Towards the development of a landslide activity map in Slovenia
- 1Geological Survey of Slovenia, Geological Information Centre, Ljubljana, Slovenia
- 2University of Ljubljana, Faculty of Civil and Geodetic Engineering, Ljubljana, Slovenia
- 3GeoCodis Ltd., Kranj, Slovenia
To create the landslide activity map, we implemented and tested the procedure to fully utilise the 6-day repeatability of the Sentinel-1 constellation in three pilot areas in Slovenia for the observation period from 2017 to 2021. The interferometric processing of the Sentinel-1 images was carried out with ENVI SARScape, while the interpretation of the persistent scatterers InSAR data was done in three steps. In the first step, a preliminary interpretation of the landslide areas was performed by integrating the PS InSAR data into a GIS environment with information that could be relevant to explain the movement patterns of the PS InSAR points. In the second step, a field validation was performed to check the PS InSAR in the field and record the potential damage to the objects indicating the slope mass movements. In the third step, the deformations were identified, and areas of significant movement were determined, consisting of clusters of at least 3 persistent scatterers (PS) with a maximum spacing of 10 m. The landslide activity map was created based on the landslide areas categorised into four classes based on the geotechnical analyses, yearly velocity data obtained by PS InSAR, and validation of annual velocity data obtained by in-situ and GNSS monitoring and field observation. A total of 21 polygons with different landslide activities were identified in three study areas. The overall methodology will help stakeholders in the early mapping and monitoring of landslides to increase the urban resilience.
Introduction
Effective approaches to reducing landslide risk include the development of methods to identify landslide-prone areas and the development of risk reduction concepts to mitigate the effects of landslides in these areas. Among available techniques landslide monitoring present a mandatory step in collecting data on landslide conditions (e.g., areal extent, landslide kinematics, surface topography, hydrogeometeorological parameters, and failure surfaces) from different time periods and at different scales, from site-specific to local, regional, and national, to assess landslide activity (Guzzetti et al., 2012; Jemec Auflič et al., 2023).
For years, EO data have been very useful in detecting ground motion (Colesanti and Wasowski, 2006; Crosetto et al., 2016; Casagli et al., 2017; Solari et al., 2020; Jemec Auflič et al., 2023). The theory of Interferometric Synthetic Aperture Radar (InSAR) has been known for decades: after applying geometric and atmospheric corrections, high-resolution maps of surface displacements can be produced by comparing the phase of successive radar images. With a spatial resolution in the range of 10 m, a temporal resolution of less than a week, and a displacement accuracy of 1 mm, we can quantify the movement rates of the processes by which the Earth deforms and provide relevant information for disaster risk reduction. Spaceborne differential interferometric SAR (DInSAR) dates to 1989 when a technique that exploited L-band SEASAT SAR data was first described Gabriel et al. (1989). Within DInSAR, the most advanced methods that make use of large sets of SAR images date back almost 2 decades. Persistent Scatterer Interferometry (PS InSAR) technique was first described in the article by Ferretti et al. (2000) and has been used by many authors since then (Jauvin et al., 2019). DInSAR and PS InSAR have demonstrated their unmatched deformation measurement and monitoring capabilities in a wide range of application fields. The European Union’s Sentinel-1 constellation is a mission specifically designed to monitor ground deformation (Torres et al., 2012; Wasowski and Bovenga, 2014).
In 2023, severe rainfall events triggered more than 8000 landslides in Slovenia (Jež et al., 2023; Peternel et al., 2023). Most of these landslides are categorized as shallow landslides, soil slips, and landslides that primarily caused damage to buildings, infrastructure and agricultural land. Among the numerous registered landslides, there are also landslides with a volume of more than one million m3, which, in addition to the damage to buildings, also endangered the lives of hundreds of people and even claimed human lives. The damage caused by landslides only in 2023 is estimated at 2 billion euros (Jež et al., 2023; Peternel et al., 2023).
In Slovenia, a national landslide prediction system (acronym MASPREM) has been in operation since 2013, developed by the Geological Survey of Slovenia (GeoZS) and financed by the Administration for Civil Protection and Disaster Relief (ACPDR) (Jemec Auflič et al., 2016). The prediction system provides information on the probability of landslides occurring in the next 24 h based on the forecasted rainfall. The results are various landslide prediction models at national and municipal level. The information is disseminated daily to the general public via the media. Since MASPREM predicts landslides spatially and temporally, the system can be improved in terms of landslide velocity by using EO data. This paper presents the development of a method for the early detection of landslides and the creation of a map of landslide activity in three pilot areas, which could help the relevant authorities in the country to reduce the risk of landslides.
Methodology
The methodology for creating the landslide activity map consists of six main tasks, shown in Figure 1, where tasks one to five are required for PS InSAR processing and task six for generation of the landslide activity map.
Sentinel-1 PS InSAR processing
For PS InSAR analysis, we use raw Sentinel-1 SLC (Single Look Complex) radar images to calculate the interferometric phase and coherence. Coherence describes the ratio between two (usually simultaneous) images of the same area. It is calculated in interferometric processing as a measure of the correlation between the two images. It indicates whether the reflected signals of the first and second images are in phase (i.e., coherent) or not, and, therefore, whether the measurement of displacements is possible at all (Pehani et al., 2021). We use images of the same relative orbit from two consecutive passes (6 days difference between the two acquisitions). This means one Sentinel-1A image and one overlapping Sentinel-1B image. In the processing, we used both ascending (No. 44 and 146) and descending orbit (No. 22). For this study’s purposes, 1807 images were used (Table 1). The number of images is different for the period when only one Sentinel-1 satellite is operational and for the period when both satellites are operational, so the processing was performed only for the period when both satellites were fully operational, i.e. 2017-2021.
