Diagnostic Improvements of Deep Learning–Based Image Reconstruction for Assessing Calcification-Related Obstructive Coronary Artery Disease
- 1State Key Laboratory of Complex Severe and Rare Diseases, Department of Radiology, Peking Union Medical College Hospital, Chinese Academy of Medical Sciences and Peking Union Medical College, Beijing, China
- 2Canon Medical System, Beijing, China
- 3Medical Science Research Center, Peking Union Medical College Hospital, Beijing, China
Objectives: The objective of this study was to explore the diagnostic value of deep learning-based image reconstruction (DLR) and hybrid iterative reconstruction (HIR) for calcification-related obstructive coronary artery disease (CAD) evaluation by using coronary CT angiography (CCTA) images and subtraction CCTA images.
Methods: Forty-two consecutive patients with known or suspected coronary artery disease who underwent coronary CTA on a 320-row CT scanner and subsequent invasive coronary angiography (ICA), which was used as the reference standard, were enrolled. The DLR and HIR images were reconstructed as CTADLR and CTAHIR, and, based on which, the corresponding subtraction CCTA images were established as CTAsDLR and CTAsHIR, respectively. Qualitative images quality comparison was performed by using a Likert 4 stage score, and quantitative images quality parameters, including image noise, signal-to-noise ratio, and contrast-to-noise ratio were calculated. Diagnostic performance on the lesion level was assessed and compared among the four CCTA approaches (CTADLR, CTAHIR, CTAsDLR, and CTAsHIR).
Results: There were 166 lesions of 86 vessels in 42 patients (32 men and 10 women; 62.9 ± 9.3 years) finally enrolled for analysis. The qualitative and quantitative image qualities of CTAsDLR and CTADLR were superior to those of CTAsHIR and CTAHIR, respectively. The diagnostic accuracies of CTAsDLR, CTADLR, CTAsHIR, and CTAHIR to identify calcification-related obstructive diameter stenosis were 83.73%, 69.28%, 75.30%, and 65.66%, respectively. The false-positive rates of CTAsDLR, CTADLR, CTAsHIR, and CTAHIR for luminal diameter stenosis ≥50% were 15%, 31%, 24%, and 34%, respectively. The sensitivity and the specificity to identify ≥50% luminal diameter stenosis was 90.91% and 83.23% for CTAsDLR.
Conclusion: Our study showed that deep learning–based image reconstruction could improve the image quality of CCTA images and diagnostic performance for calcification-related obstructive CAD, especially when combined with subtraction technique.
Introduction
With excellent sensitivity and negative predictive value, coronary CT angiography (CCTA) has developed into one of the first choices of non-invasive diagnostic strategies for the evaluation of coronary artery disease (CAD) during clinical practice (1–4). However, on account of the blooming and beam hardening artifacts, the accuracy of stenosis evaluation related to calcified plaques was still unsatisfactory, since the calcification might lead to overestimation of the stenosis and excessive downstream testing (5–7).
Deep learning–based image reconstruction (DLR) has been demonstrated as potentially further valuable for improving image quality and reducing dose for CCTA images (8, 9). In addition, subtraction CCTA approaches have been investigated previously and put forward in decreasing the impacts of the calcification artifacts, improving the image quality (10) and diagnostic accuracy (6, 11–15). Both Guo et al. (10) and Xu et al. (15) reported that, in comparison with conventional CCTA, subtraction CCTA based on standard kernel iterative reconstruction would allow stenosis regarding and improve the diagnostic accuracy in coronary segments with severe calcification. Takamura et al. (11) also showed the diagnostic ability of subtraction CCTA using the low-radiation dose protocol for patients with calcification was superior to that of conventional CCTA alone.
However, the incremental diagnostic value of the DLR technique combined with subtraction CCTA image has not been fully explored yet. Therefore, we conducted this study to investigate the images quality and diagnostic value of the DLR approach combined with subtraction CCTA images in the evaluation of coronary artery stenosis that is caused by calcified lesions.
Materials and Methods
Patient Population
Consecutive patients with known or suspected coronary artery disease, who underwent coronary CTA and were scheduled for ICA within the next 1 month between March 2020 and April 2021, were initially included. ICA was performed due to a comprehensive situation and the clinical needs of a patient. Exclusion criteria were (1) history of contrast-related allergy; (2) impaired liver or renal function; (3) inability to sustain a breath-hold; (4) pregnancy; (5) non-sinus rhythm; (6) history of coronary bypass graft surgery. Figure 1 shows the patient inclusion flowchart.
