makitie RE, Kocijan R, Haschka J, Vazquez DC, Semmelrock E, et al. Circulating MiRNAs in Bone Health and Disease. Bone (2020) 145:115787. doi: 10.1016/j.bone.2020.115787
10. Zhao W, Shen G, Ren H, Liang, Yu X, Zhang Z, et al. Therapeutic Potential of MicroRNAs in Osteoporosis Function by Regulating the Biology of Cells Related to Bone Homeostasis. J Cell Physiol (2018) 233(12):9191–208. doi: 10.1002/jcp.26939
11. Seeliger C, Karpinski K, Haug AT, Vester H, Schmitt A, Bauer JS, et al. Five Freely Circulating MiRNAs and Bone Tissue MiRNAs Are Associated with Osteoporotic Fractures. J Bone Mine Res (2014) 29(8):1718–28. doi: 10.1002/jbmr.2175
12. Kocijan R, Muschitz C, Geiger E, Skalicky S, Baierl A, Dormann R, et al. Circulating Microrna Signatures in Patients with Idiopathic and Postmenopausal Osteoporosis and Fragility Fractures. J Clin Endocrinol Metab (2016) 101(11):4125–34. doi: 10.1210/jc.2016-2365
13. Panach L, Mifsut D, Tarin JJ, Cano A, Garcia-Perez MA. Serum Circulating MicroRNAs as Biomarkers of Osteoporotic Fracture. Calcif Tissue Int (2015) 97(5):495–505. doi: 10.1007/s00223-015-0036-z
14. Chen Z, Bemben MG, Bemben DA. Bone and Muscle Specific Circulating MicroRNAs in Postmenopausal Women Based on Osteoporosis and Sarcopenia Status. Bone (2019) 120:271–8. doi: 10.1016/j.bone.2018.11.001
15. Yavropoulou MP, Anastasilakis AD, Makras P, Tsalikakis DG, Grammatiki M, Yovos JG. Expression of MicroRNAs That Regulate Bone Turnover in the Serum of Postmenopausal Women with Low Bone Mass and Vertebral Fractures. Eur J Endocrinol (2017) 176(2):169–76. doi: 10.1530/EJE-16-0583
16. Sun M, Zhou X, Chen L, Huang S, Leung V, Wu N, et al. The Regulatory Roles of MicroRNAs in Bone Remodeling and Perspectives as Biomarkers in Osteoporosis. BioMed Res Int (2016) 2016:1652417. doi: 10.1155/2016/1652417
17. Taipaleenmaki H, Bjerre Hokland L, Chen L, Kauppinen S, Kassem M. Mechanisms in Endocrinology: Micro-RNAs: Targets for Enhancing Osteoblast Differentiation and Bone Formation. Eur J Endocrinol (2012) 166(3):359–71. doi: 10.1530/EJE-11-0646
18. Anastasilakis AD, Papachatzopoulos S, Makras P, Gkiomisi A, Nikolakopoulos P, Polyzos SA, et al. The Effect of Pharmacological Cessation and Restoration of Menstrual Cycle on Bone Metabolism in Premenopausal Women with Endometriosis. Bone (2022) 158:116354. doi: 10.1016/j.bone.2022.116354
19. Stepan JJ, Hruskova H, Kverka M. Update on Menopausal Hormone Therapy for Fracture Prevention. Curr Osteo Rep (2019) 17(6):465–73. doi: 10.1007/s11914-019-00549-3
20. World Medical A. World Medical Association Declaration of Helsinki: Ethical Principles for Medical Research Involving Human Subjects. JAMA (2013) 310(20):2191–4. doi: 10.1001/jama.2013.281053
21. Martin M. Cutadapt Removes Adapter Sequences from High-Throughput Sequencing Reads. EMBnetjournal (2011) 17:10. doi: 10.14806/ej.17.1.200
22. Andrews S. Babraham Bioinformatics - Fastqc a Quality Control Tool for High Throughput Sequence Data. Soil (1973) 5:47–81.
23. Vitsios DM, Enright AJ. Chimira: Analysis of Small RNA Sequencing Data and MicroRNA Modifications. Bioinformatics (2015) 31(20):3365–7. doi: 10.1093/bioinformatics/btv380
24. Kozomara A, Griffiths-Jones S. Mirbase: Annotating High Confidence MicroRNAs Using Deep Sequencing Data. Nucleic Acids Res (2014) 42(Database issue):D68–73. doi: 10.1093/nar/gkt1181
25. Team RC. R: A Language and Environment for Statistical Computing. Vienna, Austria: R Foundation for Statistical Computing (2021).