The interferometric processing is performed in ENVI SARScape. This approach is based on the state-of-the-art described in Fárová et al. (2019), Fiaschi et al. (2019), Solari et al. (2019), Liu et al. (2020) and Zhu et al. (2021) and includes the geological characteristics of the terrain such as geological settings, distance to faults and thrusts, slope angle, strike and dip. We used a local workstation with 13th generation Intel Core i9-13900 with 24 cores at 2.00 GHz, 64 GB memory and NVIDIA RTX 4090 connected to a NAS data storage containing all SLC data for Slovenia. We processed the data for all orbits on a single computer. The most demanding processes are subsetting (AOI selection) and coregistration, which take about 80% of the time. The total processing for one orbit and the entire period is about 100 h. After the first processing, we repeat the processing for 1 year, which means that the processing takes about 20 h. If a larger area needs to be processed, we can use the ENVI SARScape processing cluster.
The processing results are given as vector and raster data for each combination of tracks and observation period with the following attributes shown in Table 2. The processing was carried out for the three landslide areas in Slovenia (Figure 2). The total number of persistent scatterers in the areas and on the landslides is shown in Table 3.
During InSAR processing, we checked and minimized various influences on the InSAR signal, in particular temporal and spatial decorrelation and atmospheric effects. We compared the results with GNSS measurements to ensure that we only obtained relative motions for stable points. Furthermore, by using data from ascending and descending orbits, the effects of LOS observations and slope orientation were minimized. Nevertheless, the displacements obtained with InSAR and their relation to landslides were carefully checked in the interpretation phase to avoid errors.
Generation of landslide activity map
A general methodology for PS InSAR interpretation and landslide activity mapping is presented here following the steps demonstrated on Figure 1, while landslide characteristics and interpretation of PS InSAR data are discussed in the next chapter (Results and Discussion).
Firstly, a preliminary interpretation of the landslide areas was done through the integration in a GIS environment of the PS InSAR data with information which might have relevance in explaining the patterns of motions of PS InSAR points: topographic maps, aerial orthophotos, geology, Lidar (1 m resolution), landslide inventory and geotechnical database. SARScape tool produced PS InSAR data in three directions: LOS, vertical and downslope. In addition, the sign of the measured displacements has to be interpreted considering the terrain slope. The evaluation of the state of activity, where geological surveys and instrumental data were not available, was done through a morphological approach based on aerial photo interpretation of different periods; this fact complicates the comparison between the activity of landslides and the PS InSAR data. Secondly, a field validation was performed to check PS InSAR on the field and to record the potential damage on the objects indicating the slope mass movements. Thirdly, we identified deformation and identified areas of significant movement consisting of clusters of at least 3 PS with a maximum distance of 10 m. The precision of the method has been estimated to ±2 mm/yr so the measurements ranging between −2 mm/yr and +2 mm/yr have been considered to indicate areas with no significant movements. Overlaying the landslide areas with all other data layers, field observations, and PS InSAR allows us to create a map of landslide activity classified into three classes: low, medium, and high. In addition, we also created polygons indicating areas with no significant movements with velocities between −2 and +2 mm/yr. For identifying the landslide activity map, the following decision criteria have been used: 1) landslides areas defined based on the geotechnical analyses; 2) yearly velocity data in downslope direction obtained by PS InSAR ranked in four classes; 3) validating yearly velocity data obtained by in-situ and GNSS monitoring; and 4) field observation. In this study we focus on the interpretation of velocities indicating downslope moving, which is the common manifestation of slope mass movements.
Results and discussion
Čemšenik landslide
In our analysis, we have focused on the area of the Čemšenik settlement, which is located on the southern slopes of Čemšeniška planina mountain (Čemšenik plateau, Figure 3). It covers an area of about 2.3 km2 and lies at an altitude of about 630 m a.s.l. It is characterised by medium to steep slopes sloping to the south. Currently it has 205 inhabitants. Part of the settlement was built in the area of a dormant deep-seated landslide. A landslide was reactivated due to heavy rainfall in 2010. Due to reactivation of landslide new cracks appeared on the church, roads, and residential buildings, posing a direct threat to the infrastructure, residents and their property. The area is composed of the Triassic carbonate rocks thrust over Upper Carboniferous and Permian clastic rocks. Part of the settlement where the landslide was formed is covered with a thick layer of carbonate gravel deposited by the past mass flows. The landslide covers an area of around 71,000 m2 and is 400 m long and 230 m wide. According to earlier geological and geotechnical investigations, the sliding surface was formed at a depth of 18–25 m below the surface (Popović, 2022).
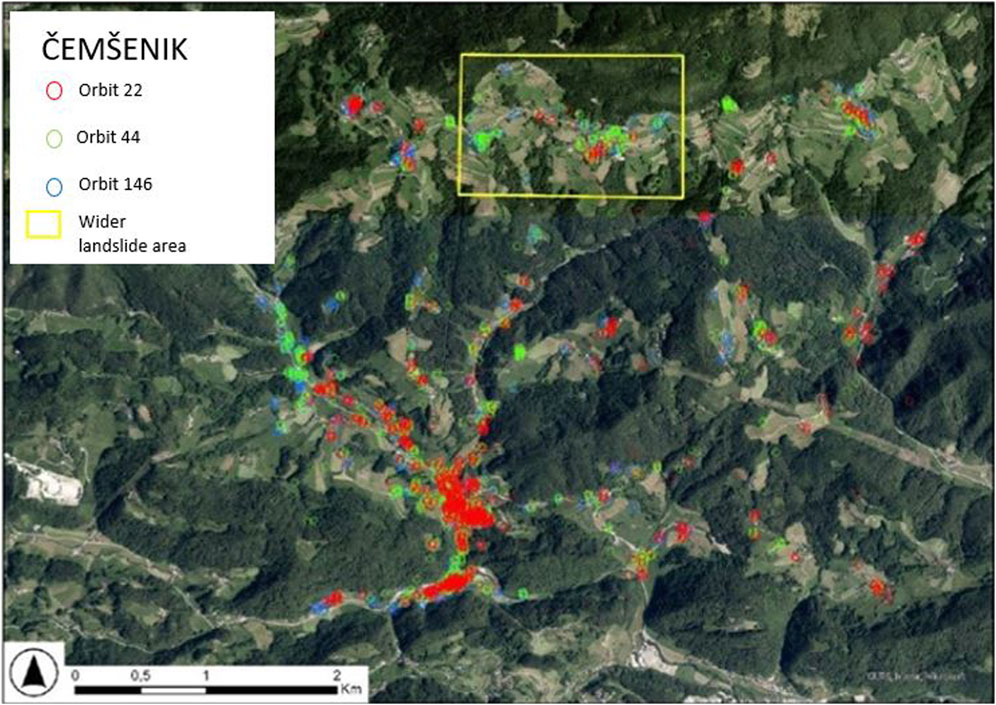
Figure 3. PS InSAR data for pilot site Čemšenik (orbits 22, 44 and 146) with the location of the landslide area.
