African Ancestry Individuals with Higher Educational Attainment Are Resilient to Alzheimer’s Disease Measured by pTau181
Abstract
Background:
Cognitive and functional abilities in individuals with Alzheimer’s disease (AD) pathology (ADP) are highly variable. Factors contributing to this variability are not well understood. Previous research indicates that higher educational attainment (EA) correlates with reduced cognitive impairments among those with ADP. While cognitive and functional impairments are correlated, they are distinguishable in their manifestations.
Objective:
To investigate whether levels of education are associated with functional impairments among those with ADP.
Methods:
This research involved 410 African American (AA) individuals (Institutional Review Boards 20070307, 01/27/2023) to ascertain whether EA correlates with functional resilience and if this effect varies between APOE ɛ4 carriers and non-carriers. Utilizing EA as a cognitive reserve proxy, CDR-FUNC as a functional difficulties measure, and blood pTau181 as an ADP proxy, the non-parametric Mann-Whitney U test assessed the relationship between EA and CDR-FUNC in individuals with advanced pTau181 levels.
Results:
The results showed that EA correlated with functional difficulties in AA individuals with high levels of pTau181, such that individuals with high EA are more likely to have better functional ability compared to those with lower EA (W = 730.5, p = 0.0007). Additionally, we found that the effect of high EA on functional resilience was stronger in ɛ4 non-carriers compared to ɛ4 carriers (W = 555.5, p = 0.022).
Conclusion:
This study extends the role of cognitive reserve and EA to functional performance showing that cognitive reserve influences the association between ADP burden and functional difficulties. Interestingly, this protective effect seems less pronounced in carriers of the strong genetic risk allele ɛ4.
INTRODUCTION
Alzheimer’s disease (AD) is the most common type of dementia. Older African ancestry individuals in US having almost two times higher prevalence and incidence compared with older non-Hispanic Whites (NHW), but substantially underrepresented in AD studies [1, 2]. Several factors influence the incidence, prevalence, and risk of AD. These include genetic, clinical, social, behavioral, and environmental determinants [3–8].
The characteristic neuropathological changes of AD, the presence of extracellular amyloid-β deposits and intracellular aggregation of neurofibrillary tangles, can now be measured using biomarkers [9, 10]. These include neuroimaging, cerebrospinal fluid (CSF), and blood-based biomarkers [9, 10]. Recent developments in the field of blood biomarkers showed that blood pTau181 levels strongly correlate with abnormal amyloid accumulation, tau deposition, and neurodegeneration [11–13]. The blood pTau181 levels have been demonstrated to differ significantly between diagnostic groups with amyloid, tau, and FDG-PET scans, further validating pTau181 as a robust biomarker for AD related pathology (ADP) [14, 15].
ADP is typically associated with the decline in cognitive, functional, and behavioral abilities seen in AD [10]. However, some studies have shown that even with advanced ADP, a subgroup of individuals can still maintain reasonable cognition and function, which reflects a better-than-expected performance in relation to the degree of ADP [16, 17]. This inconsistency between ADP and clinical symptoms underscores a possible phenotype characterized by biological pathology without clinical impairment.
‘Cognitive reserve’ (CR) may account for some of this protection from ADP [18, 19]. CR is thought to be the various thinking abilities that actively compensate for the deficits imposed by the ADP [20]. This compensation yields cognitive performance that exceeds expectations when considering life-course-related brain changes and brain injuries or disease [21].
Educational attainment (EA) is a commonly used correlate of CR. Higher EA tends to foster resilience against AD clinical manifestations, often resulting in improved cognitive performance compared to those with less education but similar levels of ADP [22–25]. Some studies also suggest an interplay between EA and the APOE ɛ4 allele, the strongest genetic risk factor for late-onset AD [26–28]. This complex relationship indicates that CR and genetic factors might interact and influence cognitive outcomes. Intriguingly, research from the MacArthur Study of Successful Aging indicates that the protective effects of education are less pronounced in APOE ɛ4 carriers, as measured by the Short Portable Mental Status Questionnaire [29]. Similar findings have been observed in APOE ɛ4 carriers during normal aging, where the protective effects of education are attenuated, possibly correlating with a more rapid cognitive decline [30]. However, the relationship between the APOE ɛ4 allele and functional abilities shows mixed results in prior studies. Some research indicates no association between APOE ɛ4 and functional status decline [31, 32], while others report greater difficulties in overall Instrumental Activities of Daily Living (IADL) capacity for APOE ɛ4 carriers [33]. These findings highlight the nuanced interaction between EA and the APOE ɛ4 in cognitive performance. However, the impact of EA on specific functional abilities, such as managing finances, engaging in social interactions, and handling personal care, particularly in the context of the APOE ɛ4 allele, remains unexplored. Our study addresses this gap by investigating the novel aspect of how high EA influences these functional abilities in the presence of genetic risk factor APOE. To our knowledge, this specific interplay has not been studied, marking a significant contribution of our research. We specifically investigated whether EA, a proxy for cognitive reserve, promotes functional resilience in African American (AA) individuals with advanced levels of pTau181 (used as a proxy for ADP) and whether this effect differs between carriers and non-carriers of the APOE ɛ4 allele.
