Individual learning paths mastering teachers’ professional vision
- 1Institute for Psychology in Education, University of Münster, Münster, Germany
- 2Schlossklinik Pröbsting, Borken, Germany
Introduction: Promoting a professional vision of teaching as a key factor of teachers’ expertise is a core challenge for teacher professionalization. While research on teaching has evolved and successfully evaluated various video-based intervention programs, a prevailing emphasis on outcome measures can yet be observed. However, the learning processes by which teachers acquire professional vision currently remain a black box. The current study sought to fill this research gap. As part of a course dedicated to promoting a professional vision of classroom management, students were imparted knowledge about classroom management that had to be applied to the analysis of authentic classroom videos. The study aimed to determine the variety of individual strategies that students applied during their video analyses, and to investigate the relationship between these and the quality of the students’ analyses, measured by their agreement with an experts’ rating of the video clips.
Methods: The sample comprised 45 undergraduate pre-service teachers enrolled in a course to acquire a professional vision of classroom management. By applying their imparted knowledge of classroom management, students engaged in the analysis of classroom videos to learn how to notice and interpret observable events that are relevant to effective classroom management. Implementing a learning analytical approach allowed for the gathering of process-related data to analyze the behavioral patterns of students within a digital learning environment. Video-based strategies were identified by conducting cluster analyses and related to the quality of the students’ analysis outcomes, measured by their concordance with the experts’ ratings.
Results: We gained insight into the learning processes involved in video-based assignments designed to foster a professional vision of classroom management, such as the areas of interest that attracted students’ heightened attention. We could also distinguish different approaches taken by students in analyzing classroom videos. Relatedly, we found clusters indicating meticulous and less meticulous approaches to analyzing classroom videos and could identify significant correlations between process and outcome variables.
Discussion: The findings of this study have implications for the design and implementation of video-based assignments for promoting professional vision, and may serve as a starting point for implementing process-based diagnostics and providing adaptive learning support.
1 Introduction
Professional vision is a key aspect of teachers’ expertise (Seidel and Stürmer, 2014; Stürmer et al., 2014). Consequently, the effective promotion of a professional vision is a core theme and challenge of teachers’ professionalization.
Over the past two decades, many interventions have been conducted that have identified successful methods for promoting a professional vision of pre-service teachers. However, most of these interventions focused on the question of what supports the outcomes best, whether it was the medium of content (video vs. written vignette), personal engagement (own vs. other teachers’ video), or the kind of feedback on students’ results from analysis (e.g. feedback from experts vs. peers) (Sherin and van Es, 2009; Baier et al., 2021; Prilop et al., 2021). Studies to date have not yet focused primarily on the ongoing process of analyzing classroom videos (König et al., 2022; Gold et al., 2023), for example, the type and choice of strategy participants applied, or, in short, professional vision in the making.
One process-oriented method is the study of the eye-tracking gaze data of participants while they are watching a real or videotaped lesson. Eye-tracking focuses on spatial perception by analyzing eye movements and fixation, which has already yielded numerous valuable results (Gegenfurtner and Stahnke, 2023). A promising alternative approach to consider is the use of Learning Analytics (LA), which can be adapted but still has to establish its suitability for a process-oriented analysis of professional vision, particularly in line with learning designs (Ahmad et al., 2022). This method facilitates the exploration of data from digital educational learning environments to make learning measurable and visible by using and extending educational data mining methods to gain insight into learning, unveiling the black box that learning processes still pose (Long et al., 2011; Siemens and Baker, 2012; Siemens, 2013; Roll and Winne, 2015; Hoppe, 2017; Knight and Buckingham Shum, 2017). In the present study, Learning Analytics was utilized to identify video-based strategies and potential barriers to learning in relation to pre-service teachers’ analyses of authentic classroom videos, primarily focused on the professional vision of events relevant to effective classroom management.
This study introduces novel perspectives on identifying successful and less successful strategies for analyzing classroom videos within the field of teachers’ professional vision. In addition, it showcases an approach to process-based learning diagnostics for acquiring a professional vision of classroom management. Developing the ability to perceive, interpret, and respond effectively to complex classroom situations is essential to preparing pre-service teachers for their future profession. This expertise plays a pivotal role in fostering a conducive learning environment for improving learning engagement and outcomes. Proactive classroom management empowers teachers to anticipate and prevent potential learning disruptions while maintaining a productive learning environment. Understanding cues relevant to learning enables teachers to intervene, adapt, and tailor their teaching to the individual needs of students or situations, enhancing the overall effectiveness of their lessons.
1.1 Teachers’ professional vision
Professional vision is a prevalent construct in German teacher education, derived and adapted from the American researchers Goodwin (1994) and Sherin (2001). According to the Perception-Interpretation-Decision-model of teacher expertise (PID-model), professional vision can considered an important situation-specific skill for teaching, mediating between cognitive and motivational dispositions and performance (Sherin and van Es, 2009; Blömeke et al., 2015; Kaiser et al., 2015). Professional vision is commonly defined as a teacher’s skill in noticing and interpreting significant classroom events and interactions that are relevant to student learning (van Es and Sherin, 2002; Sherin, 2007; Sherin and van Es, 2009; König et al., 2022), and making situationally appropriate decisions on how to proceed during a lesson (Blömeke et al., 2015; van Es and Sherin, 2021; Gippert et al., 2022). While noticing requires selective attention to perceive significant cues for learning and to neglect insignificant ones, interpreting depends on the application of appropriate knowledge in a subsequent process, often referred to as knowledge-based reasoning (Sherin and van Es, 2009; Blomberg et al., 2011; König et al., 2014; Seidel and Stürmer, 2014; Gaudin and Chaliès, 2015; Barth, 2017). The extent of mastering these skills indicates the quality of situated knowledge (Kersting et al., 2012; König et al., 2014; Seidel and Stürmer, 2014). Studies have revealed that the quality of professional vision is positively related to instructional quality, to teaching effectiveness in general (Sherin and van Es, 2009; Yeh and Santagata, 2015), as well as to the learning outcomes of students (Roth et al., 2011; Kersting et al., 2012; König et al., 2021; Blömeke et al., 2022), however, some ambiguous results regarding the association between professional vision and teaching performance have emerged in the past (Gold et al., 2021; Junker et al., 2021), additionally implying the challenges involved in measuring this construct.
1.2 Video-based promotion of professional vision using annotation tools
Linking theoretical knowledge with teaching situations poses a challenge for students, given the inconsistent availability of practical experience during their university studies. The analysis of recorded lessons to promote professional vision in teacher training constitutes an opportunity to address that challenge. Video-based training can nowadays be considered an effective and well-established practice to promote professional vision with a knowledge-based focus (Sherin and van Es, 2009; Santagata and Angelici, 2010; Santagata and Guarino, 2011; Gaudin and Chaliès, 2015; Weber et al., 2018; Gold et al., 2020; Santagata et al., 2021). This kind of video-based setting enables acquiring case-based knowledge, using authentic examples to bridge the gap between theory and practice by reviewing prototypical interactions (Zumbach et al., 2007), such as those from authentic classroom videos. It has also been shown that video-based learning environments help learners produce more sophisticated and in-depth analyses (van Es and Sherin, 2002; Star and Strickland, 2008; Stockero, 2008; Santagata and Guarino, 2011; Barnhart and van Es, 2015; Gold et al., 2020). Therefore, classroom videos are considered an appropriate medium for the application of situated concepts, taught to link knowledge and performance (Seidel and Stürmer, 2014; Barth, 2017).
These objectives can be facilitated by learning environments that provide features for coping with the complexity of teaching and the volatility of interactions in a classroom, such as the ability to pause and repeat certain sections of the video, allowing the breakdown of classroom interactions into smaller segments for a more in-depth analysis. These types of interactive features have been shown to endorse learning processes in other educational settings (Schwan and Riempp, 2004; Blau and Shamir-Inbal, 2021). Annotation tools incorporate these features and support reflective practices by enabling users to annotate video content in a structured manner. They can be used to segment a video, preserve and synchronize enhanced observations with the video timeline (Rich and Trip, 2011; Kleftodimos and Evangelidis, 2016), and are also suitable for a wide range of educational and research applications (Catherine et al., 2021). Annotation tools have previously been used effectively to develop, reflect, and evaluate students’ pre-service teaching practices and those of a learning peer group (Rich and Hannafin, 2008; Colasante, 2011; McFadden et al., 2014; van der Westhuizen and Golightly, 2015; Ardley and Johnson, 2019; Nilsson and Karlsson, 2019; Ardley and Hallare, 2020), besides to analyzing classroom videos of third-party teachers (Hörter et al., 2020; Junker et al., 2020, 2022c; Larison et al., 2022). Interactive annotation tools can foster professional vision by engaging students in sophisticated analysis for a profound understanding of classroom interactions at their pace (Schwan and Riempp, 2004; Risko et al., 2013; Merkt and Schwan, 2014; Koschel, 2021).
1.3 Professional vision of classroom management
Applying Learning Analytics to reveal strategies for analyzing classroom videos requires an analytical focus on the dimensions of teaching in classrooms that should be noticed and interpreted. Classroom management represents one of the pivotal dimensions of teaching quality, alongside cognitive activation and support for student learning (Praetorius et al., 2018; Junker et al., 2021). It involves different facets and denotes instructional strategies aimed at fostering an environment conducive to effective learning (Emmer and Stough, 2001). This includes the deliberate orchestration of teaching, encompassing the establishment of rules and routines, seamless structuring, monitoring, and pacing of classroom activities, along with the prevention and prompt resolution of any learning disruptions or misbehavior that may interfere with the learning process (Kounin, 1970; Wolff et al., 2015; Gold and Holodynski, 2017). To ensure a continuous learning process, it is essential to organize the pacing of activities and manage transitions smoothly (Kounin, 1970). Likewise, established rules provide comprehensible scopes of action, structuring interactions, and contributing to a positive relationship in the classroom (Kounin, 1970). By anticipating and perceiving learning disruptions, teachers can take proactive and reactive actions to either prevent their occurrence or remediate them early on (Kounin, 1970; Emmer and Stough, 2001; Simonsen et al., 2008). Moreover, responding adaptively to classroom situations and individual student needs contributes to maintaining a productive and supportive environment while maximizing effective learning time. This kind of pedagogical knowledge is positively associated with the learning interests and outcomes of students (Kunter et al., 2007, 2013; Seidel and Shavelson, 2007; Evertson and Emmer, 2013; Hattie, 2023). Thus, managing classrooms professionally is an important dimension of teaching quality (Shulman, 1987; König, 2015; Hattie, 2023).
Professional vision of classroom management includes the situated application of this knowledge and can be considered a prerequisite for managing classrooms successfully and effectively. Because professional vision is associated with such essential skills, it is reasonable to assume that the acquisition of professional vision is necessary for teacher education (Blömeke et al., 2015, 2016). The complexity of teaching arises, among other factors, from the simultaneous occurrence of various events in the classroom (Doyle, 1977; Jones, 1996; Wolff et al., 2017), placing high demands on the management of heterogeneous learning groups. To address these demands, video-based courses aim to promote students’ ability to notice and interpret classroom events as a basic requirement for their professional decision-making in the future. Because professional vision can be considered a domain-specific skill based on acquired knowledge (van Es and Sherin, 2002; Steffensky et al., 2015), classroom management serves as a foil for noticing, interpreting, and decision-making related to observable classroom events.
1.4 Video-based analysis strategies revealed by Learning Analytics
Learning Analytics collects, aggregates, analyzes, and evaluates data from educational learning contexts to make learning measurable and visible, opening the black box that learning processes have posed to date. It extends educational data mining methods to gain insights into learning, to tailor content to the learners’ needs, to predict and improve their performance, and to identify success factors and potential barriers concerning learning activities and student behavior (Long et al., 2011; Chatti et al., 2012; Siemens and Baker, 2012; Siemens, 2013; Hoppe, 2017; Knight and Buckingham Shum, 2017).
Learning Analytics can be deployed in educational contexts to better understand video-based learning. This media-specific type of analytics focuses on learner interactions with the video content and the context in which they are embedded (Mirriahi and Vigentini, 2017). Combining and triangulating this data pool with survey and performance data can provide an even more sophisticated view (Mirriahi and Vigentini, 2017). A main interest of this research is to explore the practical application of Learning Analytics when analyzing authentic classroom videos.
1.4.1 Video usage analytics based on clickstreams
Video usage analytics can rely on explicit factors, such as the number of views and their impact on learning outcomes, and implicit factors, such as events emitted by digital learning environments (Atapattu and Falkner, 2018). Córcoles et al. (2021) conclude that this type of data can be valuable for instructors to enhance the learning process, even in limited-scale applications such as ours. Gašević et al. (2016) and Ahmad et al. (2022) suggest that conducted analytics must be adapted to the course context and its learning design. In our study, we use both types of factors to identify participants’ analysis strategies and evaluate their concordance with learning outcomes. Video-based analysis strategies can be depicted as patterns of interactions that learners exhibit in a digital learning environment (Khalil et al., 2023). Within educational contexts, these kinds of patterns are commonly referred to as clickstreams, which can be composed and gathered in different ways. Clickstreams are digital representations of learning processes that encapsulate the behavior and interactions of learners as they engage in a digital learning environment. Clickstream data typically comprises a sequence of interactions performed by learners and environmental events that occurred within the learning process. This data stream refers to a sequence of actions that are captured within the learning activity, usually representing an individual learning journey. Logged data may include more than interactions within digital learning environments. Beyond that, several indicators could be derived from clickstreams, such as emitted events or the context and time spent on parts of the learning activity. By incorporating contextual data, the clickstream can be expanded. Depending on the implementation, clickstreams reveal individual learning paths across all logged activities, allowing longitudinal studies and cross-activity comparisons, for example, throughout a semester. Previous studies have collected clickstream data to analyze behavioral patterns and students’ engagement with learning activities. These studies serve as an orientation for extracting promising measures that can be applied to the analysis of classroom videos, and for providing ideas regarding the feasibility and expectancies of such an application. To derive our hypothesis, substantiate methods as well as data pipelines for our use case, and provide references to promising measures for conceptualizing video-based analysis strategies, previously conducted research was considered and is outlined in the following section.