About 141 persistent scatterers were captured within the known landslide area. In this area, most persistent scatterers (86%) indicate ground subsidence. Considering the downslope orientation of the displacement, the maximal velocity of the subsidence in the area is −7.2 mm/yr and the maximal velocity of the uplift is 1.1 mm/yr (Figure 4).
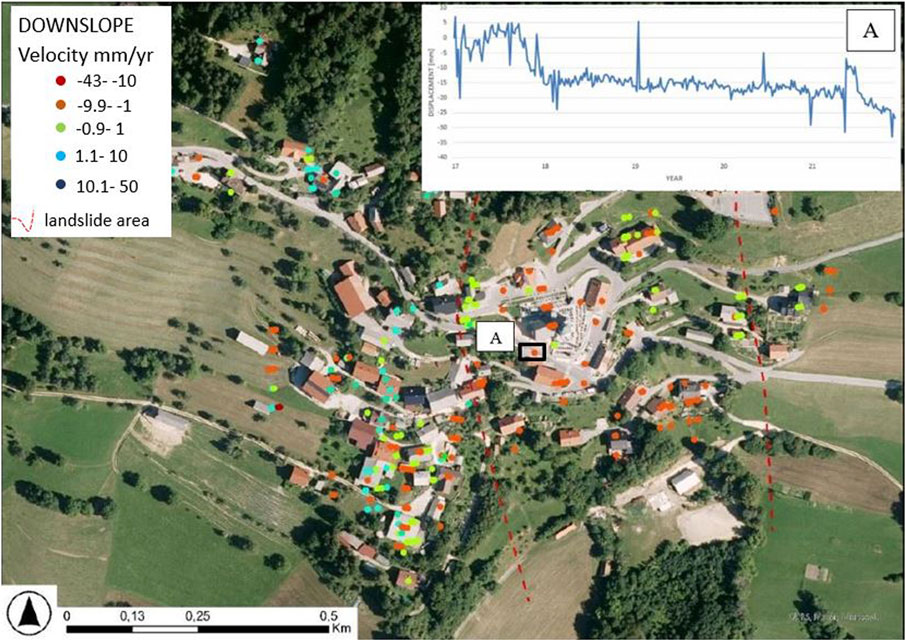
Figure 4. PS InSAR data (all orbits) for downslope direction on the Čemšenik landslide. A-time series of PS InSAR point located on the landslide body.
Persistent scatterers on the object were field-validated and the damage on the object was estimated based on visible cracks, deformation on the pavement and reports from the residents. Figure 5 shows the match between activity obtained by the PS InSAR data and field damage on objects. Some field examples of the damage are presented in Figure 6.
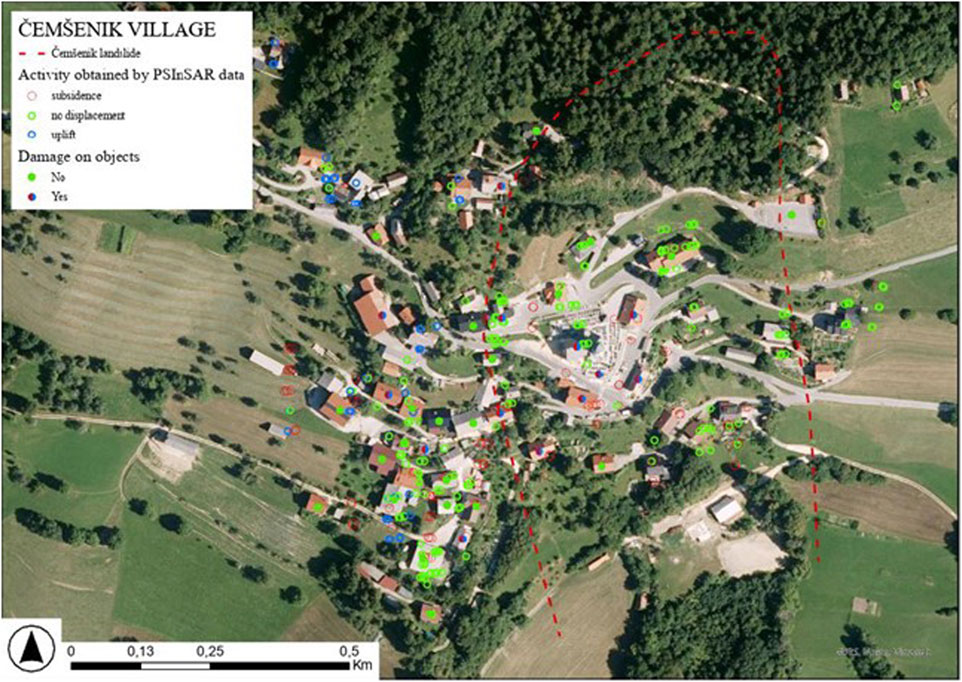
Figure 5. Activity obtained by PS InSAR data (downslope direction) and field evidence of the displacements (damage on the objects) in the Čemšenik village.
PS InSAR point data were generalized and extrapolated to form polygons with low, medium or high activity. Velocities in the high activity area range from −6 to −2.8 mm/yr, medium active area range from −2.8 to −2.4 mm/yr and low active area from −2.4 to −2 mm/yr. Its extent only partially correlates with the known landslide extent. In its southeastern part, the landslide area should be extended. On the other side, there is a large area of low activity within the known landslide area. In the existing reports, it is already stated that the eastern edge of the landslide was hard to define. It is possible that the main landslide body actually has a different shape and is related to the ridge in the central part of the village (corresponding to the high activity area). It seems like the landslide is widening in the downslope direction. We could conclude that we acquired some valuable additional information about the extent and activity of the landslide in Čemšenik village and checked the success of the protection measures. Based on the decision criteria, we identified the following four polygons with different landslide activity high, medium, low, and no significant movements (Figure 7).