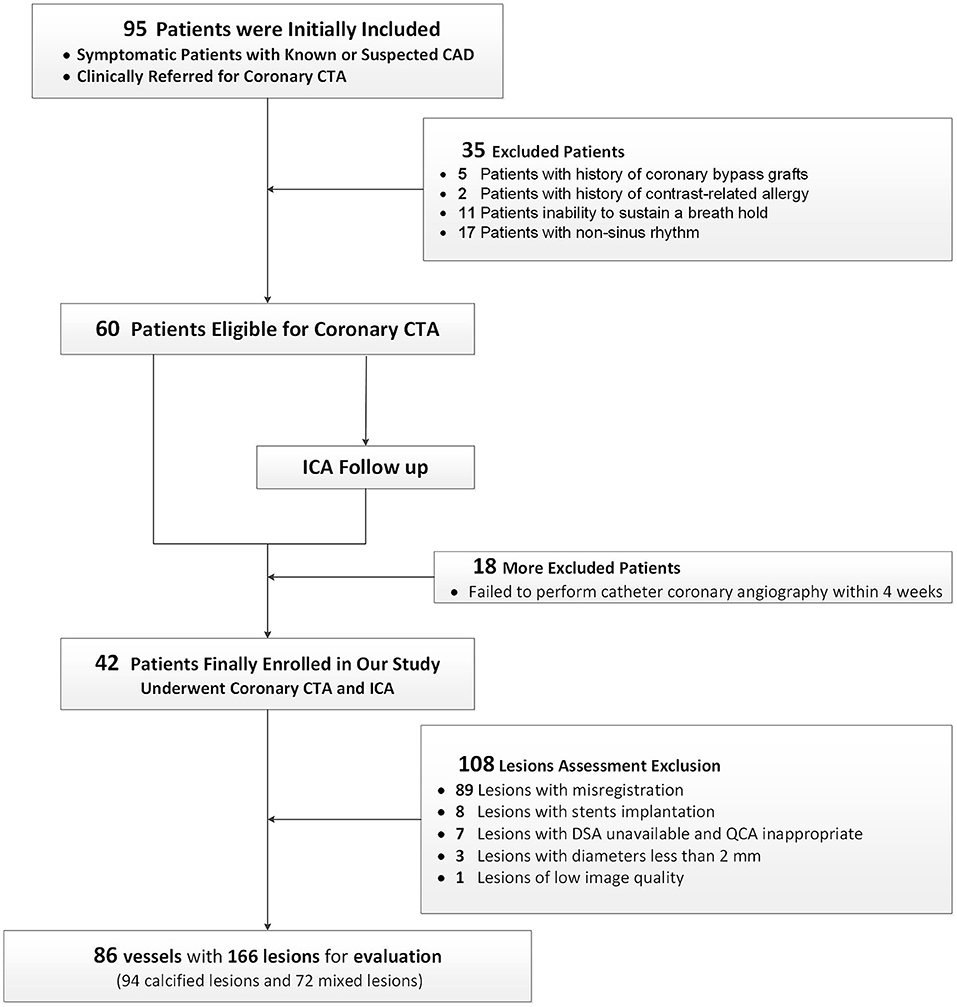
Figure 1. A flow chart. A flow chart of subject enrollment and study design. Based on the predefined inclusion and exclusion criteria, 42 patients successfully underwent CCTA and ICA. CAD, coronary artery disease; CCTA, coronary CT angiography; ICA, invasive coronary angiography.
Written informed consent was obtained from all the patients, and the study protocol was approved by the institutional ethical committee.
Coronary CTA Image Data Acquisition
The patients were scanned using a 320-row detector CT scanner (Aquilion ONE GENESIS Edition; Canon Medical Systems Corp, Japan). Sublingual nitroglycerin (1. mg) was used 1–2 min before the scan. β blockers were administrated before the examination. Using a two-breath-hold protocol, the whole scans consisted of a non-contrast scan (2–3 s), followed by a contrast-enhanced scan (5–8 s). In all the patients, the prospective one-beat CTA mode was used with the cardiac phase for scanning a set to 70–80% of the R-R interval (heart rate <75 bpm) or to 35–55% (heart rate >75 bpm). Iodinated contrast media (370 mg I/ml) was administered via a 20 G trocar in the antecubital vein by a dual-syringe power injector (DUAL SHOT GX, Nemoto-Kyorindo, Tokyo) with a protocol based on the BMI of the patients individually. The contrast agent volume was injected at a rate of body weight (kg) ×0.053 ml/s in 10 s (fixed), followed by 30-ml saline at the same injection rate. A bolus-tracking technique was used with a region of interest (ROI) placed in the descending aorta (attenuation threshold: 280 HU), and the scan started automatically with a delay time of 10 s. The scan ranged from the carina to the level of the diaphragm to include the entire heart. Other acquisition parameters were as follows: tube voltage, 100 kVp; gantry rotation time, 275 ms: z-coverage, 120–160 mm; collimation, 320 × 0.5 mm. The tube current was adjusted automatically with noise index SD = 33.
CT Image Reconstruction
The initial CCTA image data were reconstructed with Hybird-IR [Adaptive Iterative Dose Reduction (AIDR) 3D, Cardiac kernel, FC09] and DLR [Advanced Intelligent Clear-IQ Engine (AiCE), Cardiac kernel]. All reconstructions had a slice thickness of 0.5 mm with 0.5 mm intervals. Afterward, these two groups of images with their corresponding non-contrast images were sent to a dedicated post-processing software to obtain subtraction CCTA (SURESubtraction Canon Medical Systems, Otawara, Japan). The subtraction algorithm was based on the combination of global non-rigid registration and local rigid refinement, and the registered non-contrast data set was subtracted from the contrast data set to finally remove calcification (12).