MATERIALS AND METHODS
Study samples
We ascertained 410 AA participants through AD genetics studies from Wake Forest University (WF, North Carolina), and University of Miami (UM, Florida). These participants represented a diverse AA cohort across both Florida and North Carolina sites. All participants were classified as AA based on self-reported race-ethnicity. It is essential to acknowledge that race-ethnicity is dynamic, shaped by geographic, cultural, and sociopolitical forces and are social constructs. Therefore, while participants self-identified as AA, this classification reflects a complex interplay of social, cultural, and historical influences, rather than a distinct biological identity. The inclusion criteria for the study were having CDR scores, reported years of education, APOE genotypes, and blood pTau181 AD biomarker data. All participants or their consenting proxy provided written informed consent as part of the study protocols approved by the site-specific Institutional Review Boards (20070307, 01/27/2023).
Individual ancestral backgrounds were confirmed using genome-wide genetic data with EIGENSTRAT software [34]. Population substructure data sets were compared with those in the 1000 Genome reference panel YRI (Yoruba from Nigeria) and CEU (Utah Residents with Northern and Western European ancestry) populations. Outliers with respect to CEU population (overlapping within the cluster of CEU) were removed from the datasets [35].
Ascertainment protocols have been consistent across the sites and clinical data assessments capture sociodemographic information including years of education, medical and family history, dementia staging, AD/dementia symptoms, neuropsychological abilities, functional capabilities, and behavioral impairments. The CDR was used to evaluate functional capabilities and served as an outcome measure as described below in the Statistical Analysis section. Biomaterials were also collected at the time of study entry by trained phlebotomists. The detailed description of the study samples, recruitment and cognitive assessment are described elsewhere [27]. 253 participants were diagnosed as non-Cognitively Impaired (nCI), 12 as mild cognitively impaired (MCI), and 145 with Alzheimer disease (AD).
Genotyping
Genome-wide single-nucleotide polymorphism (SNP) genotyping was processed on a Global Screening Array and APOE genotyping was performed as described in Saunders et al. [36].
Measurement of serum AD biomarkers pTau181
Serum concentrations of pTau181 were measured using SIMOA chemistry implemented on the Quanterix HD-X instrument (Quanterix, Billerica, MA, USA) [37] according to manufacturer’s instructions for the pTau181 Advantage V2 assay (catalog #103714). Samples were randomized according to age, sex, and diagnosis and assayed in duplicate on each plate. Means of two measures were used in analysis. Biomarker levels were log10-transformed to satisfy normality assumptions. Samples were removed if biomarker levels were greater than three standard deviations from the mean of the rest of the samples.
CDR-FUNC
The Clinical Dementia Rating scale (CDR) is a semi-structured interview that assesses cognitive and functional impairment associated with AD to allow for staging based on a global CDR score [38]. The global CDR score is derived from box scores assigned to six domains: Memory, Orientation, Judgment and Problem solving, Home and Hobbies, Community Involvement, and Personal Care. Various studies have examined the utility of these box scores for clinical and research purposes [39–41]. The application of box scores in the assessment of functional impairment has also been studied. For example, Sudo et al. developed a composite score designed to assess functional difficulties (CDR-FUNC) [42]. This score is based on the sum of CDR box scores that are aligned with functional performance (Problem solving, Home and Hobbies, Community Involvement, and Personal Care) and that may have a different trajectory than CDR based cognitive abilities. In addition, Sudo and colleagues reported that the CDR-FUNC score is strongly correlated with the functional performance as measured by the Pfeffer’s Functional Activities Questionnaire (FAQ) [42, 43].
Statistical analysis
We hypothesized that individuals with high EA and high levels of pTau181 would present less functional difficulties compared to their counterparts with low EA. We further investigated whether EA correlates with the functional resilience (abilities managing money, interacting with others, and caring for personal needs) differently between APOE ɛ4 allele carriers and non-carriers. To model our hypothesis, we used EA as a proxy for cognitive reserve, CDR-FUNC as a measure for functional difficulties, and pTau181 as a proxy for ADP. We categorized and quantified the variables as described below:
EA. We stratified years of education into two categories: low EA, individuals up to high school (≤8 years), and high EA, individuals high school and beyond it (>8 years) [27].
CDR-FUNC. Using the non-memory components of the CDR, Judgment and Problem solving, Home and Hobbies, Community Involvement, and Personal Care, we formulated a composite score by totaling the individual score of the 4 components with the range of 0 to 3 [42]. The CDR-FUNC score then ranges from 0 to 12, with lower numbers indicating no to mild functional difficulties and higher scores indicating more severe difficulties.
