The predictive accuracy of CT radiomics combined with machine learning in predicting the invasiveness of small nodular lung adenocarcinoma
Highlight box
Key findings
• The machine learning algorithm was used to construct models based on CT radiomic characteristics to predict the invasiveness of LUAD, which has good predictive accuracy.
What is known and what is new?
• Radiomics converts traditional images into data information that can be mined and analyzed to extract characteristic data that are difficult for human eyes to observe and distinguish.
• The machine learning algorithm was used to construct models based on radiomic characteristics to predict the invasiveness of LUAD with a good prediction accuracy.
What is the implication, and what should change now?
• Machine learning algorithms were used to construct models to predict the invasiveness of small nodular LUAD based on radiomics features, which it could provide more evidence for doctors to make diagnoses. Therefore, thoracic surgeons should make more use of radiomics combined with machine learning in the diagnosis and treatment of patients with small pulmonary nodules.
Introduction
Lung cancer is the leading cause of cancer-related death worldwide, and its morbidity and mortality are gradually increasing (1,2). With the wide application of low-dose multislice spiral computed tomography (CT) and the increase of the number of physical examinations, the detection rate of pulmonary nodules is increasing (3). About 1% of pulmonary nodules are malignant tumors, most of which are lung adenocarcinoma (LUAD) (4). The management of LUAD with different invasiveness degrees is different: for pre-invasive lung nodules with slow growth rate, only regular follow-up is required, whereas invasive lung nodules require elective or immediate surgical treatment (5,6). Therefore, it is necessary to accurately diagnose the invasiveness of small nodular LUAD.
At present, the main diagnostic technique for the invasiveness of small nodular LUAD is the assessment of chest CT by thoracic surgeons (7). Surgeons judge whether small pulmonary nodules are invasive mainly by observing whether the overall morphology of CT lesions is regular, whether the edges of the lesions are lobulated, whether the density of the lesions has solid components, whether there are vascular traversing and vacuoles inside the lesions, and whether there are pleural indentations in the lesions and surrounding lung tissue (8). However, it is often difficult to make an accurate diagnosis of the invasiveness of LUAD because small pulmonary nodules with different invasiveness often have some similar imaging appearances, and the diagnosis of the same lesion is susceptible to the subjective differences between thoracic surgeons (9). The concept of radiomics was first proposed by Dutch scholar Philippe Lam Bin in 2012. Radiomics transforms traditional images into data information that can be mined and analyzed, so as to extract feature data that are difficult for human eyes to observe and distinguish (10). It is qualitative analysis for thoracic surgeons to determine whether the lesion is invasive by morphological features of the lesion, while radiomics analyzes more internal features of CT images from different levels. These characteristic data are used to perform statistical analyses to construct predictive models (11). Therefore, six machine learning algorithms were used to construct the predictive accuracy of the invasive prediction of small pulmonary nodules based on radiological characteristics. We present the following article in accordance with the TRIPOD reporting checklist (available at https://tlcr.amegroups.com/article/view/10.21037/tlcr-23-82/rc).
Methods
Patients
A total of 469 patients with small pulmonary nodule preinvasive lesions and invasive LUAD confirmed by pathology after video-assisted thoracoscopic surgery (VATS) were retrospectively analyzed in the Department of Thoracic Surgery of the First People’s Hospital of Yunnan Province from February 2020 to February 2022. Among them, 53 patients were excluded due to unclear preoperative chest CT images or incomplete patient information. In this study, the training group and validation group were randomly assigned with a ratio of 3:7 with 555 random seeds. Therefore, 416 patients were finally included in the study. Patients included in the study were divided into a training group (n=304) and a validation group (n=112). We collected pathological results from all patients with LUAD. Atypical adenomatous hyperplasia (AAH) and adenocarcinoma in situ (AIS) were classified as preinvasive lesions, while minimally invasive adenocarcinoma (MIA) and invasive adenocarcinoma (IAC) were classified as invasive lesions. In addition, the general clinical data of the two groups were retrospectively analyzed. The preoperative enhanced CT images of pulmonary window and postoperative pathological data of the 2 groups were input into the RadCloud platform (https://mics.huiyihuiying.com) for radiomics correlation analysis.
The operation procedure of CT radiomics in this study was that the CT image data were acquired and then the region of interest (ROI) was segmented. Then, radiomics features were acquired and screened. Finally, the prediction model was developed and validated using 6 machine learning models (Figure 1).
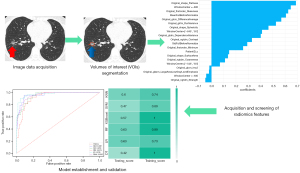
Ethical approval for the study was granted by the Ethics Committee of The First People’s Hospital of Yunnan Province (No. KHLL2022-KY012). In addition, written informed consent was provided by all patients or their legal guardians who participated in the study. This study was conducted in accordance with the Declaration of Helsinki (as revised in 2013).
Inclusion criteria
- The patient had pathologically confirmed preinvasive lesions and invasive adenocarcinoma (IA) after VATS surgery;
- The longest 2-dimensional (2D) diameter of the pulmonary nodules was ≤30 mm;
- The patient had no history of other tumors;
- The interval between chest CT examination and surgical treatment was less than 20 days.
Exclusion criteria
- The clinical and postoperative pathological data of the patients were incomplete;
- The patient’s chest CT was not clear;
- The patient had a history of other tumors or metastases;
- The patient received preoperative radiotherapy and chemotherapy;
- Multiple pulmonary nodules in the lung.