26. Levey AS, Stevens LA, Schmid CH, Zhang YL, Castro AF, Feldman HI 3rd, et al. A New Equation to Estimate Glomerular Filtration Rate. Ann Intern Med (2009) 150(9):604–12. doi: 10.7326/0003-4819-150-9-200905050-00006
27. Love MI, Huber W, Anders S. Moderated Estimation of Fold Change and Dispersion for RNA-Seq Data with Deseq2. Genome Biol (2014) 15(12):550. doi: 10.1186/s13059-014-0550-8
28. Brooks ME, Kristensen K, van Benthem KJ, Magnusson A, Berg CW, Nielsen A, et al. Glmmtmb Balances Speed and Flexibility among Packages for Zero-Inflated Generalized Linear Mixed Modeling. R J (2017) 9(2):378–400. doi: 10.32614/RJ-2017-066
29. Benjamini Y, Hochberg Y. Discovery Rate: A Practical and Powerful Approach to Multiple Testing. J R Stat Soc (1995) 57(1):289–300.
30. Zeng Y, Qu X, Li H, Huang S, Wang S, Xu Q, et al. MicroRNAa-100 Regulates Osteogenic Differentiation of Human Adipose-Derived Mesenchymal Stem Cells by Targeting Bmpr2. FEBS Lett (2012) 586(16):2375–81. doi: 10.1016/j.febslet.2012.05.049
31. Cheng VK, Au PC, Tan KC, Cheung CL. MicroRNA and Human Bone Health. JBMR Plus (2019) 3(1):2–13. doi: 10.1002/jbm4.10115
32. Weilner S, Skalicky S, Salzer B, Keider V, Wagner M, Hildner F, et al. Differentially Circulating MiRNAs after Recent Osteoporotic Fractures Can Influence Osteogenic Differentiation. Bone (2015) 79:43–51. doi: 10.1016/j.bone.2015.05.027
33. Edmonston D, Wolf M. Fgf23 at the Crossroads of Phosphate, Iron Economy and Erythropoiesis. Nat Rev Nephrol (2020) 16(1):7–19. doi: 10.1038/s41581-019-0189-5
34. Cao Z, Moore BT, Wang Y, Peng XH, Lappe JM, Recker RR, et al. Mir-422a as a Potential Cellular MicroRNA Biomarker for Postmenopausal Osteoporosis. PloS One (2014) 9(5):e97098. doi: 10.1371/journal.pone.0097098
35. Pacifici R, Brown C, Puscheck E, Friedrich E, Slatopolsky E, Maggio D, et al. Effect of Surgical Menopause and Estrogen Replacement on Cytokine Release from Human Blood Mononuclear Cells. Proc Natl Acad Sci USA (1991) 88(12):5134–8. doi: 10.1073/pnas.88.12.5134
36. D'Amelio P, Grimaldi A, Di Bella S, Brianza SZ, Cristofaro MA, Tamone C, et al. Estrogen Deficiency Increases Osteoclastogenesis up-Regulating T Cells Activity: A Key Mechanism in Osteoporosis. Bone (2008) 43(1):92–100. doi: 10.1016/j.bone.2008.02.017
37. Adeel S, Singh K, Vydareny KH, Kumari M, Shah E, Weitzmann MN, et al. Bone Loss in Surgically Ovariectomized Premenopausal Women Is Associated with T Lymphocyte Activation and Thymic Hypertrophy. J Investig Med (2013) 61(8):1178–83. doi: 10.2310/JIM.0000000000000016
38. Cauley JA, Danielson ME, Boudreau RM, Forrest KY, Zmuda JM, Pahor M, et al. Inflammatory Markers and Incident Fracture Risk in Older Men and Women: The Health Aging and Body Composition Study. J Bone Mine Res (2007) 22(7):1088–95. doi: 10.1359/jbmr.070409
39. Shevde NK, Bendixen AC, Dienger KM, Pike JW. Estrogens Suppress Rank Ligand-Induced Osteoclast Differentiation Via a Stromal Cell Independent Mechanism Involving C-Jun Repression. Proc Natl Acad Sci USA (2000) 97(14):7829–34. doi: 10.1073/pnas.130200197
40. Lehmann J, Thiele S, Baschant U, Rachner TD, Niehrs C, Hofbauer LC, et al. Mice Lacking Dkk1 in T Cells Exhibit High Bone Mass and Are Protected from Estrogen-Deficiency-Induced Bone Loss. iScience (2021) 24(3):102224. doi: 10.1016/j.isci.2021.102224
41. Tian J, Xu XJ, Shen L, Yang YP, Zhu R, Shuai B, et al. Association of Serum Dkk-1 Levels with Beta-Catenin in Patients with Postmenopausal Osteoporosis. J Huazhong Univ Sci Tech Med Sci (2015) 35(2):212–8. doi: 10.1007/s11596-015-1413-6