ADP (pTau181). We defined advanced ADP individuals as those having log10(pTau181) level greater than one standard deviation above the mean. This threshold was selected to identify individuals with notably higher pTau181 levels, reflecting a substantial deviation from the average. This approach is consistent with our aim to categorize those with advanced ADP pathology, while acknowledging the absence of a universally established criterion in ADP research.
Statistical test. To examine the relationship between EA and CDR-FUNC in individuals with advanced ADP, we used the non-parametric Mann Whitney U Test (MW). First, we conducted the analysis using the entire sample of advanced ADP individuals. Next, to determine whether the protective effect of high EA on functional resilience is modified by the presence of the ɛ4 allele, a genetic risk factor for AD, we stratified our sample by ɛ4 status (carriers and non-carriers) and re-analyzed the association between EA and CDR-FUNC in each subgroup. To better understand the influence of age on our analysis, we stratified our samples into subgroups based on an age threshold of 80. This stratification allowed for a more nuanced exploration of age-specific impacts on the relationship between EA and CDR-FUNC.
RESULTS
The study sample consisted of 73.9% females, with a mean age of 71.08 (SD = 7.42) years and a mean log10(pTau181) level of −0.01 (SD = 0.42) (Table 1). A total of 74 individuals were classified as having high log10(pTau181) level (mean + 1SD), with 43 carrying the APOE ɛ4 allele and 31 not carrying it. Our downstream analysis was performed using these 74 individuals, selected based on their classification of having high log10(pTau181) levels (Table 2).
Table 1
Study samples characteristics
Characteristics | Low EA | High EA |
Count | 42 | 368 |
Age (mean [SD]) | 76.7 [6.06] | 70.4 [7.3] |
Sex (% female) | 64.30% | 75.00% |
APOE ɛ4 (% ɛ4 carriers) | 28.57% | 26.36% |
log10(pTau181) (mean [SD]) | 0.20 [0.48] | −0.04 [0.41] |
Wake Forest University | 30 | 323 |
University of Miami | 12 | 45 |
Table 2
Characteristics of 74 selected individuals with high log10 (pTau181) levels
Characteristics | Low EA | High EA |
Count | 16 | 58 |
Age (mean [SD]) | 83.6 [7.2] | 76.0 [8.2] |
Sex (% female) | 50.0% | 81.0% |
APOE ɛ4 (% ɛ4 carriers) | 62.5% | 56.7% |
log10(pTau181) (mean [SD]) | 0.69 [0.16] | 0.57 [0.17] |
Wake Forest University | 6 | 31 |
University of Miami | 10 | 27 |
Individuals with high log10(pTau181) and low EA had significantly higher CDR-FUNC scores (median: 12.0; Q1:8.0 –Q3:12.0) compared to those with high EA (median: 3.75; Q1:0 –Q3:9.0; W = 730.5, p = 0.0007). The distribution of CDR-FUNC scores between low and high EA in individuals with high log10(pTau181) is illustrated in Fig. 1A.