Image data acquisition
All patients underwent enhanced CT before surgery to assess information about pulmonary nodules. Patients were scanned with chest CT using 128-row or 64-row SOMATOM Force CT (Siemens Healthineers, Erlangen, Germany). The 128-row SOMATOM Force CT scanning parameters were set as follows: tube voltage 120 kV, adaptive tube current, layer thickness 1.25 mm, pitch 1.2, rotation time 0.6 seconds, field of view (FOV) of 400 mm × 400 mm, and reconstructed layer thickness 1.25 mm. Advanced Modeled Iterative Reconstruction (ADMIRE) iterative technology (Siemens) was used for image reconstruction. The parameters of the 64-row SOMATOM Force CT scanning were set as follows: tube voltage 100 kV, adaptive tube current, layer thickness 1.25 mm, pitch 0.984:1, rotation time 0.6 seconds, FOV 500 mm × 500 mm, reconstructed layer thickness 1.25 mm, and ADMIRE iterative technology was used for image reconstruction.
Approximately 30 minutes before the patient underwent CT examination, the radiologist used a high-pressure syringe to inject 50–75 mL of ioversol (iodine content: 350 mg/mL, Jiangsu Hengrui Pharmaceutical Co., Ltd., Lianyungang, China) into the anterior cubital vein at a flow rate of 2.5–3.0 mL/s. Patients were trained to inhale and exhale before scanning. Patients were placed in a supine position, arms raised, head first, and scanned after breath holding at the end of inspiration. The scan range included the apex of the lung to 3 cm below the diaphragm. In this study, the latest preoperative chest CT pulmonary window image before the operation time was used for subsequent analysis.
Volumes of interest segmentation
Preoperative enhanced CT images of all patients were first exported in digital imaging and communications in medicine (DICOM) format through picture archiving and communication system (PACS) and then imported into the RadCloud platform. The RadCloud platforms were used by a young thoracic surgeon to segment the pulmonary nodules layer by layer, and then the segmentation results were reviewed, modified, and confirmed by a senior professional radiologist with experience in pulmonary class diagnosis. Finally, the segmented content of all levels of each nodule was fused into its volume of interests (VOIs) for subsequent radiomics feature analysis.
Feature extraction and screening
After lesion segmentation, the internal software of the platform automatically extracted radiomics features from the original image of each VOIs. Radiomics feature extraction is based on VOIs, and any information outside VOIs has nothing to do with features. In this study, we used the RadCloud platform to extract radiomics features from 416 patients in 2 stages, first filtering processing and then feature calculation. Some features with large variable deletion rates or no correlation were eliminated. The platform filtering processing methods are Laplacian of Gaussian (LoG), wavelet, square, square root, logarithm, and exponential. The feature calculation methods of this platform are first order statistic, shape-based and texture, the texture features include gray level cooccurence matrix (GLCM), gray level run length matrix (GLRLM), and gray level size zone matrix (GLSZM). After a large number of CT image features are extracted, the continuous dimension reduction screening method of this platform is used to screen out highly reproducible, informative, and non-redundant features, and the best radiomics features are selected to construct the prediction model. The platform dimension reduction methods are low variance, select best, least absolute shrinkage and selection operator (LASSO), principal component analysis (PCA), covariance, and clustering.
Model establishment and validation
The machine learning algorithm of the platform was used to analyze the final radiomics features and establish the radiomics model. Different algorithms depend on the purpose of the study and the category of results. The main machine learning algorithms in this platform are k-nearest neighbor (KNN), support vector machine (SVM), random forests (RF), logistic regression (LR), decision tree (DT), and eXtreme Gradient Boosting (XGBoost). Modeling methods may also affect the prediction results, so this study compared the performance of various modeling methods to select the best method.
Statistical analysis
General clinical data such as count and measurement data were analyzed by the statistical software SPSS 26.0 (IBM Corp., Armonk, NY, USA). Measurement data were expressed as mean ± standard deviation (SD), and enumeration data were expressed as frequency. If the distribution was normal, the t-test was used, otherwise, the homogeneity of variance between groups was tested. If the variance was homogenous, one-way analysis of variance (ANOVA) was used, if the variance was not homogenous, the nonparametric rank sum test (Mann-Whitney U) was used. The RadCloud platform was used for the management of patients’ CT image data, postoperative pathological data and subsequent radiomics analysis. Receiver operating characteristic (ROC) curve and area under curve (AUC) were used to evaluate the prediction accuracy of the training and validation groups. The 4 indexes in this study were P [precision = true positive/(true positive + false positive)] and R [recall = true positive/(true positive + false negative)], F1-score [F1-score = P × R × 2/(P + R)], and support (total number in the test set) to evaluate the performance of the classifier. The prediction performance of AUC <0.7 was general, 0.7≤ AUC <0.9 indicated better performance, and AUC ≥0.9 indicated the best performance. In statistical analysis, the maximum threshold of sensitivity and specificity of the prediction model was 1. P<0.05 was considered statistically significant.
Results
A total of 416 patients with 416 pulmonary nodules were finally enrolled in this study. According to random classification, patients were divided into a training group (n=304) and a validation group (n=112) in a 7:3 ratio. There was no significant difference between the two groups in age, smoking history, family history of lung cancer, body mass index, pulmonary nodule type or 2D maximum diameter of lung nodule. There were 112 cases of preinvasive lesions, including 78 in the training group and 34 in the validation group. In addition, there were 304 cases of invasive lesions, including 226 in the training group and 78 in the validation group (Table 1).