The effective radiation dose was estimated as the dose length product multiplied by a conversion coefficient for the chest (0.026 mSv/mGy/cm) (16), CTDIvol, and DLP of each patient was also recorded. The total dose was calculated for pre-contrast and post-contrast scans.
Evaluation of Image Quality
The image quality was evaluated on a per-vessel basis and a per-patients basis using the Likert 4 stage score (17). Score 4: non-diagnostic, poor image quality that precluded appropriate evaluation of the coronary arteries due to severe artifacts; score 3: adequate, reduced image quality because of artifacts but sufficient to rule out obstructive CAD; score 2: good, minor artifacts but image quality was adequate for diagnostic evaluation; and score 1: excellent, absence of artifacts and no structural discontinuity. Two radiologists with 5 (CX) and 8 years (YY) of experience in cardiac radiology assessed all images independently. Any discrepancy between the observers was settled by consensus.
The mean attenuation value and noise [defined as the SD of ROI] in the aortic root and adjacent adipose tissue were measured. The mean attenuation value in the proximal segment of the three main coronary arteries [left anterior-descending artery (LAD), left circumflex (LCX), and right coronary artery (RCA)] and the adjacent adipose tissue were also measured. The signal-to-noise ratio (SNR) and contrast-to-noise ratio (CNR) of each part were calculated. The circular ROI should be as large as possible but with care taken to avoid the tube wall and calcification. The calculation formula for SNR and CNR was as follows:
Diagnostic Performance Evaluation
All the data were transferred to a post-processing workstation for stenosis analysis (Vitrea Workstation, Version 4.0693). Of all the included patients, the calcified or mixed plaques of each segment in the per vessel were selected for obstructive CAD assessment, which was defined as ≥50% luminal stenosis in diameter and area evaluation, respectively. Segments with no plaque, non-calcified plaques, stent implanted, and a diameter <2 mm or low-image quality were initially eliminated.
All the stenosis would be evaluated on a per-lesion basis on each of the four types of images as aforementioned. Parameters, including minimum diameters, maximum diameters, effective diameters, and areas, were measured at the site of the stenosis and the proximal and distal points of the stenosis based on the cross-sectional view of multiplanar reconstruction. For quantitative evaluation, the stenosis degree was calculated using the formula below:
Invasive Coronary Angiography
Invasive coronary angiography was performed on Allura Xper UNIQ FD10 (Philips Medical Systems, Nederland) using the standard Judkins technique, and images were acquired in multiple projections, where at least two orthogonal projections were obtained in order to assess target vessels. Quantitative coronary angiography (QCA) was performed using dedicated software (AngioPlus Core 1.0, Pulse Medical Imaging Technology, Shanghai, China) by an experienced analyst. Catheter calibration or isocenter calibration was used. Based on the automatically delineated lumen contours, the coronary lumen was reconstructed. Stenosis was then manually selected on a specific segment, and stenosis parameters were quantified automatically (Figures 2, 3).
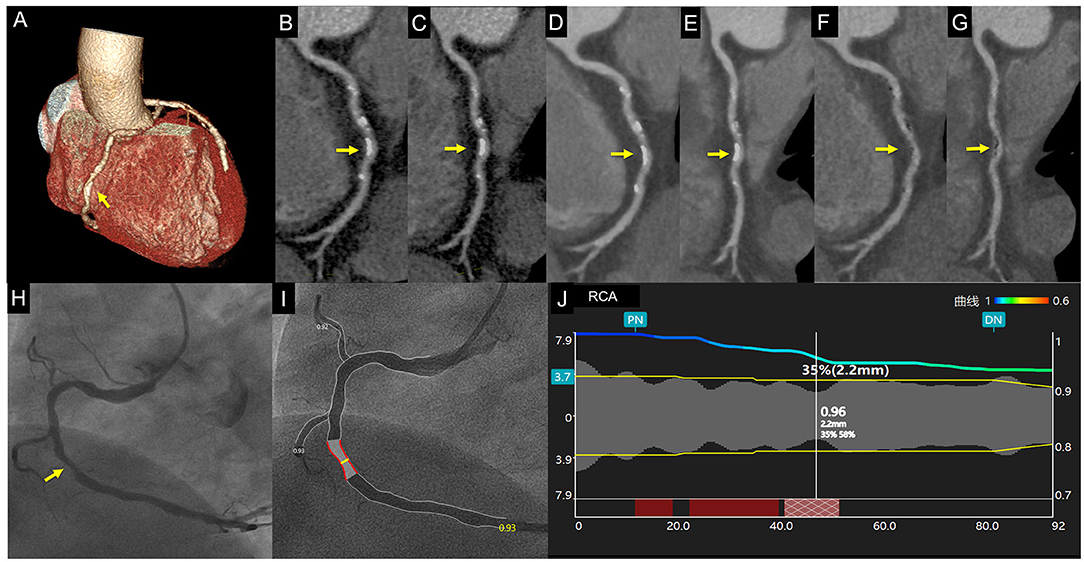
Figure 2. Case 1. A 67-year-old man with chest distress. Volume rendering and curved multiplanar reformation of traditional CTAHLR (A–C) images show ~50% stenosis of the middle right coronary artery caused by calcified plaque. CTADLR (D,E) images show superior image quality yet a similar degree of stenosis. CTAsDLR images of corresponding position (F,G) show 35% stenosis. Invasive coronary angiography (H) and quantitative coronary angiography (I,J) confirm the stenosis degree of 35%. Written informed consent was obtained from an individual for the publication of any potentially identifiable images or data included in this article. The yellow arrow shows the location of coronary stenosis.