Fig. 1
Box plot for the comparison between educational attainment (EA) levels (low and high) and CDR-FUNC score among advanced Alzheimer disease pathology individuals. (A) compare all individuals with the advanced Alzheimer disease pathology, (B) compare within subgroups stratified by the APOE ɛ4 allele, and (C) compare samples further stratified by age to investigate potential age-specific trends.
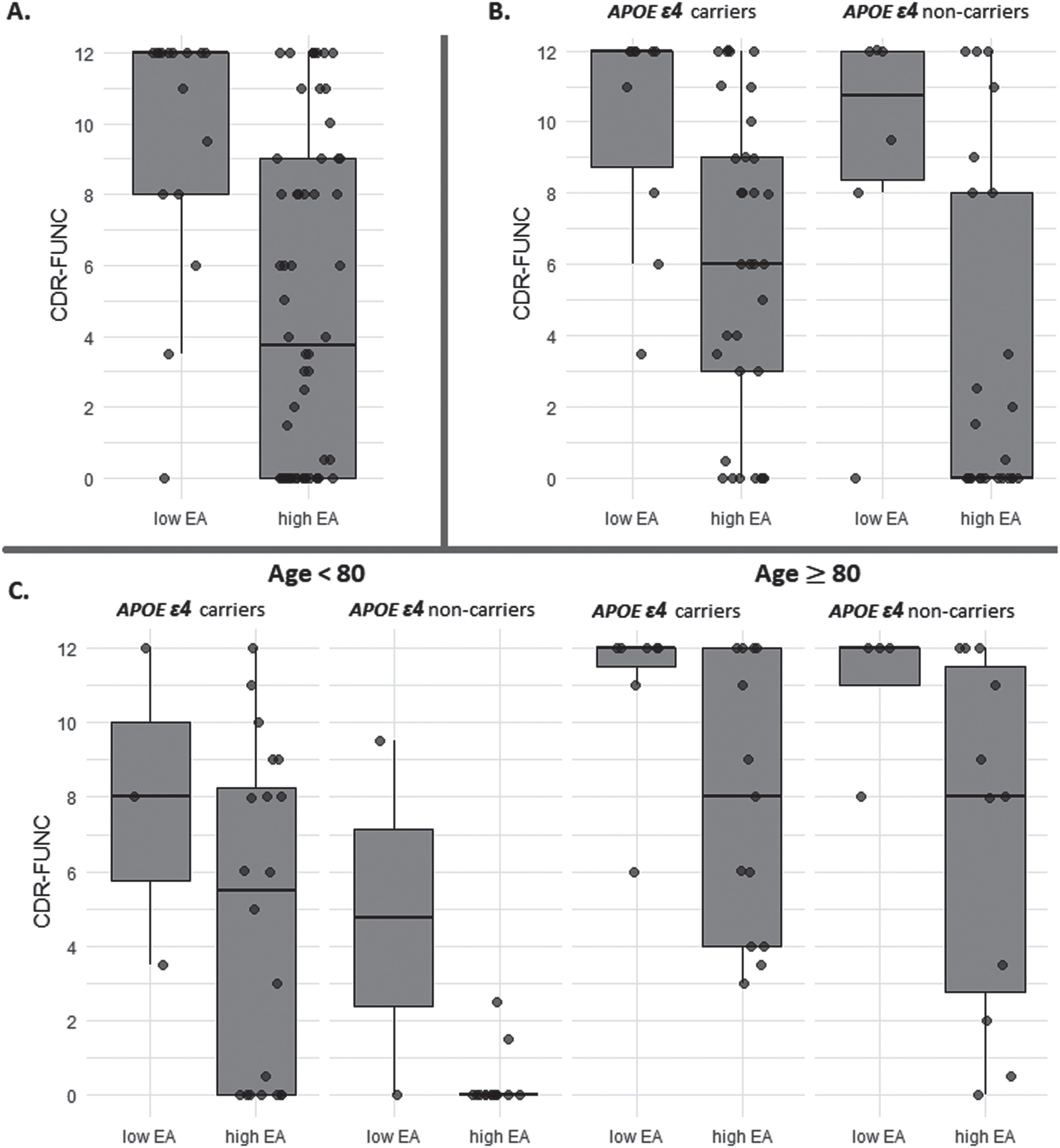
To determine the effect of the APOE ɛ4 allele on the association between EA and CDR-FUNC in high log10(pTau181) individuals, we examined this relationship separately in ɛ4 carriers and non-carriers. We found that in both subgroups, individuals with low EA had significantly higher CDR-FUNC scores (ɛ4 allele carriers: median = 12.0; Q1:8.75 –Q3:12.0; ɛ4 allele non-carriers: median = 10.75, Q1:8.375–Q3:12) than those with high EA (ɛ4 allele carriers: median = 6.0; Q1:3.0–Q3:9.0; W = 256.5, p = 0.0008; ɛ4 allele non-carriers: median = 0, Q1:0–Q3:8; W = 117, p = 0.029). However, the mean ranks of CDR-FUNC among high EA individuals were significantly higher in ɛ4 carriers compared to ɛ4 non-carriers (W = 555.5, p = 0.022). We did not observe a significant difference in CDR-FUNC score between ɛ4 carriers and non-carriers with low EA (W = 33.5, p = 0.719). Figure 1B shows the distribution of CDR-FUNC scores among advanced ADP individuals with low and high EA, stratified by ɛ4 allele.
We further stratified our samples by age to investigate potential age-specific trends. Notably, the patterns distinguishing low and high EA in these age-based subgroups were consistent with those observed in our primary dataset (Age≥80: W = 200.5, p = 0.011 and Age < 80: W = 124.5, p = 0.078) (Fig. 1C). Among high EA individuals under the age of 80, significant differences in the mean ranks of CDR-FUNC were found between ɛ4 carriers and non-carriers (W = 222, p = 0.002). However, this distinction was not evident in individuals aged 80 and above (W = 79.5, p = 0.659).
DISCUSSION
This study found that years of education are associated with better functional abilities in individuals with high log10(pTau181) levels. This effect was observed in both APOE ɛ4 carriers and non-carriers, but the protective effect of high EA was stronger in non-carriers. These results extend our understanding of the protective effect that cognitive reserve and EA may have on the functional performance of individuals with AD pathology [16, 17, 22–25]. Based on these findings, it is likely that increased years of education may explain why individuals with known genetic and AD biomarkers on average exhibit better functional performance. It can be inferred that higher education can help individuals maintain their functional abilities even with AD pathology.