Table 1
Variables | Cases | Training group (n=304) | Validation group (n=112) | P value |
---|---|---|---|---|
Age (years) | 416 (100) | 51.09±14.12 | 52.08±7.65 | 0.849 |
Sex (male/female) | 416 (100) | 101/203 (33.22%/66.78%) | 32/80 (28.57%/71.43%) | 0.367 |
Smoking history (yes/no) | 416 (100) | 73/231 (24.01%/75.99%) | 30/82 (26.79%/73.21%) | 0.561 |
Family history of lung cancer (yes/no) | 416 (100) | 26/278 (8.55%/91.45%) | 13/99 (11.61%/88.39%) | 0.343 |
BMI (kg/m2) | 416 (100) | 22.82±3.51 | 22.90±3.95 | 0.705 |
2D maximum diameter of lung nodules (mm) | 416 (100) | 14.60±5.75 | 14.31±5.23 | 0.463 |
Location of pulmonary nodules, n (%) | ||||
Left upper lobe | 100 (24.04) | 77 (18.51) | 23 (18.51) | |
Left lower lobe | 59 (14.18) | 38 (9.13) | 21 (5.05) | |
Right upper lobe | 119 (28.61) | 87 (20.91) | 32 (7.70) | |
Right middle lobe | 38 (9.13) | 35 (8.41) | 3 (0.72) | |
Right lower lobe | 100 (24.04) | 67 (16.11) | 33 (7.93) | |
Pulmonary nodule size, n (%) | 0.208 | |||
2D Maximum diameter of pulmonary nodules ≤10 mm | 129 (31.01) | 89 (21.39) | 40 (9.62) | |
2D The maximum diameter of pulmonary nodules was >10 and ≤30 mm | 287 (68.99) | 215 (51.68) | 72 (17.31) | |
Pulmonary nodule type, n (%) | 0.404 | |||
Solid pulmonary nodules | 171 (41.11) | 116 (27.88) | 55 (13.22) | |
Partial solid pulmonary nodules | 134 (32.21) | 96 (23.08) | 38 (9.13) | |
Pure ground glass pulmonary nodules | 111 (26.68) | 92 (22.12) | 19 (4.57) | |
Surgical method, n (%) | 0.177 | |||
Wedge resection | 103 (24.76) | 70 (16.83) | 33 (7.93) | |
Segmental resection | 128 (30.77) | 97 (23.32) | 31 (7.45) | |
Lobectomy | 185 (44.47) | 137 (32.93) | 48 (11.54) | |
Pathology, n (%) | 0.338 | |||
AAH | 22 (5.29) | 14 (3.37) | 8 (1.92) | |
AIS | 90 (21.63) | 64 (15.38) | 26 (6.25) | |
MIA | 73 (17.55) | 63 (15.14) | 10 (2.40) | |
IA | 231 (55.53) | 163 (39.18) | 68 (16.35) |
Continuous data are presented as mean ± SD and categoric variables as number (frequency and/or %). P<0.05 is considered significant. BMI, body mass index; 2D, 2-dimensional; AAH, atypical adenomatous hyperplasia; AIS, adenocarcinoma in situ; MIA, minimally invasive adenocarcinoma; IA, invasive adenocarcinoma; SD, standard deviation.
A total of 1,409 characteristics were extracted from each VOI using the RadCloud platform, and the extracted features included 3 categories: first-order statistical features, shape features, and texture features. In order to eliminate redundant features and screen and retain some features most relevant to the results, we first use variance threshold method for dimensionality reduction. In this method, the variance of each eigenvalue is calculated. According to the threshold, the eigenvalue with a variance is greater than the threshold is selected for the next step analysis. The threshold used in this research was 0.8, and 429 features were selected from 1,409 features (Figure 2). Then, we used univariate feature selection method to measure the relationship between characteristics and classification results by ANOVA. Characteristics with P<0.05 were selected, the other features were removed, and 33 features were selected from 429 features (Figure 3). Finally, the LASSO algorithm was used to select 4 features from 33 features that were most valuable for predicting the invasiveness of small nodular LUAD. The 4 features were as follows: wavelet-LHL-glrlm-LongRunHighGrayLevelEmphasis, wavelet-LLL-glrlm-LongRunLowGrayLevelEmphasis, wavelet-LLL-firstorder-Kurtosis, wavelet-LLH-firstorder-Kurtosis (Figure 4).
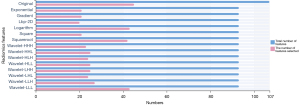
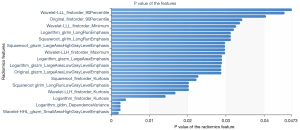
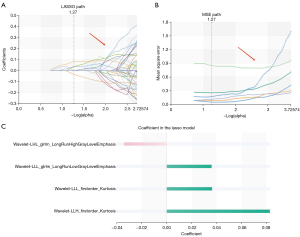
In this study, we used 6 classifiers, each of which was used to construct a radiomics-based model: KNN, SVM, RF, LR, DT, and XGBoost. The validation groups were used to evaluate the accuracy of the model. The following parameters of the 6 classifiers were applied. For KNN: n_neighbors [5], weights (uniform). For SVM: kernel (rbf), C [1], gamma (auto), class_weight (balanced), decision_ function_shape (ovr), random_state (−). For RF: n_estimators [10], class_weight (None). For LR: penalty (L1), C [5], solver (lib linear), class_weight (balanced), multi_class (ovr), random_state (−). For DT: splitter (random), criterion (entropy). For XGBoost: Eta [0.3], max_depth [6]. The AUC results of the training group and validation group using the 6 classifiers are shown in Figure 5. The 6 classifiers were used to analyze the characteristics of radiological AUC, and the 95% confidence interval (CI), sensitivity, and specificity of the training and validation groups are shown in Table 2. The AUC values of the 6 models in the training group were all qual or more than 0.914, the 95% CI was 0.857–1.00, sensitivity was qual or more than 0.87, and specificity was qual or more than 0.85. In the validation group, the AUC values of the 6 models were all qual or more than 0.732, the 95% CI was 0.651–1.00, sensitivity was qual or more than 0.70, and specificity was qual or more than 0.77. The evaluation results of the diagnostic effects of the 6 classifiers using 4 different indicators in the validation group are shown in Table 2. The precision of the 6 models was qual or more than 0.65, the recall was qual or more than 0.70, the F1-score was qual or more than 0.67, and the support was 10. In addition, calibration curves and clinical decision curves were used to evaluate the performance of the predictive model as shown in Figure 6.