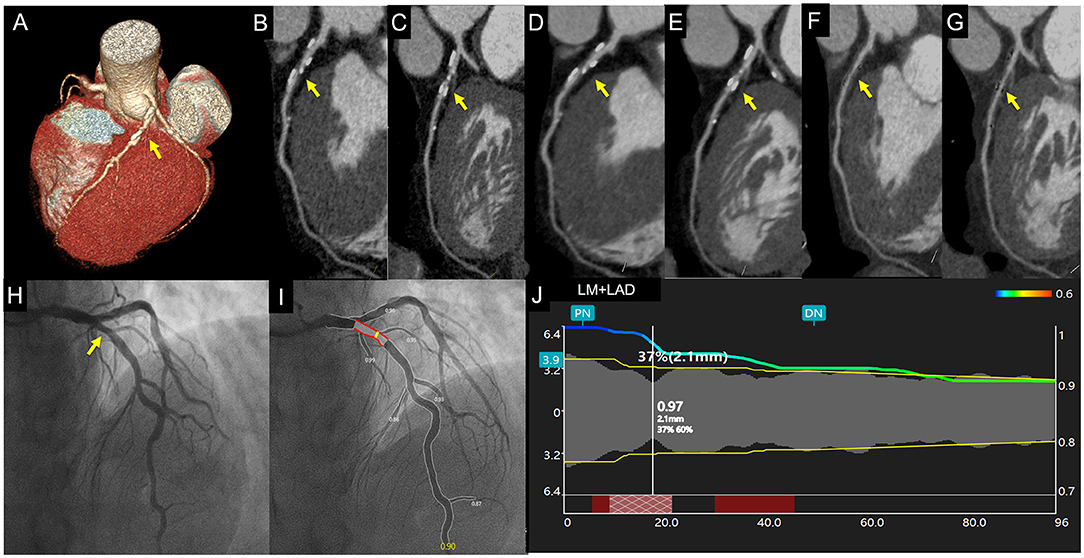
Figure 3. Case 2. A 67-year-old asymptomatic patient. Volume rendering and curved multiplanar reformation of traditional CTAHLR (A–C) images show about 65% stenosis of a proximal left anterior descending coronary artery caused by calcified plaque. CTADLR (D,E) images show superior image quality yet a similar degree of stenosis. CTAsDLR images of corresponding position (F,G) show mild stenosis (40%). Invasive coronary angiography (H) and quantitative coronary angiography (I,J) confirm the stenosis degree of 37%. Written informed consent was obtained from the individual for the publication of any potentially identifiable images or data included in this article. The yellow arrow shows the location of coronary stenosis.
Statistical Analysis
Statistical analyses were performed using R software (version 3.6.1, http://www.R-project.org). Quantitative variables were expressed as mean values ± standard deviations, and categorical variables were expressed in terms of frequency and composition ratio (%). Normally distributed continuous variables were evaluated and compared using a paired-student t-test, while the Wilcoxon test was used for continuous variables with the abnormal distribution. Image quality and stenosis assessment were compared among all four types of images: CTADLR, CTAHIR, CTAsDLR, and CTAsHIR. Interobserver agreement was assessed by using the kappa coefficient. Sensitivity, specificity, negative predictive value (NPV), positive predictive value (PPV), and diagnostic accuracy for CTA were calculated. p < 0.05 was considered statistically significant.
Results
Patient Population
Based on the predefined inclusion and exclusion criteria, a total of 42 patients (32 men and 10 women; 62.9 ± 9.3 years) were finally included (Figure 1). The characteristics of the 42 patients are specified in Table 1. Finally, 86 vessels with 166 lesions (92 calcified and 72 mixed lesions) were included for further analysis. The mean radiation dose was 3.7 ± 2.0 mSv.
Qualitative Assessment of CT Image Quality
Qualitative image quality was evaluated in a total of 42 patients with 86 coronary arteries. On a per-patient basis, the overall average image quality scores of CTADLR and CTAHIR were 1.07 ± 0.34 and 1.43 ± 0.58 (p < 0.001), and the average scores of CTAsDLR and CTAsHIR were 1.10 ± 0.29 and 1.62 ± 0.69 (p < 0.001), respectively. On a per-vessel basis, the overall average image quality scores of the three coronary arteries for CTADLR and CTAHIR were 1.16 ± 0.39 and 1.68 ± 0.71 (p < 0.001), and the average scores for CTAsDLR and CTAsHIR were 1.11 ± 0.39 and 1.49 ± 0.59 (p < 0.001), respectively.