Rebrnice
Rebrnice stands for a landslide-prone area faced SW in the Vipava valley in southwestern Slovenia (Figure 2). The landslide-prone area is crossed by a national motorway Razdrto-Vipava which is an important link between Slovenia and Italy (Figure 8). The total length of the line is almost 11,000 km, of which 3,900 km run on viaducts and in tunnels. The most active part Rebrnice is 6,200 km long. The motorway was built up on the carbonate debris deposited on flysch. Carbonate debris deposit is prone to sliding due to slope inclination, groundwater and clay zones. Superficial deposits range from large-scale, deep-seated rotational and translational slides to shallow landslides, slumps, and sedimentary gravity flows in the form of debris or mudflows reworking the carbonate scree and flysch material (Placer et al., 2008; Jemec Auflič et al., 2017; Popit et al., 2017). The route was chosen to minimize landslide areas and to retain water sources for the villages in the Vipava river valley. During the construction works some landslides were activated.
About 10,129 PS InSAR persistent scatterers were defined in the entire area (Figure 9), of which 3% PS InSAR coincided with landslides (Figure 10). Considering downslope direction of the displacement, the maximal velocity of the subsidence on the landslide is 34.0 mm/yr and the maximal velocity of the uplift is 70 mm/yr; the time series show changes in trend of the ground motion. Field investigations in the vicinity of the highway suggest that PS InSAR displacements match well with field observations, especially in the areas with the highest ground motions, indicating high and medium landslide activity. Based on previous studies and PS InSAR data we have identified at least 7 areas with different landslide activity (Figure 11).
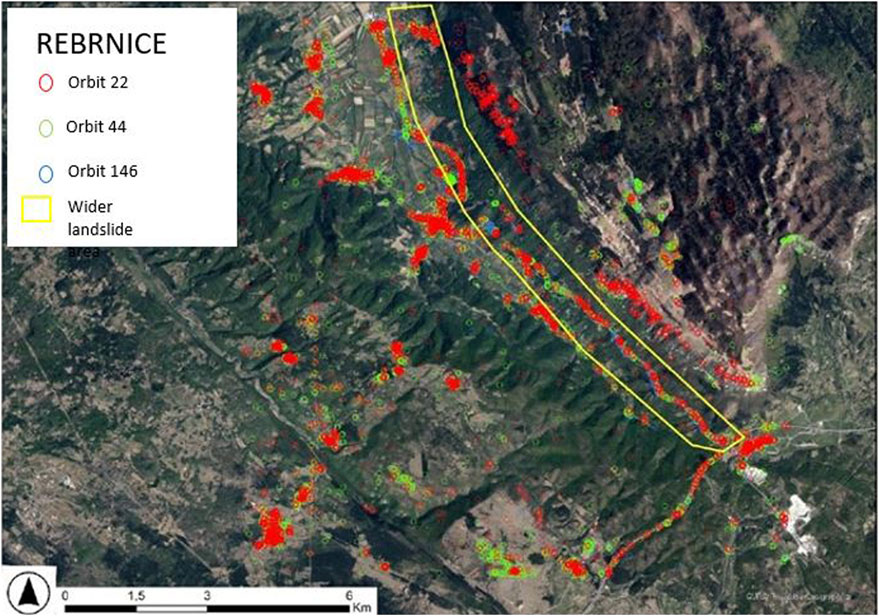
Figure 9. PS InSAR data for pilot site Rebrnice (orbits 22, 44 and 146) with the location of landslide area.
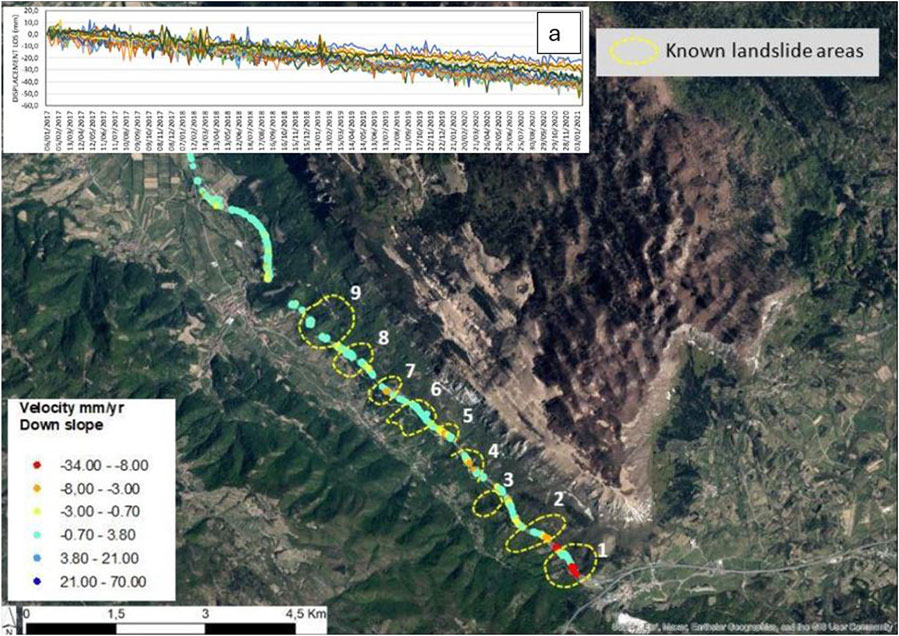
Figure 10. PS InSAR data in downslope direction for Motorvay HC-Razdrto-Vipava with marked known landslides. No. 1 marks time series of PS InSAR data.