42. Modder UI, Clowes JA, Hoey K, Peterson JM, McCready L, Oursler MJ, et al. Regulation of Circulating Sclerostin Levels by Sex Steroids in Women and in Men. J Bone Mine Res (2011) 26(1):27–34. doi: 10.1002/jbmr.128
43. Fujita K, Roforth MM, Demaray S, McGregor U, Kirmani S, McCready LK, et al. Effects of Estrogen on Bone MRNA Levels of Sclerostin and Other Genes Relevant to Bone Metabolism in Postmenopausal Women. J Clin Endocrinol Metab (2014) 99(1):E81–8. doi: 10.1210/jc.2013-3249
44. Glass DA, Bialek P 2nd, Ahn JD, Starbuck M, Patel MS, Clevers H, et al. Canonical Wnt Signaling in Differentiated Osteoblasts Controls Osteoclast Differentiation. Dev Cell (2005) 8(5):751–64. doi: 10.1016/j.devcel.2005.02.017
45. Anastasilakis AD, Makras P, Pikilidou M, Tournis S, Makris K, Bisbinas I, et al. Changes of Circulating MicroRNAs in Response to Treatment with Teriparatide or Denosumab in Postmenopausal Osteoporosis. J Clin Endocrinol Metab (2018) 103(3):1206–13. doi: 10.1210/jc.2017-02406
46. Eriksen EF. Normal and Pathological Remodeling of Human Trabecular Bone: Three Dimensional Reconstruction of the Remodeling Sequence in Normals and in Metabolic Bone Disease. Endocr Rev (1986) 7(4):379–408. doi: 10.1210/edrv-7-4-379
47. Hannon R, Blumsohn A, Naylor K, Eastell R. Response of Biochemical Markers of Bone Turnover to Hormone Replacement Therapy: Impact of Biological Variability. J Bone Mine Res (1998) 13(7):1124–33. doi: 10.1359/jbmr.1998.13.7.1124
48. Mirza FS, Padhi ID, Raisz LG, Lorenzo JA. Serum Sclerostin Levels Negatively Correlate with Parathyroid Hormone Levels and Free Estrogen Index in Postmenopausal Women. J Clin Endocrinol Metab (2010) 95(4):1991–7. doi: 10.1210/jc.2009-2283
49. Ardawi MS, Al-Kadi HA, Rouzi AA, Qari MH. Determinants of Serum Sclerostin in Healthy Pre- and Postmenopausal Women. J Bone Mine Res (2011) 26(12):2812–22. doi: 10.1002/jbmr.479
50. O'Brien CA, Plotkin LI, Galli C, Goellner JJ, Gortazar AR, Allen MR, et al. Control of Bone Mass and Remodeling by Pth Receptor Signaling in Osteocytes. PloS One (2008) 3(8):e2942. doi: 10.1371/journal.pone.0002942
51. Ahmed SF, Fouda N, Abbas AA. Serum Dickkopf-1 Level in Postmenopausal Females: Correlation with Bone Mineral Density and Serum Biochemical Markers. J Osteo (2013) 2013:460210. doi: 10.1155/2013/460210
52. Yano K, Tsuda E, Washida N, Kobayashi F, Goto M, Harada A, et al. Immunological Characterization of Circulating Osteoprotegerin/Osteoclastogenesis Inhibitory Factor: Increased Serum Concentrations in Postmenopausal Women with Osteoporosis. J Bone Mine Res (1999) 14(4):518–27. doi: 10.1359/jbmr.1999.14.4.518
53. Kudlacek S, Schneider B, Woloszczuk W, Pietschmann P, Willvonseder R. Serum Levels of Osteoprotegerin Increase with Age in a Healthy Adult Population. Bone (2003) 32(6):681–6. doi: 10.1016/S8756-3282(03)00090-5
54. Han KO, Choi JT, Choi HA, Moon IG, Yim CH, Park WK, et al. The Changes in Circulating Osteoprotegerin after Hormone Therapy in Postmenopausal Women and Their Relationship with Oestrogen Responsiveness on Bone. Clin Endocrinol (Oxf) (2005) 62(3):349–53. doi: 10.1111/j.1365-2265.2005.02221.x
55. Chung YE, Lee SH, Lee SY, Kim SY, Kim HH, Mirza FS, et al. Long-Term Treatment with Raloxifene, but Not Bisphosphonates, Reduces Circulating Sclerostin Levels in Postmenopausal Women. Osteo Int (2012) 23(4):1235–43. doi: 10.1007/s00198-011-1675-1
56. Roth MJ, Moorehead RA. The Mir-200 Family in Normal Mammary Gland Development. BMC Dev Biol (2021) 21(1):12. doi: 10.1186/s12861-021-00243-7