Our results highlight a complex relationship among the genetic risk factors, ADP-associated biomarkers, and cognitive reserve and their effect on cognitive and functional outcomes of AD. The study supports the hypothesis, as suggested by Stern et al. [25], that the brain’s adaptability and efficiency in cognitive processing can mitigate the impacts of disease-related changes. This is evident in the varying functional outcomes among individuals with high log10(pTau181) levels, where those with high EA demonstrate a more robust cognitive reserve. This reserve, influenced by EA as per the model, is likely to enable the use of alternative neural networks to maintain cognitive function, aligning with the hypothesis by Stern et al. [20]. Particularly, the interaction between EA, genetic factors such as the APOE ɛ4 allele, and AD pathology suggest that cognitive reserve’s protective effect is modulated by genetic risk factors. This underscores the role that cognitive reserve, shaped by life experiences and education, plays in determining an individual’s resilience to neurological changes and emphasizes its importance in AD management. By investigating potential interactions among EA, ADP, and other genetic and modifiable risk and protective factors for AD, such as lifestyle factors (e.g., diet, exercise, sleep), or environmental exposures (e.g., air pollution, stress) we can enhance our understanding of the relative significance of these factors in the development of AD. This knowledge can then inform the design of comprehensive interventions aimed at preventing or delaying the onset of AD.
The trend of higher CDR-FUNC scores being associated with lower EA persists across stratified age groups. This suggests that the protective effect of high EA on functional difficulties is a consistent factor, regardless of age. However, it is noteworthy that the sample sizes decrease significantly in the stratified age groups. This reduction in sample size limits the ability to draw significant inferences about the impact of education and APOE ɛ4 carrier status on CDR-FUNC scores within these age brackets. Additionally, CDR-FUNC scores tend to be higher in the older age group (≥80 years). This is indicative of a natural progression of cognitive and functional decline with increasing age, which is to be expected in an aging population and particularly in those who are already experiencing AD pathology.
Findings from this study should be interpreted with caution considering several methodological and instrumentation limitations. First, the study population is relatively small and is not representative of all African American populations. Therefore, we are unable to make universal claims that our findings will be observed in all individuals with African ancestry. Additionally, the limited sample sizes in certain subgroups, as indicated in Fig. 1, may constrain the strength of specific statistical inferences. Second, our operationalization of years in school as a proxy for educational attainment, does not capture quality of education, type of school, nature of school curriculum and location of school, all factors that could have impacted the findings. Third, the cross-sectional design of the data precluded us from making any causal claims in the associations between EA and functional status of individual with ADP. Despite these limitations, the strong community-based representation of our sample, the heterogeneity of participants across multiple sites (Florida and North Carolina) addresses concerns about not having a representative sample, and the use of gold-standard instruments to assess target variables, as well as the public health and clinical value of our findings overshadow these limitations.
In summary, this study shows the importance of using multiple variables such as genetics, education (an established social determinant of health), and AD biomarkers, to have a complete picture of the disease and its cognitive and functional outcomes. Notably, it demonstrates the need for comprehensive approaches to analyze the data, identify patterns associated with a risk of developing AD, and identify the specific combinations of factors most predictive of an individual’s risk of developing AD symptoms. Healthcare providers could use this information to identify individuals at high risk of AD and to develop personalized prevention and treatment plans for those individuals.
AUTHOR CONTRIBUTIONS
Farid Rajabli (Conceptualization; Data curation; Formal analysis; Investigation; Methodology; Software; Validation; Visualization; Writing – original draft; Writing – review & editing); Azizi A. Seixas (Conceptualization; Methodology; Supervision; Writing – original draft; Writing – review & editing); Bilcag Akgun (Data curation; Formal analysis; Software; Writing – review & editing); Larry D. Adams (Data curation; Methodology; Writing – review & editing); Jovita Inciute (Data curation; Writing – review & editing); Kara L. Hamilton (Data curation; Writing – review & editing); Patrice G. Whithead (Data curation; Writing – review & editing); Ioanna Konidari (Data curation; Writing – review & editing); Tianjie Gu (Data curation; Writing – review & editing); Jamie Arvizu (Data curation; Writing – review & editing); Charles G. Golightly (Data curation; Writing – review & editing); Takiyah D. Starks (Data curation; Writing – review & editing); Renee Laux (Data curation; Writing – review & editing); Goldie S. Byrd (Data curation; Funding acquisition; Investigation; Writing – review & editing); Jonathan L. Haines (Methodology; Writing – review & editing); Gary W Beecham (Methodology; Writing – review & editing); Anthony J. Griswold (Data curation; Methodology; Writing – review & editing); Jeffery M. Vance (Conceptualization; Methodology; Writing – review & editing); Michael L. Cuccaro (Conceptualization; Investigation; Methodology; Project administration; Writing – original draft; Writing – review & editing); Margaret A. Pericak-Vance (Conceptualization; Funding acquisition; Investigation; Methodology; Project administration; Resources; Supervision; Writing – original draft; Writing – review & editing).