1.4.2 Conceptualizing, evaluating, and categorizing student approaches to video-based learning
To investigate and explain students’ video-viewing behavior, events that occur in the learning environment, such as play, pause, and seek interactions from the video player, can be collected and evaluated (Giannakos et al., 2015; Atapattu and Falkner, 2018; Angrave et al., 2020; Hu et al., 2020). A more profound investigation of video engagement is enabled by features of video-based learning, including when and how often videos are (re-)viewed (Baker et al., 2021; Zhang et al., 2022), or which and in what order video segments are played, repeated, and viewed more frequently (Brinton et al., 2016; Angrave et al., 2020; Khalil et al., 2023). This facilitates the reconstruction of students’ video-viewing sequences and provides insights into the context and time devoted to segments of the footage. Video interaction behavior analysis has proven to be informative, particularly when exploring the relationship between student engagement and learning success (Delen et al., 2014; Atapattu and Falkner, 2018). It supplies researchers with evidence that engagement patterns might predict performance. Clickstream data approaches, which are used to investigate this kind of relationship, show that engagement patterns affect student learning performance, and that there is a coherence between the viewing behavior and the students’ performance (Giannakos et al., 2015; Brinton et al., 2016; Lan et al., 2017; Angrave et al., 2020; Lang et al., 2020). Indeed, Clickstream data possesses the potential to serve as a predictive model of learners’ performance (Mubarak et al., 2021) and to characterize learners and their likelihood of achieving success in a course.
Therefore, identifying students who are on-track, at-risk, or off-track is an important aim of categorizing learners in educational contexts (Kizilcec et al., 2013; Sinha et al., 2014). This categorization enables targeted interventions and the development of adaptive features. To cluster learners based on their activity patterns and how they achieve their learning goals, Brooks et al. (2011) created an event model for user interactions with a video player, concluding that there are different types of learners concerning time management. Similarly, Kizilcec et al. (2013) classified learners according to patterns of engagement and disengagement with lecture videos, while Sinha et al. (2014) examined the learning effectiveness by delving into clickstream data containing interactions with a video player. To analyze the clickstream data, they grouped behavioral actions into higher-level categories that served as a latent variable, for example, re-watching. Through the characterization of student engagement based on patterns of interactions, learners could be classified into groups that display either low or high engagement. Mirriahi et al. (2016) and Mirriahi et al. (2018) conducted studies to explore student engagement with an annotation tool, thereby providing a comparable environmental setting to our study case. The purpose of this tool was to facilitate reflection on practice and encourage self-regulated learning. During the annotation process, further contextual metadata was captured, such as timestamps for creating, editing, and deleting annotations. They found clusters that characterized different learning profiles, separated by the extent and point in time of their engagement. Khalil et al. (2023) tracked video-related behaviors (like playing, pausing, and seeking) and further video-interaction metrics (e.g. duration of session, maximum progress within the video) across different contexts by video analytics to reveal patterns and cluster video sessions based on the segments watched on the timeline. A common trait among these studies is their utilization of clustering approaches to uncover patterns, despite indicator variations between environments.
To further investigate any presumable associations between interactional patterns and learners’ performance, Li et al. (2015) categorized video sessions according to the characteristics of interactions in terms of frequency and time. To achieve clustering, several features were extracted, such as the number of pauses and seeks. The results showed significant differences between behavioral patterns and the resulting performance. In a similar manner, Yoon et al. (2021) delved into the analysis of behavioral patterns and learner clusters within video-based learning environments, showing that learners who actively engaged exhibited greater learning achievement.
Overall, the studies indicate that gathered clickstream data can be used to discriminate and categorize different approaches to video-based learning. Various behavioral analyses led to the classification of learners in terms of their engagement with the videos. Studies have also identified relationships between engagement behavior and performance using explicit factors, like views or annotations (Barba et al., 2016), and implicit factors, such as types of interaction, like playing, pausing, or seeking within a video (Atapattu and Falkner, 2018). Most studies mentioned focus on large-scale samples, such as Massive Open Online Courses (MOOC). Although studies analyzing exhibited strategies in digital video-based learning environments can be identified in other contexts, there are currently none in the field of professional vision, to the best of our knowledge. However, it can be assumed that similarities in learning formats allow at least some application of the approaches to this domain-specific context. It should be noted that most studies look at interactional behavior during video-viewing, but not at the process of analyzing videos. It is to be expected that interaction patterns in video-based analysis tasks will differ from patterns in video-viewing. Also, other studies typically examine videos that can be seen as an alternative format for conveying content, such as lecture recordings, implying that the videos do not represent the content but rather serve as a medium for presenting it. In our study, the videos act as the content that participants must engage with. As a result, the ways in which individuals engage with lecture recordings are likely distinct from those when analyzing authentic classroom videos, requiring consideration of the video type, activity, and environment. Different ways of conceptualizing and measuring engagement (e.g. Chi and Wylie, 2014; Angrave et al., 2020; Yoon et al., 2021) need to be contemplated, with an awareness of the learning context and goals (Trowler, 2010).
1.5 Aim of this study
Pre-service teachers struggle to identify relevant events in classroom videos selectively (van den Bogert et al., 2014) due to their lack of knowledge and experience (Sherin and van Es, 2005; Blomberg et al., 2011; Stürmer et al., 2014) and their tendency to “focus on superficial matters […] and global judgments of lesson effectiveness” (Castro et al., 2005, p. 11). In contrast, in-service teachers reveal more astute perceptions of classroom events that are relevant for learning (Berliner, 2001; Stahnke et al., 2016), thus disclosing differences between novices and experts in terms of what and how they perceive classroom events (Carter et al., 1988; König and Kramer, 2016; Meschede et al., 2017; Wolff et al., 2017; Gegenfurtner et al., 2020). Using eye-tracking and gaze data, differences in eye movements and fixations were found between novices and experts (Seidel et al., 2021; Huang et al., 2023; Kosel et al., 2023), uncovering disparate patterns of noticing concerning their professional vision. This leads to the assumption that video-based analysis strategies also differ regarding the state of expertise. Furthermore, questions arise as to what extent video-based analysis strategies of pre-service teachers vary among each other and which relationships can be identified between individual learning paths and the respective learning outcomes, primarily concerning selective attention and knowledge-based reasoning as skills related to professional vision.
While the positive outcomes of video-based learning activities developing professional vision have already been confirmed empirically, we do not know how students engage in analyzing classroom videos, which different strategies can be identified, and how they are related to appropriate noticing and interpreting of classroom management practices in the analyzed videos. It is evident that not all students apply learning activities in a way that effectively supports their learning process (Lust et al., 2011, 2013), but what distinguishes successful from less successful strategies?
One aim of this study is to identify and discriminate successful from less successful strategies that were used to cope with the video-based assignments set in the context of a university course on promoting a professional vision of classroom management. To achieve this, the present study uses a novel approach in the domain of professional vision by combining a learning analytical approach and educational data mining methods. This introduces new possibilities for gaining insights into specific learning processes in the context of acquiring professional vision by capturing and evaluating video-based strategies in a digital environment, such as a video annotation tool that accompanies the learning activities of pre-service teachers.
The following two research questions and hypotheses reveal the starting point of our explorative study. We expect findings that reflect the discussed research regarding the difference in noticing patterns and related findings, similar to research approaches in other domains and contexts.
Q1: What are the characteristics of and differences between students’ video analysis strategies?
• H1.1: Video analysis strategies can be derived and discriminated using Learning Analytics
• H1.2: Students exhibit meticulous and less meticulous video analysis strategies
Q2: What distinguishes successful from less successful video analysis strategies?
• H2.1: Video-based analysis strategies relate to learning outcomes
• H2.2: The more meticulous the video-based analysis, the better the outcome, measured as the agreement between students’ and experts’ ratings of the analyzed classroom videos.
This study investigates the behavioral patterns of students’ engagement with an annotation tool while analyzing authentic classroom videos, as well as features of students and their learning processes in relation to the outcome of their respective learning.
2 Materials and methods
2.1 Sample
Participants enrolled in the elective university course according to their curriculum. The participants in this study consisted of 45 undergraduates enrolled in a teacher training program for elementary school. These students were pursuing a bachelor’s degree at the University of Münster in Germany (North Rhine-Westphalia). Overall, 38 students stated that they are female, and 5 students stated that they are male. The distribution of gender is quite typical, given the study objective of prospective elementary school teachers. On average, the students were 21 years old, and 91% of them were in the fourth semester of their six-semester total (standard) study period (see Table 1).
2.2 Session structure for acquiring professional vision
To acquire a professional vision through video-based analyses of lesson clips, a blended learning environment is provided to students, integrating various modes of learning. In comparison with traditional modes of instruction, there is evidence that blended learning approaches tend to more effectively promote student engagement and performance (Chen et al., 2010; Al-Qahtani and Higgins, 2013). The session structure is based on a prototype for video-based teaching in the context of professional vision proposed by Junker et al. (2020), which takes media-didactic principles into account, such as the cognitive theory of multimedia learning (Mayer, 2014) as well as the cognitive apprenticeship theory (Collins et al., 1989), as the lecturer demonstrated the analysis as an expert model, scaffolded with feedback, and also supported articulation and reflection in plenary discussions.
The structure comprises different sessions that build upon each other, increasing the demands with the progression of learning. The content of the course is divided into several sessions, including an introductory session that familiarizes learners with the basic concepts and learning objectives of the course, serving as an advance organizer. Thereupon, new facets of classroom management are introduced weekly, starting with the facet “Rules, Routines and Rituals,” followed by “Monitoring” and “Managing momentum” (Gold and Holodynski, 2017; Gold et al., 2020). Overall, the learning material is presented in a learning management system (LMS) based on the open source software Moodle (RRID:SCR_024209). This approach promotes self-regulated learning and enables students to access and revisit material as needed. Included activities can be carried out at the student’s own pace.
Phases of collaborative, synchronous blended learning take place at the university. These sessions consist of a theoretical introduction to a facet of classroom management and guided exercises using a video annotation tool to practice noticing and interpreting relevant classroom events related to the specific facet in focus. The aim is to introduce new concepts to students in a guided manner, so as to ensure comprehension. Participants are provided the opportunity to practice through exercises within a video-based annotation tool during the session and discuss the results of their work in plenary.
In contrast to exercises within the sessions, asynchronous phases provide a self-regulated analysis assignment of an authentic classroom video. In order to prepare for these assignments, participants had to complete an interactive quiz that helped them recollect and reinforce the learning contents covered in the presence phase, thereby aligning their knowledge baseline. This prerequisite provides instant feedback to students regarding their theoretical knowledge of the current session phase. A working time of 60 min is proposed to establish a consistent reference point for the assignments. No time limit is enforced during the activity, nor are students given direct feedback on the actual time spent, thus promoting self-regulation skills simultaneously with the learning activity. These asynchronous phases facilitate a more in-depth understanding of concepts through their application in video-based assignments, and allow students to self-assess the skills they have acquired through completing the assignments and reflecting on them independently. In addition, instructors can use the results to identify common misconceptions and to tailor subsequent instruction and guidance to the needs of the group. This advantage of a blended learning pattern creates a more personalized learning experience for the course.
2.3 Instruments
2.3.1 Classroom videos
For the video analyses, three video clips were selected, and the video-based assignments were carried out in the listed order (see Table 2), each with a 2-week time offset. The videos and clips used in this course originate from the portals “ViU: Early Science” (Zucker et al., 2022) and “ProVision” (Junker et al., 2022b). Seamless access to the portals for the pre-service teachers was established through the Meta-Videoportal unterrichtsvideos.net (Junker et al., 2022a).
Prior to analyzing the selected video clip, contextual information about each lesson was given to help students understand the goal and content. Clip 1 showed an excerpt from a lesson, whereas clips 2 and 3 were embedded in the context of an entire lesson. In terms of the assignment, this means that the students were able to view more contextual video content in clip 2 and clip 3, beyond the temporal boundaries set by the assignments. Upon launching the annotation tool, the starting frame was automatically set to the defined time for the respective analysis. Consequently, in the subsequent subsection, we refer to video progress based on the provided analysis periods, with 100% progress indicating complete viewing of the specified section. Progress values exceeding 100% show that students have accessed additional teaching context outside the provided time intervals.
2.3.2 Coding manual for observable events related to classroom management
Participants were introduced to a coding manual of observable classroom events which are structured along the three facets of classroom management, namely “Monitoring,” “Structuring momentum,” “Rules, Routines and Rituals,” and their sub-facets according to a coding manual of Gippert et al. (2019). The manual contains labeled codes and explanations for each facet and sub-facet. This serves as a framework for supporting the analysis of classroom videos, directing students’ attention to specific aspects of the video. It standardizes observations and vocabulary use by providing meaningful codes for relevant classroom events. The provided coding manual is used to analyze authentic classroom videos by annotating segments of the video with specific codes whenever an event significant to classroom management occurs that corresponds to the sub-facets. The list of sub-facets limits the relevant events that need to be observed by basic cueing principles and therefore reduces the cognitive load during activities (Guo et al., 2014; Mayer and Fiorella, 2014; van Gog, 2014).