Potoška planina
Potoška planina stands for a landslide-prone area located in the hinterland of Koroška Bela settlement in northwestern Slovenia. Village is densely populated urban settlement located on the accumulation fan of the past debris flows. It has about 2,100 inhabitants, a well-developed steel industry and important infrastructure (railway and road) connections between Slovenia and Austria. The slopes in the area above Koroška Bela are characterized by complex morphology and unfavourable geological and tectonic conditions. In general, the area consists mainly consists of Upper Carboniferous and Permian clastic rocks–Permian carbonates and Triassic to Lower Jurassic carbonate rocks (Jež et al., 2008). The main slope instabilities are related to the tectonic contacts between the Upper Carboniferous to Permian clastic rocks (claystone, siltstone, sandstone and conglomerate) and various Permian and Triassic carbonate and clastic rocks. Tectonically, the area belongs to the Southern Alps. It is part of the Košuta fault zone and is dissected by numerous NW-SE faults that connect two major fault zones (the Sava and Periadriatic fault zones) (Jež et al., 2008). The rocks are heavily deformed and, therefore, very prone to rapid and deep weathering. The carbonate rocks in the uppermost parts of the Belščica slope are also subject to intense physical and chemical weathering, resulting in large amounts of debris and scree material.
These factors contribute to the fact that the region is highly susceptible to landslides and debris flows, which could pose a significant threat to the densely populated settlement below (Peternel et al., 2017; Peternel et al., 2018; Šegina et al., 2020; Šegina et al., 2022). It attracts additional attention due to historical evidence of the past debris flows in recent geological history (Lavtižar, 1897; Zupan, 1937). The most recent event occurred in April 2017, when part of the Čikla landslide collapsed and turned into a debris flow (Peternel et al., 2022).
The study area is covered by vegetation, thus the density of persistent scatterers is low, while their density PS InSAR in the village is very high (Figure 12). We present the displacement velocities and time series obtained with PS InSAR in the downslope direction for individual parts of Potoška planina landslide area. 349 (40%) persistent scatterers were captured within the known landslide areas and areas with different activities (Figure 13). In the hinterland area, 18% of persistent scatterers indicate ground subsidence, 19% of PS InSAR are categorized in class with no significant movements with yearly velocities ±2 mm/yr and 63% indicate surface uplift. Considering a downslope orientation of the displacement, the maximal velocity of the subsidence in the area is 30.5 mm/yr and the maximal velocity of the uplift is 478.6 mm/yr.
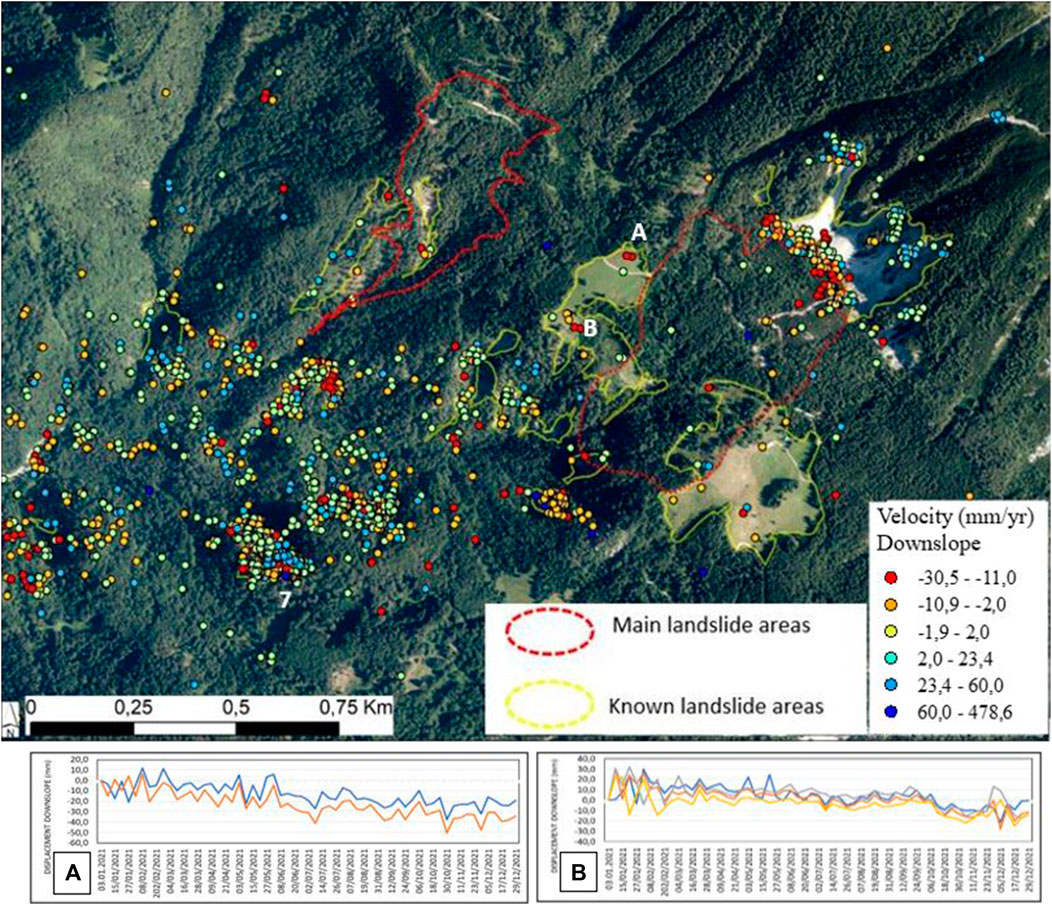
Figure 13. PS InSAR yearly velocity in downslope direction in the Potoška planina landslide area with marked PS InSAR, indicating time series (A, B).
Irregular and hummocky terrain, which consists of protrusions and depressions of varying sizes, curved trees, tension cracks, erosional slides, and wetlands all indicate an activity of landslides in this area (Figure 14). Local roads also show signs of subsidence, another indication of the area’s instability. Although many persistent scatterers PS InSAR are located on the vegetation, we also obtained PS InSAR some of them on roads, rock outcrops, and boulders that are more reliable candidates for ground motion detection (Figure 13). However, based on the geological, geotechnical, and geodetic surveys and several years of monitoring, we have identified at least 5 areas with varying landslide activity (Figure 15).