ACKNOWLEDGMENTS
This investigation was supported by grants AG072547, AG074865, AG052410, AG028786 and AG032984 from the National Institutes on Aging of National Institutes of Health (NIH), HL135452 and HL152453 from the National Heart, Lung, and Blood Institute of NIH, and A2018556F grant from the BrightFocus Foundation.
FUNDING
This research was funded by the NIH, grant number AG072547, AG074865, AG052410, AG028786, AG032984, HL135452, and HL152453, and BrightFocus Foundation, grant number A2018556F.
CONFLICT OF INTEREST
The author has no conflict of interest to report.
DATA AVAILABILITY
Data are available through the National Institute on Aging Genetics of Alzheimer’s Disease Data Storage Site (NIAGADS) Data Sharing Service (DSS): https://dss.niagads.org/datasets/ng00067/.
REFERENCES
[1] | Folstein MF , Bassett SS , Anthony JC , Romanoski AJ , Nestadt GR ((1991) ) Dementia: Case ascertainment in a community survey. J Gerontol 46: , M132–M138. |
[2] | Gurland BJ , Wilder DE , Lantigua R , Stern Y , Chen J , Killeffer EH , Mayeux R ((1999) ) Rates of dementia in three ethnoracial groups. Int J Geriatr Psychiatry 14: , 481–493. |
[3] | Gatz M , Reynolds CA , Fratiglioni L , Johansson B , Mortimer JA , Berg S , Fiske A , Pedersen NL ((2006) ) Role of genes and environments for explaining Alzheimer disease. Arch Gen Psychiatry 63: , 168–174. |
[4] | Migliore L , Coppedè F ((2022) ) Gene-environment interactions in Alzheimer disease: The emerging role of epigenetics. Nat Rev Neurol 18: , 643–660. |
[5] | Litke R , Garcharna LC , Jiwani S , Neugroschl J ((2021) ) Modifiable risk factors in Alzheimer disease and related dementias: A review. Clin Ther 43: , 953–965. |
[6] | Majoka MA , Schimming C ((2021) ) Effect of social determinants of health on cognition and risk of Alzheimer disease and related dementias. Clin Ther 43: , 922–929. |
[7] | Nianogo RA , Rosenwohl-Mack A , Yaffe K , Carrasco A , Hoffmann CM , Barnes DE ((2022) ) Risk factors associated with Alzheimer disease and related dementias by sex and race and ethnicity in the US. JAMA Neurol 79: , 584–591. |
[8] | Bellenguez C , Kücçükali F , Jansen IE , Kleineidam L , Moreno-Grau S , Amin N , Naj AC , Campos-Martin R , Grenier-Boley B , Andrade V , et al., ((2022) ) New insights into the genetic etiology of Alzheimer’s disease and related dementias. Nat Genet 54: , 412–436. |
[9] | Jack CR Jr , Bennett DA , Blennow K , Carrillo MC , Dunn B , Haeberlein SB , Holtzman DM , Jagust W , Jessen F , Karlawish J , Liu E , Molinuevo JL , Montine T , Phelps C , Rankin KP , Rowe CC , Scheltens P , Siemers E , Snyder HM , Sperling R ; Contributors ((2018) ) NIA-AA research framework: Toward a biological definition of Alzheimer’s disease. Alzheimers Dement 14: , 535–562. |
[10] | Hampel H , Cummings J , Blennow K , Gao P , Jack CR Jr , Vergallo A ((2021) ) Developing the ATX(N) classification for use across the Alzheimer disease continuum. Nat Rev Neurol 17: , 580–589. |
[11] | Karikari TK , Pascoal TA , Ashton NJ , Janelidze S , Benedet AL , Rodriguez JL , Chamoun M , Savard M , Kang MS , Therriault J , Schöll M , Massarweh G , Soucy JP , Höglund K , Brinkmalm G , Mattsson N , Palmqvist S , Gauthier S , Stomrud E , Zetterberg H , Hansson O , Rosa-Neto P , Blennow K ((2020) ) Blood phosphorylated tau 181 as a biomarker for Alzheimer’s disease: A diagnostic performance and prediction modelling study using data from four prospective cohorts. Lancet Neurol 19: , 422–433. |
[12] | Tissot C , Benedet LA , Therriault J , Pascoal TA , Lussier FZ , Saha-Chaudhuri P , Chamoun M , Savard M , Mathotaarachchi SS , Bezgin G , Wang YT , Fernandez Arias J , Rodriguez JL , Snellman A , Ashton NJ , Karikari TK , Blennow K , Zetterberg H , De Villers-Sidani E , Huot P , Gauthier S , Rosa-Neto P ; Alzheimer’s Disease Neuroimaging Initiative ((2021) ) Plasma pTau181 predicts cortical brain atrophy in aging and Alzheimer’s disease. Alzheimers Res Ther 13: , 69. |