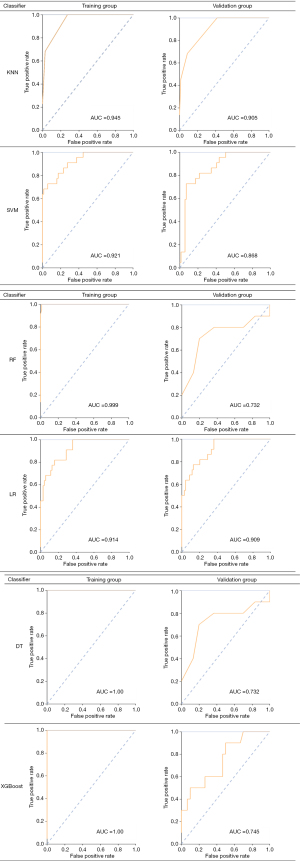
Table 2
Classifiers | Category | AUC | 95% CI | Sensitivity | Specificity | Precision | Recall | F1-score | Support |
---|---|---|---|---|---|---|---|---|---|
KNN | Training group | 0.945 | 0.890–1.000 | 1 | 0.895 | ||||
Validation group | 0.905 | 0.887–1.000 | 0.997 | 0.873 | 0.89 | 0.97 | 0.85 | 10 | |
SVM | Training group | 0.921 | 0.857–0.992 | 0.89 | 0.91 | ||||
Validation group | 0.868 | 0.763–0.973 | 0.77 | 0.8 | 0.73 | 0.77 | 0.93 | 10 | |
RF | Training group | 0.999 | 0.932–1.000 | 1 | 0.931 | ||||
Validation group | 0.732 | 0.753–0.877 | 0.76 | 0.9 | 0.65 | 0.76 | 0.84 | 10 | |
LR | Training group | 0.914 | 0.910–0.978 | 0.87 | 0.85 | ||||
Validation group | 0.909 | 0.896–0.939 | 0.79 | 0.87 | 0.85 | 0.79 | 0.67 | 10 | |
DT | Training group | 1 | 1.000–1.000 | 1 | 1 | ||||
Validation group | 0.732 | 0.679–0.887 | 0.8 | 0.77 | 0.93 | 0.8 | 0.91 | 10 | |
XGBoost | Training group | 1 | 0.956–1.000 | 0.95 | 1 | ||||
Validation group | 0.745 | 0.651–0.873 | 0.7 | 0.79 | 0.97 | 0.7 | 0.84 | 10 |
Precision = true positive/(true positive + false positive); recall = true positive/(true positive + false negative); F1-score = P × R × 2/(P + R); support (total number in the validation group) to evaluate the performance of the classifier. ROC, receiver operating characteristic; KNN, k-nearest neighbor; SVM, support vector machine; RF, random forests; LR, logistic regression; DT, decision tree; XGBoost, extreme gradient boosting; AUC, area under curve; CI, confidence interval.
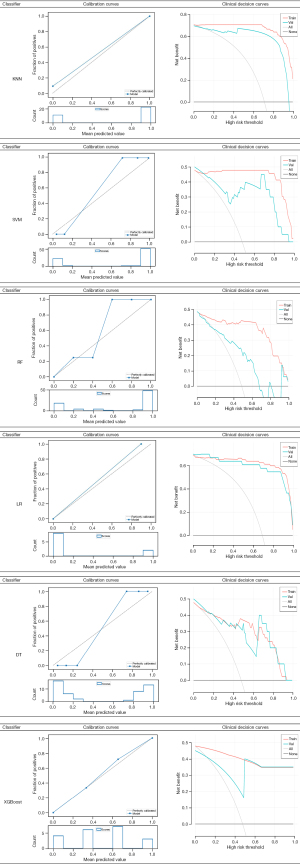
Discussion
Lung cancer has a high incidence and mortality, and LUAD accounts for the largest proportion among lung cancers (2). In 2021, WHO classification of thoracic tumor histology defined AAH and AIS as preinvasive lesions with slow growth rate, which generally only requires regular follow-up observation. MIA and IAC are invasive lesions that require immediate or selective surgical treatment (12). Therefore, early identification of the invasiveness of lung nodular adenocarcinoma can not only enable doctors to make appropriate treatment for patients, but also improve the survival rate of patients.
Radiomics, as a high-throughput quantitative image feature mining technology, has emerged in recent years (13). It uses complex image analysis technology to rapidly analyze and validate medical imaging data, and automatically or semi-automatically extract a large amount of quantifiable information or image features from the ROI of images and apply them to clinical practice, so as to improve the accuracy of diagnosis, prognosis, and prediction of tumor lesions (14,15). The basis of radiomics feature extraction is that ROI is accurately segmented, and correct ROI ensures the reliability of radiomics research (16,17). In this study, small pulmonary nodules in the lung window of patients with preoperative CT enhancement were the ROI of this study. The fitting and delineation method of the RadCloud platform was used to segment small pulmonary nodules from the lung window, which is more accurate than rectangular, oval, or free shape segmentation. In addition, the segmentation by the young doctor was then modified and confirmed by the senior radiologist, which further ensured the accuracy of the ROI.