57. Fontana A, Barbano R, Dama E, Pasculli B, Rendina M, Morritti MG, et al. Combined Analysis of Mir-200 Family and Its Significance for Breast Cancer. Sci Rep (2021) 11(1):2980. doi: 10.1038/s41598-021-82286-1
58. Kerschan-Schindl K, Hackl M, Boschitsch E, Foger-Samwald U, Nagele O, Skalicky S, et al. Diagnostic Performance of a Panel of MiRNAs (Osteomir) for Osteoporosis in a Cohort of Postmenopausal Women. Calcif Tissue Int (2021) 108(6):725–37. doi: 10.1007/s00223-020-00802-3
59. Straub RH. The Complex Role of Estrogens in Inflammation. Endocr Rev (2007) 28(5):521–74. doi: 10.1210/er.2007-0001
60. Faubion L, White TA, Peterson BJ, Geske JR, LeBrasseur NK, Schafer MJ, et al. Effect of Menopausal Hormone Therapy on Proteins Associated with Senescence and Inflammation. Physiol Rep (2020) 8(16):e14535. doi: 10.14814/phy2.14535
61. Lisse TS, Chun RF, Rieger S, Adams JS, Hewison M. Vitamin D Activation of Functionally Distinct Regulatory MiRNAs in Primary Human Osteoblasts. J Bone Mine Res (2013) 28(6):1478–88. doi: 10.1002/jbmr.1882
62. Bellido T, Ali AA, Gubrij I, Plotkin LI, Fu Q, O'Brien CA, et al. Chronic Elevation of Parathyroid Hormone in Mice Reduces Expression of Sclerostin by Osteocytes: A Novel Mechanism for Hormonal Control of Osteoblastogenesis. Endocrinology (2005) 146(11):4577–83. doi: 10.1210/en.2005-0239
63. Dawson-Hughes B, Harris SS, Ceglia L, Palermo NJ. Effect of Supplemental Vitamin D and Calcium on Serum Sclerostin Levels. Eur J Endocrinol (2014) 170(4):645–50. doi: 10.1530/EJE-13-0862
64. Acibucu F, Dokmetas HS, Acibucu DO, Kilicli F, Aydemir M, Cakmak E. Effect of Vitamin D Treatment on Serum Sclerostin Level. Exp Clin Endocrinol Diabetes (2017) 125(9):634–7. doi: 10.1055/s-0035-1559790
65. Randolph JF Jr, Sowers M, Bondarenko IV, Harlow SD, Luborsky JL, Little RJ. Change in Estradiol and Follicle-Stimulating Hormone across the Early Menopausal Transition: Effects of Ethnicity and Age. J Clin Endocrinol Metab (2004) 89(4):1555–61. doi: 10.1210/jc.2003-031183
66. Feurer E, Kan C, Croset M, Sornay-Rendu E, Chapurlat R. Lack of Association between Select Circulating MiRNAs and Bone Mass, Turnover, and Fractures: Data from the Ofely Cohort. J Bone Mine Res (2019) 34(6):1074–85. doi: 10.1002/jbmr.3685
Keywords: estrogen, circulating miRNA, bone remodeling, oophorectomy, osteoporosis prevention and control
Citation: Baloun J, Pekacova A, Wenchich L, Hruskova H, Senolt L, Svec X, Pavelka K and Stepan JJ (2022) Menopausal Transition: Prospective Study of Estrogen Status, Circulating MicroRNAs, and Biomarkers of Bone Metabolism. Front. Endocrinol. 13:864299. doi: 10.3389/fendo.2022.864299
Received: 28 January 2022; Accepted: 28 March 2022;
Published: 13 May 2022.
Edited by:
Daniela Merlotti, University of Siena, ItalyReviewed by:
Athanasios D. Anastasilakis, 424 General Military Hospital, GreeceBente L. Langdahl, Aarhus University, Denmark
Copyright © 2022 Baloun, Pekacova, Wenchich, Hruskova, Senolt, Svec, Pavelka and Stepan. This is an open-access article distributed under the terms of the Creative Commons Attribution License (CC BY). The use, distribution or reproduction in other forums is permitted, provided the original author(s) and the copyright owner(s) are credited and that the original publication in this journal is cited, in accordance with accepted academic practice. No use, distribution or reproduction is permitted which does not comply with these terms.
*Correspondence: Jan J. Stepan, stepan@revma.cz