There was a significant difference between CTA images with DLR reconstruction and HIR reconstruction in both patient-based and vessel-based IQ scores (all p < 0.001). Table 2 reports the detailed IQ scores (1–4) for qualitative image quality evaluation.
Quantitative Assessment of CT Image Quality
There was significant difference in CT attenuation and noise at the aortic root between CTADLR and CTAHIR (465.66 ± 79.31 vs. 475.50 ± 85.06, p = 0.001; 18.00 ± 3.62 vs. 26.56 ± 4.27, p < 0.001). Analogously, significant difference in CT attenuation and noise at the aortic root has been shown between CTAsDLR and CTAsHIR (420.25 ± 85.36 vs. 427.10 ± 84.28, p = 0.003; 25.25 ± 4.43 vs. 34.23 ± 7.64, p < 0.001; Table 3).
The SNR values at the aortic root of CTADLR, CTAHIR, CTAsDLR, and CTAsHIR were 27.24 ± 8.72, 18.32 ± 4.24, 17.54 ± 7.22, and 13.15 ± 4.07, respectively. The CNR values at the aortic root of CTADLR, CTAHIR, CTAsDLR, and CTAsHIR were 33.20 ± 10.05, 22.28 ± 4.85, 21.72 ± 8.26, and 16.23 ± 4.58, respectively. The SNR and CNR at the aortic root of CTA images with DLR reconstruction were significantly superior to that with HIR reconstruction for both situations with and without subtraction (all p < 0.001). Detailed results of the quantitative image analysis are listed in Table 3.
Diagnostic Performance Evaluation
About 274 calcified or mixed coronary lesions were obtained. One hundred and eight lesions were eliminated: eighty-nine lesions with misregistration, seven lesions with QCA unavailable, three vessels with diameters <2 mm, eight vessels with stent implantation, and one lesion of low-image quality. Finally, 166 calcified or mixed coronary lesions in 86 vessels of 42 patients were analyzed for diagnostic performance. The kappa value of the interobserver agreement was good (kappa = 0.82).
The sensitivity and the specificity to detect area stenosis ≥50% defined by QCA were 74.19% and 85.58% for CTAsDLR; 79.03% and 75.96% for CTADLR; 74.19% and 83.65% for CTAsHIR; 85.48% and 74.04% for CTAHIR, respectively. The sensitivity and the specificity to detect diameter stenosis ≥50% defined by QCA were 90.91% and 83.23% for CTAsDLR; 100.00% and 67.10% for CTADLR; 90.91% and 74.19% for CTAsHIR, 100.00% and 63.23% for CTAHIR, respectively.
The false positive rates of CTAsDLR, CTADLR, CTAsHIR, and CTAHIR for luminal area stenosis ≥50% were 9%, 15%, 10%, and 16%, respectively. And the corresponding false positive rates for luminal diameter stenosis ≥50% were 15%, 31%, 24%, and 34%, respectively (Figure 4).
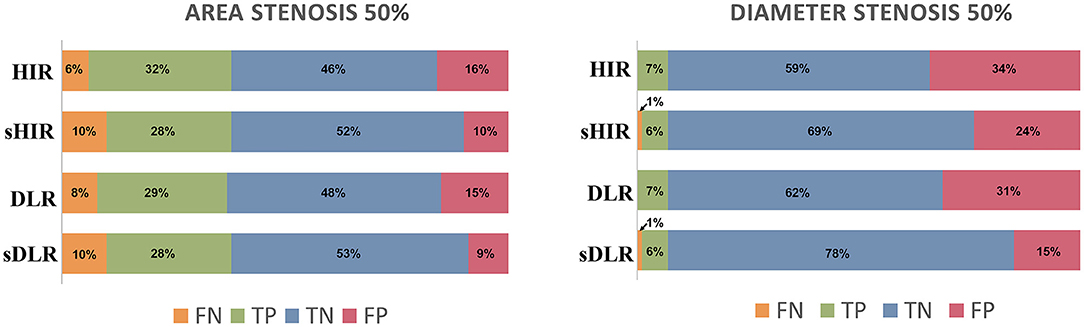
Figure 4. Stenosis evaluation. The false positive rates of CTAsDLR and CTAsHIR for luminal area stenosis ≥50% were 9 and 10%, respectively, while the corresponding values for luminal diameter stenosis ≥50% were 15 and 24%. DLR, deep learning–based image reconstruction; HIR, iterative reconstruction; sDLR, subtraction deep learning–based image reconstruction; sHIR, subtraction iterative reconstruction; TP, true positive; FP, false positive; TN, true negative; FN, false negative.
The diagnostic accuracies of CTAsDLR, CTADLR, CTAsHIR, and CTAHIR to identify calcification-related obstructive area stenosis and diameter stenosis were 81.33%, 77.11%, 80.12%, and 78.31%, respectively; and 83.73%, 69.28%, 75.30%, and 65.66%, respectively. The CTAsDLR with calcification-related obstructive area stenosis evaluation showed the highest diagnostic performance. Table 4 shows the detailed diagnostic values of the CTA images with different reconstruction techniques.