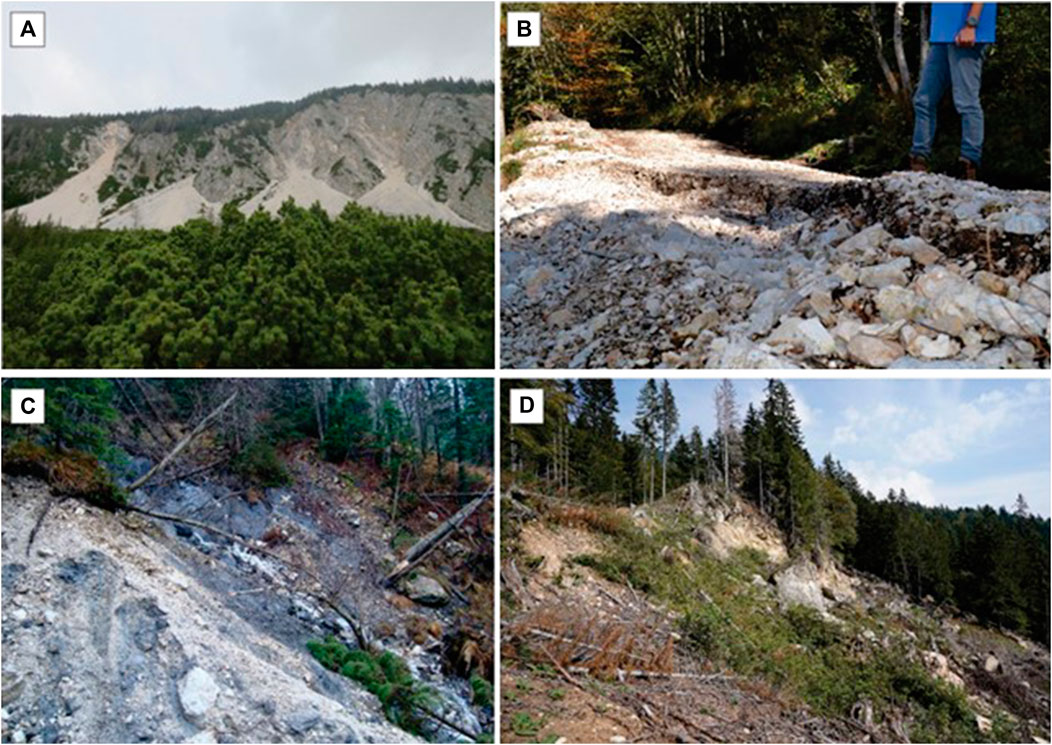
Figure 14. Field evidence of surface activity in the Potoška planina landslide area. (A) scree area; (B) road deformation; (C) active lower part of the landslide; (D) source area of Čikla landslide.
The most active area is the lower part of the Urbas landslide which has the potential to mobilise into a debris flow. Field observation revealed very active erosion processes, curved trees, wetlands, road subsidence, open cracks and scarps. Persistent scatterers show movements in slope direction from −30 to −23 mm/yr, while GNSS monitoring indicates the displacement of around 60 mm/yr in the upper part of the landslide and over 400 mm/yr in the lower part of the landslide, which is considerably more than the values obtained by the PS InSAR (both values are obtained for the period between the years 2019 and 2021). Another very active area is Čikla. Field observation shows the active area consists of debris deposits of various sizes constantly moving downslope. Persistent scatterers were detected in the lower part of the Čikla landslide and outside polygons, but in this case PS InSAR can be considered as an indicator of ground movement. Moderately active is upper part of the hinterland covered with scree material and characterized as rockfall area. Persistent scatterers show movements in downslope direction from −23–31 mm/year. Low active areas marked with green polygons are in the central and lower parts of the hinterland. Field observations showed these are deforestation areas with PS InSAR displacements from −20–25 mm/yr, while GNSS monitoring shows small displacements in range of 4.2 mm/yr (GNSS 1) and 1.2 mm/yr (GNSS 2).
Conclusion
We present a methodology for the development of a landslide activity map based on Sentinel-1 data PS InSAR processing for three landslide areas in Slovenia. Based on the yearly velocities of downslope data, the following conclusions can be drawn.
(1) The highest velocities were measured in Potoška planina (−30 to −7 mm/yr), followed by Rebrnice (−15 to −10 mm/yr) and Čemšenik (−6 to −2.8 mm/yr). From the geological point of view, all three study areas are characterized by permeable scree talus material and clastic impermeable layers of different depths. Slip surfaces occur at the contact between these two lithological layers (Potoška planina 10–15 m, Rebrnice 15–50 m, Čemšenik 15–25 m). The Čemšenik and Rebrnice areas were recently remediated, while no remediation work has been carried out in the Potoška planina so far. Despite the remediation work, PS InSAR detected a higher deformation of the landslide where the sliding surface is closer to the surface. Geodetic data in Potoška planina showed significantly higher movements than PS InSAR for the Urbas and Čikla landslides, while in Rebrnice GNSS data match PS InSAR measurements. For the Čemšenik area, GNSS data are not available for the observation period (2017-2021). Due to the dense vegetation in Potoška planina, we considered PS InSAR data with a coherence of 0.50, while at the other two sites we considered a coherence of 0.75. This shows that some site-specific adjustments are necessary to extract the best possible information from the PS InSAR data. Although remediation work were carried out we can it can be seen we can assume that the highest velocities have been observed in the landslide where the sliding surface is closest to the surface.
(2) The study demonstrates that the applied technique is well suited to complement and integrate information from conventional landslide detection methods. Furthermore, it extends our understanding of slope mass movements beyond the boundaries of closely monitored sites.
(3) The success of the PS InSAR method also depends on landslide typology, associated kinematics, landslide and slope orientation, vegetation, and existing permanent objects on the surface. In practice, the PS InSAR method is particularly valuable for monitoring the gradual evolution of slow and extremely slow landslides characterized by uniform rates of deformation. Its ability to track subtle ground movements over long periods of time makes it an indispensable tool for landslide research.