A total of 1,409 radiomics characteristics were extracted from the RadCloud platform in this study. After 3 consecutive dimensionality reduction processes, redundant features with low correlation were removed and more discriminative features were retained. The 4 most discriminating radiological features were finally extracted to predict the invasiveness of small nodular LUAD. The 4 features included wavelet-LHL-glrlm-LongRunHighGrayLevelEmphasis, wavelet-LLL-glrlm-LongRunLowGrayLevelEmphasis, wavelet-LLL-firstorder-Kurtosis, and wavelet-LLH-firstorder-Kurtosis. Clearly, all radiological features are first-order features and texture features. These features extracted in this study also suggest that the intensity difference between preinvasive lesions and invasive lesions is greater than the morphological difference in small nodular LUAD. These radiomic features are similar to previous research results (18), it may be related to the comparability and overlap of morphological characteristics between preinvasive and invasive lesions in small lung nodular adenocarcinoma, which is why they are difficult to distinguish in traditional visual assessment (19).
The first-order feature describes the pixel intensity and its distribution in small lung nodules by basic first-order statistics (20). The first-order feature we extracted in this study is kurtosis, which is a measure of the “peak” of the median ROI distribution of the image. The high kurtosis of our results means that the mass of the distribution is concentrated in the tail rather than the mean (21). Texture features are the underlying features of images, which can comprehensively reflect the gray statistics, spatial distribution, and structural information of images (22). Texture analysis can be used to detect subtle differences that cannot be detected by the naked eye, and it is more objective to identify the invasiveness of adenocarcinoma with small lung nodules (23). Among them, GLRLM describes the texture by recording the occurrence of consecutive multiple identical pixel values in the 1-dimensional direction of the image. In coarse texture, the gray level changes smoothly, so the gray run length is longer, however, in fine texture, gray value mutation is more, resulting in shorter run (24). The long run high gray level emphasis (LRHGLRE) and long run low gray level emphasis (LRLGLRE) texture features were ultimately selected for this study. The LRHGLRE represents the joint distribution of long-distance running lengths with higher gray values, whereas LRLGLRE represents the joint distribution of long-distance running lengths with lower gray values (25).
The heterogeneity of lung cancer is caused by the tissue structure changes caused by the uneven distribution of cell density, hemorrhage, necrosis, myxoid degeneration (26). According to histopathology, most small nodular LUADs show histological heterogeneity, and a lesion may contain 1 major pathological component and several minor pathological components (27). Compared with preinvasive lesions of LUAD, invasive lesions begin to present invasive components such as alveoli, papillae, micropapillae, or solid components (28). Therefore, intratumoral heterogeneity is more obvious in invasive lesions of small lung nodules, and tumors with greater heterogeneity may be more invasive (29). However, traditional biopsy and even postoperative pathological examination have limited value in reflecting tumor heterogeneity, because pathological examination usually focuses on parts of the tumor and cannot fully reflect the tumor as a whole (30). Radiomics can capture the spatial heterogeneity and biological invasiveness of tumor internal structure by extracting texture features using high-throughput data characterization algorithms (31). The texture feature extracted in this study was GLRLM, which indicates that the coarser the texture in the gray value area of the image, the higher degree of cancer cell accumulation, and the stronger the invasiveness of small nodule LUAD. It is worth mentioning that the extraction of radiomics texture features in this study was based on preoperative enhanced CT images of patients. Compared with ordinary CT plain images, enhanced images can more accurately reflect more valuable information such as the edge of the lesion, tumor blood supply, and the interior of the lesion, and the tumor heterogeneity is more obvious (32).
In recent years, there have also been studies on the use of radiomics to predict the invasiveness of LUAD (33-37). The 15 radiomics features selected in the study of Zhao et al. combined with the average value of CT, were used to construct a prediction model for sub-centimeter LUAD nodule invasion. The AUC of the model in the training group and validation group were 0.716 and 0.7070, respectively (22). Xue et al. also conducted a similar study, and the AUC of the established radiomics nomogram for predicting the invasiveness of ground glass nodules was 0.79 (95% CI: 0.71–0.88) (38). Luo et al. also established a radiomics model that could predict the invasiveness of ground glass nodules with an AUC value of 0.90 (39). Compared with the above studies and other similar studies, the small lung nodules included in this study include all types of solid nodules, subsolid nodules, and ground glass nodules. The types and clinical characteristics of small lung nodules included in this study were more comprehensive, which can not only explore more radiomic features, but also reflect the actual clinical situation. In addition, the CT images of patients were filtered and preprocessed by the RadCloud platform before extracting radiomics features in this study. This not only reduced the poor reproducibility of radiomics features caused by different scan parameters or scanning CT machines, but also made the research results more stable and reliable.