2.3.3 Video-based assignments
The video clips were analyzed using the open source, web-based Opencast Annotation Tool (OAT; RRID:SCR_023934). This digital video annotation tool is part of a local on-premises video streaming and research service which is based on Opencast (RRID:SCR_024764). The OAT is initialized by the students through the LMS, using the learning tools interoperability (LTI) e-learning standard, to achieve a seamless learning experience. By supplying the annotation tool with individual access roles, a pseudonymous identifier, and the course context, students can utilize their existing single sign-on session (SSO) for authentication and authorization, which is pertinent because of legal restrictions on viewing authentic classroom videos. To ensure the protection of privacy for individuals who have consented to the collection of learning data, as well as the teachers and students featured in the classroom video, it is essential to establish proper authorization measures. This type of implementation also creates a protected digital learning space that keeps learning activities and interactions within the established learning context. Ensuring a comfortable learning environment is vital for maintaining a focused learning process, allowing for interpretive and evaluative mistakes, and encouraging collaboration and discourse between students and instructors. The annotation tool serves as a digital learning environment and offers several features for analyzing videos (see Figure 1). The features of the annotation tool can help students observe volatile classroom events. In our study, the OAT was used to annotate classroom videos with the provided coding manual and a specific annotation template.
Categories and codes. The annotation tool assists the analysis tasks by providing a user interface to annotate the video with codes from color-coded categories representing the facets of classroom management required for analyzing the lesson recordings in our use case.
Views and playback controls. Students can navigate video reception with basic playback controls, such as play, pause, loop, and seek. Switching to full screen allows for focusing on details, including background interactions. The tool experience can be personalized with unique split-screen views by adjusting the feature areas.
Timeline, Tracks, Annotation types. Annotations are organized and viewed with precision using a timeline. The timeline aids in recognizing specific segments of the video along with the annotated content. Students can create connected multi-content annotations (MCA) using various annotation types, for example, by combining free text and codes with or without a scale. Annotations containing codes are displayed in a color-coded format and can be arranged on multiple tracks in the timeline.
2.4 Data collection
2.4.1 Capturing video-based analysis strategies revealed by Learning Analytics
To collect measures composing the strategies, we extended the OAT with the capability to exchange data that is compliant with the Experience Application Programming Interface (xAPI) e-learning standard, following the specifications of the Advanced Distributed Learning Initiative (ADL, 2017). This enables data gathering from learning experiences in a standardized format, such as video-based assignments, using the OAT. To store the generated data on our premises, we deployed a compliant Learning Record Store (LRS). This data repository stores the data issued by the OAT, which acts as a relaying Learning Record Provider (LRP). The data model itself and the web service conform to the IEEE 9274.1.1 standard (IEEE, 2023). An implementation of a player adapter serves as a proxy between the video player and other components within the OAT, managing events related to the player that occur during tool usage. This setup allows for a standardized retrieval of events within the video-based exercises and assignments.
The structure of the transmitted data is defined by xAPI, consisting primarily of statements issued by the LRP (ADL, 2019). Statements include (meta-)data about the learner (actor), the specific type of interaction (verb), the related video-based exercise or assignment (object), and contextual (context) or outcome-related information (result) (ADL, 2019; xAPI Video Community of Practice, 2019b). Statements are used for describing data points of events, indicating individual experiences in a learning activity, for example:
A student (actor) can pause (verb) the video in the learning activity (object) within a specific session (context) and might have achieved outcomes, for example, a set of segments played (result) so far.
Because there can be various experiences within learning activities, a standardization of the statements beyond the structure is necessary. To enhance the semantic interoperability of the data, we adapted the official xAPI Video Profile v1.0, created by the ADL xAPI Video Community of Practice (2019b). This application profile standardizes statement content and prevents fragmentation across implementations. It defines a default set of rules regarding the use of statements and concepts, such as types of interactions based on a controlled vocabulary (verbs), to ensure that the interpretation and meaning of the data are consistent between platforms. Based on the previous explanations, the specific nature of the learning activity must be kept in mind. The profile is limited to video-based experiences. Since our learning activity is not a purely reception-oriented experience, it is necessary to extend this default set to better track the learning experiences and related interactions within the OAT. Therefore, we reused related concepts from the xAPI Profile Server (ADL, 2023) and the xAPI Registry (Brown, 2018), such as the standardized verbs annotated, commented, and replied as types of interaction that can also occur in the OAT, complementing the xAPI Video Profile. We were able to obtain several measures that are used to express the composition of video-based strategies and outcomes.
2.4.2 Measures of video-based analysis strategies
Measures expressing video-based strategies were extracted from the databases (see Table 3). The gathered xAPI statements included the following interactional events: played, paused, completed, interacted (toggle full-screen video-viewing), seeked [sic] (xAPI Video Community of Practice, 2019a). The data was aggregated by the respective video activities and students. This enables tracing individual learning paths based on sequential viewing and interactional behavior as well as the annotation process, including creating and revising annotations on the video timeline.
2.4.3 Outcome measures
Measures expressing learning outcomes were extracted as well (see Table 4). Learning outcomes that are related to the quality of the participants’ professional vision of classroom management were assessed by comparing the participants’ annotations of each video clip with the annotations of experts, resulting in an agreement score. A rating of experts (n = 4) was used to compare the quality of the analysis. To create this rating, experts were asked to use the coding manual to annotate relevant events in the respective video clips. The resulting experts’ rating served as a reference for the evaluation of students’ codings.
2.5 Data analysis
2.5.1 Cluster analyses of process-related measures
We conducted cluster analyses to identify structures, separating students based on distinguishable analysis strategies. According to our assumption, we expected to find at least two groups, classified into (1) meticulous analysts, who performed a fine-grained analysis with great diligence, and (2) students with a more superficial view of and engagement with the video content. Therefore, we expected at least two derivable clusters (meticulous and less meticulous analysts).
2.5.1.1 Cluster analysis I (played segment data)
Although we already expected a certain number of clusters, we did not split up the data into a pre-defined number of clusters but analyzed the data in a more explorative bottom-up manner within the data pool of our video clips. Hierarchical clustering enables us to gain this knowledge directly from the data, without relying on assumptions about the shape or size of clusters. Given the intention of merging students based on their approach to video-based analysis, the hierarchical clustering method is a suitable choice. The data was hierarchically clustered using normalized Euclidean distances and the Ward linkage method because of its robustness with outliers, in order to create well-balanced clusters with small variances (Ward, 1963). The quality indices of the clusters were calculated and compared with the standardized data centered and scaled to unit variance for up to ten cluster solutions to determine the optimal number of clusters.
2.5.1.2 Cluster analysis II (students process and outcome data)
In order to identify structures across all clips, a second cluster analysis was performed using the process variables. This analysis utilized a hybrid two-step cluster analysis approach, combining the variance-based approach of Ward and k-means (Punj and Stewart, 1983). The distances were computed with log-likelihood and the optimum number of clusters was determined using the Bayesian Information Criterion (BIC). To test our hypothesis that certain analysis strategies lead to more accurate results, a cluster analysis was performed on each clip.
2.5.2 Correlation and regression analyses
To understand the relationship between process variables and outcome variables, we conducted correlation and regression analyses. The primary goal was to identify process variables that might serve as a predictor for outcomes. Possible correlations might indicate distinguishable differences between student behavior and outcomes, enabling us to conclude what variables shape and possibly determine students on a learning path with a greater probability of succeeding in terms of the defined outcomes.
3 Results
In an effort to grasp how students approach the assignments, an exemplary analysis is offered as an introductory exploration of student engagement with the analysis of classroom videos. Before comparing the students in between, we try to understand individual differences in the video-viewing behavior by looking more closely at the video analysis metadata and video segments that were frequently watched and repeated.
3.1 Video-based analysis strategies for classroom videos
Compared to more receptively oriented learning activities, the discrepancy between the session duration of the analysis and the effective viewing time of video material is striking in video annotation assignments (see Table 5).
In this analysis example (see Table 5), a little over 30 min were spent using the interactive functions of the annotation tool, and only about 8 min were devoted to receptive activities.
In addition to the process and outcome variables of the analysis presented, the number and order of video segments viewed, provide insights into how students proceed with their analysis. As a result, frequently repeated segments in videos become evident, as well as segments that received less attention. Played video segments also indicate the sequential watch order of time intervals within the video, representing the video-specific navigation and viewing behavior. A larger number of played segments represents a more fine-grained and meticulous analysis than a lower number of segments, which may then cover segments with longer periods.
To understand differences in the viewing behavior displayed by students, we gain a first impression through the respective repeated video segment heatmaps. The following heatmap of repeated segments compares the video-based analysis of a student example (S: a07) with the played segments of the group (G), capped to the specified range of the assignment from second 315 to 490 with a total duration of 175 s in the context of a whole lesson recording (clip 3) (see Figure 2).
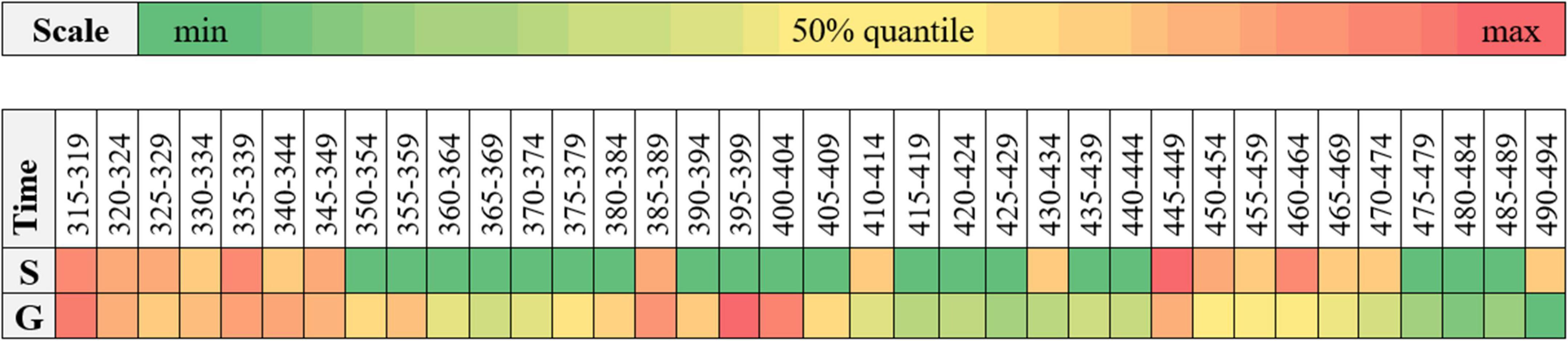
Figure 2. Number of repeated segments per 5-second time frame, compared between student a07 (S) and the mean of the group (G), where green indicates minimum repetitions, yellow the 50% quantile, and red is the maximum repetition.
It is noticeable that this student paid closer attention to the beginning timeframe (315–350) and later portions of the video (445–475), since these segments have more repetitions, but reviewed the middle part less meticulously (see Figure 2). However, the group additionally focused on a time period in the middle (395–405), which indeed includes classroom events relevant to learning that were not annotated and thus overlooked by this student. As this data provides some initial indications regarding the hypothesis that there are differences in terms of individual analysis strategies, in particular, more meticulous and less meticulous approaches, we consider the comparison of the entire group below.
3.2 Cluster analysis of heatmap data
To analyze and compare the group of students, the heatmap data of repeated segments for clip 3 was clustered hierarchically. The heatmap data illustrates the repetition of segments played, with individual students displayed in rows and clip time per 5-s period in columns (see Figure 3). The colors represent the number of repetitions, while blue colors express none to just a few segment repeats, red colors indicate numerous segment repeats, and green colors repetitions in between. Different patterns visualized within the heatmap serve as a reference point for our hypothesis that there is a difference in how meticulously students analyze the classroom video clips.