(4) The PS InSAR results provide valuable insights into landslide velocities and the temporal evolution of ground movements. This information is applicable not only applicable to the three known landslides, but also to previously unstudied areas.
(5) An important milestone is the use of Sentinel-1 data to monitor complex, deep-seated landslides in Slovenia. This novel approach has the potential to improve the early mapping and monitoring of landslides and thus contribute to the resilience of cities. The integration of the landslide activity map into the MASPREM service provides both spatial and temporal information on landslide occurrence, filling a current gap in the available data.
Data availability statement
The raw data supporting the conclusion of this article will be made available by the authors, without undue reservation.
Author contributions
MJ: Project Administration, Supervision, Conceptualization, Investigation, Methodology, Writing–original draft, Writing–review and editing; KO: Methodology, Processing data, Writing–review and editing; TG: Writing–review and editing; MI: Writing–review and editing; TP: Investigation, Writing–original draft; EŠ: Investigation, Visualization, Writing–original draft.
Funding
The author(s) declare financial support was received for the research, authorship, and/or publication of this article. This work was carried out within the framework of ESA project no. 4000139004/22/I-DT-bgh (EO4MASRISK). The authors are grateful for this project’s funding. This study was also financial supported by the research programme P2-0406 Earth observation and geoinformatics and P1-0419 Earth Dynamics.
Conflict of interest
Author MI was employed by GeoCodis Ltd.
The remaining authors declare that the research was conducted in the absence of any commercial or financial relationships that could be construed as a potential conflict of interest.
Publisher’s note
All claims expressed in this article are solely those of the authors and do not necessarily represent those of their affiliated organizations, or those of the publisher, the editors and the reviewers. Any product that may be evaluated in this article, or claim that may be made by its manufacturer, is not guaranteed or endorsed by the publisher.
References
Casagli, N., Frodella, W., Morelli, S., Tofani, V., Ciampalini, A., Intrieri, E., et al. (2017). Spaceborne, UAV and ground-based remote sensing techniques for landslide mapping, monitoring and early warning. Geoenvironmental Disasters 4 (1), 9. doi:10.1186/s40677-017-0073-1
Colesanti, C., and Wasowski, J. (2006). Investigating landslides with space-borne synthetic aperture radar (SAR) Interferometry. Eng. Geol. 88 (3–4), 173–199. doi:10.1016/j.enggeo.2006.09.013
Crosetto, M., Monserrat, O., Cuevas-González, M., Devanthéry, N., and Crippa, B. (2016). Persistent scatterer Interferometry: a review. ISPRS J. Photogrammetry Remote Sens. 115, 78–89. doi:10.1016/j.isprsjprs.2015.10.011
Ela, Š., Mateja, J. A., Matija, Z., Jernej, J., and Tina, P. (2022). Composite landslide in the dynamic alpine conditions: a case study of Urbas landslide. Geologija 65 (2), 161–175. doi:10.5474/geologija.2022.010
Fárová, K., Jelének, J., Kopačková-Strnadová, V., and Kycl, P. (2019). Comparing DInSAR and PSI techniques employed to sentinel-1 data to monitor highway stability: a case study of a massive dobkovičky landslide, Czech republic. Remote Sens. 11 (22), 2670. doi:10.3390/rs11222670
Ferretti, A., Prati, C., and Rocca, F. (2000). Nonlinear subsidence rate estimation using permanent scatterers in differential SAR Interferometry. IEEE Trans. Geoscience Remote Sens. 38 (5 I), 2202–2212. doi:10.1109/36.868878
Fiaschi, S., Holohan, E. P., Sheehy, M., and Floris, M. (2019). PS-InSAR analysis of sentinel-1 data for detecting ground motion in temperate oceanic climate zones: a case study in the republic of Ireland. Remote Sens. 11 (3), 348. doi:10.3390/rs11030348
Gabriel, A. K., Goldstein, R. M., and Zebker, H. A. (1989). Mapping small elevation changes over large areas: differential radar Interferometry. J. Geophys. Res. 94 (B7), 9183–9191. doi:10.1029/JB094iB07p09183
Guzzetti, F., Mondini, A. C., Cardinali, M., Fiorucci, F., Santangelo, M., and Chang, K.-T. (2012). Landslide inventory maps: new tools for an old problem. Earth-Science Rev. 112 (1), 42–66. doi:10.1016/j.earscirev.2012.02.001
Jauvin, M., Yan, Y., Trouvé, E., Fruneau, B., Gay, M., and Girard, B. (2019). Integration of corner reflectors for the monitoring of mountain glacier areas with sentinel-1 time series. Remote Sens. 11 (8), 988. doi:10.3390/rs11080988
Jemec Auflič, M., Šinigoj, M. J., Krivic, M., Podboj, M., Peternel, T., and Komac, M.(2016). Landslide prediction system for rainfall induced landslides in Slovenia (masprem). Geologija 59(2 SE-Articles), 259–271. doi:10.5474/geologija.2016.016
Jemec Auflič, M., Herrera, G. M., Mateos, R. M., Poyiadji, E., Quental, L., Severine, B., et al. (2023). Landslide monitoring techniques in the geological surveys of europe. Landslides 20(5), 951–965. doi:10.1007/s10346-022-02007-1
Jemec Auflič, M., Jež, J., Popit, T., Košir, A., Maček, M., Logar, J., et al. (2017). The variety of landslide forms in Slovenia and its immediate NW surroundings. Landslides 14(4), 1537–1546. doi:10.1007/s10346-017-0848-1
Jež, J., Mikoš, M., Trajanova, M., Kumelj, Š., Budkovič, T., and Bavec, M. (2008). Koro ka Bela alluvial fan – the result of the catastrophic slope events;Karavanke Mountains, NW Slovenia. Geologija 51 (2 SE-Articles), 219–227. doi:10.5474/geologija.2008.022
Jež, J., Novak, A., Peternel, T., AufličMateja, J., Šegina, E., Teran, K., et al. (2023). Pobočni masni premiki v Sloveniji med ujmo - avgust 2023. V: ROŽIČ, Boštjan (ur.). 26. posvetovanje slovenskih geologov = 26th Meeting of Slovenian Geologists. Ljubljana, Slovenia: Univerza v Ljubljani, Naravoslovnotehniška fakulteta, Oddelek za geologijo, 69–77.Geološki zbornik, 26
Liu, L., Yu, J., Chen, B., and Wang, Y. (2020). Urban subsidence monitoring by SBAS-InSAR technique with multi-platform SAR images: a case study of Beijing plain, China. Eur. J. Remote Sens. 53 (Suppl. 1), 141–153. doi:10.1080/22797254.2020.1728582
Pehani, P., Čož, N., Veljanovski, T., Kokalj, Ž., Lisec, A., and Oštir, K. (2021). Automatic processing of Sentinel-1 sigma and coherence: technical report: technical report. Ljubljana, Slovenia: ZRC SAZU.