[13] | Hirota Y , Sakakibara Y , Ibaraki K , Takei K , Iijima KM , Sekiya M ((2022) ) Distinct brain pathologies associated with Alzheimer’s disease biomarker-related phospho-tau 181 and phospho-tau 217 in knock-in mouse models of amyloid-β amyloidosis. Brain Commun 4: , fcac286. |
[14] | Shen XN , Huang YY , Chen SD , Guo Y , Tan L , Dong Q , Yu JT ; Alzheimer’s Disease Neuroimaging Initiative ((2021) ) Plasma phosphorylated-tau181 as a predictive biomarker for Alzheimer’s amyloid, tau and FDG PET status. Transl Psychiatry 11: , 585. |
[15] | Therriault J , Vermeiren M , Servaes S , Tissot C , Ashton NJ , Benedet AL , Karikari TK , Lantero-Rodriguez J , Brum WS , Lussier FZ , Bezgin G , Stevenson J , Rahmouni N , Kunach P , Wang YT , Fernandez-Arias J , Socualaya KQ , Macedo AC , Ferrari-Souza JP , Ferreira PCL , Bellaver B , Leffa DT , Zimmer ER , Vitali P , Soucy JP , Triana-Baltzer G , Kolb HC , Pascoal TA , Saha-Chaudhuri P , Gauthier S , Zetterberg H , Blennow K , Rosa-Neto P ((2023) ) Association of phosphorylated tau biomarkers with amyloid positron emission tomography vs tau positron emission tomography. JAMA Neurol 80: , 188–199. |
[16] | Arenaza-Urquijo EM , Vemuri P ((2018) ) Resistance vs resilience to Alzheimer disease: Clarifying terminology for preclinical studies. Neurology 90: , 695–703. |
[17] | Stern Y , Gurland B , Tatemichi TK , Tang MX , Wilder D , Mayeux R ((1994) ) Influence of education and occupation on the incidence of Alzheimer’s disease. JAMA 271: , 1004–1010. |
[18] | Stern Y , Barulli D ((2019) ) Cognitive reserve. Handb Clin Neurol 167: , 181–190. |
[19] | Stern Y ((2012) ) Cognitive reserve in ageing and Alzheimer’s disease. Lancet Neurol 11: , 1006–1012. |
[20] | Stern Y , Barnes CA , Grady C , Jones RN , Raz N ((2019) ) Brain reserve, cognitive reserve, compensation, and maintenance: Operationalization, validity, and mechanisms of cognitive resilience. Neurobiol Aging 83: , 124–129. |
[21] | Collaboratory on Research Definitions for Reserve and Resilience in Cognitive Aging and Dementia (2022) “Framework for Terms Used in the Research of Reserve and Resilience,” https://reserveandresilience.com/framework/ |
[22] | Avila JF , Rentería MA , Jones RN , Vonk JMJ , Turney I , Sol K , Seblova D , Arias F , Hill-Jarrett T , Levy SA , Meyer O , Racine AM , Tom SE , Melrose RJ , Deters K , Medina LD , Carrión CI , Díaz-Santos M , Byrd DR , Chesebro A , Colon J , Igwe KC , Maas B , Brickman AM , Schupf N , Mayeux R , Manly JJ ((2021) ) Educationdifferentially contributes to cognitive reserve across racial/ethnicgroups. Alzheimers Dement 17: , 70–80. |
[23] | Lövdén M , Fratiglioni L , Glymour MM , Lindenberger U , Tucker-Drob EM ((2020) ) Education and cognitive functioning across the life span. Psychol Sci Public Interest 21: , 6–41. |
[24] | Seblova D , Berggren R , Lövdén M ((2020) ) Education and age-related decline in cognitive performance: Systematic review and meta-analysis of longitudinal cohort studies. Ageing Res Rev 58: , 101005. |
[25] | Stern Y , Arenaza-Urquijo EM , Bartrés-Faz D , Belleville S , Cantilon M , Chetelat G , Ewers M , Franzmeier N , Kempermann G , Kremen WS , Okonkwo O , Scarmeas N , Soldan A , Udeh-Momoh C , Valenzuela M , Vemuri P , Vuoksimaa E ((2020) ) The reserve, resilience and protective factors PIA empirical definitions and conceptual frameworks workgroup. Whitepaper: Defining and investigating cognitive reserve, brain reserve, and brain maintenance. Alzheimers Dement 16: , 1305–1311. |
[26] | Corder EH , Saunders AM , Strittmatter WJ , Schmechel DE , Gaskell PC , Small GW , Roses AD , Haines JL , Pericak-Vance MA ((1993) ) Gene dose of apolipoprotein E type 4 allele and the risk of Alzheimer’s disease in late onset families. Science 261: , 921–923. |
[27] | Vonk JMJ , Arce Rentería M , Medina VM , Pericak-Vance MA , Byrd GS , Haines J , Brickman AM , Manly JJ ((2019) ) Education moderates therelation between APOE ɛ4 and memory in nondementedNon-Hispanic Black older adults. J Alzheimers Dis 72: , 495–506. |