In the patient-based analysis, ICA identified 30 patients with significant stenosis (positive) and 12 patients without significant stenosis (negative). CTAHIR accurately identified 8 negative patients and 25 positive patients and misjudged 9 patients. CTADLR accurately identified 11 negative patients and 22 positive patients and misjudged 9 patients. CTAsDLR identified all negative patients and 24 positive patients but misjudged 6 patients. Compare to HIR combined with subtraction technique, additional three patients (33%) were correctly diagnosed using DLR combined with subtraction technique.
Discussion
This study has two main findings: (1) The image quality of CTA images with deep learning-based reconstruction (DLR) was improved compared to that of CTA images with hybrid iterative reconstruction (IR). (2) DLR technique combined with subtraction CTA images enhanced diagnostic performance for coronary stenosis evaluation of calcified plaques.
The diagnostic performance of traditional coronary computed tomography angiography imaging was limited in the event of calcified plaques, especially for the diffuse or large calcification with intermediate to a high degree of coronary artery calcium score (18, 19). DLR has been developed and utilized in recent years. Previous studies (8, 9) reported that DLR helps in reducing image noise in coronary CTA compared to iterative reconstructions. Our study demonstrated consistent results with that. This can be mainly attributed to higher spatial resolution and better image-noise reduction, which are inherited from the high-quality model-based IR (MBIR) images by means of DLR image training (8, 20).
Besides the image quality improvement with DLR, the diagnostic accuracy could be further increased when coronary subtraction was integrated. Previous studies (15, 21) have concluded diagnostic value enhancement of subtraction CTA in severe calcifications using HIR. Xu et al. (15) showed better diagnostic accuracy and confidence in cases of severe calcification with HIR-based subtraction CCTA information. Vilades Medel et al. (22) reported that subtraction CTA is promising in overcoming the limitations of conventional CTA due to calcium or metal artifacts. In a prospective coronary subtraction multicenter trial, Fuchs et al. (6) further demonstrated that HIR-based subtraction CTA reduced the false-positive rate in well-aligned, calcified segments. The accuracy calculations in target segments without misregistration showed a reduction of the false positives from 72% in conventional CTA to 33% in subtraction CTA.
To our knowledge, this is the first study that evaluated the diagnostic value of DLR combined with subtraction CTA images for calcified-specific stenosis by using ICA as the reference standard. In this study, our results of CTAsHIR for identifying luminal diameter stenosis ≥50% showed a comparative false positive rate of 30%, which was in accordance with that of Fuchs et al. (6). Moreover, we have successfully reduced the false positive rate of CTAsDLR for ≥50% luminal diameter stenosis to 15% (Figures 2, 3). As Fuchs et al. (6) reported, they reduced the false-positive rate in subtraction CTA and came at the expense of 7% false-negative segments, while, in this study, the results showed the corresponding false-negative rate was only 1%. The diagnostic performance of subtraction CCTA is further improved by using DLR with increased specificity (85.58% vs. 74.19%, p <0.001) and accuracy (83.73% vs. 75.30%, p <0.001). The refined results might be attributed to the enhanced coronary subtraction process with the inputting image quality improvement of the DLR reconstruction approach, i.e., image-noise reduction, blooming artifacts mitigation, and spatial resolution enhancement. In addition, in our study, we found that the false positive rate of CTAsDLR for luminal area stenosis ≥50% has been further reduced to 9%. This may support that the area assessment may also provide valuable information in luminal stenosis evaluation, which could be further analyzed.
The main limitations of this study include the following: First, the sample size of this single-center study was relatively small, it was not capable of detailed analysis based on subtype levels of coronary plaques or gender analysis, and bias might exist since the average calcification degree of patients in this study was not that severe. However, patients with significant coronary stenosis and obstructive CAD might be more likely to be scheduled for ICA directly. Second, to be consistent with the previous studies, the stenosis calculation, including proximal and distal vessels diameters, might carry some limitations since vessel caliber could change abruptly along the vessel course, and we attempted hard to avoid this situation during measurement. Third, subtraction CTA can be challenging due to the periodical heart motion and variation of breath-holding cooperation from the patients; only ones with expected good image quality might be suitable or successful for the subtraction exploration. Although the misregistration artifacts in this study were significantly lower than previously reported (32.5 vs. 50%) (6), further modified schemes, e.g., one-breath protocol or individualization scanning protocol would be needed.
To validate the clinical reliability and reproducibility of DLR combined with subtraction CTA, multicenter prospective trials will be needed. And based on the better imaged quality of DLR subtraction CTA, lower radiation dose protocol, and functional evaluation need to be investigated in subsequent research to further optimize the CCTA performance for patients with obstructive CAD.
In conclusion, the study demonstrated that deep learning–based image reconstruction could improve the image quality of CTA images and diagnostic performance for calcification-related obstructive CAD, especially when combined with subtraction technique.