The biggest advantage of radiomics is that these high-dimensional quantitative features can be combined with machine learning to build predictive models (40). It can greatly improve the ability of clinicians to predict the invasiveness of small lung nodular LUAD based on evidence, and provide a new method and possibility for early diagnosis of small lung nodular LUAD before surgery (41). To date, there have been many studies conducted on radiomics models based on CT images in identifying the invasiveness of LUAD, but there have been few studies on building a variety of machine learning models based on radiomics characteristics to predict the invasiveness of small lung nodular LUAD (11,42). In this study, 6 machine learning classifiers (KNN, SVM, RF, LR, DT, XGBoost) were used to establish prediction models for the invasiveness of small nodular LUAD according to the final 4 optimal features extracted. In the training group, the AUC values of the 6 models were all more than 0.914, the 95% CI was 0.857–1.00, the sensitivity was more than 0.87, and the specificity was more than 0.85. In the validation more, the AUC values of the 6 models were all more than 0.732, the 95% CI was 0.651–1.00, the sensitivity was more than 0.7, and the specificity was more than 0.77. The specificity, sensitivity, and AUC of the 6 models in the training group and the validation group all showed good predictive ability, which confirmed the feasibility of the machine learning model based on radiomics features to predict the invasiveness of small nodular LUAD (43,44). In addition, She et al. proposed that machine learning models constructed based on radiomics features may help in the development of predictive model stability (45). Similarly, our results show that the performance of using 6 machine learning algorithms to construct prediction models based on 4 radiomics features was good in the validation group. This also indicates that the 4 extracted radiomics features are highly reliable and can be utilized even if different machine learning algorithms are used for model construction.
In order to further verify the accuracy of machine learning model prediction, this study analyzed the characteristics of 6 machine learning classifiers through 4 clinical indicators (precision, recall, f1 score, and support). In the validation group, the precision of the 6 models was the highest 0.97, the recall was higher than 0.7, the F1-score was the highest 0.93, and the support was 10. These results further demonstrate the accuracy of our study in predicting the invasiveness of small nodular LUAD. In addition, the performance of these 6 prediction models is not the same. In the validation group, the highest precision of the XGBoost and DT algorithm was 0.97 and 0.93, respectively, which are higher than the other 4 machine learning algorithms. Some studies involving a number of different modeling methods have also found that the precision of the constructed models is not the same under different machine learning algorithms (46-48). Therefore, we should combine more radiomics features and try more machine learning algorithms to build models to obtain the best performance model for predicting the invasiveness of small nodal LUAD.
Study limitations
This study had some limitations. First, this study was a single-center retrospective study, which may have led to selection bias. Second, the CT scanning equipment used on different patients was different. It had a certain effect on the homogenization of the obtained image data. Third, the sample size of the study was small, and the number of adenocarcinomas with preinvasive lesions and invasive lesions was not balanced. Finally, segmentation of small lung nodules was performed manually, which may be subject to the subjective influence of different doctors.
Conclusions
In this study, radiomics combined with machine learning was used to investigate the efficacy of chest enhanced CT in predicting the invasiveness of small nodular LUAD, which has great predictive value. This method is helpful to improve the early diagnosis rate of small pulmonary nodules and prevent misdiagnosis and mistreatment. At the same time, it provides a basis for thoracic surgeons to provide more personalized diagnosis and treatment plan for each small lung nodular LUAD patient, which is more in line with the patient’s own condition.
Acknowledgments
Funding: This study was supported by the Science and Technology Program of Kunming City (No. 2020-1-H-003), the Key Project of Basic Research Program of Yunnan Province (No. 202201AS070009), and the Opening Project of Chest Disease Clinical Medical Center of Yunnan First People’s Hospital in 2021 (No. 2021LCZXXF-XB03).
Footnote
Reporting Checklist: The authors have completed the TRIPOD reporting checklist. Available at https://tlcr.amegroups.com/article/view/10.21037/tlcr-23-82/rc
Data Sharing Statement: Available at https://tlcr.amegroups.com/article/view/10.21037/tlcr-23-82/dss
Peer Review File: Available at https://tlcr.amegroups.com/article/view/10.21037/tlcr-23-82/prf
Conflicts of Interest: All authors have completed the ICMJE uniform disclosure form (available at https://tlcr.amegroups.com/article/view/10.21037/tlcr-23-82/coif). The authors have no conflicts of interest to declare.
Ethical Statement: The authors are accountable for all aspects of the work in ensuring that questions related to the accuracy or integrity of any part of the work are appropriately investigated and resolved. Ethical approval for the study was granted by the Ethics Committee of The First People’s Hospital of Yunnan Province (No. KHLL2022-KY012). Written informed consent was provided by all patients or their legal guardians who participated in the study. This study was conducted in accordance with the Declaration of Helsinki (as revised in 2013).
Open Access Statement: This is an Open Access article distributed in accordance with the Creative Commons Attribution-NonCommercial-NoDerivs 4.0 International License (CC BY-NC-ND 4.0), which permits the non-commercial replication and distribution of the article with the strict proviso that no changes or edits are made and the original work is properly cited (including links to both the formal publication through the relevant DOI and the license). See: https://creativecommons.org/licenses/by-nc-nd/4.0/.