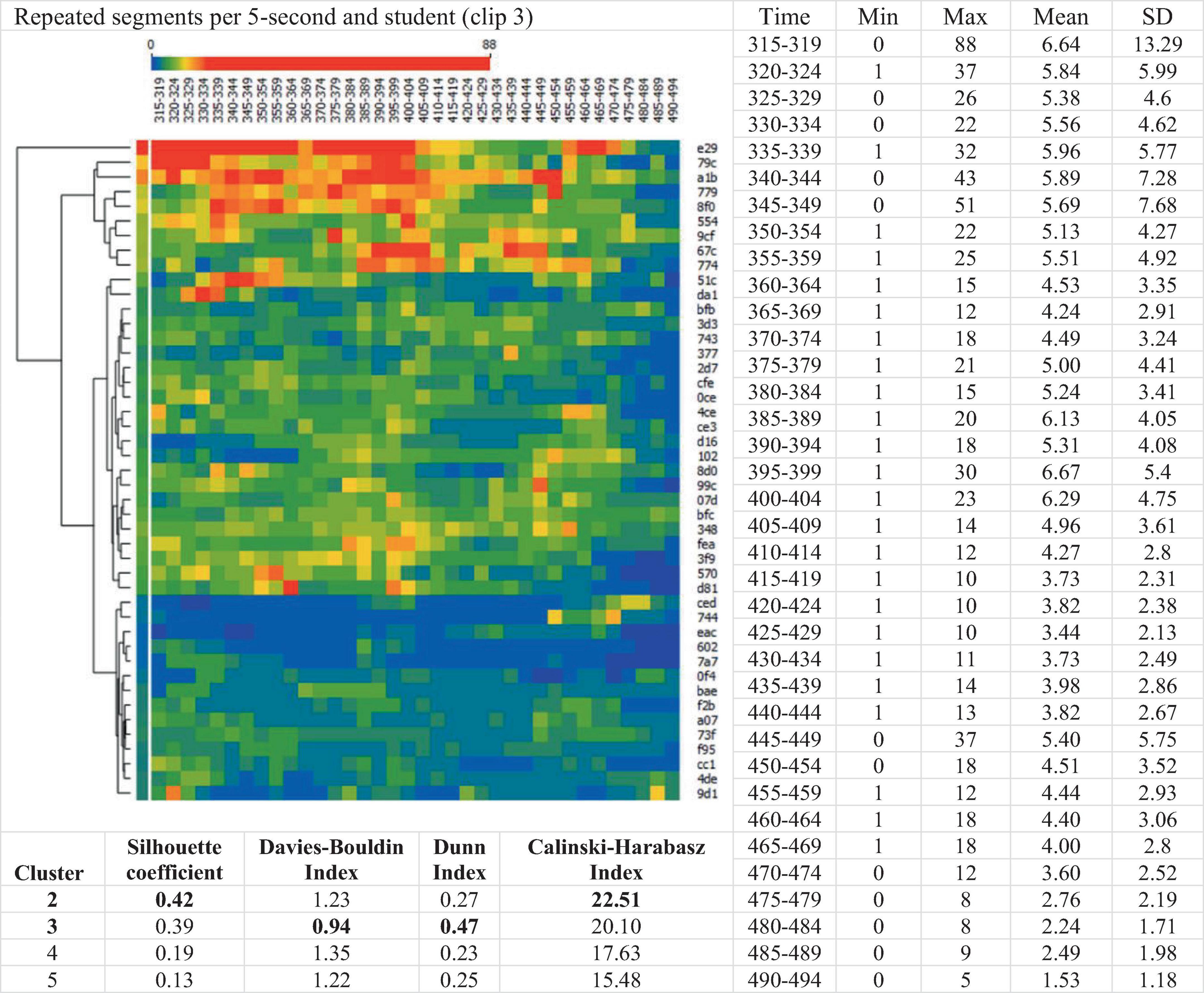
Figure 3. Heatmap and descriptive data presenting the number of repeated segments (Clip 3) per 5-second time frame, showing clusters, with optimal cluster sizes highlighted.
The hierarchical clustering shown in the heatmap (see Figure 3) is ordered by the similarities of the adjacent elements, to minimize the distances. Clusters at the top contain students with a greater number of repetitions, while clusters at the bottom signal fewer repetitions, as alternatively hinted by the colors of the heatmap. While the Silhouette score and Calinski-Harabasz index favored a two-cluster model, the Davies-Bouldin and Dunn index favored three clusters. The Silhouette scores of two and three clusters were only slightly apart, and both indicated a moderate structure. Since the quality of clusters was lower for a larger number of clusters, only up to five cluster splits were reported here. In conclusion, it is possible to differentiate between at least two groups of students. Those exhibiting high repeating behavior, thus analyzing the video content more meticulously, and those who re-watched video segments less often, signaling that they had not dealt with the video content as meticulously as the other group did. Based on the heatmap data, it also seems salient that later segments were repeated less frequently, suggesting an overall reduction in the intensity of observation toward the end of the clip.
3.3 Comparison of the three video clips
Descriptive data yield insights into process-related variables and their distribution within the group by comparing data from the video clips (see Table 6). This also allows for the comparison of interaction behavior across different video annotation assignments.
3.3.1 Inter-individual differences and process-related similarities
There was a high standard deviation in the count of segments played, the count of revisions, and the count of total statements (see Table 6). Used as a measure for distinguishing meticulous from less meticulous analysts, this deviation supports the hypothesis that video-based analysis is performed with different strategy use, derivable using Learning Analytics as considered. Concerning the technical video-player interactions within the process of analyzing, such as play, pause, seek, differences between the video clips were not significant (n.s.). Similarly, the count of statements and played segments were not significantly different, presumably because of the comparable clip lengths.
In terms of outcome variables, the clips deviated significantly (see Table 7). Students managed to achieve a greater percentage of agreement with experts, with fewer events interpreted in the last clip (clip 3). However, a learning gain regarding the outcome variables can neither be directly inferred nor rejected, based on this data. The data shows students tend to underestimate events overall, compared to experts. Nevertheless, there was an acceptable agreement of about 60% for clips 1 and 3 and about 50% for clip 2 of the students’ ratings with the experts’ rating.
3.4 Correlations between process- and outcome-related variables
We also performed analyses on the aggregated data of all clips, as well as each clip on its own. By observing significant correlations between process variables in the aggregated data of all clips, we can draw some conclusions about students’ consistent learning behavior in the digital environment and video-based assignments (see Table 8). The correlation matrix (see Figure 4) shows relationships between all process variables as well as our outcome variable. The brighter the color within the correlation matrix, the greater the Pearson correlation coefficient. Below, we take a more in-depth look at significant correlations that highlight relationships between the process data.
3.4.1 Students who revisit the activity
Students revisiting the learning activity (count of sessions) also revised their annotations more often than others (r = 0.323, p = 0.030). One reason for this may be the harnessing of other learning resources, e.g. the content of course sessions, thus leading to a greater extent of revisions afterward. They also engaged with the video content for a longer period (effective watch-time) (r = 0.420, p = 0.004) and more granular regarding the count of played segments (r = 0.341, p = 0.022). Students with more frequent revisits also exhibited a greater engagement in terms of statement count (r = 0.381, p = 0.010).
3.4.2 Students with high engagement
Students engaging with the digital learning environment more frequently (count of statements), thus creating a larger number of statements, displayed a significantly larger effective watching time (r = 0.723, p < 0.001). Those students tended to show a more meticulous analysis, which was characterized by a video-viewing behavior that was very granular, with a larger count of played segments (r = 0.758, p < 0.001) and more frequent cycles of playing and pausing the video.
3.4.3 Students with full-screen viewing behavior
Students who switched to a full-screen video-viewing mode more regularly (count of interacted statements) also had a larger number of segments played and thus exhibited more granular viewing behavior (r = 0.691, p < 0.001). Students who used the full-screen viewing have a moderately larger count of matched events (r = 0.299, p = 0.048). Moreover, full-screen mode might be more engaging as there was a moderate relationship between the switches to full-screen and the progress (r = 0.423, p = 0.004) as well as watch-time (r = 0.572, p < 0.001).
3.4.4 Students with a high event count
Aggregated data from all three clips shows that students’ event count correlated strongly with the count of matched events with the experts’ rating (r = 0.710, p < 0.001, R2 = 0.503, SER = 1.59) (see Figure 5). This means that a consistent set of their observations matched those of the experts across the clips. With an increasing number of coded events, the rate of matching events remained constant. Even though an increasing number of coded events does not necessarily tell us anything about performance classes the student might belong to, the count of events seems a predictive measure across all clips regarding our outcome variable. As the students display underestimating behavior in general, several factors might contribute to the missing out on events in the classroom relevant to learning.
3.4.5 Correlations with the count of matched events
Considering the count of matched events with the experts’ rating as outcome variable, we found weak to strong correlations with process variables (see Table 9). We discovered a strong and consistent relationship between the count of events coded by students and the count of matched events. This connection was present across all video clips and clearly relates video-based analysis strategies to learning outcomes as hypothesized (see Figure 5). Besides this, carrying out a more meticulous video-based analysis was positively associated with better results in terms of matched events with the experts’ rating, although a significant relationship could only be determined for clip 3, therefore showing only limited evidence that there is a moderate effect regarding a more granular viewing of the clip and intended outcomes. However, the presence of significant correlations between the process variables and our outcome variable still strengthens our hypothesis that analysis strategies can be differentiated based on their effectiveness and process-related data, though this kind of relationship was not consistently observable within the data of our other video clips. Furthermore, this also indicates that Learning Analytics is indeed capable of uncovering these kinds of relationships to some extent in the first place, allowing for building data-based interventions and adaptive learning support later on.
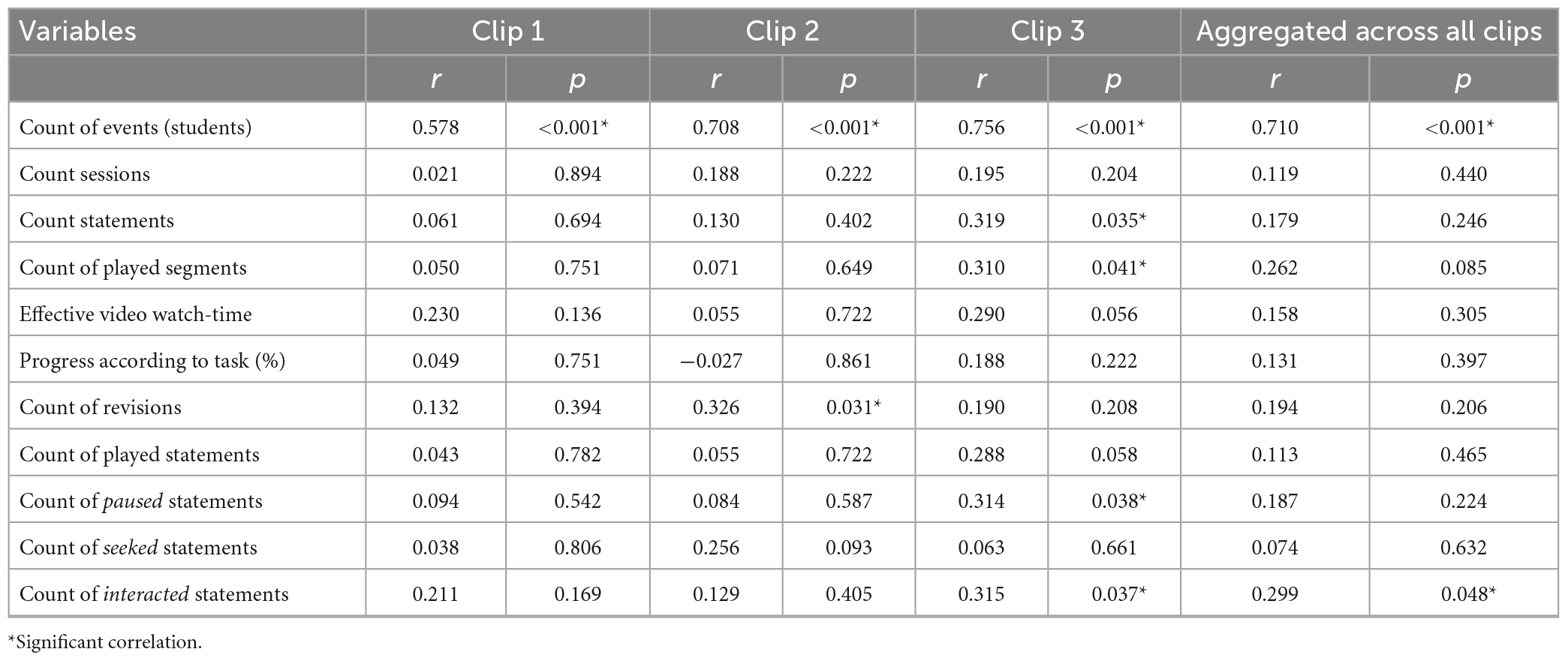
Table 9. Clip-wise correlations between process variables and outcome variable (count of matched events).
3.5 Cluster analysis
To test our hypothesis that certain analysis strategies lead to better outcomes, a cluster analysis was performed on each clip, and correlations of the cluster membership with outcomes were investigated. The count of specific statements was not used to form the clusters, because of collinearity with the count of statements. We conducted the two-step cluster analysis and computed the distances with the log-likelihood function. The optimum number of clusters was determined using the Bayesian information criterion (BIC).
The silhouette coefficient as a measure of cohesion and separation was moderate (see Table 10). Cluster membership was positively associated with learning outcomes. However, the relationship was weak and not significant. This suggests that the expected relationship between a more meticulous student analysis and an improved agreement with the experts’ rating cannot be concluded from this specific cluster separation, although at least the direction of the correlation corresponds to the expectations. To further investigate the separation and differences between the clusters on a feature basis, we conducted a t-test (see Table 11).
The results indicate the clusters differed significantly in various process variables used to constitute these clusters, so that well-separated clusters can be identified. Less meticulous analysts exhibited fewer revisions of annotations (count of revisions), visited the learning activities less often (count of sessions), showed fewer overall engagement (count of statements), and viewed the video only to the extent necessary (progress), without voluntarily including larger teaching contexts, what could otherwise express motivated self-interest or a desire for more in-depth understanding of contexts. The clustering offers an understanding of the features and distinctions among students’ video-based analysis strategies, in relation to our research question. It is illustrated that the utilization of Learning Analytics provides insights into whether students analyze classroom videos in a more meticulous or less meticulous manner.
4 Discussion
The aim of this study was to research individual approaches to analyzing classroom videos to promote professional vision of classroom management. In this section, an overview of the study’s findings is provided and discussed, addressing the research questions and hypotheses established.