[28] | Dekhtyar S , Marseglia A , Xu W , Darin-Mattsson A , Wang HX , Fratiglioni L ((2019) ) Genetic risk of dementia mitigated by cognitive reserve: A cohort study. Ann Neurol 86: , 68–78. |
[29] | Seeman TE , Huang MH , Bretsky P , Crimmins E , Launer L , Guralnik JM ((2005) ) Education and APOE-e4 in longitudinal cognitive decline: MacArthur Studies of Successful Aging. J Gerontol B Psychol Sci Soc Sci 60: , P74–P83. |
[30] | López ME , Turrero A , Delgado ML , Rodríguez-Rojo IC , Arrazola J , Barabash A , Maestú F , Fernández A ((2017) ) APOEɛ4 genotype and cognitive reserve effects on thecognitive functioning of healthy elders. Dement Geriatr CognDisord 44: , 328–342. |
[31] | Blazer DG , Fillenbaum G , Burchett B ((2001) ) The APOE-E4 allele and the risk of functional decline in a community sample of African American and white older adults. J Gerontol A Biol Sci Med Sci 56: , M785–M789. |
[32] | Lan TY , Chang WC , Lan TH , Hurng BS ((2009) ) Apolipoprotein E genotype and risk of developing physical limitations in elderly people. J Am Geriatr Soc 57: , 1308–1309. |
[33] | Lindbergh CA , Puente AN , MacKillop J , Faraco C , Brown C , Terry D , Mewborn C , Miller LS ((2015) ) ApoE-ɛ4 allele status is associated with functional limitations in community-dwelling older adults. Alzheimers Dement 11: (7 Suppl), P659. |
[34] | Price AL , Patterson NJ , Plenge RM , Weinblatt ME , Shadick NA , Reich D ((2006) ) Principal components analysis corrects for stratification in genome-wide association studies. Nat Genet 38: , 904–909. |
[35] | Genomes Project Consortium; Auton A , Brooks LD , Durbin RM , Garrison EP , Kang HM , Korbel JO , Marchini JL , McCarthy S , McVean GA , Abecasis GR ((2015) ) A global reference for human genetic variation. Nature 526: , 68–74. |
[36] | Saunders AM , Strittmatter WJ , Schmechel D , George-Hyslop PH , Pericak-Vance MA , Joo SH , Rosi BL , Gusella JF , Crapper-MacLachlan DR , Alberts MJ ((1993) ) Association of apolipoprotein E allele epsilon 4 with late-onset familial and sporadic Alzheimer’s disease. Neurology 43: , 1467–1472. |
[37] | Wilson DH , Rissin DM , Kan CW , Fournier DR , Piech T , Campbell TG , Meyer RE , Fishburn MW , Cabrera C , Patel PP , Frew E , Chen Y , Chang L , Ferrell EP , von Einem V , McGuigan W , Reinhardt M , Sayer H , Vielsack C , Duffy DC ((2016) ) The Simoa HD-1 analyzer: A novel fully automated digital immunoassay analyzer with single-molecule sensitivity and multiplexing. J Lab Autom 21: , 533–547. |
[38] | Hughes CP , Berg L , Danziger WL , Coben LA , Martin RL ((1982) ) A new clinical scale for the staging of dementia. Br J Psychiatry 140: , 566–572. |
[39] | Julayanont P , DeToledo JC ((2022) ) Validity of the Clinical Dementia Rating Scale Sum Of Boxes in staging and detection of cognitive impairment in Mexican Americans. J Geriatr Psychiatry Neurol 35: , 128–134. |
[40] | O’Bryant SE , Waring SC , Cullum CM , Hall J , Lacritz L , Massman PJ , Lupo PJ , Reisch JS , Doody R ; Texas Alzheimer’s Research Consortium ((2008) ) Staging dementia using Clinical Dementia Rating Scale Sum Of Boxes scores: A Texas Alzheimer’s research consortium study. Arch Neurol 65: , 1091–1095. |
[41] | Grober E , Qi Q , Kuo L , Hassenstab J , Perrin RJ , Lipton RB ((2021) ) Stages of objective memory impairment predict Alzheimer’s disease neuropathology: Comparison with the clinical dementia rating scale-sum of boxes. J Alzheimers Dis 80: , 185–195. |
[42] | Sudo FK , Alves GS , Moreira DM , Laks J , Engelhardt E ((2016) ) Subcortical vascular cognitive impairment staged through CDR’s functional subsum (CDR-func): Preliminary results from an outpatient sample. eNeurologicalSci 10: , 7–10. |
[43] | Pfeffer RI , Kurosaki TT , Harrah CH , Chance JM , Filos S ((1982) ) Measurement of functional activities in older adults in the community. J Gerontol 37: , 323–329. |