References
- Bade BC, Dela Cruz CS. Lung Cancer 2020: Epidemiology, Etiology, and Prevention. Clin Chest Med 2020;41:1-24. [Crossref] [PubMed]
- Barta JA, Powell CA, Wisnivesky JP. Global Epidemiology of Lung Cancer. Ann Glob Health 2019;85:8. [Crossref] [PubMed]
- Gheysens G, De Wever W, Cockmartin L, et al. Detection of pulmonary nodules with scoutless fixed-dose ultra-low-dose CT: a prospective study. Eur Radiol 2022;32:4437-45. [Crossref] [PubMed]
- Chen G, Bai T, Wen LJ, et al. Predictive model for the probability of malignancy in solitary pulmonary nodules: a meta-analysis. J Cardiothorac Surg 2022;17:102. [Crossref] [PubMed]
- Mazzone PJ, Gould MK, Arenberg DA, et al. Management of Lung Nodules and Lung Cancer Screening During the COVID-19 Pandemic: CHEST Expert Panel Report. Chest 2020;158:406-15. [Crossref] [PubMed]
- Wu SY, Lazar AA, Gubens MA, et al. Evaluation of a National Comprehensive Cancer Network Guidelines-Based Decision Support Tool in Patients With Non-Small Cell Lung Cancer: A Nonrandomized Clinical Trial. JAMA Netw Open 2020;3:e209750. [Crossref] [PubMed]
- Chen Sihong, Qin Jing, Ji Xing, et al. Automatic Scoring of Multiple Semantic Attributes With Multi-Task Feature Leverage: A Study on Pulmonary Nodules in CT Images. IEEE Trans Med Imaging 2017;36:802-14. [Crossref] [PubMed]
- Feng H, Shi G, Xu Q, et al. Radiomics-based analysis of CT imaging for the preoperative prediction of invasiveness in pure ground-glass nodule lung adenocarcinomas. Insights Imaging 2023;14:24. [Crossref] [PubMed]
- Roh W, Geffen Y, Cha H, et al. High-Resolution Profiling of Lung Adenocarcinoma Identifies Expression Subtypes with Specific Biomarkers and Clinically Relevant Vulnerabilities. Cancer Res 2022;82:3917-31. [Crossref] [PubMed]
- Lambin P, Rios-Velazquez E, Leijenaar R, et al. Radiomics: extracting more information from medical images using advanced feature analysis. Eur J Cancer 2012;48:441-6. [Crossref] [PubMed]
- Sun Y, Li C, Jin L, et al. Radiomics for lung adenocarcinoma manifesting as pure ground-glass nodules: invasive prediction. Eur Radiol 2020;30:3650-9. [Crossref] [PubMed]
- Nicholson AG, Tsao MS, Beasley MB, et al. The 2021 WHO Classification of Lung Tumors: Impact of Advances Since 2015. J Thorac Oncol 2022;17:362-87. [Crossref] [PubMed]
- Khalvati F, Zhang Y, Wong A, et al. Radiomics. Encyclopedia of Biomedical Engineering 2019;2:597-603.
- Ma M, Gan L, Liu Y, et al. Radiomics features based on automatic segmented MRI images: Prognostic biomarkers for triple-negative breast cancer treated with neoadjuvant chemotherapy. Eur J Radiol 2022;146:110095. [Crossref] [PubMed]
- Ma Y, Li J, Xu X, et al. The CT delta-radiomics based machine learning approach in evaluating multiple primary lung adenocarcinoma. BMC Cancer 2022;22:949. [Crossref] [PubMed]
- Xue C, Yuan J, Lo GG, et al. Radiomics feature reliability assessed by intraclass correlation coefficient: a systematic review. Quant Imaging Med Surg 2021;11:4431-60. [Crossref] [PubMed]
- Ren H, Xiao Z, Ling C, et al. Development of a novel nomogram-based model incorporating 3D radiomic signatures and lung CT radiological features for differentiating invasive adenocarcinoma from adenocarcinoma in situ and minimally invasive adenocarcinoma. Quant Imaging Med Surg 2023;13:237-48. [Crossref] [PubMed]
- Xu F, Zhu W, Shen Y, et al. Radiomic-Based Quantitative CT Analysis of Pure Ground-Glass Nodules to Predict the Invasiveness of Lung Adenocarcinoma. Front Oncol 2020;10:872. [Crossref] [PubMed]
- Perez-Johnston R, Araujo-Filho JA, Connolly JG, et al. CT-based Radiogenomic Analysis of Clinical Stage I Lung Adenocarcinoma with Histopathologic Features and Oncologic Outcomes. Radiology 2022;303:664-72. [Crossref] [PubMed]
- She Y, Zhang L, Zhu H, et al. The predictive value of CT-based radiomics in differentiating indolent from invasive lung adenocarcinoma in patients with pulmonary nodules. Eur Radiol 2018;28:5121-8. [Crossref] [PubMed]
- Peng Q, Tang W, Huang Y, et al. Diffusion kurtosis imaging: correlation analysis of quantitative model parameters with molecular features in advanced lung adenocarcinoma. Chin Med J (Engl) 2020;133:2403-9. [Crossref] [PubMed]
- Gao C, Xiang P, Ye J, et al. Can texture features improve the differentiation of infiltrative lung adenocarcinoma appearing as ground glass nodules in contrast-enhanced CT? Eur J Radiol 2019;117:126-31. [Crossref] [PubMed]
- Zhao W, Xu Y, Yang Z, et al. Development and validation of a radiomics nomogram for identifying invasiveness of pulmonary adenocarcinomas appearing as subcentimeter ground-glass opacity nodules. Eur J Radiol 2019;112:161-8. [Crossref] [PubMed]
- Yan M, Wang W. A Non-invasive Method to Diagnose Lung Adenocarcinoma. Front Oncol 2020;10:602. [Crossref] [PubMed]
- Orhan K, Driesen L, Shujaat S, et al. Development and Validation of a Magnetic Resonance Imaging-Based Machine Learning Model for TMJ Pathologies. Biomed Res Int 2021;2021:6656773. [Crossref] [PubMed]