4.1 The learning analytical approach and the role of segments in video-based analysis strategies
Our first hypothesis, investigating the characteristics and differences between students’ video analysis strategies, stated that distinguishable video analysis strategies can be derived using Learning Analytics while students analyze a classroom video using an annotation tool.
We showcased that Learning Analytics can reveal insights into learning processes in the context of video-based assignments to promote a professional vision of classroom management and allows for deriving and distinguishing video-based analysis strategies from process-based data. By observing granular video-watching behavior during the analysis of classroom videos, we could uncover that students exhibit varying approaches to video-based analysis, demonstrating strategies for coping with the teaching complexity featured in the respective video segments. Clusters emerged from the examination of segment repetitions, encompassing less meticulous and meticulous approaches to video-based analysis, representing the notable deviation in segment repetitions between individuals (see Figure 3). These clusters bear resemblance to findings in other studies, where clusters were formed based on student engagement with videos in different contexts (Kizilcec et al., 2013; Lust et al., 2013; Mirriahi et al., 2016; Khalil et al., 2023). The segment analysis revealed sequential viewing behavior, suggesting that students who rewatched fewer video segments showed a less meticulous and superficial engagement with the video content, compared to more meticulous analysts who exhibited a fine-grained viewing behavior, paying closer attention to several parts of the video content.
We pointed out indications that observing segment repetition and sequential viewing behavior can help identify patterns and infer conclusions about the viewer’s engagement with the video content. These conclusions might be suitable for providing adaptive learning support during the analysis in the future, for example, cueing specific video segments that were missed but contain important classroom events. The results also revealed several potential follow-up research topics to further investigate this type of data, such as examining student behavior concerning a more content-oriented perspective of the video segments, like the difficulty (Li et al., 2005) or concrete classroom events that are observable, for example, by using the experts’ rating as an underlying semantic content structure for the video. Incorporating segment data is a fundamental aspect when embarking on the initial stages of creating a personalized learning experience. By leveraging the acquired heatmap data, it becomes possible to provide customized feedback that aligns with individual student behavior.
4.2 Types of video-based analysis strategies
To examine the characteristics and differences between students’ video analysis strategies, our second hypothesis stated that students exhibit individual strategies that can be discriminated into meticulous and less-meticulous approaches to analyzing classroom videos.
The exploration of the played segment data revealed that a group of students analyzed the videos in a fine-grained manner, thus using a more meticulous strategy to reveal the events relevant to classroom management, while another group explored the content on the surface, with fewer repetitions of video segments. A further qualitative evaluation seems desirable, given the moderate quality of the cluster (see Figure 3).
In terms of overall engagement, the number of repetitions decreased throughout the clip, implying a reduction in the intensity of observations by students toward the end of the clip. This result might indicate a decrease in attention or motivation, according to similar findings in the video-viewing behavior of students within other contexts (Guo et al., 2014; Kim et al., 2014; Mayer and Fiorella, 2014; Manasrah et al., 2021). Another reason for such an analysis pattern could be the lack of self-assigned time spent on the activity in a self-regulated setting, or other duties, since the work on the video-based assignments could be interrupted at any time.
A correlation of the segment repetitions with the outcome variables was examined only indirectly through the number of segments played. It cannot be concluded, based on the played segment data, that more segment repetitions are associated with better learning outcomes, as this was not part of the study. Within other contexts, it was found that rewatches of (lecture) videos supported memory recalls and had a positive effect on subsequent exam scores (Smidt and Hegelheimer, 2004; Patel et al., 2019). Further research seems necessary to investigate this type of relationship in the context of acquiring professional vision, not least because an alternative explanation is that some students may simply have taken longer than others to recognize relevant events in the video and thus have displayed more segment repetitions. This reasoning aligns with findings for lecture videos, either where less frequent views indicated high-achieving students and a high number of repetitions indicated low achievers (Owston et al., 2011), or where students struggled with the difficulty of the video content (Li et al., 2005, 2015). This kind of observation has to be examined carefully, as interesting and confusing parts of a video both lead to a peak in repetitions (Smidt and Hegelheimer, 2004). As these results were found in the context of videos presenting the content rather than being the subject of learning, such as lecture videos, they cannot be applied elsewhere without further adaptation, but reveal possible research topics for our field of interest. With recourse to Kalyuga (2009) and Costley et al. (2021), another explanation could be that strategic use entails additional cognitive load.
4.3 Analysis strategies compared across different classroom videos
The use of Learning Analytics revealed inter-individual differences in analyzing classroom videos. Considering the process variables, students showed process-related similarities in the choice and technical nature of video-based analysis strategies across the three video clips, but also inter-individual differences, confirming the hypothesis that students could be divided into different groups concerning the pattern of their applied strategies. The high deviation between process variables indicates differences in engaging with the videos (see Table 6). It is not surprising that there were non-significant differences between process variables across the clips, and we did not expect to find any in the first place. The similarity in lengths of the video clips is one possible factor for the lack of significance. It may be the case that analyzing clips of the same length simply leads to similar characteristics of the process variables about the technical interaction behavior with the video player. Another possible explanation is that students’ individual strategy use did not change over time, and thus these students showed consistent analysis behavior in different video-based assignments. In this case, the non-significance would be roughly as expected.
The data also shows that students tend to underestimate events overall, in comparison to experts (see Table 7). Underestimation of events can have several causes. The events varied in number and difficulty between the clips, for example, because multiple events overlapped and occurred at the same time. In alignment with knowledge about the acquisition of professional vision as outlined in the introduction, students might have spent time noticing irrelevant events, were simply not yet able to notice the relevant ones in strict comparison to the experts’ rating, showing a lack of expertise in observing specific events (van den Bogert et al., 2014), or simply may not have had or taken enough time to identify all the noticeable events. In order to determine if certain types of events are being overlooked, further research could involve analyzing events at a content-level, considering the present absence of data on the difficulty of observable events.
4.4 Relationships between process- and outcome measures
To examine what distinguishes successful from less successful video analysis strategies, our third hypothesis stated that the strategic use of video-based analysis relates to success in learning.
We have discovered notable correlations among various process variables that represent student behavior, thereby adding further evidence to support the identification of different strategy use (see Table 8). Students who revisited the activity more often demonstrated a more nuanced viewing behavior, characterized by an increase in the number of segments played and effective watch-time, although these significant correlations were only weak to moderate. Revisiting the learning activity may indicate the use of other learning resources, such as the content of course sessions, and thus lead to a larger number of subsequent revisions observed. In this case, triangulating other data may prove advantageous in uncovering further explanatory approaches to reveal the learning paths. Students who displayed high engagement or engaged in full-screen viewing behavior showed a notable increase in both watch-time and segments played. This implies a more meticulous analysis, although only the particular type of full-screen interaction showed a weak significant correlation with the count of matched events as the outcome variable, suggesting that full-screen viewing behavior may be beneficial in discovering relevant classroom events. Moreover, full-screen mode might be more engaging, as there was a moderate relationship with the video progress.
The count of events that students observed as relevant for classroom management was a consistent predictor of our outcome variable across all clips (see Table 9). The more events a student coded, the more events matched with the experts’ rating of events. This means that a consistent set of their observations matched those of the experts across all clips, and that as the number of coded events increased, the rate of matching events remained constant.
Beyond this observation, only clip 3 showed further considerably significant correlations of process variables with outcomes. This might be explained by the fact that the conditions for working on the task were different for clip 3 than clips 1 and 2. While the latter were analyzed at home in a fully self-regulated learning environment, the assignment for clip 3 was conducted within a regular seminar session. Although both the given reference time for each analysis and the intended lack of assistance from the lecturer (faded-out learning support) were identical as contextual conditions, the process variables of the setting that were regulated more by external circumstances, corresponded more clearly to our assumptions in comparison to the setting with no supportive regulation. This suggests a different way of working, such as due to the greater freedom in fully self-regulated environments. Several factors can distract students, compared to attending a regular seminar (e.g. their private schedule, other duties, or time spent or set). By triangulating additional data in the future, these kinds of relationships seem worthwhile to explore.
To examine what distinguishes successful from less successful video analysis strategies, our fourth hypothesis stated that more meticulous video-based analyses relate to a greater agreement between students’ ratings and the experts’ rating. Within our dataset, we could not confirm that a more meticulous video-based analysis leads to a greater agreement between the ratings of students and experts, as no significant correlation was revealed between the two clusters and students’ matched events with the experts’ ratings (see Table 10). Students with a meticulous analysis of the video clip did not display significantly more matched events than students with a less meticulous analysis. This resulted for all three video clips. However, the possibility of an intended process-based diagnostics still seems a thoroughly plausible addition to this approach, as we found positive but non-significant correlations. Furthermore, findings from other contexts suggest, that patterns of greater engagement are positively associated with learning outcomes (Soffer and Cohen, 2019; Wang et al., 2021) or that a more passive engagement is insufficient for learning (Koedinger et al., 2015).
Investigating the well-separated clusters of meticulous and less meticulous analysts on a feature basis, we found significant differences in various process variables, characterizing students’ approaches (see Table 11). Less meticulous analysts lacked revisions in annotations, visited learning activities less frequently, exhibited reduced overall engagement, and viewed the video solely to the extent required. The application of Learning Analytics reveals whether students examine classroom videos with meticulousness or lack thereof.
5 Conclusion
In summary, our research highlighted that behavioral data derived from learning processes using Learning Analytics can provide valuable evidence of students’ utilization of analysis strategies in such video-based annotation assignments. It was shown to what extent analysis strategies differed, and that students individually exhibited more meticulous as well as less meticulous proceedings during their analyses. The presence of indications suggesting a potential link between a meticulous approach and a greater quality of analysis does not yield conclusive evidence across all videos. It is plausible that there are alternative process variables that hold greater predictive value regarding our chosen outcome variable. Promising further variables could be user-generated or qualitatively determined data that go beyond pure interaction behavior and better represent interrelationships.
As video-based assignments are a popular method for acquiring professional vision, and are frequently used in teacher training, there is a considerable potential for adapting Learning Analytics to gain more insights into the process of analysis and its relation to outcomes. Prerequisites include the use of a video annotation tool and an e-learning standard, in addition to a standardized content structure. They help adapt the method suggested in the didactic design of video-based assignments.
Therefore, the findings of this study have implications for the design and implementation of video-based assignments in courses to promote professional vision. This study sets a prerequisite for the broader goal of creating and establishing an adaptive learning environment, including individual feedback and learning support. Exploring different approaches to analyzing classroom videos to promote a professional vision using a learning analytic approach provides opportunities for a wide range of application perspectives that become conceivable. Gained knowledge of process-related behaviors enables the implementation of learning support that adapts to the needs of learners’ analyzing the classroom video, such as providing individual feedback and visual cueing to support developing the ability to notice and interpret classroom events relevant to learning.
Accordingly, we attempted to gain insights into the learning processes involved in developing professional vision by using a digital video annotation tool. Understanding the strategies of video-based learning and distinguishing between successful and less successful strategies will help to create a learning environment that aligns with the students’ behavior and pacing displayed during the tasks. Adaptive learning support can be provided, such as cues to take a closer look at a previously neglected video segment or consider overlooked categories for a section, addressing the increasing need to provide more personalized content in e-learning (Sinha et al., 2014). This enhances the learning experience in blended learning formats, where the asynchronous phases often lack individual support and feedback. The discovery of behavioral patterns within the data might serve as a foundation for developing adaptive features in the future. With the heatmap data and knowledge of important video segments, students’ attention could be focused on areas of interest, supporting the analysis with visual cues within the classroom videos. Our article offers new perspectives in the field of research related to professional vision and contributes a starting point for further studies, as reported indicators of video-based strategies could be used for predictive analytics of learning outcomes and process-based learning diagnostics for developing the ability to notice and interpret classroom events.
Data availability statement
The raw data supporting the conclusions of this article will be made available by the authors, without undue reservation.
Ethics statement
Ethical approval was not required for the studies involving humans because the aims of the research to improve educational practices did not pose a risk to participants, their rights, or interfere with students’ academic progress, as the data were collected in compliance with the law, privacy and data policies, using a pseudonymous identifier and aggregated to anonymize the data. In addition, no sensitive data was collected for this study. All students were adults and therefore able to consent to participate in the study. The studies were conducted in accordance with the local legislation and institutional requirements. The participants provided their written informed consent to participate in this study.
Author contributions
MO: Conceptualization, Data curation, Formal analysis, Investigation, Methodology, Project administration, Resources, Software, Supervision, Validation, Visualization, Writing—original draft, Writing—review and editing. RJ: Writing—review and editing. MH: Conceptualization, Data curation, Formal analysis, Methodology, Project administration, Supervision, Validation, Writing—review and editing.
Funding
The author(s) declare that no financial support was received for the research, authorship, and/or publication of this article.
Acknowledgments
Brian Bloch for the linguistic review. We would particularly like to thank Sabrina Konjer, Christina Dückers (born Gippert), and Philip Hörter who were involved in the original design and implementation of the seminar sessions.
Conflict of interest
The authors declare that the research was conducted in the absence of any commercial or financial relationships that could be construed as a potential conflict of interest.
Publisher’s note
All claims expressed in this article are solely those of the authors and do not necessarily represent those of their affiliated organizations, or those of the publisher, the editors and the reviewers. Any product that may be evaluated in this article, or claim that may be made by its manufacturer, is not guaranteed or endorsed by the publisher.