Data Availability Statement
The raw data supporting the conclusions of this article will be made available by the authors, without undue reservation.
Ethics Statement
The studies involving human participants were reviewed and approved by Peking Union Medical College Hospital, Chinese Academy of Medical Sciences and Peking Union Medical College. The patients/participants provided their written informed consent to participate in this study.
Author Contributions
YY, CX, MX, JY, and Y-NW were contributed to the conception and design, data measurement, and writing the article or revision of the article. Y-YL, S-JY, JW, and Y-BG were contributed to data measurement. YW, Y-ML, and Z-YJ were contributed to study implementation. All authors approved the final manuscript submitted.
Funding
This research was supported by Beijing Natural Science Foundation (Grant No. Z210013, 2021); the China Postdoctoral Science Foundation (Grant No. 2020T130071, 2020); the CAMS Innovation Fund for Medical Sciences (CIFMS) (Grant No. 2020-I2M-C&T-B-034); the National Natural Science Foundation of China (Grant No. 81873891, 2019); and the Major International (Regional) Joint Research Project of National Natural Science Foundation of China (Grant No. 82020108018, 2021).
Conflict of Interest
MX, JY, and JW were employed by Canon Medical System (China) Co., Ltd.
The remaining authors declare that the research was conducted in the absence of any commercial or financial relationships that could be construed as a potential conflict of interest.
Publisher's Note
All claims expressed in this article are solely those of the authors and do not necessarily represent those of their affiliated organizations, or those of the publisher, the editors and the reviewers. Any product that may be evaluated in this article, or claim that may be made by its manufacturer, is not guaranteed or endorsed by the publisher.
Acknowledgments
We would like to thank Wen-Yu Yin for technical support during image processing.
References
1. Montalescot G, Sechtem U, Achenbach S, Andreotti F, Arden C, Budaj A, et al. 2013 ESC guidelines on the management of stable coronary artery disease: the Task Force on the management of stable coronary artery disease of the European Society of Cardiology. Eur Heart J. (2013) 34:2949–3003. doi: 10.1093/eurheartj/eht296
2. Görich HM, Buss SJ, Emami M, Seitz S, Lossnitzer D, Fortner P, et al. Short-time changes in coronary artery plaques assessed by follow-up coronary CT angiography-characteristics and impact on patient management. Front Cardiovasc Med. (2021) 8:691665. doi: 10.3389/fcvm.2021.691665
3. Feldman DI, Latina J, Lovell J, Blumenthal RS, Arbab-Zadeh A. Coronary computed tomography angiography in patients with stable coronary artery disease. Trends Cardiovasc Med. (2021). doi: 10.1016/j.tcm.2021.08.009. [Epub ahead of print].
4. Zhang LJ, Wang Y, Schoepf UJ, Meinel FG, Bayer RR II, Qi L, et al. Image quality, radiation dose, and diagnostic accuracy of prospectively ECG-triggered high-pitch coronary CT angiography at 70 kVp in a clinical setting: comparison with invasive coronary angiography. Eur Radiol. (2016) 26:797–806. doi: 10.1007/s00330-015-3868-z
5. Arbab-Zadeh A, Miller JM, Rochitte CE, Dewey M, Niinuma H, Gottlieb I, et al. Diagnostic accuracy of computed tomography coronary angiography according to pre-test probability of coronary artery disease and severity of coronary arterial calcification. The CORE-64 (coronary artery evaluation using 64-row multidetector computed tomography angiography) international multicenter study. J Am Coll Cardiol. (2012) 59:379–87. doi: 10.1016/j.jacc.2011.06.079
6. Fuchs A, Kuhl JT, Chen MY, Vilades Medel D, Alomar X, Shanbhag SM, et al. Subtraction CT angiography improves evaluation of significant coronary artery disease in patients with severe calcifications or stents-the C-Sub 320 multicenter trial. Eur Radiol. (2018) 28:4077–85. doi: 10.1007/s00330-018-5418-y
7. Zhang S, Levin DC, Halpern EJ, Fischman D, Savage M, Walinsky P. Accuracy of MDCT in assessing the degree of stenosis caused by calcified coronary artery plaques. AJR Am J Roentgenol. (2008) 191:1676–83. doi: 10.2214/ajr.07.4026
8. Tatsugami F, Higaki T, Nakamura Y, Yu Z, Zhou J, Lu Y, et al. Deep learning-based image restoration algorithm for coronary CT angiography. Eur Radiol. (2019) 29:5322–9. doi: 10.1007/s00330-019-06183-y
9. Benz DC, Benetos G, Rampidis G, von Felten E, Bakula A, Sustar A, et al. Validation of deep-learning image reconstruction for coronary computed tomography angiography: impact on noise, image quality and diagnostic accuracy. J Cardiovasc Comput Tomogr. (2020) 14:444–51. doi: 10.1016/j.jcct.2020.01.002