- de Sousa VML, Carvalho L. Heterogeneity in Lung Cancer. Pathobiology 2018;85:96-107. [Crossref] [PubMed]
- Del Gobbo A, Pellegrinelli A, Gaudioso G, et al. Analysis of NSCLC tumour heterogeneity, proliferative and 18F-FDG PET indices reveals Ki67 prognostic role in adenocarcinomas. Histopathology 2016;68:746-51. [Crossref] [PubMed]
- Zhang R, Hu G, Qiu J, et al. Clinical significance of the cribriform pattern in invasive adenocarcinoma of the lung. J Clin Pathol 2019;72:682-8. [Crossref] [PubMed]
- Yin J, Xi J, Liang J, et al. Solid Components in the Mediastinal Window of Computed Tomography Define a Distinct Subtype of Subsolid Nodules in Clinical Stage I Lung Cancers. Clin Lung Cancer 2021;22:324-31. [Crossref] [PubMed]
- Munari E, Zamboni G, Lunardi G, et al. PD-L1 Expression Heterogeneity in Non-Small Cell Lung Cancer: Defining Criteria for Harmonization between Biopsy Specimens and Whole Sections. J Thorac Oncol 2018;13:1113-20. [Crossref] [PubMed]
- Chaunzwa TL, Hosny A, Xu Y, et al. Deep learning classification of lung cancer histology using CT images. Sci Rep 2021;11:5471. [Crossref] [PubMed]
- Nakao M, Omura K, Hashimoto K, et al. Three-dimensional image simulation for lung segmentectomy from unenhanced computed tomography data. Gen Thorac Cardiovasc Surg 2022;70:312-4. [Crossref] [PubMed]
- Zhong Y, Yuan M, Zhang T, et al. Radiomics Approach to Prediction of Occult Mediastinal Lymph Node Metastasis of Lung Adenocarcinoma. AJR Am J Roentgenol 2018;211:109-13. [Crossref] [PubMed]
- Oikonomou A, Salazar P, Zhang Y, et al. Histogram-based models on non-thin section chest CT predict invasiveness of primary lung adenocarcinoma subsolid nodules. Sci Rep 2019;9:6009. [Crossref] [PubMed]
- Cai J, Liu H, Yuan H, et al. A radiomics study to predict invasive pulmonary adenocarcinoma appearing as pure ground-glass nodules. Clin Radiol 2021;76:143-51. [Crossref] [PubMed]
- Liu J, Yang X, Li Y, et al. Development and validation of qualitative and quantitative models to predict invasiveness of lung adenocarcinomas manifesting as pure ground-glass nodules based on low-dose computed tomography during lung cancer screening. Quant Imaging Med Surg 2022;12:2917-31. [Crossref] [PubMed]
- Peikert T, Bartholmai BJ, Maldonado F. Radiomics-based Management of Indeterminate Lung Nodules? Are We There Yet? Am J Respir Crit Care Med 2020;202:165-7. [Crossref] [PubMed]
- Xue X, Yang Y, Huang Q, et al. Use of a Radiomics Model to Predict Tumor Invasiveness of Pulmonary Adenocarcinomas Appearing as Pulmonary Ground-Glass Nodules. Biomed Res Int 2018;2018:6803971. [Crossref] [PubMed]
- Luo T, Xu K, Zhang Z, et al. Radiomic features from computed tomography to differentiate invasive pulmonary adenocarcinomas from non-invasive pulmonary adenocarcinomas appearing as part-solid ground-glass nodules. Chin J Cancer Res 2019;31:329-38. [Crossref] [PubMed]
- Lu J, Ji X, Wang L, et al. Machine Learning-Based Radiomics for Prediction of Epidermal Growth Factor Receptor Mutations in Lung Adenocarcinoma. Dis Markers 2022;2022:2056837. [Crossref] [PubMed]
- Lee SH, Han P, Hales RK, et al. Multi-view radiomics and dosiomics analysis with machine learning for predicting acute-phase weight loss in lung cancer patients treated with radiotherapy. Phys Med Biol 2020;65:195015. [Crossref] [PubMed]
- Yamanashi K, Hamaji M, Murakami K, et al. Prognostic role of preoperative carcinoembryonic antigen level in part-solid lung adenocarcinoma. Asian Cardiovasc Thorac Ann 2022;30:457-67. [Crossref] [PubMed]
- Zhu M, Yang Z, Wang M, et al. A computerized tomography-based radiomic model for assessing the invasiveness of lung adenocarcinoma manifesting as ground-glass opacity nodules. Respir Res 2022;23:96. [Crossref] [PubMed]
- Liu LP, Lu L, Zhao QQ, et al. Identification and Validation of the Pyroptosis-Related Molecular Subtypes of Lung Adenocarcinoma by Bioinformatics and Machine Learning. Front Cell Dev Biol 2021;9:756340. [Crossref] [PubMed]
- She Y, Jin Z, Wu J, et al. Development and Validation of a Deep Learning Model for Non-Small Cell Lung Cancer Survival. JAMA Netw Open 2020;3:e205842. [Crossref] [PubMed]
- Yu Z, Xu C, Zhang Y, et al. A triple-classification for the evaluation of lung nodules manifesting as pure ground-glass sign: a CT-based radiomic analysis. BMC Med Imaging 2022;22:133. [Crossref] [PubMed]
- Yang H, Chen L, Cheng Z, et al. Deep learning-based six-type classifier for lung cancer and mimics from histopathological whole slide images: a retrospective study. BMC Med 2021;19:80. [Crossref] [PubMed]
- Hyun SH, Ahn MS, Koh YW, et al. A Machine-Learning Approach Using PET-Based Radiomics to Predict the Histological Subtypes of Lung Cancer. Clin Nucl Med 2019;44:956-60. [Crossref] [PubMed]
(English Language Editor: J. Jones)