References
ADL (ed.). (2017). Part One: About the Experience API. Available online at: https://github.com/adlnet/xAPI-Spec/blob/IEEE/xAPI-About.md (archive: https://web.archive.org/web/20230908093130if_/https://raw.githubusercontent.com/adlnet/xAPI-Spec/IEEE/xAPI-About.md) (accessed September 30, 2023).
ADL (ed.). (2019). Part Two: Experience API Data. Available online at: https://github.com/adlnet/xAPI-Spec/blob/IEEE/xAPI-Data.md (archive: https://web.archive.org/web/20230908093201if_/https://raw.githubusercontent.com/adlnet/xAPI-Spec/IEEE/xAPI-Data.md) (accessed September 30, 2023).
ADL (ed.). (2023). xAPI Profile Server. Available online at: https://profiles.adlnet.gov/ (accessed September 30, 2023).
Ahmad, A., Schneider, J., Griffiths, D., Biedermann, D., Schiffner, D., Greller, W., et al. (2022). Connecting the dots – A literature review on learning analytics indicators from a learning design perspective. J. Comput. Assist. Learn. doi: 10.1111/jcal.12716
Al-Qahtani, A. A., and Higgins, S. E. (2013). Effects of traditional, blended and e-learning on students’ achievement in higher education. J. Comput. Assist. Learn. 29, 220–234. doi: 10.1111/j.1365-2729.2012.00490.x
Angrave, L., Zhang, Z., Henricks, G., and Mahipal, C. (2020). “Who benefits?,” in Proceedings of the 51st ACM Technical Symposium on Computer Science Education, eds J. Zhang, M. Sherriff, S. Heckman, P. Cutter, and A. Monge (New York, NY: ACM), 1193–1199. doi: 10.1145/3328778.3366953
Ardley, J., and Hallare, M. (2020). The feedback cycle: Lessons learned with video annotation software during student teaching. J. Educ. Technol. Syst. 49, 94–112. doi: 10.1177/0047239520912343
Ardley, J., and Johnson, J. (2019). Video annotation software in teacher education: Researching University supervisor’s perspective of a 21st-Century Technology. J. Educ. Technol. Syst. 47, 479–499. doi: 10.1177/0047239518812715
Atapattu, T., and Falkner, K. (2018). Impact of lecturer’s discourse for students’ video engagement: Video learning analytics case study of MOOCs. J. Learn. Anal. 5, 182–197. doi: 10.18608/jla.2018.53.12
Baier, F., Maurer, C., Dignath, C., and Kunter, M. (2021). Fostering pre-service teachers’ theoretical knowledge application. Studying with and without text-based cases. Instruct. Sci. 49, 855–876.
Baker, S., Field, C., Lee, J. S., and Saintilan, N. (2021). Supporting students’ academic literacies in post-COVID-19 times: Developing digital videos to develop students’ critical academic reading practices. J. Univ. Teach. Learn. Pract. 18, 35–49. doi: 10.53761/1.18.4.5
Barba, P. G., de Kennedy, G. E., and Ainley, M. D. (2016). The role of students’ motivation and participation in predicting performance in a MOOC. J. Comput. Assist. Learn. 32, 218–231. doi: 10.1111/jcal.12130
Barnhart, T., and van Es, E. (2015). Studying teacher noticing: Examining the relationship among pre-service science teachers’ ability to attend, analyze and respond to student thinking. Teach. Teach. Educ. 45, 83–93. doi: 10.1016/j.tate.2014.09.005
Barth, V. L. (2017). Professionelle Wahrnehmung von Störungen im Unterricht. Berlin: Springer Fachmedien Wiesbaden. doi: 10.1007/978-3-658-16371-6
Berliner, D. C. (2001). Learning about and learning from expert teachers. Int. J. Educ. Res. 35, 463–482. doi: 10.1016/S0883-0355(02)00004-6
Blau, I., and Shamir-Inbal, T. (2021). Writing private and shared annotations and lurking in Annoto hyper-video in academia: Insights from learning analytics, content analysis, and interviews with lecturers and students. Educ. Technol. Res. Dev. 69, 763–786. doi: 10.1007/s11423-021-09984-5
Blomberg, G., Stürmer, K., and Seidel, T. (2011). How pre-service teachers observe teaching on video: Effects of viewers’ teaching subjects and the subject of the video. Teach. Teach. Educ. 27, 1131–1140. doi: 10.1016/j.tate.2011.04.008
Blömeke, S., Busse, A., Kaiser, G., König, J., and Suhl, U. (2016). The relation between content-specific and general teacher knowledge and skills. Teach. Teach. Educ. 56, 35–46. doi: 10.1016/j.tate.2016.02.003
Blömeke, S., Gustafsson, J. E., and Shavelson, R. J. (2015). Beyond dichotomies: Competence viewed as a continuum. Z. Psychol. 223, 3–13. doi: 10.1027/2151-2604/a000194
Blömeke, S., Jentsch, A., Ross, N., Kaiser, G., and König, J. (2022). Opening up the black box: Teacher competence, instructional quality, and students’ learning progress. Learn. Instruct. 79:101600. doi: 10.1016/j.learninstruc.2022.101600
Brinton, C. G., Buccapatnam, S., Chiang, M., and Poor, H. V. (2016). Mining MOOC Clickstreams: Video-watching behavior vs. in-video quiz performance. IEEE Trans. Signal Process. 64, 3677–3692. doi: 10.1109/TSP.2016.2546228
Brooks, C., Epp, C. D., Logan, G., and Greer, J. (2011). “The who, what, when, and why of lecture capture,” in Proceedings of the 1st International Conference on Learning Analytics and Knowledge, eds P. Long, G. Siemens, G. Conole, and D. Gašević (New York, NY: ACM), 86–92. doi: 10.1145/2090116.2090128
Brown, E. (2018). xAPI Registry. Available online at: https://xapi.com/registry/ (accessed September 30, 2023).
Carter, K., Cushing, K., Sabers, D., Stein, P., and Berliner, D. (1988). Expert-novice differences in perceiving and processing visual classroom information. J. Teach. Educ. 39, 25–31. doi: 10.1177/002248718803900306
Castro, A., Clark, K., Jacobs, J., and Givvin, K. (2005). Response to theory & practice question: Using video to support teacher learning. Assoc. Math. Teach. Educ. Connect. 14, 8–12.
Catherine, C., Lam, N., and Habil, H. (2021). The use of video annotation in education: A review. Asian J. Univ. Educ. 17:84. doi: 10.24191/ajue.v17i4.16208
Chatti, M. A., Dyckhoff, A. L., Schroeder, U., and Thüs, H. (2012). A reference model for learning analytics. Int. J. Technol. Enhanc. Learn. 4, 318–331. doi: 10.1504/IJTEL.2012.051815
Chen, P. S. D., Lambert, A. D., and Guidry, K. R. (2010). Engaging online learners: The impact of Web-based learning technology on college student engagement. Comput. Educ. 54, 1222–1232. doi: 10.1016/j.compedu.2009.11.008
Chi, M. T. H., and Wylie, R. (2014). The ICAP Framework: Linking cognitive engagement to active learning outcomes. Educ. Psychol. 49, 219–243. doi: 10.1080/00461520.2014.965823
Colasante, M. (2011). Using video annotation to reflect on and evaluate physical education pre-service teaching practice. Aust. J. Educ. Technol. 27, 66–88. doi: 10.14742/ajet.983
Collins, A., Brown, J. S., and Newman, S. E. (1989). “Cognitive apprenticeship: Teaching the crafts of reading, writing, and mathematics,” in Knowing, Learning, and Instruction: Essays in Honor of Robert Glaser, ed. L. B. Resnick (Mahwah, NJ: Lawrence Erlbaum Associates Publishers), 453–494. doi: 10.1016/j.nedt.2006.02.010
Córcoles, C., Cobo, G., and Guerrero-Roldán, A. -E. (2021). The usefulness of video learning analytics in small scale E-learning scenarios. Appl. Sci. 11:10366. doi: 10.3390/app112110366
Costley, J., Fanguy, M., Lange, C., and Baldwin, M. (2021). The effects of video lecture viewing strategies on cognitive load. J. Comput. High. Educ. 33, 19–38. doi: 10.1007/s12528-020-09254-y
Delen, E., Liew, J., and Willson, V. (2014). Effects of interactivity and instructional scaffolding on learning: Self-regulation in online video-based environments. Comput. Educ. 78, 312–320. doi: 10.1016/j.compedu.2014.06.018
Doyle, W. (1977). Learning the classroom environment: An ecological analysis. J. Teach. Educ. 28, 51–55. doi: 10.1177/002248717702800616
Emmer, E. T., and Stough, L. M. (2001). Classroom management: A critical part of educational psychology, with implications for teacher education. Educ. Psychol. 36, 103–112. doi: 10.1207/S15326985EP3602_5
Evertson, C. M., and Emmer, E. T. (2013). Classroom Management for Elementary Teachers, 9. Edn. London: Pearson.
Gašević, D., Dawson, S., Rogers, T., and Gasevic, D. (2016). Learning analytics should not promote one size fits all: The effects of instructional conditions in predicting academic success. Internet High. Educ. 28, 68–84. doi: 10.1016/j.iheduc.2015.10.002
Gaudin, C., and Chaliès, S. (2015). Video viewing in teacher education and professional development: A literature review. Educ. Res. Rev. 16, 41–67. doi: 10.1016/j.edurev.2015.06.001
Gegenfurtner, A., Lewalter, D., Lehtinen, E., Schmidt, M., and Gruber, H. (2020). Teacher expertise and professional vision: Examining knowledge-based reasoning of pre-service teachers, in-service teachers, and school principals. Front. Educ. 5:59. doi: 10.3389/feduc.2020.00059
Gegenfurtner, A., and Stahnke, R. (2023). Teacher Professional Vision: Theoretical and Methodological Advances. London: Routledge.
Giannakos, M. N., Chorianopoulos, K., and Chrisochoides, N. (2015). Making sense of video analytics: Lessons learned from clickstream interactions, attitudes, and learning outcome in a video-assisted course. Int. Rev. Res. Open Distribut. Learn. 16, 260–283. doi: 10.19173/irrodl.v16i1.1976
Gippert, C., Gold, B., Seeger, D., Junker, R., and Holodynski, M. (2019). Manual zur theoriegeleiteten Interpretation klassenführungsrelevanter Unterrichtsereignisse. Institut für Psychologie in Bildung und Erziehung, Universität Münster. Available online at: https://go.wwu.de/dthla (accessed September 30, 2023).
Gippert, C., Hörter, P., Junker, R., and Holodynski, M. (2022). Professional vision of teaching as a focus-specific or focus-integrated skill – Conceptual considerations and video-based assessment. Teach. Teach. Educ. 117:103797. doi: 10.1016/j.tate.2022.103797
Gold, B., and Holodynski, M. (2017). Using digital video to measure the professional vision of elementary classroom management: Test validation and methodological challenges. Comput. Educ. 107, 13–30. doi: 10.1016/j.compedu.2016.12.012
Gold, B., Junker, R., Wissemann, M., Klassen, C., and Holodynski, M. (2021). Are good observers good classroom managers? The relationship between teachers’ professional vision and their students’ ratings on classroom management. Int. J. Educ. Res. 109:101811. doi: 10.1016/j.ijer.2021.101811
Gold, B., Müller, M., Weber, K., and Prilop, C. (2023). “Characteristics and validation of video-based standardized instruments measuring professional vision – a closer look at the predictive validity of different task formats,” in Teacher Professional Vision: Theoretical and Methodological Advances, eds A. Gegenfurtner and R. Stahnke (London: Routledge).
Gold, B., Pfirrmann, C., and Holodynski, M. (2020). Promoting professional vision of classroom management through different analytic perspectives in video-based learning environments. J. Teach. Educ. 72, 431–447. doi: 10.1177/0022487120963681
Goodwin, C. (1994). Professional vision. Am. Anthropol. 96, 606–633. doi: 10.1525/aa.1994.96.3.02a00100
Guo, P. J., Kim, J., and Rubin, R. (2014). “How video production affects student engagement,” in Proceedings of the First ACM conference on Learning @ scale conference, eds M. Sahami, A. Fox, M. A. Hearst, and M. T. Chi (NewYork, NY: ACM), 41–50. doi: 10.1145/2556325.2566239
Hattie, J. (2023). Visible Learning: The Sequel; a Synthesis of Over 2,100 Meta-Analyses Relating to Achievement, 1st Edn. London: Routledge.
Hoppe, H. U. (2017). “Computational methods for the analysis of learning and knowledge building communities,” in Handbook of Learning Analytics, eds C. Lang, G. Siemens, A. Wise, and D. Gasevic (Edmonton, AB: Society for Learning Analytics Research (SoLAR)), 23–33. doi: 10.18608/hla17.002
Hörter, P., Gippert, C., Holodynski, M., and Stein, M. (2020). Klassenführung und Fachdidaktik im (Anfangs-)Unterricht Mathematik erfolgreich integrieren – Konzeption einer videobasierten Lehrveranstaltung zur Förderung der professionellen Unterrichtswahrnehmung. Herausforderung Lehrer*innenbildung – Zeitschrift Zur Konzeption, Gestaltung Und Diskussion 3, 256–282. doi: 10.4119/hlz-2551
Hu, H., Zhang, G., Gao, W., and Wang, M. (2020). Big data analytics for MOOC video watching behavior based on Spark. Neural Comput. Applicat. 32, 6481–6489. doi: 10.1007/s00521-018-03983-z
Huang, Y., Miller, K. F., Cortina, K. S., and Richter, D. (2023). Teachers’ professional vision in action. Z. Pädagogische Psychol. 37, 122–139. doi: 10.1024/1010-0652/a000313
IEEE (2023). IEEE Approved Draft Standard for Learning Technology - JavaScript Object Notation (JSON) Data Model Format and Representational State Transfer (RESTful) Web Service for Learner Experience Data Tracking and Access. Available online at: https://ieeexplore.ieee.org/servlet/opac?punumber=10040586 (accessed September 30, 2023).