10. Guo W, Tripathi P, Yang S, Qian J, Rai B, Zeng M. Modified subtraction coronary CT angiography with a two-breathhold technique: image quality and diagnostic accuracy in patients with coronary calcifications. Korean J Radiol. (2019) 20:1146–55. doi: 10.3348/kjr.2018.0845
11. Takamura K, Fujimoto S, Kawaguchi Y, Kato E, Aoshima C, Hiki M, et al. The usefulness of low radiation dose subtraction coronary computed tomography angiography for patients with calcification using 320-row area detector CT. J Cardiol. (2019) 73:58–64. doi: 10.1016/j.jjcc.2018.05.015
12. Yoshioka K, Tanaka R, Takagi H, Nagata K, Chiba T, Takeda K, et al. Diagnostic accuracy of a modified subtraction coronary CT angiography method with short breath-holding time: a feasibility study. Br J Radiol. (2016) 89:20160489. doi: 10.1259/bjr.20160489
13. Amanuma M, Kondo T, Sano T, Sekine T, Takayanagi T, Matsutani H, et al. Subtraction coronary computed tomography in patients with severe calcification. Int J Cardiovasc Imaging. (2015) 31:1635–42. doi: 10.1007/s10554-015-0746-3
14. Fuchs A, Kuhl JT, Chen MY, Helqvist S, Razeto M, Arakita K, et al. Feasibility of coronary calcium and stent image subtraction using 320-detector row CT angiography. J Cardiovasc Comput Tomogr. (2015) 9:393–8. doi: 10.1016/j.jcct.2015.03.016
15. Xu L, Li F, Wu K, Zhong Z, Huang R, Xu Y, et al. Subtraction improves the accuracy of coronary CT angiography for detecting obstructive disease in severely calcified segments. Eur Radiol. (2021) 31:6211–9. doi: 10.1007/s00330-021-08092-5
16. Trattner S, Halliburton S, Thompson CM, Xu Y, Chelliah A, Jambawalikar SR, et al. Cardiac-specific conversion factors to estimate radiation effective dose from dose-length product in computed tomography. JACC Cardiovasc Imaging. (2018) 11:64–74. doi: 10.1016/j.jcmg.2017.06.006
17. Pflederer T, Rudofsky L, Ropers D, Bachmann S, Marwan M, Daniel WG, et al. Image quality in a low radiation exposure protocol for retrospectively ECG-gated coronary CT angiography. AJR Am J Roentgenol. (2009) 192:1045–50. doi: 10.2214/ajr.08.1025
18. Carrascosa P, Leipsic JA, Deviggiano A, Capunay C, Vallejos J, Goldsmit A, et al. Virtual monochromatic imaging in patients with intermediate to high likelihood of coronary artery disease: impact of coronary calcification. Acad Radiol. (2016) 23:1490–7. doi: 10.1016/j.acra.2016.08.002
19. Yan RT, Miller JM, Rochitte CE, Dewey M, Niinuma H, Clouse ME, et al. Predictors of inaccurate coronary arterial stenosis assessment by CT angiography. JACC Cardiovasc Imaging. (2013) 6:963–72. doi: 10.1016/j.jcmg.2013.02.011
20. Geyer LL, Schoepf UJ, Meinel FG, Nance JW Jr., Bastarrika G, Leipsic A, et al. State of the art: iterative CT reconstruction techniques. Radiology. (2015) 276:339–57. doi: 10.1148/radiol.2015132766
21. Yoshioka K, Tanaka R, Muranaka K, Sasaki T, Ueda T, Chiba T, et al. Subtraction coronary CT angiography using second-generation 320-detector row CT. Int J Cardiovasc Imaging. (2015) 31(Suppl. 1):51–8. doi: 10.1007/s10554-015-0630-1
Keywords: deep learning, subtraction technique, computed tomography angiography, vascular calcification, coronary artery disease
Citation: Yi Y, Xu C, Xu M, Yan J, Li Y-Y, Wang J, Yang S-J, Guo Y-B, Wang Y, Li Y-M, Jin Z-Y and Wang Y-N (2021) Diagnostic Improvements of Deep Learning–Based Image Reconstruction for Assessing Calcification-Related Obstructive Coronary Artery Disease. Front. Cardiovasc. Med. 8:758793. doi: 10.3389/fcvm.2021.758793
Received: 15 August 2021; Accepted: 01 October 2021;
Published: 03 November 2021.
Edited by:
Grigorios Korosoglou, GRN Klinik Weinheim, GermanyReviewed by:
Gitsios Gitsioudis, Universitätsklinikum Ulm, GermanyPhilipp Thomas Fortner, The Radiological Center, Germany
Copyright © 2021 Yi, Xu, Xu, Yan, Li, Wang, Yang, Guo, Wang, Li, Jin and Wang. This is an open-access article distributed under the terms of the Creative Commons Attribution License (CC BY). The use, distribution or reproduction in other forums is permitted, provided the original author(s) and the copyright owner(s) are credited and that the original publication in this journal is cited, in accordance with accepted academic practice. No use, distribution or reproduction is permitted which does not comply with these terms.
*Correspondence: Yi-Ning Wang, wangyining@pumch.cn
†These authors have contributed equally to this work and share first authorship