Peternel, T., Janža, M., Šegina, E., Bezak, N., and Maček, M. (2022a). Recognition of landslide triggering mechanisms and dynamics using GNSS, UAV photogrammetry and in situ monitoring data. Remote Sens. 14 (14), 3277. doi:10.3390/rs14143277
Peternel, T., Jež, J., Milanič, B., Markelj, A., and Auflič, M. J. (2018). Engineering-geological conditions of landslides above the settlement of Koroška Bela (NW Slovenia). Geologija 61 (2 SE-Articles), 177–189. doi:10.5474/geologija.2018.012
Peternel, T., Jež, J., Novak, A., Auflič, J., Šegina, M., Ela, B., et al. (2023). Zemeljski plazovi in ostali pojavi pobočnega masnega premikanja med ujmo avgusta 2023 V: GLOBEVNIK, Lidija (ur.), PREŠEREN, Anton (ur.) Tretji slovenski kongres o vodah 2023: zbornik. Kamnik: DVS- Društvo vodarjev Slovenije, 25–31.[Ptuj, 19.-20.10.2023]
Peternel, T., Kumelj, Š., Oštir, K., and Komac, M. (2017). Monitoring the Potoška planina landslide (NW Slovenia) using UAV photogrammetry and tachometeric measurements. Landslides 14 (1), 395–406. doi:10.1007/s10346-016-0759-6
Peternel, T., Šinigoj, J., Auflič, J., Kumelj, M., Špela, K., Matija. Masprem - Slovenian landslide forecasting and warning system V: Peranić, Josip (ur.), , et al. (2022b). Landslide modelling and applications: proceedings of the 5th regional symposium on landslides in the adriatic-balkan region: [23-26 march 2022, rijeka]. Rijeka, Croatia: Faculty of Civil Engineering, University of Rijeka; Zagreb: Faculty of Mining, Geology and Petroleum Engineering, University of Zagreb, 113–118.
Placer, L., Jež, J., and Atanackov, J. (2008). Strukturni pogled Na plaz slano blato structural aspect on the slano blato landslide (Ljubljana, Slovenia). Geologija 512 (2005). doi:10.5474/geologija.2008.023
Popit, T., Jež, J., and Verbovšek, T. (2017). “Mass movement processes of quaternary deposits in the Vipava Valley, SW Slovenia,” in Advancing culture of living with landslides (Berlin, Germany: Springer). doi:10.1007/978-3-319-53485-5_66
Šegina, E., Peternel, T., Urbančič, T., Realini, E., Zupan, M., Jež, J., et al. (2020). Monitoring surface displacement of a deep-seated landslide by a low-cost and near real-time GNSS system. Remote Sens. 12, 3375. doi:10.3390/rs12203375
Solari, L., Del Soldato, M., Raspini, F., Barra, A., Bianchini, S., Confuorto, P., et al. (2020). Review of satellite Interferometry for landslide detection in Italy. Remote Sens. 12, 1351. doi:10.3390/rs12081351
Solari, L., Soldato, M. D., Montalti, R., Bianchini, S., Raspini, F., Thuegaz, P., et al. (2019). A sentinel-1 based hot-spot analysis: landslide mapping in north-western Italy. Int. J. Remote Sens. 40 (20), 7898–7921. doi:10.1080/01431161.2019.1607612
Torres, R., Snoeij, P., Geudtner, D., Bibby, D., Davidson, M., Attema, E., et al. (2012). GMES sentinel-1 mission. Remote Sens. Environ. 120, 9–24. doi:10.1016/j.rse.2011.05.028
Wasowski, J., and Fabio, B. (2014). Investigating landslides and unstable slopes with satellite multi temporal Interferometry: current issues and future perspectives. Eng. Geol. 174, 103–138. doi:10.1016/j.enggeo.2014.03.003
Zhu, K., Xu, P., Cao, C., Zheng, L., Liu, Y., and Dong, X. (2021). Preliminary identification of geological hazards from songpinggou to feihong in mao county along the minjiang river using sbas-insar technique integrated multiple spatial analysis methods. Sustain. Switz. 13 (3), 1017. doi:10.3390/SU13031017
Keywords: landslides, EO data, sentinel, time series, methodology, Slovenia
Citation: Jemec Auflič M, Oštir K, Grabrijan T, Ivačič M, Peternel T and Šegina E (2024) Towards the development of a landslide activity map in Slovenia. Front. Earth Sci. 12:1368405. doi: 10.3389/feart.2024.1368405
Received: 10 January 2024; Accepted: 25 March 2024;
Published: 24 April 2024.
Edited by:
Veronica Pazzi, University of Trieste, ItalyReviewed by:
Stefano Devoto, University of Trieste, ItalyGuangyu Xu, East China University of Technology, China
Copyright © 2024 Jemec Auflič, Oštir, Grabrijan, Ivačič, Peternel and Šegina. This is an open-access article distributed under the terms of the Creative Commons Attribution License (CC BY). The use, distribution or reproduction in other forums is permitted, provided the original author(s) and the copyright owner(s) are credited and that the original publication in this journal is cited, in accordance with accepted academic practice. No use, distribution or reproduction is permitted which does not comply with these terms.
*Correspondence: Mateja Jemec Auflič, mateja.jemec-auflic@geo-zs.si