Jones, V. (1996). “Classroom management,” in Handbook of Research on Teacher Education: A Project of the Association of Teacher Educators, eds J. P. Sikula, T. Buttery, and E. Guyton (London: Macmillan Library Reference USA), 503–521.
Junker, R., Gold, B., and Holodynski, M. (2021). Classroom management of pre-service and beginning teachers: From dispositions to performance. Int. J. Modern Educ. Stud. 5, 339–363. doi: 10.51383/ijonmes.2021.137
Junker, R., Oellers, M., Konjer, S., Rauterberg, T., Zucker, V., Meschede, N., et al. (2022a). “Das Meta-Videoportal unterrichtsvideos.net für die Lehrkräftebildung,” in Lehren und Forschen mit Videos in der Lehrkräftebildung, eds R. Junker, V. Zucker, M. Oellers, T. Rauterberg, S. Konjer, N. Meschede, et al. (München: Waxmann), 181–195.
Junker, R., Rauterberg, T. Möller, K., and Holodynski, M. (2020). Videobasierte Lehrmodule zur Förderung der professionellen Wahrnehmung von heterogenitätssensiblem Unterricht. Herausforderung Lehrer*innenbildung – Zeitschrift Zur Konzeption, Gestaltung Und Diskussion, 3, 236–255. doi: 10.4119/HLZ-2554
Junker, R., Rauterberg, T., Zucker, V., Meschede, N., and Holodynski, M. (2022b). “Das videoportal provision: Umgang mit heterogenität im unterricht professionell wahrnehmen,” in Lehren und Forschen mit Videos in der Lehrkräftebildung, eds R. Junker, V. Zucker, M. Oellers, T. Rauterberg, S. Konjer, N. Meschede, et al. (München: Waxmann), 165–180.
Junker, R., Zucker, V., Oellers, M., Rauterberg, T., Konjer, S., Meschede, N., et al. (2022c). Lehren und Forschen mit Videos in der Lehrkräftebildung. München: Waxmann.
Kaiser, G., Busse, A., Hoth, J., König, J., and Blömeke, S. (2015). About the complexities of video-based assessments: Theoretical and methodological approaches to overcoming shortcomings of research on teachers’ competence. Int. J. Sci. Math. Educ. 13, 369–387. doi: 10.1007/s10763-015-9616-7
Kalyuga, S. (2009). Managing Cognitive Load in Adaptive Multimedia Learning. Hershey, PA: IGI Global. doi: 10.4018/978-1-60566-048-6
Kersting, N. B., Givvin, K. B., Thompson, B. J., Santagata, R., and Stigler, J. W. (2012). Measuring usable knowledge. Am. Educ. Res. J. 49, 568–589. doi: 10.3102/0002831212437853
Khalil, M., Topali, P., Ortega-Arranz, A., Er, E., Akçapınar, G., and Belokrys, G. (2023). Video analytics in digital learning environments: Exploring student behaviour across different learning contexts. Technol. Knowl. Learn. doi: 10.1007/s10758-023-09680-8
Kim, J., Guo, P. J., Seaton, D. T., Mitros, P., Gajos, K. Z., and Miller, R. C. (2014). “Understanding in-video dropouts and interaction peaks inonline lecture videos,” in Proceedings of the first ACM conference on Learning @ scale conference, eds M. Sahami, A. Fox, M. A. Hearst, and M. T. Chi (NewYork, NY: ACM), 31–40. doi: 10.1145/2556325.2566237
Kizilcec, R. F., Piech, C., and Schneider, E. (2013). “Deconstructing disengagement,” in Proceedings of the Third International Conference on Learning Analytics and Knowledge, eds D. Suthers, K. Verbert, E. Duval, and X. Ochoa (NewYork, NY: ACM), 170–179. doi: 10.1145/2460296.2460330
Kleftodimos, A., and Evangelidis, G. (2016). Using open source technologies and open internet resources for building an interactive video based learning environment that supports learning analytics. Smart Learn. Environ. 3:9. doi: 10.1186/s40561-016-0032-4
Knight, S., and Buckingham Shum, S. (2017). “Theory and learning analytics,” in Handbook of Learning Analytics, eds C. Lang, G. Siemens, A. Wise, and D. Gasevic (Beaumont, AB: Society for Learning Analytics Research (SoLAR)), 17–22. doi: 10.18608/hla17.001
Koedinger, K. R., Kim, J., Jia, J. Z., McLaughlin, E. A., and Bier, N. L. (2015). “Learning is not a spectator sport,” in Proceedings of the Second (2015) ACM Conference on Learning @ Scale, eds G. Kiczales, D. M. Russell, and B. Woolf (NewYork, NY: ACM), 111–120. doi: 10.1145/2724660.2724681
König, J. (2015). Measuring classroom management expertise (CME) of teachers: A video-based assessment approach and statistical results. Cogent Educ. 2:991178. doi: 10.1080/2331186X.2014.991178
König, J., Blömeke, S., Jentsch, A., Schlesinger, L., née Nehls, C. F., Musekamp, F., et al. (2021). The links between pedagogical competence, instructional quality, and mathematics achievement in the lower secondary classroom. Educ. Stud. Math. 107, 189–212. doi: 10.1007/s10649-020-10021-0
König, J., Blömeke, S., Klein, P., Suhl, U., Busse, A., and Kaiser, G. (2014). Is teachers’ general pedagogical knowledge a premise for noticing and interpreting classroom situations? A video-based assessment approach. Teach. Teach. Educ. 38, 76–88. doi: 10.1016/j.tate.2013.11.004
König, J., and Kramer, C. (2016). Teacher professional knowledge and classroom management: On the relation of general pedagogical knowledge (GPK) and classroom management expertise (CME). ZDM 48, 139–151. doi: 10.1007/s11858-015-0705-4
König, J., Santagata, R., Scheiner, T., Adleff, A. -K., Yang, X., and Kaiser, G. (2022). Teacher noticing: A systematic literature review of conceptualizations, research designs, and findings on learning to notice. Educ. Res. Rev. 36:100453. doi: 10.1016/j.edurev.2022.100453
Koschel, W. (2021). Zur Förderung professioneller Unterrichtswahrnehmung im Kontext von Heterogenität im Unterricht: Eine Interventionsstudie am Beispiel des beruflichen Lehramtsstudiums. Opladen: Budrich Academic Press. doi: 10.3224/96665036
Kosel, C., Mooseder, A., Seidel, T., and Pfeffer, J. (2023). Measuring teachers’ visual expertise using the gaze relational index based on real-world eye-tracking data and varying velocity thresholds. arXiv [preprint]. doi: 10.48550/arXiv.2304.05143
Kounin, J. S. (1970). Discipline and Group Management in Classroom. NewYork, NY: Holt, Rinehart & Winston.
Kunter, M., Baumert, J., and Köller, O. (2007). Effective classroom management and the development of subject-related interest. Learn. Instruct. 17, 494–509. doi: 10.1016/j.learninstruc.2007.09.002
Kunter, M., Klusmann, U., Baumert, J., Richter, D., Voss, T., and Hachfeld, A. (2013). Professional competence of teachers: Effects on instructional quality and student development. J. Educ. Psychol. 105, 805–820. doi: 10.1037/a0032583
Lan, A. S., Brinton, C. G., Yang, T. Y., and Mung Chiang. (2017). “Behavior-based latent variable model for learner engagement,” in Proceedings of the 10th International Conference on Educational Data Mining, eds X. Hu, T. Barnes, A. Hershkovitz, and L. Paquette, (Wuhan).
Lang, D., Chen, G., Mirzaei, K., and Paepcke, A. (2020). “Is faster better?,” in Proceedings of the Tenth International Conference on Learning Analytics & Knowledge, eds C. Rensing, H. Drachsler, V. Kovanović, N. Pinkwart, M. Scheffel, and K. Verbert (NewYork, NY: ACM), 260–269. doi: 10.1145/3375462.3375466
Larison, S., Richards, J., and Sherin, M. G. (2022). Tools for supporting teacher noticing about classroom video in online professional development. J. Math. Teach. Educ. doi: 10.1007/s10857-022-09554-3
Li, N., Kidzinski, L., Jermann, P., and Dillenbourg, P. (2005). “How do in-video interactions refect perceived video difculty?,” in Proceedings of the European MOOCs stakeholder summit 2015 (EPFL-CONF-207968), (Ludhiana: PAU Education), 112–121.
Li, N., Kidziński, Ł, Jermann, P., and Dillenbourg, P. (2015). “MOOC Video Interaction Patterns: What Do They Tell Us?,” in Lecture Notes in Computer Science. Design for Teaching and Learning in a Networked World, Vol. 9307, eds G. Conole, T. Klobuèar, C. Rensing, J. Konert, and E. Lavoué (Cham: Springer International Publishing), 197–210. doi: 10.1007/978-3-319-24258-3_15
Long, P., Siemens, G., Conole, G., and Gašević, D. (2011). “Message from the LAK 2011 General & Program Chairs,” in Proceedings of the 1st International Conference on Learning Analytics and Knowledge, eds P. Long, G. Siemens, G. Conole, and D. Gašević (NewYork, NY: ACM).
Lust, G., Elen, J., and Clarebout, G. (2013). Regulation of tool-use within a blended course: Student differences and performance effects. Comput. Educ. 60, 385–395. doi: 10.1016/j.compedu.2012.09.001
Lust, G., Vandewaetere, M., Ceulemans, E., Elen, J., and Clarebout, G. (2011). Tool-use in a blended undergraduate course: In Search of user profiles. Comput. Educ. 57, 2135–2144. doi: 10.1016/j.compedu.2011.05.010
Manasrah, A., Masoud, M., and Jaradat, Y. (2021). “Short videos, or long videos? A study on the ideal video length in online learning,” in Proceedings of the2021 International Conference on Information Technology (ICIT), (Amman: IEEE), 366–370. doi: 10.1109/ICIT52682.2021.9491115
Mayer, R. E. (2014). “Cognitive Theory of Multimedia Learning,” in The Cambridge Handbook of Multimedia Learning, ed. R. E. Mayer (Cambridge: Cambridge University Press).
Mayer, R. E., and Fiorella, L. (2014). “Principles for reducing extraneous processing in multimedia learning: Coherence, signaling, redundancy, spatial contiguity, and temporal contiguity principles,” in The Cambridge Handbook of Multimedia Learning, ed. R. E. Mayer (Cambridge: Cambridge University Press), 279–315. doi: 10.1017/CBO9781139547369.015
McFadden, J., Ellis, J., Anwar, T., and Roehrig, G. (2014). Beginning science teachers’ use of a digital video annotation tool to promote reflective practices. J. Sci. Educ. Technol. 23, 458–470. doi: 10.1007/s10956-013-9476-2
Merkt, M., and Schwan, S. (2014). How does interactivity in videos affect task performance? Comput. Hum. Behav. 31, 172–181. doi: 10.1016/j.chb.2013.10.018
Meschede, N., Fiebranz, A., Möller, K., and Steffensky, M. (2017). Teachers’ professional vision, pedagogical content knowledge and beliefs: On its relation and differences between pre-service and in-service teachers. Teach. Teach. Educ. 66, 158–170. doi: 10.1016/j.tate.2017.04.010
Mirriahi, N., Jovanovic, J., Dawson, S., Gašević, D., and Pardo, A. (2018). Identifying engagement patterns with video annotation activities: A case study in professional development. Aust. J. Educ. Technol. 34, 57–72. doi: 10.14742/ajet.3207
Mirriahi, N., Liaqat, D., Dawson, S., and Gašević, D. (2016). Uncovering student learning profiles with a video annotation tool: Reflective learning with and without instructional norms. Educ. Technol. Res. Dev. 64, 1083–1106. doi: 10.1007/s11423-016-9449-2
Mirriahi, N., and Vigentini, L. (2017). “Analytics of learner video use,” in Handbook of Learning Analytics, eds C. Lang, G. Siemens, A. Wise, and D. Gasevic (Beaumont, AB: Society for Learning Analytics Research (SoLAR)), 251–267. doi: 10.18608/hla17.022
Mubarak, A. A., Cao, H., Zhang, W., and Zhang, W. (2021). Visual analytics of video-clickstream data and prediction of learners’ performance using deep learning models in MOOCs’ courses. Comput. Appl. Eng. Educ. 29, 710–732. doi: 10.1002/cae.22328
Nilsson, P., and Karlsson, G. (2019). Capturing student teachers’ pedagogical content knowledge (PCK) using CoRes and digital technology. Int. J. Sci. Educ. 41, 419–447. doi: 10.1080/09500693.2018.1551642
Owston, R., Lupshenyuk, D., and Wideman, H. (2011). Lecture capture in large undergraduate classes: Student perceptions and academic performance. Internet High. Educ. 14, 262–268. doi: 10.1016/j.iheduc.2011.05.006
Patel, B., Yook, G., Mislan, S., and Persky, A. M. (2019). Exploring the consequences on memory of students who know they have access to recorded lectures. Am. J. Pharm. Educ. 83:6958. doi: 10.5688/ajpe6958
Praetorius, A. K., Klieme, E., Herbert, B., and Pinger, P. (2018). Generic dimensions of teaching quality: The German framework of Three Basic Dimensions. ZDM 50, 407–426. doi: 10.1007/s11858-018-0918-4
Prilop, C. N., Weber, K. E., and Kleinknecht, M. (2021). The role of expert feedback in the development of pre-service teachers’ professional vision of classroom management in an online blended learning environment. Teaching Teach. Educ. 99:103276. doi: 10.1016/j.tate.2020.103276
ProVision. (2023). Geographie - 5. Klasse - Landwirtschaft ernährt uns - Stunde 6 (Klassenperspektive). Universität Münster. Available online at: https://www.uni-muenster.de/ProVision/video/#GEO_K5_LK1_1ES_LAN_KF (accessed September 30, 2023).
Punj, G., and Stewart, D. W. (1983). Cluster analysis in marketing research: Review and suggestions for application. J. Mark. Res. 20:134. doi: 10.2307/3151680
Rich, P. J., and Hannafin, M. (2008). Video annotation tools: Technologies to scaffold, structure, and transform teacher reflection. J. Teach. Educ. 60, 52–67. doi: 10.1177/0022487108328486
Rich, P. J., and Trip, T. (2011). Ten essential questions educators should ask when using video annotation tools. TechTrends 55, 16–24. doi: 10.1007/s11528-011-0537-1
Risko, E. F., Foulsham, T., Dawson, S., and Kingstone, A. (2013). The collaborative lecture annotation system (CLAS): A new TOOL for distributed learning. IEEE Trans. Learn. Technol. 6, 4–13. doi: 10.1109/TLT.2012.15
Roll, I., and Winne, P. H. (2015). Understanding, evaluating, and supporting self-regulated learning using learning analytics. J. Learn. Anal. 2, 7–12. doi: 10.18608/jla.2015.21.2
Roth, K. J., Garnier, H. E., Chen, C., Lemmens, M., Schwille, K., and Wickler, N. I. (2011). Videobased lesson analysis: Effective science PD for teacher and student learning. J. Res. Sci. Teach. 48, 117–148. doi: 10.1002/tea.20408
Santagata, R., and Angelici, G. (2010). Studying the impact of the lesson analysis framework on preservice teachers’ abilities to reflect on videos of classroom teaching. J. Teach. Educ. 61, 339–349. doi: 10.1177/0022487110369555
Santagata, R., and Guarino, J. (2011). Using video to teach future teachers to learn from teaching. ZDM 43, 133–145. doi: 10.1007/s11858-010-0292-3
Santagata, R., König, J., Scheiner, T., Nguyen, H., Adleff, A. K., Yang, X., et al. (2021). Mathematics teacher learning to notice: A systematic review of studies of video-based programs. ZDM 53, 119–134. doi: 10.1007/s11858-020-01216-z
Schwan, S., and Riempp, R. (2004). The cognitive benefits of interactive videos: Learning to tie nautical knots. Learn. Instruct. 14, 293–305. doi: 10.1016/j.learninstruc.2004.06.005
Seidel, T., Schnitzler, K., Kosel, C., Stürmer, K., and Holzberger, D. (2021). Student characteristics in the eyes of teachers: Differences between novice and expert teachers in judgment accuracy, observed behavioral cues, and gaze. Educ. Psychol. Rev. 33, 69–89. doi: 10.1007/s10648-020-09532-2
Seidel, T., and Shavelson, R. J. (2007). Teaching effectiveness research in the past decade: The role of theory and research design in disentangling meta-analysis results. Rev. Educ. Res. 77, 454–499. doi: 10.3102/0034654307310317
Seidel, T., and Stürmer, K. (2014). Modeling and measuring the structure of professional vision in preservice teachers. Am. Educ. Res. J. 51, 739–771. doi: 10.3102/0002831214531321
Sherin, M. (2001). “Developing a professional vision of classroom events,” in Studies in Mathematical Thinking and Learning. Beyond Classical Pedagogy: Teaching Elementary School Mathematics, eds T. Wood, B. S. Nelson, and J. Warfield (London: Routledge), 75–93.
Sherin, M. (2007). “The development of teachers’ professional vision in video clubs,” in Video Research in the Learning, eds R. Goldman, R. Pea, B. Barron, and S. Derry (Mahwah, NJ: Erlbaum), 383–395.
Sherin, M., and van Es, E. A. (2005). Using video to support teachers’ ability to notice classroom interactions. J. Technol. Teach. Educ. 13, 475–491.
Sherin, M., and van Es, E. A. (2009). Effects of video club participation on teachers’ professional vision. J. Teach. Educ. 60, 20–37. doi: 10.1177/0022487108328155
Shulman, L. (1987). Knowledge and teaching: Foundations of the new reform. Harvard Educ. Rev. 57, 1–23. doi: 10.17763/haer.57.1.j463w79r56455411
Siemens, G. (2013). Learning analytics: The emerge of a discipline. Am. Behav. Sci. 57, 1380–1400. doi: 10.1177/0002764213498851
Siemens, G., and Baker, R. S. J. D. (2012). “Learning analytics and educational data mining: Towards communication and collaboration,” in Proceedings of the 2nd International Conference on Learning Analytics and Knowledge, eds S. Dawson, C. Haythornthwaite, S. Buckingham Shum, D. Gasevic, and R. Ferguson (NewYork, NY: ACM), 252–254. doi: 10.1145/2330601.2330661
Simonsen, B., Fairbanks, S., Briesch, A., Myers, D., and Sugai, G. (2008). Evidence-based practices in classroom management: Considerations for research to practice. Educ. Treat. Child. 31, 351–380. doi: 10.1353/etc.0.0007
Sinha, T., Jermann, P., Li, N., and Dillenbourg, P. (2014). “Your click decides your fate: Inferring information processing and attrition behavior from MOOC video clickstream interactions,” in Proceedings of the EMNLP 2014 Workshop on Analysis of Large Scale Social Interaction in MOOCs, eds C. Rose and G. Siemens (Stroudsburg, PA: Association for Computational Linguistics), 3–14. doi: 10.3115/v1/W14-4102
Smidt, E., and Hegelheimer, V. (2004). Effects of online academic lectures on esl listening comprehension, incidental vocabulary acquisition, and strategy use. Comput. Assist. Lang. Learn. 17, 517–556. doi: 10.1080/0958822042000319692
Soffer, T., and Cohen, A. (2019). Students’ engagement characteristics predict success and completion of online courses. J. Comput. Assist. Learn. 35, 378–389. doi: 10.1111/jcal.12340
Stahnke, R., Schueler, S., and Roesken-Winter, B. (2016). Teachers’ perception, interpretation, and decision-making: A systematic review of empirical mathematics education research. ZDM 48, 1–27. doi: 10.1007/s11858-016-0775-y
Star, J. R., and Strickland, S. K. (2008). Learning to observe: Using video to improve preservice mathematics teachers’ ability to notice. J. Math. Teach. Educ. 11, 107–125. doi: 10.1007/s10857-007-9063-7
Steffensky, M., Gold, B., Holdynski, M., and Möller, K. (2015). Professional vision of classroom management and learning support in science classrooms—does professional vision differ across general and content-specific classroom interactions? Int. J. Sci. Math. Educ. 13, 351–368. doi: 10.1007/s10763-014-9607-0
Stockero, S. L. (2008). Using a video-based curriculum to develop a reflective stance in prospective mathematics teachers. J. Math. Teach. Educ. 11, 373–394. doi: 10.1007/s10857-008-9079-7
Stürmer, K., Könings, K. D., and Seidel, T. (2014). Factors within university-based teacher education relating to preservice teachers’ professional vision. Vocat. Learn. 8, 35–54. doi: 10.1007/s12186-014-9122-z
van den Bogert, N., van Bruggen, J., Kostons, D., and Jochems, W. (2014). First steps into understanding teachers’ visual perception of classroom events. Teach. Teach. Educ. 37, 208–216. doi: 10.1016/j.tate.2013.09.001
van der Westhuizen, C. P., and Golightly, A. (2015). Video annotation software application for thorough collaborative assessment of and feedback on microteaching lessons in geography education. J. Geogr. High. Educ. 39, 420–436. doi: 10.1080/03098265.2015.1053802
van Es, E. A., and Sherin, M. (2002). Learning to notice: Scaffolding new teachers’ interpretations of classroom interactions. J. Technol. Teach. Educ. 571–596.
van Es, E. A., and Sherin, M. G. (2021). Expanding on prior conceptualizations of teacher noticing. ZDM 53, 17–27. doi: 10.1007/s11858-020-01211-4
van Gog, T. (2014). “The signaling (or cueing) principle in multimedia learning,” in The Cambridge Handbook of Multimedia Learning, ed. R. E. Mayer (Cambridge: Cambridge University Press), 263–278. doi: 10.1017/CBO9781139547369.014
ViU: Early Science. (2023a). In Welchen Aggregatzuständen kommt Wasser vor? Universität Münster. Available online at: https://www.uni-muenster.de/Koviu/video/#1UE_Aggregat_Klasse_1DS (accessed September 30, 2023).
ViU: Early Science. (2023b). Klassenführung – Allgegenwärtigkeit - Clip 1. Universität Münster. Available online at: https://www.uni-muenster.de/Koviu/video/#Clip1_Allgegenwaertigkeit (accessed September 30, 2023).
Wang, J. Y., Yang, C. H., Liao, W. C., Yang, K. C., Chang, I. W., Sheu, B. C., et al. (2021). Highly engaged video-watching pattern in asynchronous online pharmacology course in pre-clinical 4th-year medical students was associated with a good self-expectation, understanding, and performance. Front. Med. 8:799412. doi: 10.3389/fmed.2021.799412
Ward, J. H. (1963). Hierarchical grouping to optimize an objective function. J. Am. Stat. Assoc. 58:236. doi: 10.2307/2282967
Weber, K. E., Gold, B., Prilop, C. N., and Kleinknecht, M. (2018). Promoting pre-service teachers’ professional vision of classroom management during practical school training: Effects of a structured online- and video-based self-reflection and feedback intervention. Teach. Teach. Educ. 76, 39–49. doi: 10.1016/j.tate.2018.08.008
Wolff, C. E., Boshuizen, H. P. A., and Jarodzka, H. M. (2015). Revisiting ‘Withitness’: Differences in Teachers’ Representations, Perceptions, and Interpretations of Classroom Management. Amsterdam: Open Universiteit.
Wolff, C. E., Jarodzka, H., and Boshuizen, H. P. (2017). See and tell: Differences between expert and novice teachers’ interpretations of problematic classroom management events. Teach. Teach. Educ. 66, 295–308. doi: 10.1016/j.tate.2017.04.015
xAPI Video Community of Practice (2019a). xAPI Statement Data Model. Available online at: https://liveaspankaj.gitbooks.io/xapi-video-profile/content/statement_data_model.html (accessed September 30, 2023).
xAPI Video Community of Practice (2019b). xAPI Video Profile. Available online at: https://profiles.adlnet.gov/profile/90b2c849-d744-4d0c-8bd0-403e7859a35b (accessed September 30, 2023).
Yeh, C., and Santagata, R. (2015). Preservice teachers’ learning to generate evidence-based hypotheses about the impact of mathematics teaching on learning. J. Teach. Educ. 66, 21–34. doi: 10.1177/0022487114549470
Yoon, M., Lee, J., and Jo, I. H. (2021). Video learning analytics: Investigating behavioral patterns and learner clusters in video-based online learning. Internet High. Educ. 50:100806. doi: 10.1016/j.iheduc.2021.100806
Zhang, J., Huang, Y., and Gao, M. (2022). Video Features, engagement, and patterns of collective attention allocation. J. Learn. Anal. 9, 32–52. doi: 10.18608/jla.2022.7421
Zucker, V., Sunder, C., Konjer, S., Rauterberg, T., Junker, R., Meschede, N., et al. (2022). “Das Videoportal ViU: Early Science: Lernunterstützung und Klassenführung im naturwissenschaftlichen und technischen Sachunterricht professionell wahrnehmen,” in Lehren und Forschen mit Videos in der Lehrkräftebildung, eds R. Junker, V. Zucker, M. Oellers, T. Rauterberg, S. Konjer, N. Meschede, et al. (Montreal, QC: Waxmann), 145–164.
Keywords: professional vision of classroom management, noticing, knowledge-based reasoning, video-based learning, learning analytics, learning path, pre-service teacher training, analysis strategies
Citation: Oellers M, Junker R and Holodynski M (2024) Individual learning paths mastering teachers’ professional vision. Front. Educ. 9:1305073. doi: 10.3389/feduc.2024.1305073
Received: 30 September 2023; Accepted: 08 January 2024;
Published: 01 February 2024.
Edited by:
Christian Kosel, Technical University of Munich, GermanyReviewed by:
Warren Kidd, University of East London, United KingdomMeher Rizvi, The Aga Khan University, Pakistan
Copyright © 2024 Oellers, Junker and Holodynski. This is an open-access article distributed under the terms of the Creative Commons Attribution License (CC BY). The use, distribution or reproduction in other forums is permitted, provided the original author(s) and the copyright owner(s) are credited and that the original publication in this journal is cited, in accordance with accepted academic practice. No use, distribution or reproduction is permitted which does not comply with these terms.
*Correspondence: Manuel Oellers, manuel.oellers@uni-muenster.de